The Future of Health Data Analytics
In today's fast-paced world, the healthcare industry is undergoing a remarkable transformation, driven by the power of health data analytics. Imagine a scenario where doctors can predict health issues before they arise, hospitals can streamline their operations to save time and resources, and patients receive care tailored specifically to their unique needs. This is not just a dream—it's becoming a reality thanks to the innovative use of data analytics in healthcare. As we delve into the future of health data analytics, we'll explore how it's reshaping patient care, enhancing operational efficiency, and paving the way for predictive modeling.
At the core of this revolution is big data. It’s like having a treasure trove of information at our fingertips, allowing healthcare providers to sift through vast amounts of data to uncover insights that were previously hidden. With the right tools, healthcare professionals can analyze patterns and trends that lead to improved patient outcomes and more personalized medicine. Think of it as having a GPS for navigating the complex landscape of healthcare—guiding practitioners to make informed decisions that ultimately benefit patients.
However, the journey into the future of health data analytics is not without its bumps in the road. While the potential is immense, challenges such as data integration, privacy concerns, and ethical considerations must be addressed. As we look ahead, it’s essential to strike a balance between leveraging data for better health outcomes and ensuring that patient rights are protected. This article will take you through the exciting developments in health data analytics, the hurdles we still face, and the promising future that lies ahead.
Big data is revolutionizing healthcare by enabling the analysis of vast amounts of information, leading to improved patient outcomes, personalized medicine, and more efficient healthcare delivery systems. With the advent of electronic health records (EHRs), wearable technology, and mobile health applications, a wealth of data is generated daily. This data, when analyzed effectively, can provide critical insights into patient health trends and treatment efficacy.
Moreover, big data analytics can help healthcare organizations identify patterns that may not be immediately obvious. For instance, analyzing patient demographics alongside treatment outcomes can help reveal disparities in care quality. By understanding these patterns, healthcare providers can implement targeted interventions that ensure equitable care for all patients.
Predictive analytics is one of the most exciting applications of health data analytics. By leveraging historical data, healthcare providers can forecast future health events, allowing them to proactively manage patient care. Imagine a world where a doctor can predict a patient's risk of developing diabetes based on their lifestyle choices and family history. This proactive approach not only reduces hospital readmissions but also enhances overall health outcomes.
Machine learning algorithms are increasingly being used to analyze health data, uncover patterns, and support clinical decision-making. These algorithms can process vast datasets far more quickly than a human ever could, identifying trends that allow healthcare professionals to make informed decisions. For example, a machine learning model might analyze thousands of patient records to determine which treatments are most effective for specific conditions, ultimately enhancing the accuracy and efficiency of healthcare services.
Natural Language Processing (NLP) technologies are transforming how health records are analyzed. NLP enables the extraction of valuable insights from unstructured data such as physician notes and patient communications. This means that healthcare providers can gain a deeper understanding of patient needs and experiences, leading to more personalized care. Imagine being able to analyze a doctor's handwritten notes for trends in patient symptoms—NLP makes this possible.
Real-time data monitoring tools provide healthcare professionals with immediate access to patient information. This capability facilitates timely interventions and improves patient safety and care quality. For instance, if a patient's vital signs indicate distress, healthcare providers can be alerted instantly, allowing them to take action before a situation escalates. It’s like having a watchful guardian, always ready to respond to a patient’s needs.
Despite the advancements, integrating diverse health data sources remains a significant challenge. Disparate systems and data formats can hinder the seamless flow of information essential for effective analytics. Think of it as trying to fit puzzle pieces from different boxes together—without the right tools, it can be frustrating and time-consuming. Overcoming these integration challenges is crucial for maximizing the potential of health data analytics.
As health data analytics evolves, regulatory and ethical considerations become paramount. Ensuring patient privacy, data security, and compliance with healthcare laws is essential while still promoting innovation. The healthcare industry must navigate these complex waters carefully to maintain trust and integrity.
Robust data privacy and security measures are essential to protect sensitive health information. Maintaining patient trust is critical, and adherence to regulations like HIPAA and GDPR is non-negotiable. Healthcare providers must implement stringent security protocols to safeguard data from breaches and unauthorized access, ensuring that patients feel secure in sharing their information.
The ethical use of health data involves addressing concerns related to consent, bias in algorithms, and the potential for misuse. It’s crucial to foster a responsible approach to data analytics in healthcare. By prioritizing ethical considerations, we can ensure that health data analytics serves its intended purpose—improving patient care and outcomes.
- What is health data analytics? Health data analytics refers to the process of collecting, analyzing, and interpreting health-related data to improve patient care and operational efficiency.
