How AI is Enhancing Decision-Making Processes
The landscape of decision-making is undergoing a seismic shift, courtesy of artificial intelligence (AI). In a world overflowing with data, the ability to make informed decisions quickly is more crucial than ever. Imagine navigating a vast ocean of information without a compass; that’s what traditional decision-making feels like. However, with AI as our guide, we can chart a course through the chaos, ensuring that decisions are not just made but are made with precision and insight. This article delves into how AI is transforming decision-making across various sectors, highlighting its benefits, challenges, and future implications.
At its core, AI enhances decision-making by processing and analyzing data at speeds and volumes that humans simply cannot match. It can sift through terabytes of information, identify patterns, and deliver actionable insights in seconds. This capability is particularly invaluable in fields like business, healthcare, and finance, where timely decisions can lead to significant advantages. For instance, businesses leveraging AI can optimize their operations, improve customer engagement, and make strategic choices based on data-driven insights rather than gut feelings.
Moreover, the integration of AI into decision-making processes is not just about speed; it’s also about enhancing the quality of decisions. By eliminating human biases and providing objective data analysis, AI helps organizations make choices that are not only informed but also fair and equitable. However, while the advantages are compelling, the journey towards AI-enhanced decision-making is not without its challenges. Issues such as data privacy, algorithmic bias, and the need for human oversight remain critical considerations that organizations must address to harness AI's full potential responsibly.
AI technologies are revolutionizing how businesses make decisions, enabling data-driven strategies that enhance efficiency and accuracy in operations and customer engagement. In today’s fast-paced market, companies that can quickly adapt to changing circumstances have a significant edge. By utilizing AI, businesses can analyze consumer behavior, forecast trends, and optimize inventory management, thus ensuring they meet customer demands effectively.
For example, consider a retail company that employs AI algorithms to analyze purchasing patterns. By examining historical data and current trends, the AI can predict which products will be in demand during specific seasons. This predictive capability allows the company to stock up on popular items, reducing waste and maximizing sales. In essence, AI acts as a crystal ball, enabling businesses to foresee market shifts and respond proactively.
In the realm of healthcare, AI is a game changer. It assists professionals in diagnosing diseases, personalizing treatment plans, and predicting patient outcomes, ultimately improving the quality of care and operational efficiency. Imagine a doctor having access to a digital assistant that can analyze a patient’s symptoms and medical history in seconds, providing a list of potential diagnoses and treatment options. This is not a distant dream; it’s happening now.
Predictive analytics powered by AI allows healthcare providers to anticipate patient needs, manage resources effectively, and reduce costs by identifying trends and patterns in patient data. For instance, hospitals can predict patient admissions during flu season and allocate resources accordingly, ensuring they are prepared for the influx of patients. This proactive approach can save lives and reduce strain on healthcare systems.
Machine learning algorithms analyze vast datasets to uncover insights that help healthcare professionals make informed decisions, leading to better patient outcomes and streamlined processes. These algorithms can learn from new data, continuously improving their accuracy over time. For example, an algorithm trained on thousands of medical images can detect anomalies that even seasoned radiologists might miss, leading to earlier interventions and better patient prognoses.
AI-assisted diagnostics utilize advanced imaging and data analysis to support clinicians in identifying conditions more accurately and swiftly, enhancing the overall diagnostic process. By integrating AI into diagnostic tools, healthcare providers can ensure that they are making the best possible decisions for their patients, ultimately leading to improved health outcomes.
In finance, AI algorithms analyze market trends and consumer behavior, providing insights that help investors and financial institutions make informed decisions and manage risks effectively. The stock market, for example, is influenced by countless variables, and AI can process this information to identify profitable investment opportunities. Think of AI as a sophisticated financial advisor, capable of crunching numbers and analyzing trends at lightning speed.
Despite its advantages, integrating AI into decision-making processes poses challenges such as data privacy concerns, algorithm bias, and the need for human oversight to ensure ethical practices. Organizations must tread carefully, balancing the benefits of AI with the potential pitfalls.
Data privacy and security are paramount when implementing AI systems, as organizations must safeguard sensitive information while complying with regulations to maintain trust and integrity. The misuse of data can lead to severe consequences, both legally and ethically.
Addressing algorithmic bias is crucial for ensuring fairness in AI-driven decisions, necessitating ongoing evaluations and adjustments to algorithms to mitigate potential discrimination and inaccuracies. Organizations must be vigilant in monitoring AI outputs to ensure that they reflect equitable practices.
