How AI is Transforming Data Management
Artificial Intelligence (AI) is not just a buzzword; it's a game changer that is redefining the way we manage data. In an era where data is as valuable as gold, the ability to harness it effectively can make or break a business. Imagine trying to find a needle in a haystack—this is what traditional data management feels like without the power of AI. By automating processes and enhancing accuracy, AI is transforming data management from a cumbersome task into a streamlined, efficient operation. But how exactly is AI making waves in this field? Let’s dive deeper into the various facets of AI's impact on data management.
Data collection has traditionally been a labor-intensive process, often marred by human error and inefficiency. Enter AI technologies, which are revolutionizing this aspect by automating data gathering and improving accuracy across the board. From chatbots that gather customer feedback to sensors that collect real-time data in manufacturing, AI tools are enhancing data collection methods across various industries. For instance, businesses can now utilize AI-driven platforms that not only collect data but also analyze it on the fly, providing real-time insights that were previously unimaginable.
Once data is collected, the next step is to ensure that it’s clean and ready for analysis. This is where AI really shines. By leveraging machine learning algorithms, organizations can identify and rectify inconsistencies in their datasets. Imagine trying to bake a cake with spoiled ingredients; similarly, using flawed data can lead to disastrous outcomes in decision-making. AI tools can automatically flag errors, suggest corrections, and even prepare data in a way that makes it easily digestible for analysis.
One of the significant advantages of AI in data management is its ability to perform automated data validation. This means that before any analysis takes place, the data is verified for accuracy and integrity. Techniques such as anomaly detection and pattern recognition are employed to ensure that the datasets are reliable. By reducing human intervention, organizations can significantly lower the chances of errors that could skew results.
Machine learning models are increasingly being utilized for data validation tasks. These models can learn from historical data to predict and identify potential errors in new datasets. For example, a machine learning algorithm could analyze past sales data to determine what constitutes a normal transaction. If a new transaction deviates significantly from this norm, the algorithm flags it for review, ensuring that only high-quality data is used for analysis.
In today's fast-paced business environment, real-time data monitoring is crucial. AI enables continuous oversight of data integrity, allowing organizations to catch issues before they escalate. Imagine having a security camera that not only records but also alerts you when something unusual happens. This is what AI does for data management—it provides a safety net that helps businesses maintain the quality of their data.
AI technologies are also revolutionizing data storage solutions. With the exponential growth of data, traditional storage methods are becoming obsolete. AI optimizes space and retrieval processes, making it easier for organizations to manage large volumes of data efficiently. For instance, AI algorithms can analyze usage patterns to determine which data is accessed most frequently, allowing companies to prioritize storage resources accordingly.
Once the data is collected, cleaned, and stored, the next step is analysis. AI-powered analytics tools provide deeper insights into complex datasets, enabling organizations to make informed decisions. Techniques such as predictive analytics and natural language processing (NLP) are at the forefront of this transformation. With AI, businesses can sift through mountains of data to uncover trends and insights that would be impossible to detect manually.
Predictive analytics, facilitated by AI, allows businesses to forecast trends and behaviors. This capability is invaluable for strategic planning and decision-making. For example, retailers can predict which products will be in demand based on historical sales data, allowing them to optimize inventory levels. This not only enhances customer satisfaction but also improves profit margins.
Natural Language Processing (NLP) is another exciting application of AI in data management. NLP enables machines to interpret human language, making data analysis more accessible. For instance, businesses can analyze customer feedback from social media or reviews to gauge public sentiment. This capability allows organizations to extract actionable insights from unstructured data, leading to more informed decision-making.
Despite its numerous benefits, the integration of AI in data management is not without challenges. Issues such as data privacy, bias, and integration hurdles can complicate the implementation of AI technologies. Organizations must navigate these challenges carefully to ensure that they are not only compliant with regulations but also fair in their data practices.
- What is AI's role in data management? AI automates tasks, improves accuracy, and enables real-time insights, significantly enhancing data management processes.
- How does AI improve data cleaning? AI uses machine learning algorithms to identify inconsistencies and rectify errors in datasets, ensuring high-quality data for analysis.
- What are the challenges of implementing AI in data management? Challenges include data privacy concerns, potential bias in algorithms, and integration issues with existing systems.
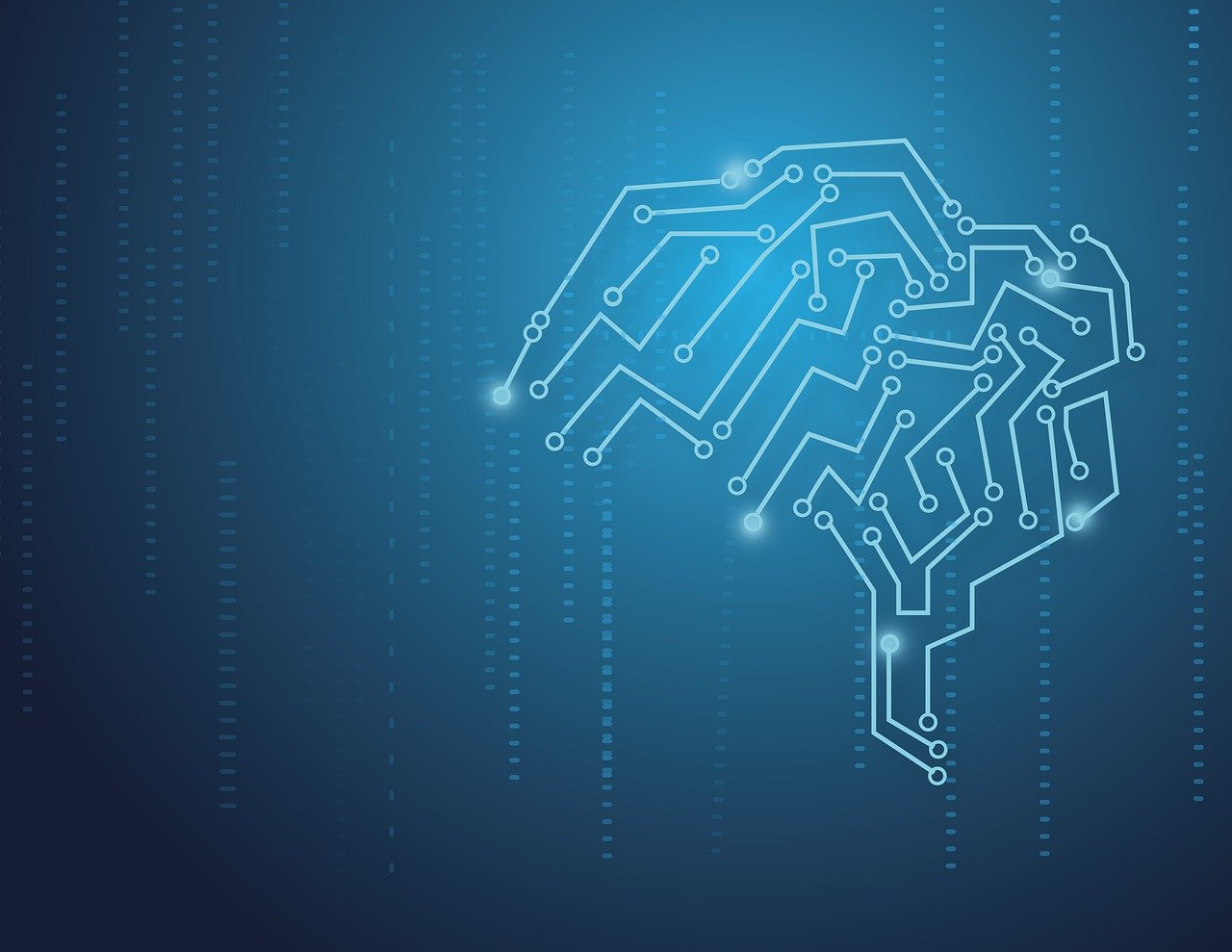
The Role of AI in Data Collection
In today's fast-paced digital world, the significance of data collection cannot be overstated. With the sheer volume of data generated every second, traditional methods of gathering information often fall short. This is where artificial intelligence (AI) steps in, revolutionizing the way we collect and process data. Imagine having a team of tireless assistants who can sift through mountains of information, identifying valuable insights while eliminating human error. Sounds like a dream, right? Well, it’s a reality thanks to AI technologies.