- How does big data impact healthcare? Big data allows healthcare providers to analyze vast amounts of information, leading to improved patient outcomes and more personalized care.
- What are the challenges of health data analytics? Key challenges include data integration, privacy concerns, and ethical considerations.
- How can predictive analytics benefit patient care? Predictive analytics can help healthcare providers anticipate patient needs and manage care proactively, reducing hospital readmissions and enhancing health outcomes.

The Rise of Big Data in Healthcare
In today's fast-paced world, big data is not just a buzzword; it's a game-changer in the healthcare industry. Imagine having access to a treasure trove of information that can significantly improve patient outcomes and streamline operations. That's precisely what big data offers. With the ability to analyze vast amounts of information from various sources, healthcare providers are now equipped to make informed decisions that enhance patient care and operational efficiency.
The healthcare sector is experiencing a seismic shift thanks to the integration of big data analytics. This transformation is driven by several factors, including the proliferation of electronic health records (EHRs), advancements in technology, and the increasing availability of health-related data from wearable devices and mobile applications. Personalized medicine is becoming a reality, as healthcare professionals can tailor treatments based on individual patient data, leading to better health outcomes.
One of the most exciting aspects of big data in healthcare is its ability to identify patterns and trends that were previously invisible. For instance, by analyzing patient demographics, treatment histories, and outcomes, healthcare providers can uncover insights that help them understand disease progression and treatment effectiveness. This can lead to the early detection of potential health issues, allowing for timely interventions that can save lives.
However, the rise of big data is not without its challenges. The sheer volume of data generated can be overwhelming, and integrating diverse data sources often poses significant hurdles. Data silos—where information is trapped within separate systems—can impede the flow of information, making it difficult for healthcare providers to access the comprehensive data they need for effective analysis. To address this, organizations are investing in advanced analytics platforms that can seamlessly integrate data from various sources, ensuring a holistic view of patient health.
Furthermore, big data analytics is paving the way for improved operational efficiency. By analyzing workflow patterns and resource utilization, healthcare organizations can identify bottlenecks and streamline processes. This not only reduces costs but also enhances the patient experience. For example, hospitals can optimize staffing levels based on predictive analytics, ensuring that the right number of healthcare professionals are available at peak times.
In conclusion, the rise of big data in healthcare is transforming the industry in unprecedented ways. As technology continues to advance, we can expect even more innovative applications of data analytics that will further enhance patient care and operational efficiency. The potential for big data to revolutionize healthcare is immense, and those who embrace it will likely lead the charge toward a healthier future.
- What is big data in healthcare? Big data in healthcare refers to the vast amounts of structured and unstructured data generated from various sources, including electronic health records, medical imaging, and patient monitoring devices.
- How does big data improve patient care? By analyzing large datasets, healthcare providers can identify trends, predict outcomes, and tailor treatments to individual patients, leading to improved health outcomes.
- What are the challenges of implementing big data analytics? Challenges include data integration, ensuring data privacy and security, and the need for skilled personnel to analyze and interpret the data.

Predictive Analytics: Anticipating Patient Needs
In the rapidly evolving landscape of healthcare, predictive analytics stands out as a game-changer, allowing providers to not just react to patient needs but to anticipate them. Imagine walking into a doctor’s office where your physician already knows your health risks, your likely future conditions, and even potential treatment paths—all before you’ve said a word. This is the power of predictive analytics, and it’s reshaping the way we approach patient care.
At its core, predictive analytics involves the use of historical health data to forecast future health events. By analyzing patterns from past patient interactions, healthcare providers can identify at-risk populations and tailor interventions accordingly. For example, if a hospital notices a trend of increased readmissions among patients with certain chronic conditions, they can proactively implement targeted programs to manage those patients better. This not only improves patient outcomes but also significantly reduces costs associated with unnecessary hospital visits.
One of the most exciting aspects of predictive analytics is its ability to leverage machine learning algorithms. These algorithms sift through mountains of data, uncovering hidden patterns that human analysts might miss. Think of it as having a super-intelligent assistant that not only keeps track of your medical history but also learns from it, continually improving its predictions. This technology can help healthcare professionals make informed decisions, leading to timely interventions and better patient management.
Moreover, predictive analytics can be instrumental in reducing hospital readmissions. By identifying patients at higher risk of returning to the hospital, care teams can develop personalized follow-up plans. For instance, a patient recovering from heart surgery may benefit from home health visits or telehealth check-ins to monitor their recovery. This proactive approach not only enhances the quality of care but also fosters a sense of trust and security among patients.