- What is AI? AI, or artificial intelligence, refers to the simulation of human intelligence in machines that are programmed to think and learn.
- How does AI help in decision-making? AI enhances decision-making by analyzing large datasets quickly, identifying patterns, and providing actionable insights.
- What are the challenges of using AI in decision-making? Challenges include data privacy concerns, algorithmic bias, and the necessity for human oversight.
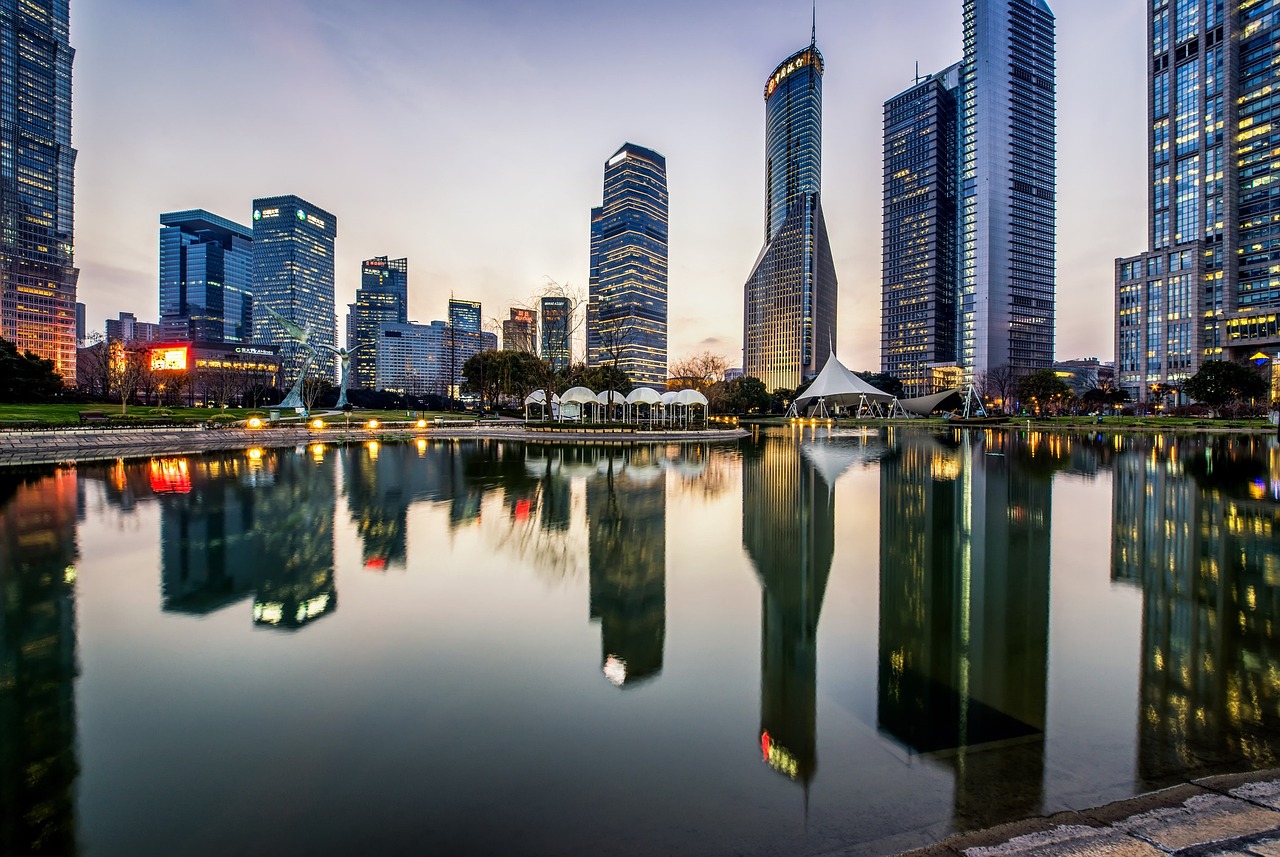
The Role of AI in Business Decisions
Artificial Intelligence (AI) is not just a buzzword; it’s a game-changer in the business landscape today. Imagine a world where businesses can make decisions faster, more accurately, and with less human error. Sounds like a dream, right? Well, with AI technologies, this dream is becoming a reality. AI is revolutionizing how organizations formulate strategies, optimize operations, and engage with customers. By leveraging vast amounts of data, AI enables businesses to adopt data-driven strategies that enhance efficiency and accuracy across all levels of operation.