AI enhances data collection processes by automating repetitive tasks, which not only saves time but also improves accuracy. For instance, industries ranging from healthcare to finance are leveraging AI tools to gather data more efficiently. In healthcare, AI systems can analyze patient records and collect data on treatment outcomes, which can be invaluable for research and improving patient care. Similarly, in finance, AI can track market trends and consumer behaviors, providing businesses with real-time insights that were previously unattainable.
One of the most exciting aspects of AI in data collection is its ability to enable real-time insights. Traditional data collection methods often involve delays, which can lead to missed opportunities. However, AI algorithms can process incoming data streams instantly, allowing organizations to react swiftly to changes in the market. For example, retailers can adjust their inventory based on real-time sales data, ensuring they meet customer demand without overstocking.
Moreover, AI can enhance the quality of data collected through advanced algorithms that filter out noise and irrelevant information. This means that businesses can focus on the data that truly matters. Consider a scenario where a company is collecting feedback from customers. An AI-driven tool can analyze responses, categorize sentiments, and identify key themes, providing a clear picture of customer satisfaction without the need for manual sorting.
To illustrate the transformative impact of AI on data collection, let's take a look at some of the AI tools currently making waves across industries:
Industry | AI Tool | Functionality |
---|---|---|
Healthcare | IBM Watson | Analyzes patient data for better treatment recommendations |
Finance | Alteryx | Automates data preparation and predictive analytics |
Retail | Google Analytics | Tracks customer behavior and sales trends in real-time |
As we continue to embrace AI in data collection, it’s important to recognize the potential for innovation. The ability to gather and analyze data efficiently not only enhances operational efficiency but also opens doors to new business models and strategies. The question is, are organizations ready to harness this power? As we explore further, it becomes clear that the integration of AI into data collection is just the beginning of a much larger transformation in how we manage and utilize data.
In conclusion, the role of AI in data collection is pivotal for organizations aiming to stay competitive in an ever-evolving landscape. By automating processes, providing real-time insights, and improving data quality, AI is not just a tool but a game-changer that can redefine the future of data management.
- What are the main benefits of using AI for data collection? AI improves efficiency, accuracy, and provides real-time insights, enabling organizations to make informed decisions quickly.
- Can AI tools be integrated with existing data systems? Yes, many AI tools are designed to integrate seamlessly with existing systems, enhancing their capabilities without requiring a complete overhaul.
- How does AI ensure data quality during collection? AI algorithms can filter out irrelevant data, identify anomalies, and categorize information, ensuring that only high-quality data is collected and analyzed.
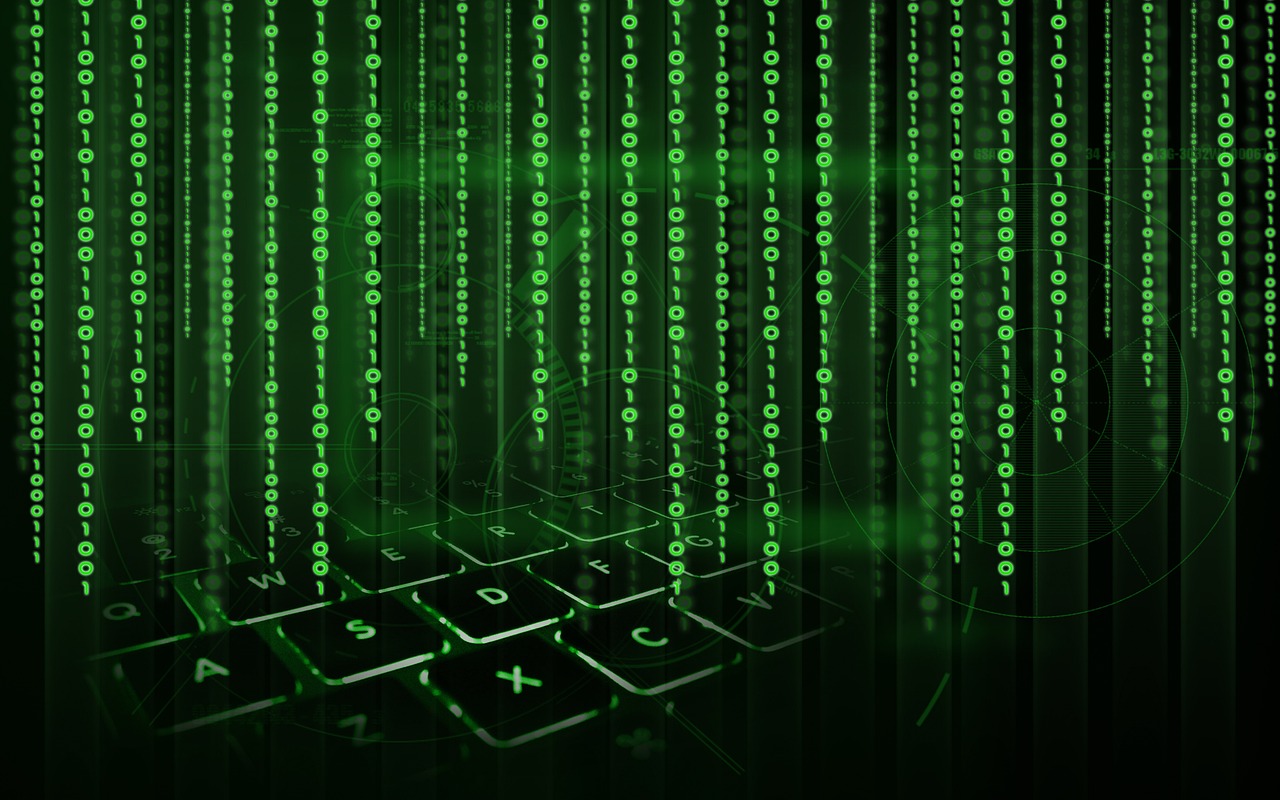
Data Cleaning and Preparation with AI
In the world of data management, the importance of cleaning and preparing data cannot be overstated. It’s like getting your house ready for guests; if it’s cluttered and messy, it’s hard to appreciate the beauty of the space. Similarly, raw data often comes with inconsistencies, errors, and noise that can obscure valuable insights. This is where artificial intelligence steps in, revolutionizing the way we approach data cleaning and preparation.
AI plays a pivotal role in transforming chaotic datasets into structured, high-quality information. By leveraging advanced algorithms and machine learning techniques, organizations can automate the arduous task of data cleaning. Imagine having a diligent assistant who tirelessly combs through your data, identifying and correcting errors while you focus on strategic decision-making. Sounds appealing, right?
One of the most significant advantages of using AI for data cleaning is its ability to identify patterns and anomalies that might go unnoticed by human analysts. For instance, machine learning algorithms can detect outliers in datasets that could skew results, ensuring that only reliable data informs your business strategies. This automated process not only saves time but also enhances the overall accuracy of the datasets.
When it comes to data preparation, automated data validation techniques powered by AI can significantly reduce errors. Traditional validation methods often involve manual checks, which can be time-consuming and prone to human error. In contrast, AI systems can quickly verify the accuracy and integrity of data before it’s used for analysis. For example, an AI model can be trained to recognize valid data formats and flag entries that deviate from these norms, ensuring that only the best quality data is utilized.
Machine learning models are increasingly employed for data validation tasks. These models can learn from historical data and improve over time, becoming more adept at identifying discrepancies. For instance, a supervised learning model can be trained on a labeled dataset, learning to distinguish between valid and invalid entries. As it processes new data, the model applies its learned knowledge to maintain high dataset quality. The effectiveness of these algorithms in maintaining dataset quality is remarkable, as they can adapt to changing data patterns and continuously improve.
Another game-changing aspect of AI in data preparation is real-time data monitoring. With the rise of big data, the ability to monitor data integrity continuously has become essential. AI technologies enable organizations to keep a vigilant eye on their datasets, detecting issues as they arise. For example, if a data stream suddenly experiences a spike in errors, AI can alert the team immediately, allowing for swift corrective action. This proactive approach ensures that potential problems are addressed before they escalate, safeguarding the integrity of business operations.
In summary, the integration of AI in data cleaning and preparation is transforming the landscape of data management. By automating validation processes, leveraging machine learning for accuracy, and enabling real-time monitoring, organizations can ensure that their datasets are not only clean but also reliable. This shift not only enhances decision-making but also empowers businesses to harness the full potential of their data.
- What is data cleaning? Data cleaning is the process of identifying and correcting errors or inconsistencies in a dataset to improve its quality.
- How does AI improve data cleaning? AI improves data cleaning by automating tasks, identifying anomalies, and ensuring data accuracy through machine learning algorithms.
- What are some challenges of using AI for data preparation? Challenges include potential biases in algorithms, the need for high-quality training data, and integration with existing systems.