However, the implementation of predictive analytics is not without its challenges. Data quality is paramount; if the data fed into predictive models is incomplete or inaccurate, the predictions themselves will be flawed. Healthcare organizations must invest in robust data collection and management systems to ensure that they are working with the best possible information. Additionally, integrating data from various sources—like electronic health records, lab results, and even wearable health devices—can be a complex task that requires careful planning and execution.
As we look to the future, the potential of predictive analytics in healthcare is vast. Here are a few key areas where we can expect to see significant advancements:
- Chronic Disease Management: Enhanced monitoring and personalized care plans for conditions like diabetes and hypertension.
- Emergency Response: Improved systems for predicting surges in patient volume during crises, enabling better resource allocation.
- Population Health: More effective public health strategies through the analysis of community health trends.
In conclusion, predictive analytics is not just a technological advancement; it’s a fundamental shift in how healthcare is delivered. By anticipating patient needs, healthcare providers can create a more personalized, efficient, and effective care experience. The future is bright, and with continued investment in this area, we can look forward to a healthcare system that is not only reactive but also proactive in its approach to patient care.
- What is predictive analytics in healthcare? Predictive analytics in healthcare refers to the use of historical data and statistical algorithms to forecast future health events, allowing for proactive patient management.
- How does predictive analytics improve patient outcomes? By identifying at-risk patients and tailoring interventions, predictive analytics helps reduce hospital readmissions and enhances overall patient care.
- What challenges does predictive analytics face? Key challenges include ensuring data quality, integrating diverse data sources, and addressing privacy concerns.

Machine Learning Applications
This article explores the transformative impact of health data analytics on patient care, operational efficiency, and predictive modeling, highlighting trends, challenges, and future directions in the healthcare industry.
Big data is revolutionizing healthcare by enabling the analysis of vast amounts of information, leading to improved patient outcomes, personalized medicine, and more efficient healthcare delivery systems.
Predictive analytics leverages historical data to forecast future health events, allowing healthcare providers to proactively manage patient care, reduce hospital readmissions, and enhance overall health outcomes.
Machine learning (ML) is like giving a computer the ability to learn from data, enhancing its ability to make decisions without being explicitly programmed. In the healthcare sector, this technology is making waves by analyzing complex datasets and uncovering patterns that were previously hidden. Imagine a detective piecing together clues; that’s how machine learning algorithms work with health data.
One of the primary applications of machine learning in healthcare is in the realm of diagnostics. Algorithms can analyze medical images, such as X-rays and MRIs, identifying anomalies with remarkable accuracy. For instance, a study found that ML models can detect certain cancers earlier than human radiologists, significantly improving patient outcomes.
Moreover, ML is instrumental in predictive analytics. By examining historical patient data, these algorithms can forecast potential health issues before they arise. For example, they can predict which patients are at risk of developing chronic conditions like diabetes or heart disease based on their medical history, lifestyle, and even genetic information. This proactive approach allows healthcare providers to intervene early, potentially saving lives.
Additionally, machine learning is transforming personalized medicine. By analyzing a patient’s unique genetic makeup, treatment plans can be tailored specifically to the individual, enhancing the effectiveness of therapies. This is akin to having a custom-made suit; it fits perfectly and meets the specific needs of the wearer.
However, the integration of machine learning into healthcare isn’t without its challenges. The quality of the data used is paramount. If the data is biased or incomplete, the algorithms can produce skewed results, leading to misdiagnoses or ineffective treatment plans. Therefore, ensuring high-quality, diverse datasets is crucial for the success of ML applications in healthcare.
In summary, the applications of machine learning in healthcare are vast and varied, from enhancing diagnostics to enabling personalized treatment plans. As technology continues to evolve, the integration of machine learning will undoubtedly reshape the landscape of healthcare, making it more efficient and patient-centered.
Natural language processing (NLP) technologies are transforming how health records are analyzed, enabling the extraction of valuable insights from unstructured data such as physician notes and patient communications.
Real-time data monitoring tools provide healthcare professionals with immediate access to patient information, facilitating timely interventions and improving patient safety and care quality.
Integrating diverse health data sources remains a significant challenge, as disparate systems and data formats can hinder the seamless flow of information essential for effective analytics.
As health data analytics evolves, regulatory and ethical considerations become paramount, ensuring patient privacy, data security, and compliance with healthcare laws while promoting innovation.
Robust data privacy and security measures are essential to protect sensitive health information, maintaining patient trust and adhering to regulations like HIPAA and GDPR.
The ethical use of health data involves addressing concerns related to consent, bias in algorithms, and the potential for misuse, fostering a responsible approach to data analytics in healthcare.