One of the key advantages of integrating AI into business decision-making is the ability to analyze data at lightning speed. Traditional methods of data analysis can be time-consuming and often lead to missed opportunities. With AI, businesses can process and analyze data in real-time, allowing them to respond swiftly to market changes and consumer demands. This capability not only improves operational efficiency but also enhances customer engagement by providing personalized experiences. For instance, AI algorithms can analyze customer behavior and preferences to tailor marketing strategies that resonate with individual users, ultimately driving sales and customer loyalty.
Moreover, AI can help in predicting future trends, enabling businesses to stay ahead of the curve. By using advanced analytics, companies can identify patterns and make forecasts that inform their strategic decisions. This predictive capability is particularly useful in industries like retail, where understanding consumer behavior can lead to more effective inventory management and sales strategies. For example, a retail company might use AI to analyze purchasing trends, allowing them to stock up on popular items before peak shopping seasons, thus maximizing profits.
Let’s not forget about the impact of AI on risk management. In an ever-changing business environment, the ability to assess risks and make informed decisions is crucial. AI systems can analyze historical data and current market conditions to identify potential risks, allowing businesses to mitigate them proactively. This not only protects the company’s bottom line but also builds trust with stakeholders who value transparency and accountability.
However, while the benefits of AI in business decision-making are substantial, it’s essential to approach its implementation thoughtfully. Companies must ensure that they have the right infrastructure in place to support AI technologies. This includes investing in quality data management systems and training staff to work alongside AI tools effectively. Additionally, organizations should remain vigilant about the ethical implications of AI, ensuring that their systems are designed to promote fairness and transparency.
In conclusion, the role of AI in business decisions is transformative. By enabling data-driven strategies, improving operational efficiency, enhancing customer engagement, and aiding in risk management, AI is paving the way for a more intelligent and responsive business environment. As companies continue to embrace AI, those that prioritize ethical practices and invest in the right resources will undoubtedly lead the charge into the future.
- What is the main benefit of using AI in business decisions? The main benefit is the ability to analyze vast amounts of data quickly and accurately, leading to better strategic decisions.
- How does AI improve customer engagement? AI analyzes customer behavior and preferences, allowing businesses to create personalized experiences that resonate with individual users.
- Are there risks associated with AI in decision-making? Yes, there are risks such as data privacy concerns and the potential for algorithmic bias, which must be managed carefully.