- Can AI handle unstructured data? Yes, AI, particularly through natural language processing, can analyze and clean unstructured data, making it more usable for analysis.
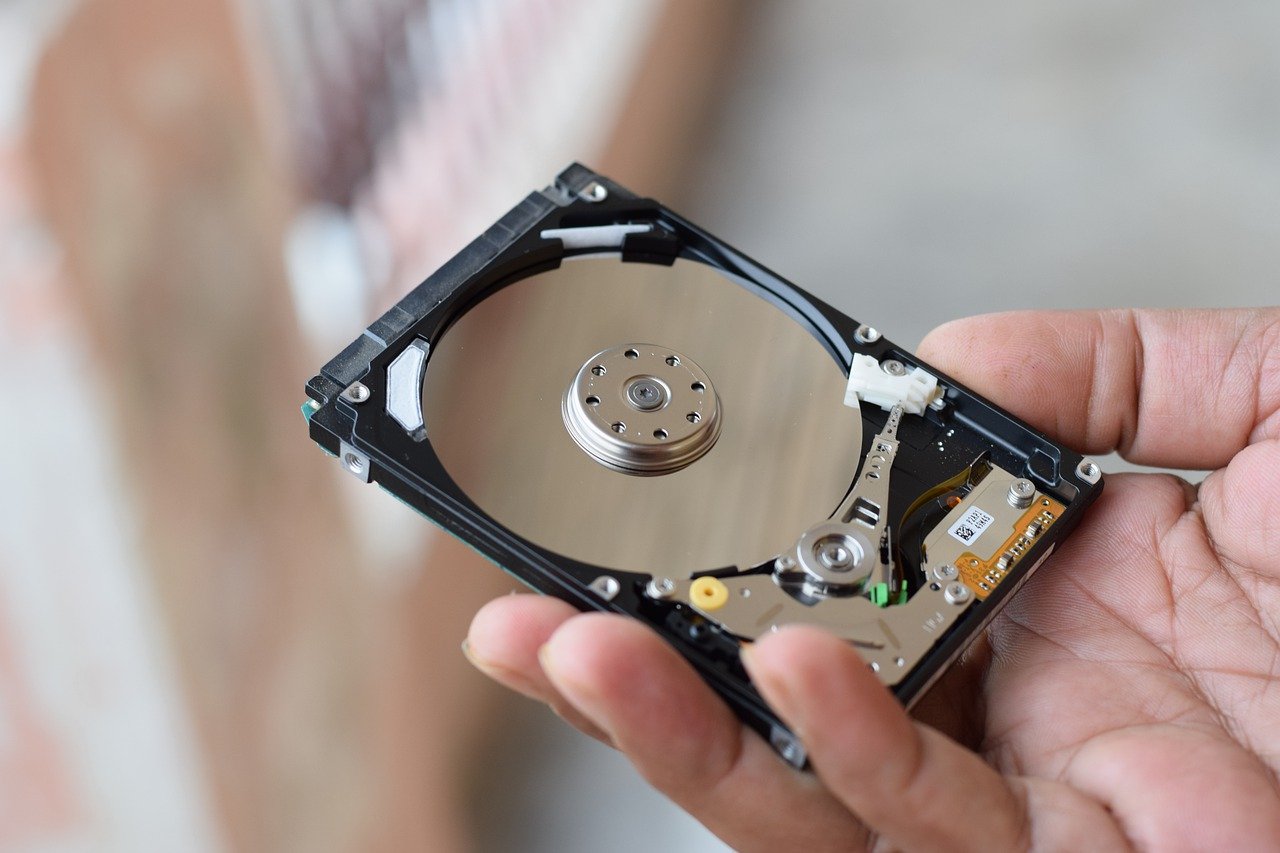
Automated Data Validation
Automated data validation is a game changer in the realm of data management, especially when it comes to ensuring the integrity and accuracy of datasets. Imagine trying to make decisions based on faulty data—it's like navigating a ship through fog without a compass. You might end up in the wrong place, or worse, capsizing your operations! With AI stepping into the spotlight, organizations can now rely on sophisticated algorithms that continuously check and verify data as it flows into their systems.
The beauty of automated data validation lies in its ability to operate at lightning speed, scanning vast amounts of data and identifying discrepancies that a human might overlook. This process not only saves time but also significantly reduces the risk of human error. For instance, consider a financial institution that processes thousands of transactions daily. By employing AI-driven validation techniques, they can quickly flag anomalies such as duplicate entries, incorrect formats, or missing values, ensuring that all data is consistent and reliable.
Moreover, the methods used in automated data validation can be categorized into several key techniques:
- Rule-based Validation: This approach involves setting predefined rules that the data must adhere to. For example, if a field is supposed to contain a date, the system will check that the input matches a valid date format.
- Statistical Validation: Here, statistical methods are used to identify outliers or anomalies within the dataset. If a transaction amount is significantly higher or lower than the average, it can be flagged for review.
- Cross-Validation: This technique involves comparing data across multiple sources to ensure consistency. For instance, customer information from different databases can be cross-checked to confirm accuracy.
As organizations continue to embrace automated validation, they are discovering that the benefits extend beyond mere accuracy. These systems can also provide valuable insights into data quality trends, helping businesses identify recurring issues and improve their data collection processes. By leveraging machine learning models, companies can refine their validation techniques over time, making them smarter and more efficient.
In conclusion, automated data validation is not just a luxury—it's a necessity in today’s data-driven world. By integrating AI-driven validation systems, organizations can enhance their data integrity, reduce operational risks, and ultimately make more informed decisions. The future of data management is here, and it's automated!
What is automated data validation?
Automated data validation refers to the use of AI and algorithms to automatically check and verify the accuracy and integrity of data as it is collected and processed. This helps in identifying errors and inconsistencies without manual intervention.
Why is automated data validation important?
It is crucial because it significantly reduces the risk of human error, enhances data integrity, and saves time. Accurate data is essential for making informed business decisions and maintaining operational efficiency.
What techniques are used in automated data validation?
Common techniques include rule-based validation, statistical validation, and cross-validation, each serving to ensure that the data adheres to specific quality standards.
Can automated data validation adapt over time?
Yes, many automated validation systems use machine learning, allowing them to learn from past data quality issues and improve their validation processes over time.
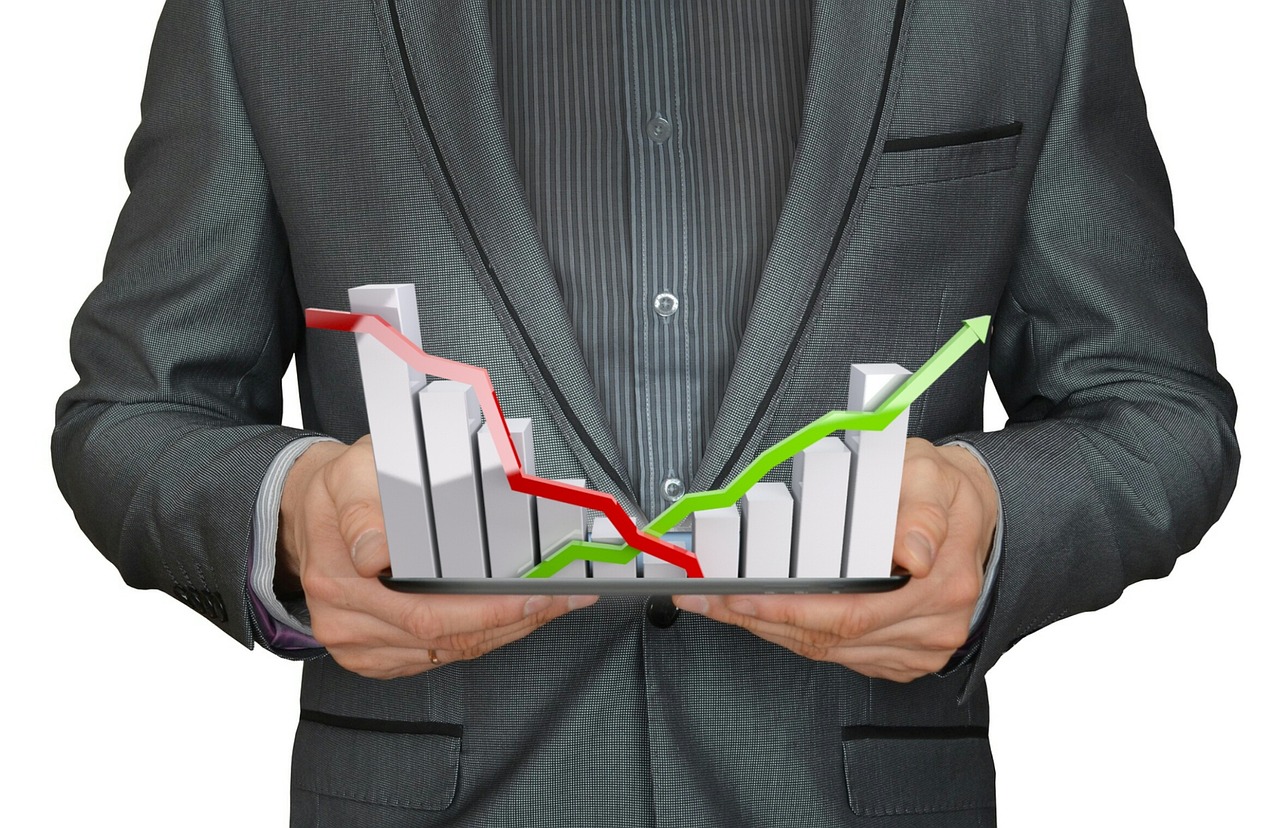
Machine Learning Models for Validation
When it comes to ensuring the integrity of data, machine learning models have emerged as powerful allies in the realm of validation. These models don't just streamline the process; they enhance the accuracy and reliability of data verification, making them indispensable in today's data-driven world. By employing sophisticated algorithms, organizations can automatically identify anomalies and inconsistencies that might otherwise go unnoticed. Imagine having a vigilant assistant that tirelessly scans through mountains of data, flagging potential errors before they wreak havoc on your analysis!