- What is health data analytics? Health data analytics refers to the process of collecting, analyzing, and interpreting health-related data to improve patient care and operational efficiency.
- How does machine learning benefit healthcare? Machine learning enhances diagnostics, enables predictive analytics, and supports personalized medicine, ultimately improving patient outcomes.
- What are the challenges in implementing health data analytics? Key challenges include data integration, ensuring data quality, and addressing regulatory and ethical considerations.
- How is patient privacy protected in health data analytics? Patient privacy is safeguarded through robust data security measures and adherence to regulations such as HIPAA and GDPR.

Natural Language Processing in Health Records
Natural Language Processing (NLP) is a fascinating field that bridges the gap between human language and computer understanding. In the context of healthcare, NLP is becoming a game changer, enabling the extraction of valuable insights from unstructured data found in health records. Think about all the notes doctors jot down during patient visits, the myriad of conversations between healthcare providers, and even the feedback from patients themselves. Traditionally, this treasure trove of information was left largely untapped, but with NLP, we're unlocking a wealth of knowledge that can significantly enhance patient care.
One of the most exciting aspects of NLP in health records is its ability to analyze free-text data. This means that instead of only relying on structured data—like numbers and checkboxes—NLP can sift through lengthy physician notes, radiology reports, and discharge summaries to find critical information. This capability not only saves time but also improves accuracy, allowing healthcare professionals to make better-informed decisions. For example, an NLP system can identify key symptoms or medications mentioned in a patient's record, providing a quick overview that aids in diagnosis and treatment planning.
Moreover, NLP can enhance clinical workflows by automating routine tasks. Imagine a scenario where a doctor no longer needs to manually input data into electronic health records (EHRs). Instead, an NLP system automatically captures relevant information from patient interactions and populates the EHR. This not only reduces the administrative burden on healthcare providers but also minimizes the risk of errors that can occur during manual data entry.
However, the implementation of NLP in health records is not without its challenges. Data privacy and security are paramount, especially when dealing with sensitive patient information. Healthcare organizations must ensure that any NLP tools they deploy comply with regulations like HIPAA and GDPR, safeguarding patient data while still reaping the benefits of advanced analytics. Additionally, there is the issue of algorithmic bias. If the data used to train NLP models is skewed or unrepresentative, it could lead to inaccuracies in patient assessments or treatment recommendations.
Despite these challenges, the potential benefits of NLP in healthcare are immense. As technology continues to evolve, we can expect to see more sophisticated NLP applications that will not only enhance the efficiency of healthcare delivery but also improve patient outcomes. In a world where every second counts, having real-time access to insightful data can be the difference between a good and great healthcare experience.
- What is Natural Language Processing? NLP is a branch of artificial intelligence that focuses on the interaction between computers and humans through natural language.
- How does NLP benefit healthcare? NLP helps in extracting meaningful information from unstructured data, automating administrative tasks, and improving clinical decision-making.
- Are there any challenges associated with NLP in health records? Yes, challenges include data privacy concerns, algorithmic bias, and the need for compliance with healthcare regulations.

Real-Time Data Monitoring
In today’s fast-paced healthcare environment, has emerged as a game-changer. Imagine a world where healthcare professionals can access critical patient information instantly, allowing for immediate interventions. This is not just a dream; it’s a reality made possible by advancements in technology. Real-time data monitoring tools equip healthcare providers with the ability to track patient vitals, medication adherence, and even lifestyle choices as they happen. This capability is akin to having a personal health assistant that never sleeps, always alert to any changes in a patient's condition.
The impact of real-time monitoring on patient care is profound. For instance, consider a patient with chronic heart disease. With continuous monitoring, healthcare professionals can detect irregular heartbeats or sudden changes in blood pressure as they occur. This allows for timely interventions that can prevent serious complications, such as heart attacks or strokes. Furthermore, the integration of these monitoring systems with electronic health records (EHR) means that all relevant data is automatically updated, providing a comprehensive view of the patient's health status.
Additionally, real-time data monitoring fosters a more proactive approach to healthcare. Instead of waiting for patients to report issues, providers can identify potential problems before they escalate. This not only improves patient outcomes but also enhances overall operational efficiency within healthcare facilities. For example, hospitals can better allocate resources based on real-time data insights, ensuring that staff and equipment are available where they are needed most.
However, the implementation of real-time monitoring systems is not without its challenges. Data overload can occur when healthcare providers are inundated with information, making it difficult to discern what is truly important. To combat this, many systems utilize advanced analytics to filter and prioritize data, presenting healthcare professionals with the most relevant information at a glance. This is similar to having a personal assistant who sorts through mountains of paperwork to deliver only the essential documents to you.