- What industries can benefit from AI in decision-making? Almost any industry can benefit, but particularly retail, finance, and healthcare have seen significant advancements.
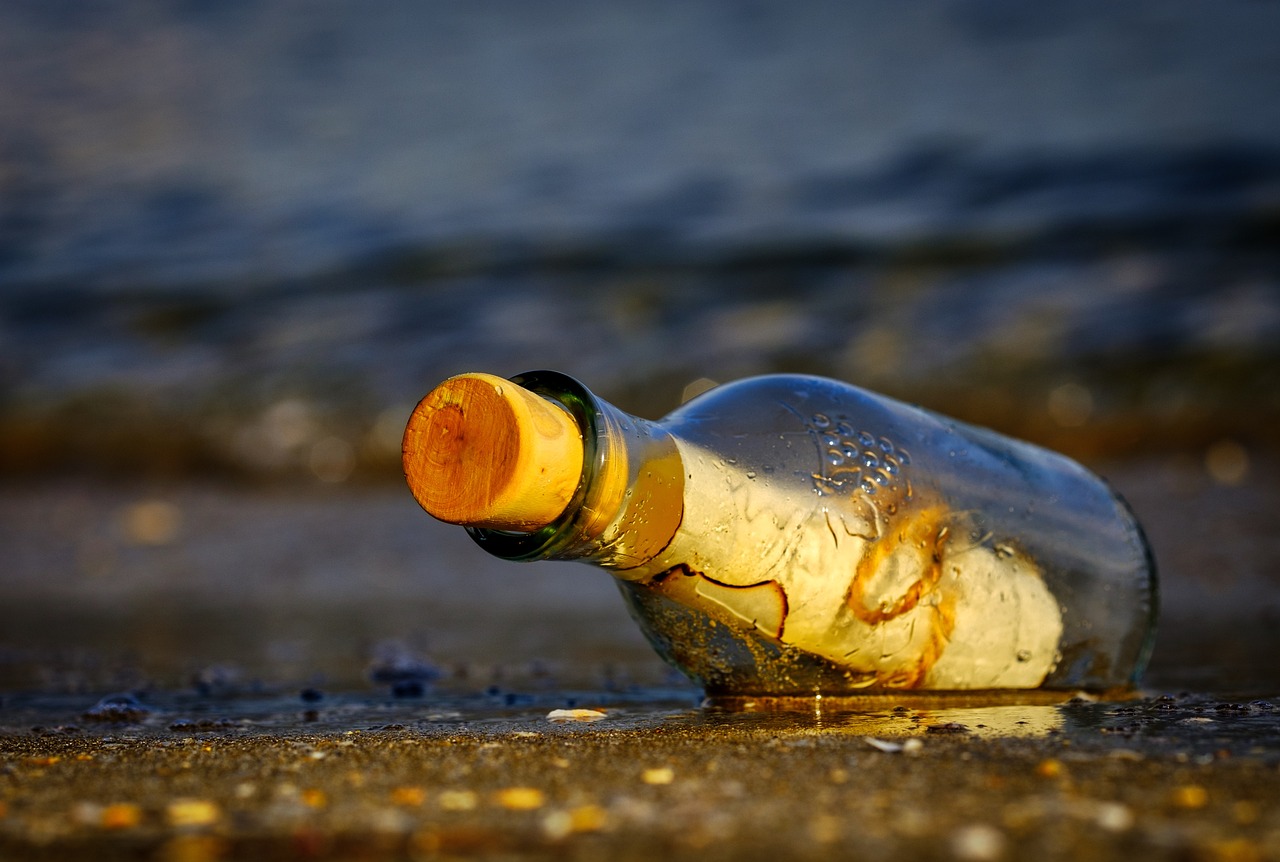
AI in Healthcare Decision-Making
In the ever-evolving landscape of healthcare, artificial intelligence (AI) is making waves by revolutionizing the way decisions are made. The integration of AI into healthcare decision-making processes is not just a trend; it's a transformative shift that enhances the quality of care provided to patients. Imagine walking into a hospital where the system can predict your health needs even before you arrive—this is the power of AI in action. By leveraging vast amounts of data, AI tools assist healthcare professionals in diagnosing diseases, personalizing treatment plans, and predicting patient outcomes with an unprecedented level of precision.
One of the most significant advantages of AI in healthcare is its ability to process and analyze large datasets quickly. This capability allows for predictive analytics, which helps healthcare providers anticipate patient needs and manage resources effectively. For instance, AI can identify trends in patient data that may indicate an increase in specific health issues within a community, enabling hospitals to prepare in advance. Consequently, this proactive approach not only improves patient care but also significantly reduces operational costs.
Predictive analytics powered by AI is a game changer. By analyzing historical data, AI algorithms can forecast potential health crises, helping healthcare organizations allocate resources more efficiently. For example, if data shows a spike in flu cases during a particular season, hospitals can prepare by increasing staff and stockpiling necessary medical supplies. This foresight allows healthcare providers to maintain a high standard of care while also managing costs effectively.
Machine learning algorithms are at the heart of AI's impact on healthcare decision-making. These algorithms analyze vast datasets, uncovering insights that help healthcare professionals make informed decisions. For instance, they can identify patterns in patient history that may suggest a predisposition to certain conditions, allowing for early intervention. The result? Better patient outcomes and more streamlined processes. It's like having a personal assistant who knows your health history better than you do, guiding you toward the best possible decisions.
Another remarkable application of AI in healthcare is in the realm of diagnostics. AI-assisted diagnostics utilize advanced imaging and data analysis techniques to support clinicians in identifying conditions more accurately and swiftly. Imagine a radiologist using AI to analyze X-rays or MRIs; the AI can highlight potential areas of concern that might be missed by the human eye, thus enhancing the overall diagnostic process. This collaboration between AI and healthcare professionals not only speeds up diagnoses but also improves accuracy, ultimately leading to better treatment plans and improved patient outcomes.