One of the most compelling aspects of machine learning models for validation is their ability to learn from historical data. As they process more information, these models become increasingly adept at recognizing patterns and trends. For instance, a model trained on past transaction data can learn to spot fraudulent activities by identifying deviations from established norms. This continuous learning process not only improves the model's performance but also builds a robust framework for data validation that evolves with the organization’s needs.
Several types of machine learning algorithms are commonly used for data validation, each with its own strengths. Here’s a brief overview of some popular models:
Algorithm | Description | Use Case |
---|---|---|
Decision Trees | Tree-like model used to make decisions based on data features. | Identifying data points that deviate from expected outcomes. |
Random Forest | An ensemble of decision trees that improves accuracy through averaging. | Robust validation in complex datasets with many variables. |
Support Vector Machines (SVM) | Classifies data by finding the best hyperplane that separates classes. | Effective in high-dimensional spaces, ideal for complex validation tasks. |
Neural Networks | Computational models inspired by the human brain, capable of learning from vast amounts of data. | Deep learning for intricate data patterns and anomalies. |
Choosing the right model depends on the specific requirements of the organization and the nature of the data being validated. For instance, if you're dealing with a vast dataset that includes numerous variables, a Random Forest might be your best bet due to its ability to handle complexity and reduce overfitting. On the other hand, if your data is more linear, a Support Vector Machine could provide the precision you need.
Furthermore, real-time data validation is becoming increasingly feasible thanks to advancements in machine learning. By integrating these models into data pipelines, organizations can monitor data continuously, ensuring that any inconsistencies are flagged immediately. This proactive approach not only saves time but also enhances the overall quality of the data being used for decision-making.
In summary, machine learning models for validation are revolutionizing how organizations handle data integrity. They not only automate the validation process but also provide a level of accuracy and adaptability that traditional methods simply cannot match. As businesses continue to rely on data-driven insights, leveraging these sophisticated models will be crucial in maintaining the quality and reliability of their datasets.
- What is the primary benefit of using machine learning for data validation? Machine learning models can automatically identify and rectify inconsistencies in data, ensuring higher accuracy and reliability.
- How do machine learning models learn over time? These models improve their performance by analyzing historical data and recognizing patterns, allowing them to adapt to new data inputs.
- Can machine learning models handle large datasets? Yes, many machine learning algorithms are designed to efficiently process and analyze large amounts of data, making them ideal for complex validation tasks.
- What types of algorithms are best for data validation? Algorithms like Decision Trees, Random Forest, Support Vector Machines, and Neural Networks are commonly used, each suited to different types of data and validation needs.
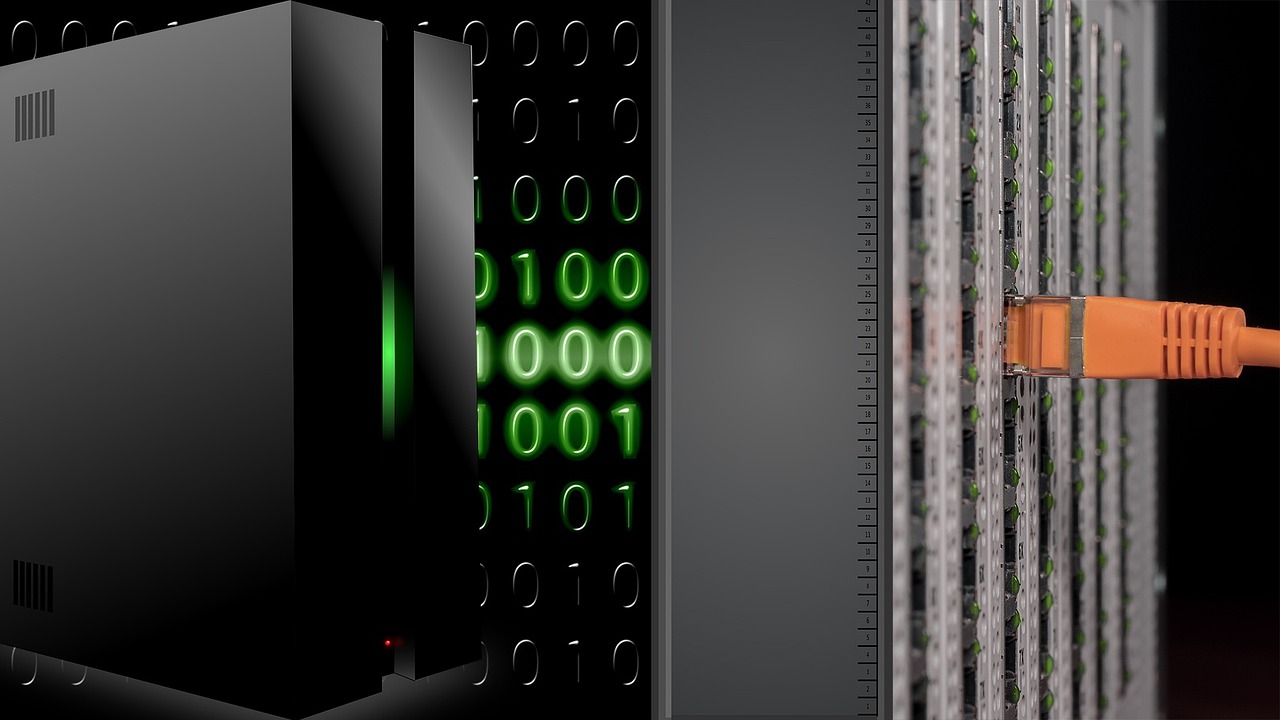
Real-time Data Monitoring
In today's fast-paced digital landscape, has become a game changer for businesses striving to maintain a competitive edge. Imagine having the ability to oversee your data as it flows in, like a vigilant lifeguard watching over a bustling beach. This proactive approach allows organizations to identify potential issues before they escalate into significant problems, ensuring smooth operations and informed decision-making.
AI technologies are at the forefront of this revolution, providing sophisticated tools that continuously analyze data streams. By employing algorithms capable of detecting anomalies, businesses can react swiftly to unexpected changes. For instance, if a sudden spike in website traffic occurs, AI systems can alert marketing teams to capitalize on the surge, potentially boosting sales and engagement. This level of responsiveness is crucial in an era where timeliness can mean the difference between seizing an opportunity and losing it to competitors.
Moreover, real-time monitoring enhances data integrity by implementing continuous checks on incoming data. This process not only identifies errors but also ensures that the data being used for analysis is accurate and reliable. Think of it as having a quality control inspector on the production line, ensuring that every product meets the required standards before reaching the consumer. In this scenario, organizations can trust that their data-driven decisions are based on solid foundations.