Moreover, the integration of real-time monitoring tools with mobile devices and wearables has revolutionized patient engagement. Patients can now track their health metrics from the comfort of their homes, receiving alerts and reminders that encourage them to stay on top of their health. This shift not only empowers patients but also creates a collaborative atmosphere where healthcare providers and patients work together towards better health outcomes.
In conclusion, real-time data monitoring represents a significant leap forward in the healthcare industry. By enabling immediate access to patient information, it enhances the quality of care, promotes proactive health management, and improves operational efficiency. As technology continues to evolve, we can expect even more innovative solutions that will further transform how healthcare is delivered.
- What is real-time data monitoring in healthcare? Real-time data monitoring refers to the continuous tracking of patient health metrics, allowing healthcare providers to access critical information instantly and make timely decisions.
- How does real-time monitoring improve patient care? It allows for immediate interventions when changes in a patient's condition are detected, which can prevent complications and enhance overall health outcomes.
- What technologies are used for real-time data monitoring? Technologies include wearables, mobile health applications, and integrated electronic health record systems that provide real-time updates on patient data.
- What challenges does real-time data monitoring face? Challenges include data overload, integration with existing systems, and ensuring patient privacy and security.

Challenges in Data Integration
Integrating diverse health data sources remains a significant challenge in the healthcare industry. With the rapid growth of digital health solutions, hospitals and clinics are inundated with a plethora of data from various platforms, including electronic health records (EHRs), wearable devices, and telehealth services. This data, while rich in potential insights, often exists in silos, making it difficult for healthcare providers to access and analyze the information cohesively. Imagine trying to solve a jigsaw puzzle, but half of the pieces are missing or belong to different sets; that's the current state of health data integration.
One of the primary hurdles in achieving seamless data integration is the disparity in data formats and standards. Different systems may use various coding languages, terminologies, and structures, leading to inconsistencies that complicate the merging of information. For instance, one hospital might record a patient's allergy to penicillin in a specific format, while another may use a different term altogether. This lack of uniformity can hinder the ability to perform comprehensive analyses and make informed decisions.
Moreover, the integration process is often hampered by legacy systems that are not designed to communicate with newer technologies. Many healthcare organizations still rely on outdated software that lacks the flexibility to adapt to modern data integration needs. This technological gap can lead to delays in accessing critical patient data, ultimately affecting the quality of care provided. In fact, studies have shown that inefficient data integration can contribute to increased healthcare costs and poor patient outcomes.
Another significant challenge is ensuring data accuracy and quality. When integrating multiple data sources, there is a risk of introducing errors or duplicating information, which can skew analysis results. Healthcare providers must invest time and resources into data cleansing and validation processes to ensure that the information they are working with is reliable. This can be a daunting task, especially when dealing with vast amounts of data collected from various points of care.
Despite these challenges, the potential benefits of effective data integration are immense. By overcoming these obstacles, healthcare organizations can unlock valuable insights that lead to improved patient outcomes, enhanced operational efficiency, and more personalized care. It’s essential for stakeholders to collaborate on developing standardized protocols and investing in technologies that facilitate seamless data sharing. After all, in the world of healthcare, every piece of data counts.
- What are the main challenges in health data integration?
The main challenges include disparate data formats, legacy systems, data accuracy, and quality issues. - Why is data integration important in healthcare?
Effective data integration is crucial for improving patient outcomes, operational efficiency, and providing personalized care. - How can healthcare organizations overcome integration challenges?
Organizations can overcome challenges by collaborating on standardized protocols and investing in modern integration technologies.

Regulatory and Ethical Considerations
As we venture deeper into the world of health data analytics, the importance of cannot be overstated. These factors serve as the backbone of trust between patients and healthcare providers, ensuring that sensitive information remains protected while fostering innovation in the field. With the rapid evolution of technology, it is crucial to strike a balance between leveraging data for improved patient outcomes and safeguarding individual privacy.
One of the primary regulatory frameworks governing health data is the Health Insurance Portability and Accountability Act (HIPAA) in the United States. HIPAA sets strict guidelines on how personal health information (PHI) should be handled, emphasizing the need for confidentiality and security. Compliance with these regulations is not just a legal obligation; it is essential for maintaining patient trust. Healthcare organizations must invest in robust data security measures to protect sensitive information from breaches and unauthorized access.