While the benefits of AI in healthcare are substantial, it is essential to recognize the challenges that come with its implementation. Issues such as data privacy and algorithmic bias must be addressed to ensure ethical practices. For instance, healthcare organizations must safeguard sensitive patient information while complying with regulations to maintain trust and integrity. Furthermore, addressing algorithmic bias is crucial to ensure fairness in AI-driven decisions. This requires ongoing evaluations and adjustments to algorithms to mitigate potential discrimination and inaccuracies.
- What is the role of AI in healthcare? AI enhances decision-making in healthcare by assisting in diagnostics, predicting patient outcomes, and personalizing treatment plans.
- How does predictive analytics work in healthcare? Predictive analytics uses historical data to forecast future health trends, allowing healthcare providers to prepare resources in advance.
- What are the challenges of using AI in healthcare? Challenges include data privacy concerns, algorithm bias, and the need for human oversight to maintain ethical standards.
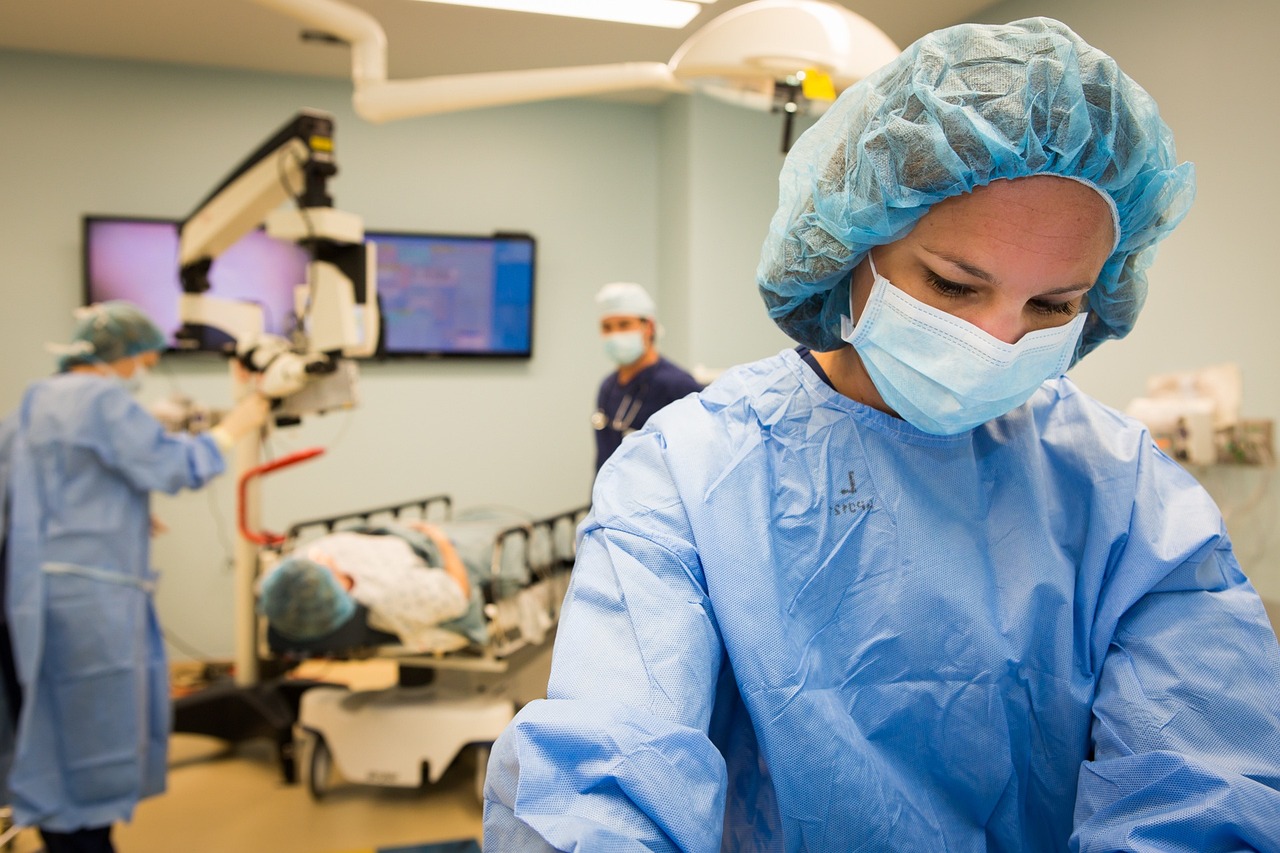
Predictive Analytics in Healthcare
Predictive analytics is a game-changer in the healthcare sector, leveraging the power of artificial intelligence to transform how medical professionals approach patient care. Imagine being able to foresee potential health issues before they even arise—sounds like something out of a science fiction movie, right? But this is exactly what predictive analytics does. By harnessing vast amounts of data, healthcare providers can identify patterns and trends that guide their decisions, ultimately leading to improved patient outcomes.