To illustrate the impact of real-time data monitoring, consider the following table that highlights key benefits:
Benefit | Description |
---|---|
Immediate Alerts | Receive notifications for anomalies or potential issues as they occur, allowing for quick intervention. |
Enhanced Decision-Making | Make informed decisions based on the most current data available, improving overall strategy. |
Operational Efficiency | Streamline processes by identifying bottlenecks and inefficiencies in real-time. |
However, implementing real-time data monitoring is not without its challenges. Organizations must ensure that they have the appropriate infrastructure in place to handle the vast amounts of data generated continuously. This includes investing in robust data storage solutions and choosing the right AI tools that can integrate seamlessly with existing systems. Furthermore, data privacy concerns must be addressed, as constant monitoring can lead to potential breaches if not managed correctly.
In conclusion, real-time data monitoring powered by AI is transforming how businesses operate. By enabling organizations to stay ahead of the curve, this innovation not only enhances data integrity but also fosters a culture of proactive decision-making. As companies continue to adapt to the demands of the digital age, those that embrace these advanced monitoring techniques will likely lead the charge in their respective industries.
- What is real-time data monitoring? Real-time data monitoring involves continuously overseeing data as it is collected, allowing organizations to identify issues and respond quickly.
- How does AI enhance real-time monitoring? AI enhances real-time monitoring by using algorithms to detect anomalies and ensure data integrity, enabling faster decision-making.
- What are the challenges of implementing real-time monitoring? Challenges include ensuring appropriate infrastructure, addressing data privacy concerns, and integrating with existing systems.
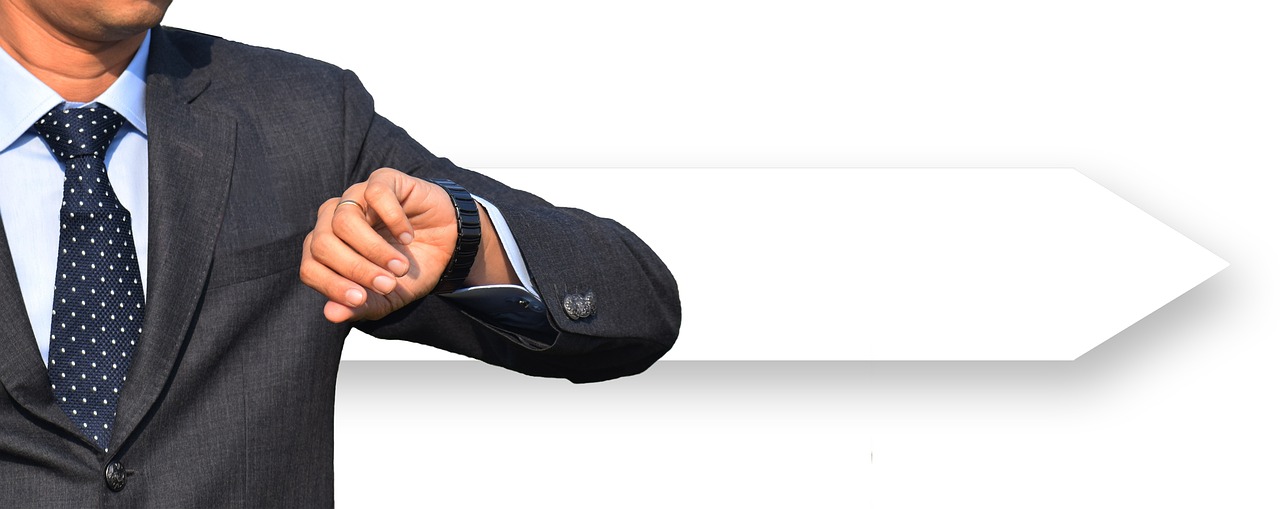
Enhancing Data Storage Solutions
In the rapidly evolving landscape of data management, artificial intelligence (AI) is playing a transformative role in enhancing data storage solutions. Traditional storage methods often struggle to keep pace with the exponential growth of data, leading to inefficiencies and increased costs. However, with AI-driven technologies, organizations can optimize their data storage strategies, ensuring that they not only store vast amounts of information but also retrieve it swiftly and efficiently.
One of the key innovations introduced by AI in data storage is the ability to predict storage needs based on usage patterns. By analyzing historical data, AI algorithms can forecast future storage requirements, allowing businesses to scale their resources proactively rather than reactively. This predictive capability not only saves costs but also minimizes the risk of running out of storage space during critical operations.
Furthermore, AI enhances data retrieval processes through intelligent indexing and search capabilities. Traditional search methods can be cumbersome and time-consuming, often requiring users to sift through countless files and folders. AI-powered solutions, on the other hand, utilize machine learning to improve search accuracy and speed. These systems understand user behavior and preferences, making it easier for individuals to find the information they need without unnecessary delays.
Another significant benefit of AI in data storage is its ability to automate data organization. With the sheer volume of data generated daily, manually categorizing and tagging information can be a daunting task. AI algorithms can automatically label and organize data based on predefined criteria, ensuring that everything is in its right place. This not only saves time but also enhances data accessibility, allowing teams to collaborate more effectively.
Moreover, AI-driven storage solutions can optimize data redundancy and backup processes. By analyzing data usage patterns, these systems can determine which data is accessed frequently and which is not. This insight enables organizations to prioritize which data to back up and replicate, reducing storage costs while ensuring that critical information is always protected. In essence, AI helps businesses implement a more efficient data lifecycle management strategy.
As we look to the future, the integration of AI in data storage solutions is likely to lead to even more groundbreaking advancements. From cloud storage optimization to enhanced data security measures, the possibilities are endless. Organizations that embrace these technologies will not only improve their operational efficiency but also gain a competitive edge in the data-driven marketplace.
In summary, AI is revolutionizing the way we approach data storage. By leveraging predictive analytics, intelligent search capabilities, automated organization, and optimized backup processes, businesses can enhance their data management practices significantly. As we continue to generate and rely on vast amounts of data, the role of AI in storage solutions will only become more critical.
- How does AI improve data retrieval? AI enhances data retrieval by utilizing intelligent indexing and machine learning algorithms to understand user preferences, making searches faster and more accurate.
- What are the benefits of automated data organization? Automated data organization saves time, reduces human error, and improves data accessibility, allowing teams to collaborate more effectively.
- Can AI help in predicting future storage needs? Yes, AI can analyze historical usage patterns to forecast future storage requirements, enabling proactive scaling of resources.
- How does AI optimize backup processes? By analyzing data usage, AI can prioritize which data to back up, reducing costs while ensuring critical information is protected.
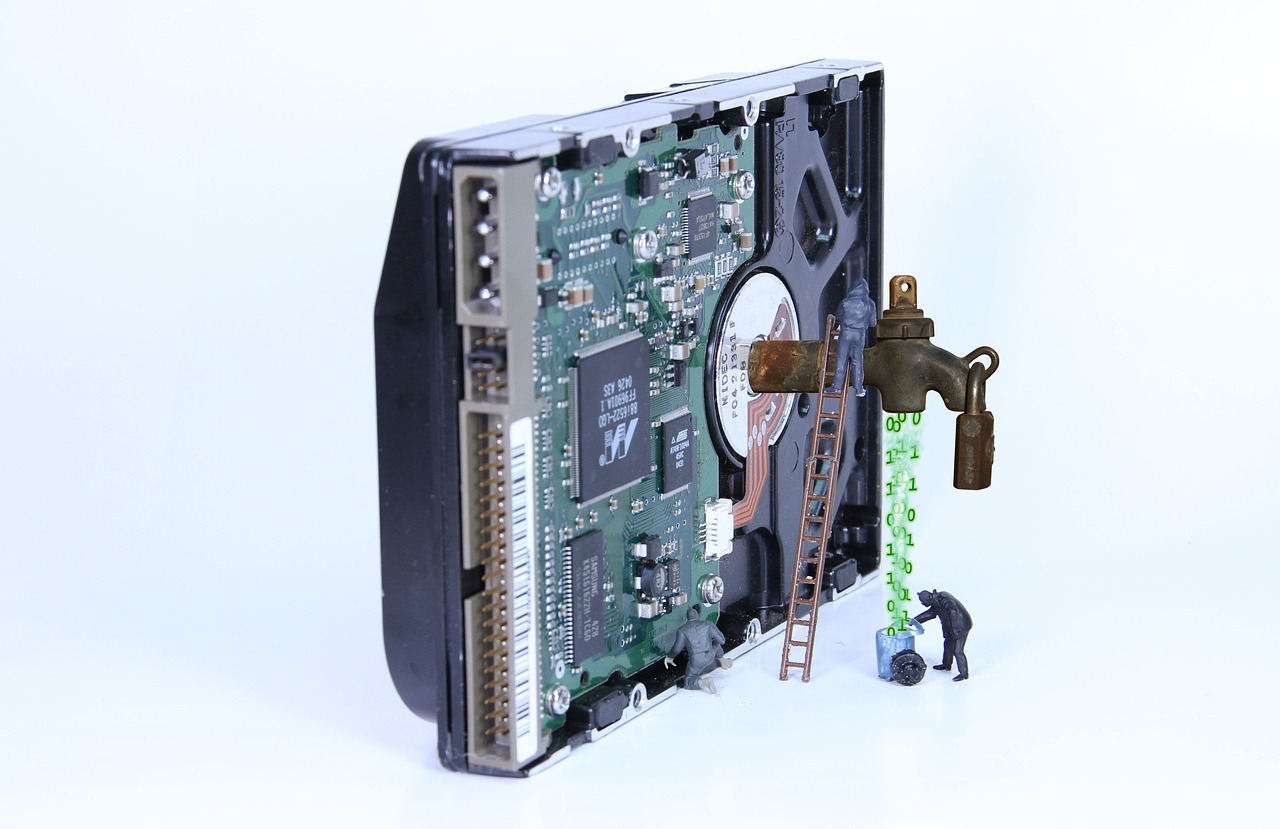
AI-driven Data Analysis Techniques
In today's fast-paced digital world, the ability to extract meaningful insights from vast amounts of data has become a game changer for businesses. AI-powered analytics tools are at the forefront of this transformation, enabling organizations to dive deeper into complex datasets like never before. Imagine trying to find a needle in a haystack; now, picture having a magnet that not only finds that needle but also tells you how it got there and what it means for your future operations. That's the power of AI in data analysis!