Another significant regulation is the General Data Protection Regulation (GDPR), which applies to organizations operating within the European Union. GDPR mandates that organizations must obtain explicit consent from individuals before collecting or processing their data. This regulation highlights the importance of transparency and accountability in data practices. As healthcare providers increasingly rely on data analytics, understanding and adhering to these regulations is paramount to ensuring ethical practices.
Moreover, the ethical use of health data extends beyond mere compliance with laws. It involves a commitment to responsible data stewardship. This means addressing concerns related to consent, particularly in cases where data is used for research or analytics without direct patient involvement. Organizations must ensure that patients are fully informed about how their data will be used, empowering them to make educated decisions about their health information.
In addition to consent, there is a growing concern regarding bias in algorithms used for data analytics. If the data used to train these algorithms is not representative of the diverse patient population, it can lead to disparities in healthcare outcomes. For example, if an algorithm is primarily trained on data from one demographic group, it may not perform effectively for others, potentially exacerbating existing health inequalities. Thus, healthcare organizations must actively work towards creating inclusive datasets that reflect the diversity of the populations they serve.
Lastly, the potential for misuse of health data is a pressing issue. With the rise of data breaches and cyber threats, there is a real risk that sensitive health information could be exploited for malicious purposes. To combat this, healthcare organizations must implement comprehensive data governance frameworks that include regular audits, employee training, and incident response plans. Such measures not only protect patient data but also enhance the overall integrity of healthcare systems.
In summary, as health data analytics continues to evolve, the regulatory and ethical landscape must keep pace. By prioritizing patient privacy, adhering to established laws, and fostering a culture of ethical data use, healthcare organizations can harness the power of analytics while maintaining the trust and confidence of their patients. The journey towards effective and responsible health data analytics is ongoing, and it is a shared responsibility among all stakeholders in the healthcare ecosystem.
- What is HIPAA and why is it important? HIPAA is a U.S. law that protects patient health information. It is crucial for maintaining patient confidentiality and trust in the healthcare system.
- How does GDPR affect healthcare data practices? GDPR requires organizations to obtain explicit consent from individuals before processing their data, emphasizing transparency and accountability.
- What are the risks of biased algorithms in healthcare? Biased algorithms can lead to disparities in healthcare outcomes, particularly if they are trained on non-representative data.
- What measures can organizations take to protect health data? Organizations should implement robust data governance frameworks, conduct regular audits, and train employees on data security best practices.
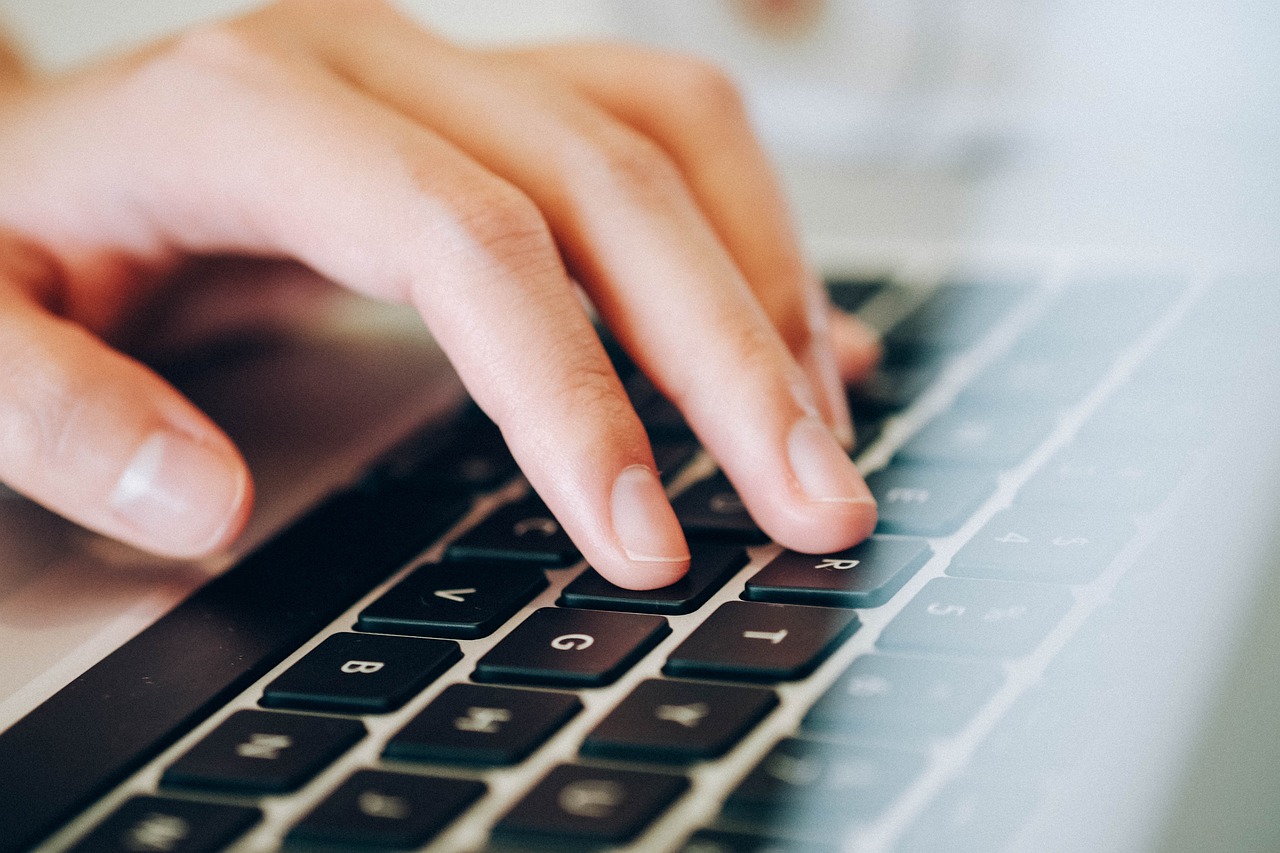
Data Privacy and Security Measures
In the rapidly evolving landscape of health data analytics, data privacy and security measures are not just afterthoughts; they are the very foundation upon which patient trust and healthcare innovation stand. With the increasing digitization of health records and the rise of big data analytics, the potential for sensitive information to be compromised is a pressing concern. Imagine your health data being exposed—it's not just numbers and statistics; it’s your personal story, your health journey, and your future. This is why robust security protocols are essential.