At its core, predictive analytics involves the use of complex algorithms and machine learning models to analyze historical and real-time data. This enables healthcare professionals to make informed decisions based on evidence rather than intuition alone. For instance, by examining data from previous patients, algorithms can predict which individuals are at higher risk for certain diseases, allowing for timely interventions. This proactive approach not only enhances patient care but also optimizes resource allocation within healthcare facilities.
One of the most significant advantages of predictive analytics is its ability to manage resources effectively. Hospitals often face challenges such as overcrowding and limited staffing. By predicting patient inflow and identifying peak times for services, healthcare administrators can better allocate resources, ensuring that patients receive the care they need when they need it. This not only improves operational efficiency but also enhances the overall patient experience.
Moreover, predictive analytics can significantly reduce healthcare costs. By identifying high-risk patients early, healthcare providers can implement preventive measures that avoid costly treatments down the line. For example, a patient with a history of heart issues might benefit from regular check-ups and lifestyle interventions, which can prevent the need for expensive surgeries or emergency care later. This not only saves money but also contributes to better health outcomes for patients.
To illustrate the impact of predictive analytics, consider the following table that outlines key benefits:
Benefit | Description |
---|---|
Early Intervention | Identifying at-risk patients allows for timely medical interventions. |
Resource Optimization | Improved staffing and resource allocation based on predicted patient inflow. |
Cost Reduction | Preventive measures reduce the need for expensive treatments. |
Enhanced Patient Experience | Proactive care leads to higher patient satisfaction. |
However, while the benefits are substantial, the implementation of predictive analytics is not without its challenges. Data privacy and security are paramount concerns, as sensitive patient information must be protected at all costs. Additionally, the accuracy of predictive models relies heavily on the quality of data input; poor data can lead to misleading predictions and, consequently, suboptimal patient care.
In conclusion, predictive analytics is revolutionizing the way healthcare providers make decisions. By anticipating patient needs and optimizing resources, it not only improves patient outcomes but also enhances the overall efficiency of healthcare systems. As technology continues to advance, the potential for predictive analytics in healthcare is boundless, paving the way for a future where data-driven decisions are the norm.
- What is predictive analytics in healthcare? Predictive analytics in healthcare refers to the use of data analysis techniques to predict future patient outcomes and improve decision-making.
- How does predictive analytics benefit patients? It allows for early intervention, personalized treatment plans, and better management of healthcare resources, ultimately enhancing patient care.
- What challenges does predictive analytics face? Key challenges include data privacy concerns, the need for high-quality data, and ensuring the accuracy of predictive models.
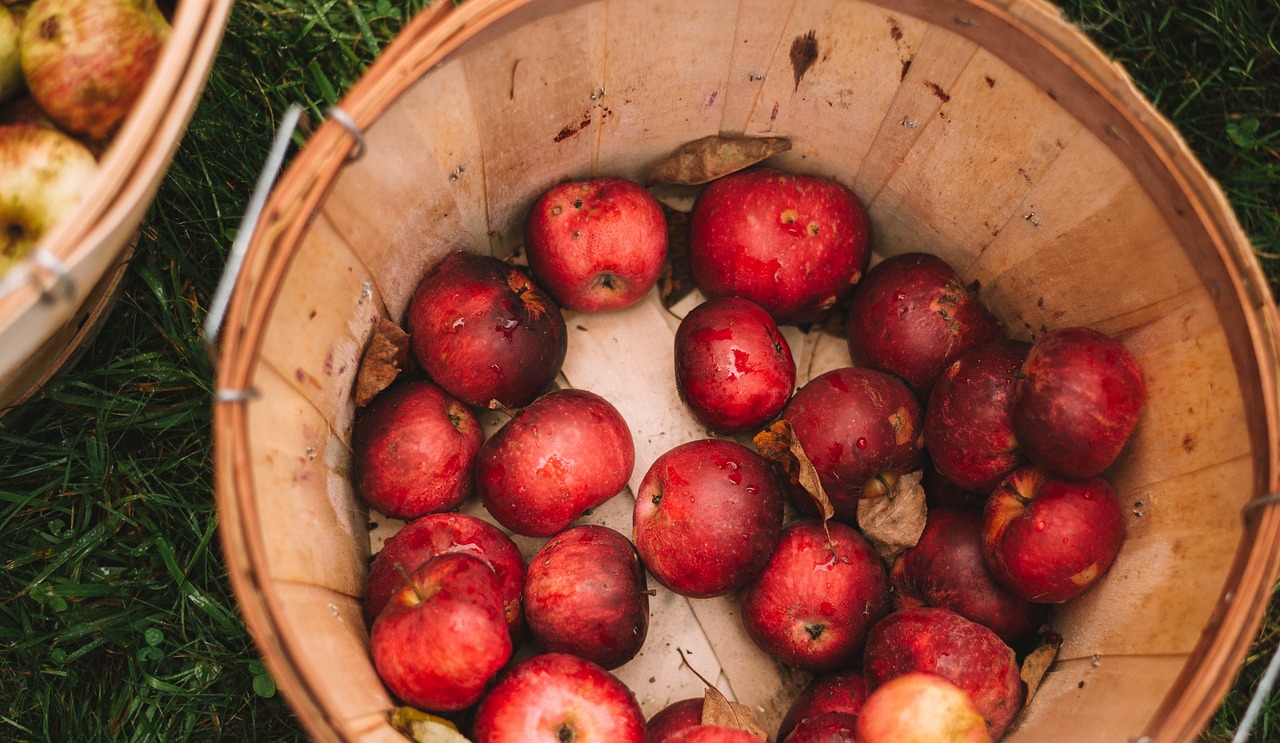
Machine Learning Algorithms