One of the standout features of AI-driven data analysis is its capability to perform predictive analytics. This technique allows businesses to forecast trends and behaviors by analyzing historical data patterns. For instance, retail companies can predict customer purchasing behavior based on past sales data, seasonal trends, and even social media interactions. This predictive power helps organizations make informed decisions, optimize inventory, and tailor marketing strategies to meet customer demands. In fact, a study by McKinsey found that companies leveraging predictive analytics can achieve up to a 20% increase in revenue compared to those that do not.
Another exciting advancement in AI-driven data analysis is the use of Natural Language Processing (NLP). NLP enables machines to understand and interpret human language, making data analysis not just a task for data scientists but an accessible tool for everyone in the organization. Imagine being able to ask your analytics platform a question in plain English, like, "What were our top-selling products last quarter?" and receiving an accurate, insightful response in seconds. This capability opens up a world of possibilities for extracting insights from unstructured data sources, such as emails, social media posts, and customer reviews.
To illustrate the impact of these AI techniques, consider the following table that outlines some key AI-driven analysis methods and their applications:
Technique | Description | Applications |
---|---|---|
Predictive Analytics | Analyzes historical data to forecast future trends. | Retail sales forecasting, financial risk assessment. |
Natural Language Processing (NLP) | Enables machines to understand and interpret human language. | Sentiment analysis, chatbots, automated reporting. |
Machine Learning | Uses algorithms to identify patterns and make predictions. | Fraud detection, customer segmentation. |
As organizations continue to embrace these AI-driven techniques, they are not just enhancing their data analysis capabilities but also fostering a culture of data-driven decision-making. The ability to quickly analyze data and gain actionable insights is akin to having a crystal ball that reveals hidden opportunities and potential pitfalls. Companies that harness these technologies will not only stay ahead of the competition but also create more personalized experiences for their customers.
However, while the benefits of AI in data analysis are significant, it's crucial to recognize that the technology is not infallible. Organizations must remain vigilant about the quality of their data, as the old adage goes, "Garbage in, garbage out." Ensuring that data is accurate, comprehensive, and relevant is essential for the success of any AI-driven analysis initiative.
- What is AI-driven data analysis? AI-driven data analysis refers to the use of artificial intelligence technologies to analyze and interpret data, providing insights and predictions that aid decision-making.
- How does predictive analytics work? Predictive analytics uses statistical algorithms and machine learning techniques to identify patterns in historical data, which can then be used to forecast future outcomes.
- What role does NLP play in data analysis? Natural Language Processing allows computers to understand and process human language, enabling more intuitive and accessible data analysis through conversational interfaces.
- What are the challenges of implementing AI in data analysis? Challenges include ensuring data quality, addressing biases in algorithms, and integrating AI tools with existing data systems.
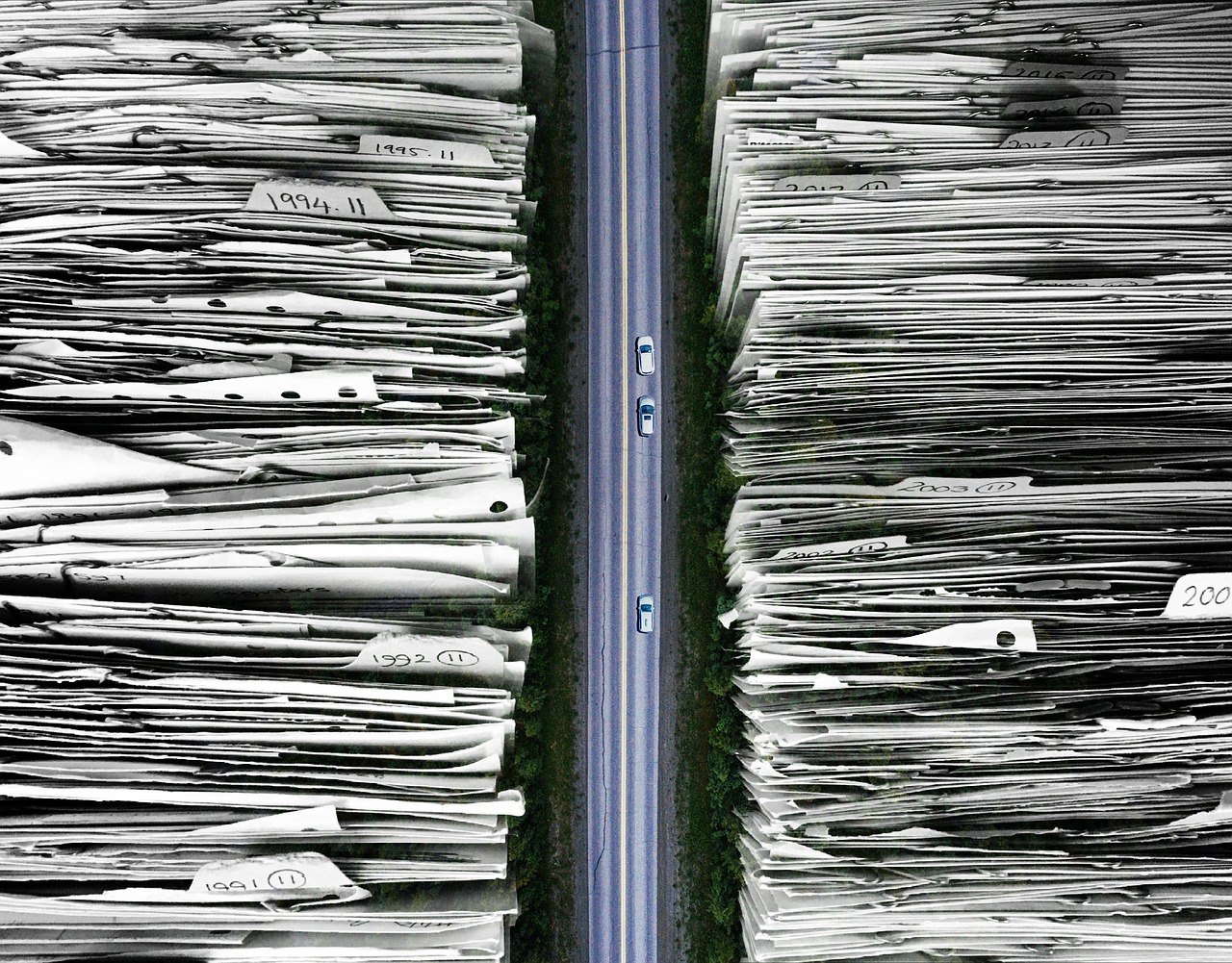
Predictive Analytics in Business
Predictive analytics is the crystal ball of the business world, allowing organizations to look into the future and make informed decisions based on data-driven insights. By harnessing the power of artificial intelligence, businesses can analyze historical data, identify patterns, and predict future outcomes with remarkable accuracy. This not only helps in anticipating customer behavior but also enables companies to optimize their operations and resources effectively.