Healthcare organizations are now required to implement comprehensive data protection strategies that encompass everything from encryption to access controls. For instance, encryption ensures that even if data is intercepted, it remains unreadable without the correct decryption key. This is akin to locking your valuable possessions in a safe; even if someone breaks in, they can't access what’s inside without the combination. Additionally, access controls allow organizations to regulate who can view or manipulate sensitive health information, thereby minimizing the risk of internal breaches.
Moreover, adherence to regulations like HIPAA (Health Insurance Portability and Accountability Act) in the United States and GDPR (General Data Protection Regulation) in Europe is crucial. These laws not only set stringent guidelines for data handling but also impose heavy penalties for non-compliance. To give you a clearer picture, here’s a comparison of some key aspects of these regulations:
Aspect | HIPAA | GDPR |
---|---|---|
Scope | Healthcare entities in the US | All organizations processing personal data in the EU |
Data Protection Officer | Not mandatory | Mandatory for certain organizations |
Patient Rights | Access to records, request corrections | Right to be forgotten, data portability |
Penalties for Non-Compliance | Up to $1.5 million per violation | Up to 4% of annual global turnover |
In addition to regulatory compliance, healthcare organizations must also foster a culture of data ethics. This means being transparent with patients about how their data is used and ensuring that consent is obtained before any data is shared or analyzed. It’s crucial to address potential biases in algorithms that could lead to unequal treatment or outcomes. By prioritizing ethical considerations, organizations can build a framework that not only protects data but also respects the rights and dignity of patients.
To summarize, the integration of effective data privacy and security measures is paramount in the realm of health data analytics. By employing advanced technologies, adhering to strict regulations, and fostering ethical practices, healthcare organizations can safeguard sensitive information while simultaneously promoting innovation. The goal is clear: to create a healthcare environment where data is not just a tool for improvement, but a trusted ally in the journey toward better health outcomes.
- What is HIPAA? HIPAA stands for the Health Insurance Portability and Accountability Act, which sets standards for protecting sensitive patient information.
- How does GDPR affect healthcare organizations? GDPR imposes strict guidelines on how personal data is processed and gives patients greater control over their information.
- What are some common data security measures? Common measures include encryption, access controls, regular audits, and employee training on data protection.
- Why is patient consent important? Patient consent is crucial as it ensures that individuals are aware of and agree to how their data will be used, promoting trust in healthcare systems.

Ethical Use of Health Data
The ethical use of health data is a critical aspect of healthcare analytics that cannot be overlooked. As the industry continues to embrace the power of data, it is essential to navigate the complex landscape of ethics surrounding patient information. After all, health data is not just numbers and statistics; it represents real individuals with unique stories, vulnerabilities, and rights. So, how do we ensure that this data is used responsibly and ethically?