Machine learning algorithms are at the forefront of transforming healthcare decision-making. These algorithms are designed to analyze massive datasets, uncovering patterns and insights that might be invisible to the human eye. Imagine having a super-smart assistant that can sift through years of patient records, medical literature, and real-time data in the blink of an eye. That's the kind of power machine learning brings to the table!
One of the remarkable aspects of machine learning is its ability to learn and improve over time. As these algorithms process more data, they become increasingly adept at predicting patient outcomes and identifying potential health risks. For instance, a machine learning model trained on historical patient data can help doctors predict which patients might be at risk for conditions like diabetes or heart disease. This not only empowers healthcare providers to intervene early but also fosters a more proactive approach to patient care.
Furthermore, machine learning algorithms can be categorized into different types based on their learning styles. Here’s a quick breakdown:
- Supervised Learning: This involves training the algorithm on labeled datasets, where the outcome is known. For example, a model can learn to classify images of tumors based on previous examples.
- Unsupervised Learning: Here, the algorithm identifies patterns in data without prior labeling. This can be particularly useful for clustering similar patient profiles based on symptoms.
- Reinforcement Learning: This type of learning utilizes feedback from actions to improve decision-making over time, much like how a child learns from their experiences.
The implications of these algorithms in healthcare are profound. They not only enhance diagnostic accuracy but also streamline various processes, reducing the time healthcare professionals spend on administrative tasks. By automating routine functions, these algorithms free up valuable time for clinicians, allowing them to focus on what truly matters: patient care.
Moreover, the integration of machine learning algorithms into clinical workflows can lead to improved operational efficiency. For instance, hospitals that employ predictive analytics can better manage their resources, ensuring that they have the right staff and equipment available when needed. This can significantly reduce wait times and improve patient satisfaction, creating a win-win situation for both healthcare providers and patients.
In conclusion, machine learning algorithms are not just a technological advancement; they represent a paradigm shift in how healthcare decisions are made. By harnessing the power of these algorithms, healthcare professionals can deliver more personalized care, anticipate patient needs, and ultimately improve health outcomes. The future of healthcare is undoubtedly intertwined with the capabilities of machine learning, and the possibilities are as exciting as they are transformative!
Q: What are machine learning algorithms?
A: Machine learning algorithms are computational models that learn from data to make predictions or decisions without being explicitly programmed for specific tasks.
Q: How do machine learning algorithms benefit healthcare?
A: They improve diagnostic accuracy, personalize treatment plans, and enhance operational efficiency by analyzing vast amounts of patient data to uncover insights.
Q: What types of machine learning are used in healthcare?
A: The main types include supervised learning, unsupervised learning, and reinforcement learning, each serving different purposes in data analysis and decision-making.