Imagine a retail store that uses predictive analytics to forecast customer purchasing trends. By analyzing past sales data, customer demographics, and seasonal trends, the store can predict which products are likely to be in high demand during the upcoming holiday season. This foresight allows them to stock up on popular items, ensuring they meet customer demand without overstocking, which can lead to wasted resources. The result? Increased sales and a happier customer base.
Moreover, predictive analytics isn't just limited to retail; it's making waves across various sectors. For instance, in healthcare, predictive models can identify patients at risk of developing chronic conditions, enabling proactive interventions. In finance, institutions can assess the likelihood of loan defaults, allowing them to make better lending decisions. The versatility of predictive analytics is truly astounding, impacting industries from marketing to manufacturing.
To give you a clearer picture, let's look at some key applications of predictive analytics across different business functions:
Business Function | Application | Benefits |
---|---|---|
Marketing | Customer segmentation and targeting | Increased ROI on marketing campaigns |
Sales | Sales forecasting | Improved inventory management |
Finance | Risk assessment | Reduced financial losses |
Operations | Supply chain optimization | Enhanced efficiency and reduced costs |
The benefits of incorporating predictive analytics into business strategies are profound, but they come with their own set of challenges. Organizations must ensure they have high-quality data to fuel their predictive models. Poor data quality can lead to inaccurate predictions, which can be detrimental to decision-making processes. Additionally, as businesses increasingly rely on these insights, they must also be cautious about data privacy and ethical considerations surrounding the use of personal information.
In conclusion, predictive analytics is transforming the way businesses operate, enabling them to stay ahead of the curve and make proactive decisions. As technology continues to evolve, those who embrace predictive analytics will likely find themselves at a significant competitive advantage, navigating the complexities of the market with confidence and precision.
- What is predictive analytics?
Predictive analytics involves using statistical algorithms and machine learning techniques to identify the likelihood of future outcomes based on historical data.
- How can businesses benefit from predictive analytics?
Businesses can enhance decision-making, improve customer satisfaction, optimize operations, and increase profitability through predictive analytics.
- What industries can utilize predictive analytics?
Predictive analytics can be applied in various industries, including retail, healthcare, finance, manufacturing, and marketing.
- What are some challenges associated with predictive analytics?
Challenges include the need for high-quality data, potential bias in algorithms, and ensuring data privacy and security.
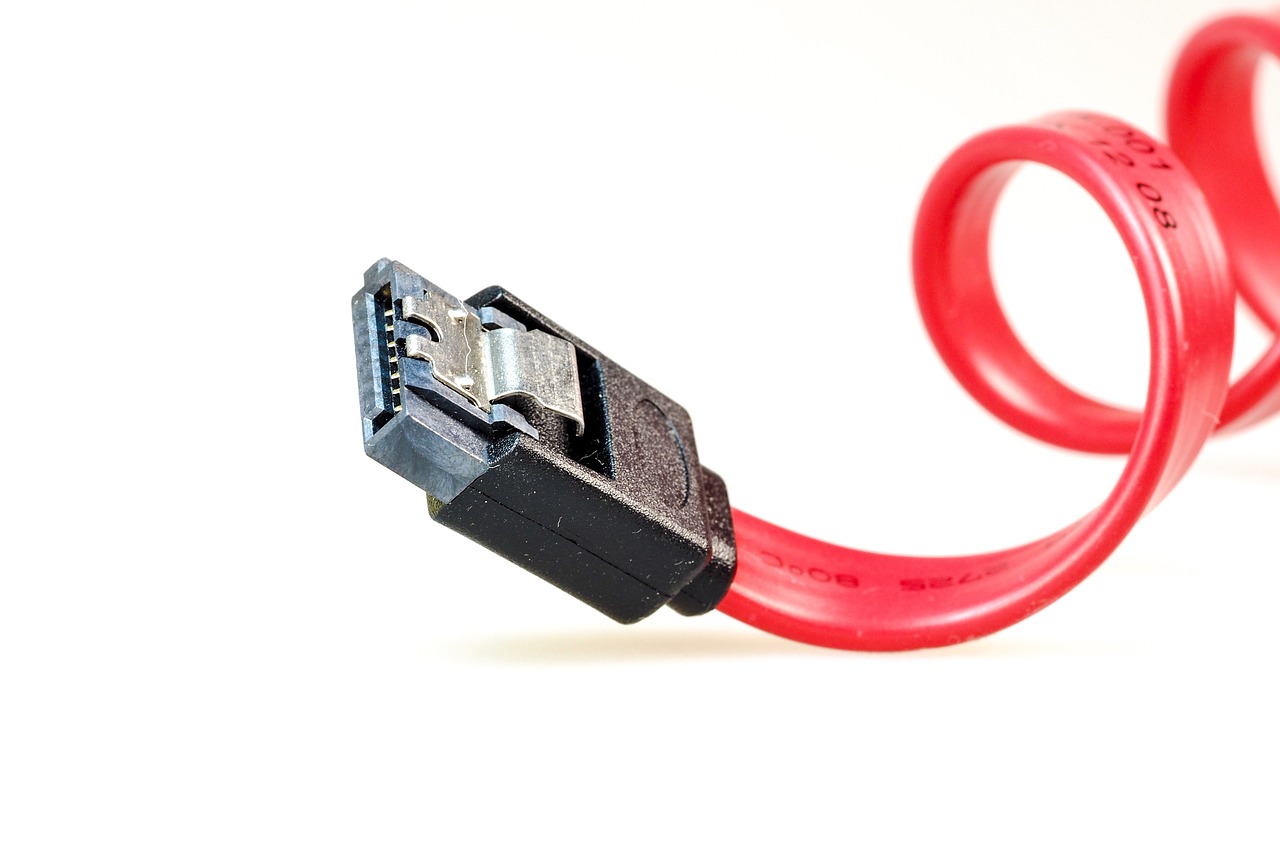
NLP for Data Interpretation
Natural Language Processing, or NLP, is like having a super-smart translator in the world of data analysis. Imagine trying to make sense of a mountain of text data—emails, social media posts, customer reviews, and more. It's overwhelming, right? That's where NLP comes in, transforming this chaotic jumble into structured, actionable insights. By enabling machines to understand, interpret, and respond to human language, NLP bridges the gap between human communication and data analysis.
One of the most exciting aspects of NLP is its ability to extract valuable information from unstructured data. Think about it: a company might receive thousands of customer feedback forms, each filled with unique phrases and sentiments. Manually sifting through this feedback to gauge customer satisfaction would take ages. However, with NLP, algorithms can analyze this data quickly, identifying trends, sentiments, and key themes. It’s like having a data analyst who never sleeps and works at lightning speed!
NLP also enhances the way we interpret data by employing techniques such as sentiment analysis. This process allows organizations to determine whether the public's opinion is positive, negative, or neutral regarding their products or services. For instance, if a restaurant launches a new dish, NLP can analyze social media mentions and customer reviews to give immediate feedback on how well the dish is being received. This real-time insight can be invaluable for making quick adjustments to marketing strategies or menu offerings.
Moreover, NLP can categorize and summarize large volumes of text, making it easier for businesses to digest critical information. By using algorithms that can identify keywords and themes, companies can create concise reports that highlight essential findings without getting bogged down in endless details. For example, a financial services firm might use NLP to analyze thousands of news articles and reports, summarizing the most relevant information to inform investment decisions.
To illustrate the power of NLP in data interpretation, consider the following table that summarizes some common applications of NLP in various industries:
Industry | Application | Benefit |
---|---|---|
Retail | Customer Feedback Analysis | Improved product offerings based on customer sentiment |
Healthcare | Patient Report Analysis | Enhanced patient care through understanding patient experiences |
Finance | Market Sentiment Analysis | Informed investment decisions based on public sentiment |
Education | Student Feedback Analysis | Improved curriculum based on student sentiments |
In conclusion, NLP is revolutionizing how we interpret data by providing tools that can analyze human language at scale. As organizations continue to harness the power of NLP, they will unlock deeper insights from their data, enabling smarter decision-making and ultimately driving better business outcomes. So, the next time you think about data analysis, remember the silent hero working behind the scenes: Natural Language Processing.
- What is NLP? NLP stands for Natural Language Processing, a branch of artificial intelligence that helps machines understand and interpret human language.
- How does NLP benefit data analysis? NLP enables the extraction of insights from unstructured data, allowing for faster and more accurate analysis of text data.
- Can NLP be used in real-time? Yes, NLP can analyze data in real-time, providing immediate insights that can inform business decisions.