One of the foremost considerations is obtaining informed consent from patients. Individuals should be fully aware of how their data will be used, who it will be shared with, and the potential implications of its use. This transparency fosters trust between patients and healthcare providers, which is paramount in maintaining a healthy relationship. Imagine walking into a clinic and feeling confident that your personal information is in safe hands—this is the kind of trust we should aim for.
Moreover, the potential for bias in algorithms is another ethical concern that cannot be ignored. If the data used to train machine learning models is skewed or unrepresentative, the resulting insights may perpetuate existing disparities in healthcare. For instance, if a predictive model is primarily trained on data from a specific demographic, it may fail to accurately predict health outcomes for other groups. This could lead to unequal treatment and exacerbate health inequalities. Therefore, it is vital to ensure that the data sets used are diverse and inclusive, reflecting the population's true makeup.
Additionally, there is the risk of misuse of health data, whether intentional or accidental. Organizations must implement robust data governance frameworks to mitigate this risk. This includes establishing strict access controls, regular audits, and comprehensive training for staff on data handling and privacy regulations. By creating a culture of accountability and vigilance, healthcare organizations can safeguard against potential breaches and misuse.
Another critical aspect of ethical data use involves balancing innovation with patient rights. While the potential for groundbreaking advancements in healthcare through data analytics is immense, it should never come at the expense of patient privacy. Regulations such as HIPAA in the United States and GDPR in Europe set forth guidelines that organizations must follow to protect patient information. Compliance is not just a legal obligation; it is a moral imperative to respect patients' rights and dignity.
In conclusion, the ethical use of health data is a multifaceted challenge that requires ongoing dialogue and action. By prioritizing informed consent, addressing algorithmic bias, preventing misuse, and adhering to regulatory standards, we can harness the power of health data analytics while upholding the highest ethical standards. After all, the ultimate goal is to improve patient care and outcomes, and this can only be achieved through responsible and ethical practices.
- What is informed consent in health data analytics?
Informed consent refers to the process by which patients are made aware of how their data will be used, allowing them to make an educated decision about whether to participate. - How can bias in algorithms affect healthcare?
Bias in algorithms can lead to unequal treatment and health disparities, as models trained on skewed data may not accurately represent the broader population. - What are the main regulations governing health data use?
Key regulations include HIPAA in the United States, which protects patient privacy, and GDPR in Europe, which governs data protection and privacy.
Frequently Asked Questions
- What is health data analytics?
Health data analytics refers to the process of examining and interpreting large sets of healthcare data to improve patient outcomes, enhance operational efficiency, and support clinical decision-making. It leverages advanced technologies like big data, machine learning, and predictive analytics to extract valuable insights from various data sources.
- How does big data impact patient care?
Big data significantly enhances patient care by enabling healthcare providers to analyze vast amounts of information, leading to personalized medicine and improved treatment plans. By understanding patient histories and trends, providers can tailor their approaches, resulting in better health outcomes.
- What role does predictive analytics play in healthcare?
Predictive analytics uses historical data to forecast future health events, allowing healthcare professionals to anticipate patient needs proactively. This approach helps in reducing hospital readmissions and enhances overall health management, making it a crucial tool in modern healthcare.
- Can you explain the use of machine learning in health data?
Machine learning algorithms analyze health data to identify patterns and trends that might not be immediately apparent. By supporting clinical decision-making, these algorithms enhance the accuracy of diagnoses and treatment plans, ultimately improving the efficiency of healthcare services.
- What is natural language processing (NLP) in health records?
NLP is a technology that allows the analysis of unstructured data, such as physician notes and patient communications. By extracting meaningful insights from this data, NLP helps healthcare providers make informed decisions and improve patient care.
- What are the challenges in integrating health data?
Integrating diverse health data sources can be challenging due to the existence of disparate systems and varying data formats. These issues can hinder the seamless flow of information, which is essential for effective analytics and improved patient care.
- What are the regulatory considerations in health data analytics?
As health data analytics evolves, regulatory considerations such as patient privacy, data security, and compliance with laws like HIPAA and GDPR become increasingly important. These regulations ensure that sensitive health information is protected while promoting innovation in the field.
- How can healthcare organizations ensure data privacy and security?
Healthcare organizations can ensure data privacy and security by implementing robust measures such as encryption, access controls, and regular audits. These practices help maintain patient trust and ensure compliance with relevant regulations.
- What ethical concerns are associated with health data analytics?
Ethical concerns in health data analytics include issues related to patient consent, potential bias in algorithms, and the risk of data misuse. Addressing these concerns is crucial for fostering a responsible approach to health data analytics and ensuring that patient rights are respected.