- What industries can benefit from NLP? NLP can be applied across various industries, including retail, healthcare, finance, and education, to enhance data interpretation and decision-making.
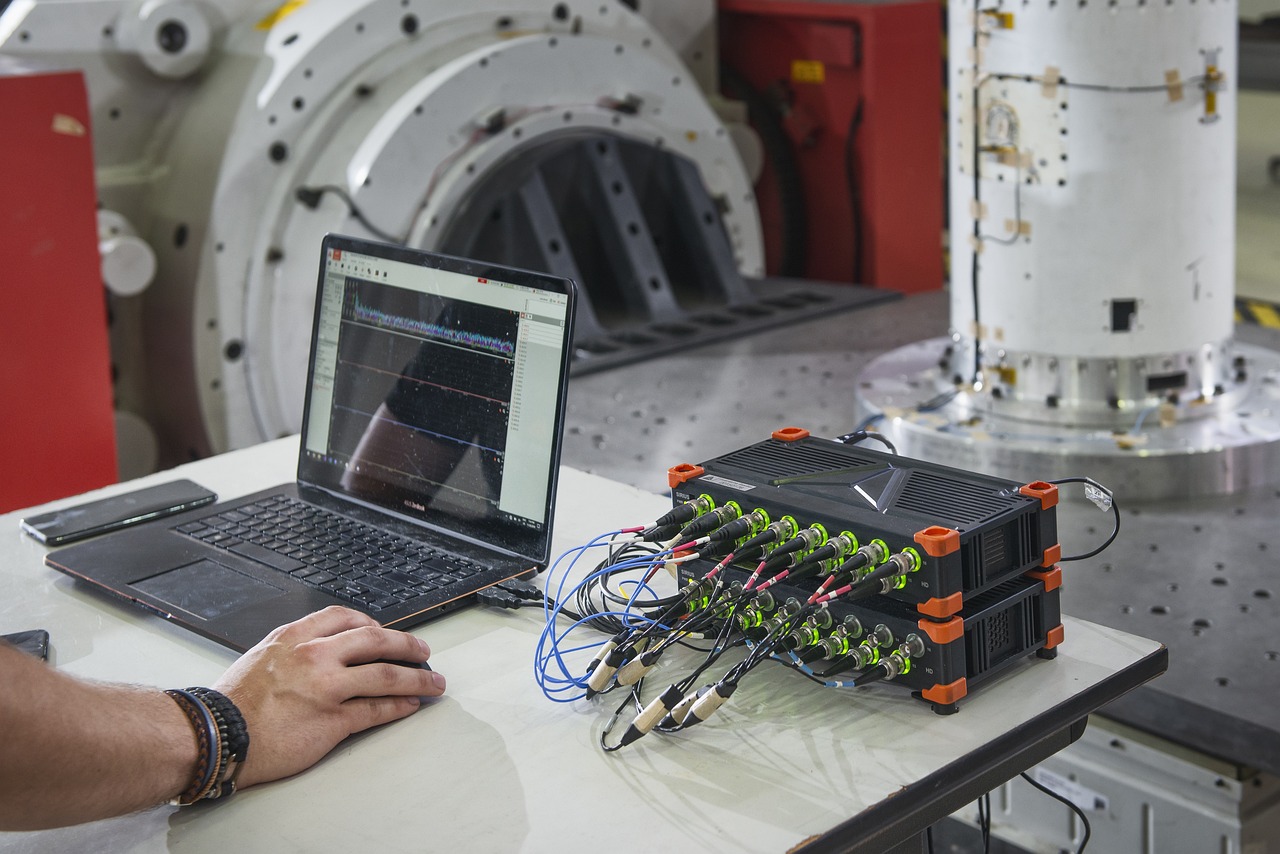
Challenges in AI Data Management
As we dive deeper into the realm of artificial intelligence (AI), it's essential to acknowledge the challenges that accompany its integration into data management practices. While AI offers remarkable benefits, the journey isn't without its hurdles. One of the most pressing issues is data privacy. With the rise of data breaches and stringent regulations like GDPR, organizations must tread carefully to ensure that sensitive information remains secure. The challenge lies in balancing the need for data access and analysis with the imperative to protect individual privacy.
Another significant concern is bias in AI algorithms. If the datasets used to train AI models are biased, the outcomes will also reflect those biases. This can lead to skewed insights and decision-making processes that perpetuate inequality. It's crucial for organizations to actively work on identifying and mitigating biases in their data to foster fairness and inclusivity in their AI applications.
Moreover, integration issues pose a considerable challenge. Many businesses operate with legacy systems that may not easily accommodate new AI technologies. This can result in a fragmented data landscape where information is siloed, making it difficult to achieve a comprehensive view of the data. Organizations must invest in modernizing their infrastructure and ensuring seamless integration of AI tools to harness the full potential of their data.
In addition to these challenges, the complexity of AI technologies can be daunting for many organizations. Understanding the intricacies of machine learning models and their applications requires specialized knowledge. Companies often find themselves in a race to upskill their workforce or hire new talent, which can be both time-consuming and costly. The lack of skilled professionals in the field further exacerbates this issue, leading to a skills gap that can hinder progress.
Lastly, the cost of implementation cannot be overlooked. Integrating AI into data management processes often requires substantial investment in technology, training, and ongoing maintenance. For small to medium-sized enterprises, these costs can be prohibitive, leading to a reluctance to adopt AI solutions. Organizations must carefully evaluate their budgets and consider the long-term benefits of AI against the initial outlay.
In summary, while AI holds the promise of transforming data management, organizations must navigate a landscape filled with challenges. From ensuring data privacy and combating bias to overcoming integration hurdles and managing costs, the road to successful AI adoption is paved with obstacles. However, with strategic planning and a commitment to addressing these issues, businesses can unlock the full potential of AI in their data management practices.
- What are the main challenges of AI in data management? The primary challenges include data privacy, bias in algorithms, integration issues with legacy systems, complexity of AI technologies, and the cost of implementation.
- How can organizations address data privacy concerns? Organizations can implement strict access controls, anonymize data, and comply with regulations like GDPR to enhance data privacy.
- What steps can be taken to mitigate bias in AI? It's vital to use diverse datasets for training, regularly audit algorithms for bias, and involve diverse teams in the AI development process.
- Why is integration a challenge for AI? Many businesses still rely on outdated systems that may not support modern AI technologies, leading to fragmented data and inefficiencies.
- How can companies manage the costs of AI implementation? Companies should consider phased implementations, seek partnerships, and explore cloud-based AI solutions to spread out costs.
Frequently Asked Questions
- How does AI improve data collection?
AI enhances data collection by automating repetitive tasks, which not only speeds up the process but also improves accuracy. Imagine having a smart assistant that can gather, sort, and analyze data in real-time—this is what AI does for businesses, allowing them to gain insights faster than ever before.
- What role does AI play in data cleaning?
AI is crucial for cleaning data by identifying inconsistencies and errors that could skew analysis. Think of it as a meticulous editor who combs through a manuscript to catch every typo and grammatical mistake, ensuring the final product is polished and ready for publication.
- Can AI automate data validation?
Absolutely! AI can automate data validation processes, significantly reducing human error. It uses sophisticated algorithms to verify data accuracy and integrity, much like a security system that constantly monitors for breaches, ensuring everything is in check before any analysis takes place.
- How does real-time monitoring of data work?
Real-time monitoring powered by AI keeps an eye on data integrity continuously. This proactive approach allows businesses to catch and address potential issues before they escalate, similar to having a smoke detector that alerts you before a fire gets out of control.
- What advancements are being made in AI-driven data storage?
AI is revolutionizing data storage by optimizing how data is stored and retrieved. With AI, storage systems can learn usage patterns and adjust accordingly, making data access faster and more efficient, just like a well-organized library where you can find any book in seconds.
- How does predictive analytics benefit businesses?
Predictive analytics enables businesses to forecast trends and consumer behaviors. By leveraging AI, companies can make informed decisions and strategize effectively, much like a seasoned sailor who reads the wind patterns to navigate the waters smoothly.
- What is the significance of NLP in data interpretation?
Natural Language Processing (NLP) allows machines to understand and interpret human language, making data analysis more intuitive. It’s like having a translator who can convert complex data into simple insights, making it easier for everyone to grasp and utilize.
- What challenges do organizations face with AI in data management?
While AI offers many benefits, organizations face challenges such as data privacy concerns, potential biases in algorithms, and integration issues with existing systems. It’s akin to navigating a maze where one wrong turn can lead to significant setbacks, requiring careful planning and execution.