Q: Can machine learning algorithms replace healthcare professionals?
A: No, they are designed to assist healthcare professionals by providing insights and predictions, allowing clinicians to make better-informed decisions.
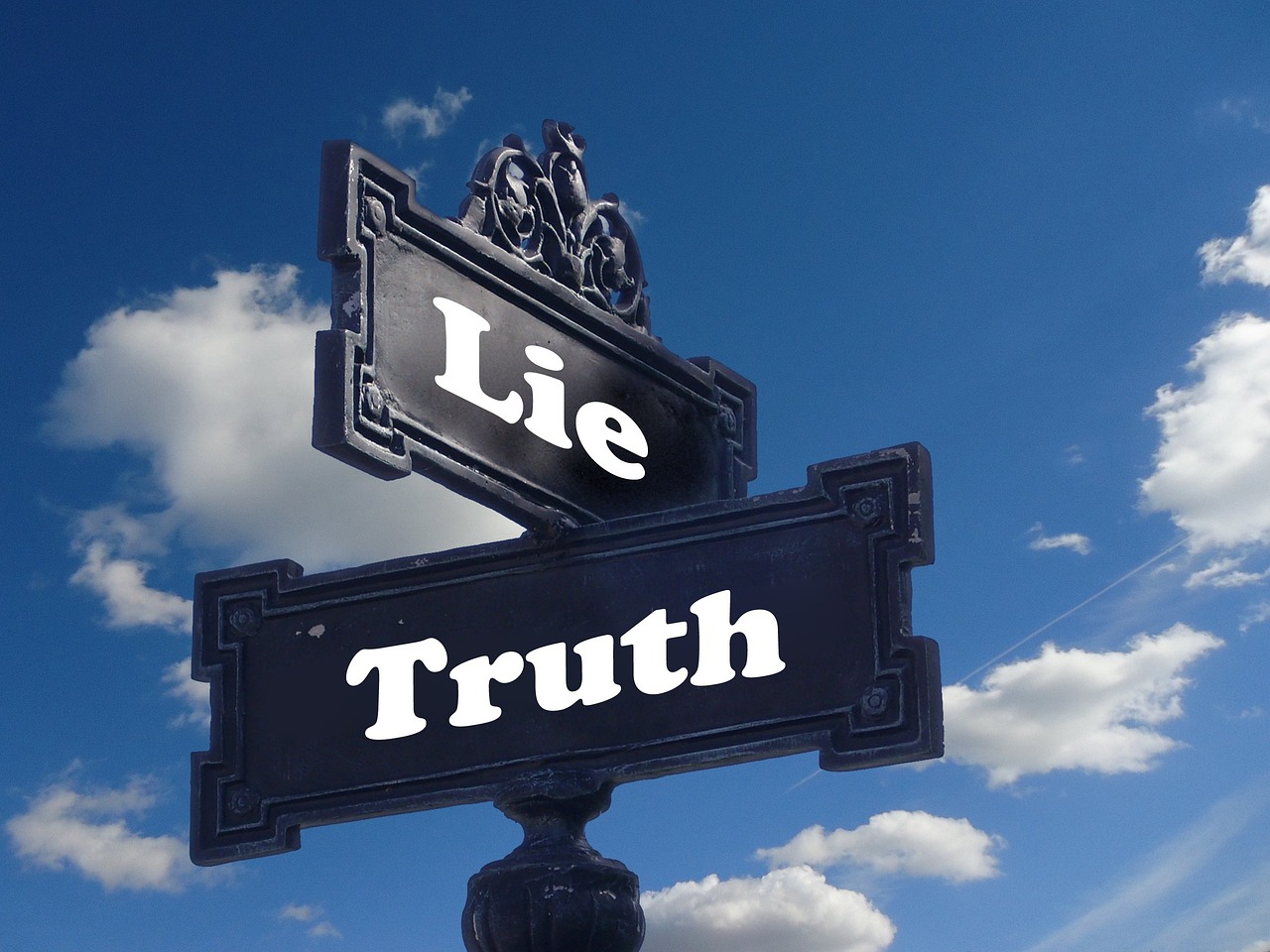
AI-Assisted Diagnostics
In today's fast-paced medical landscape, are emerging as a game-changer, revolutionizing the way healthcare professionals identify and treat diseases. Imagine a world where doctors have access to advanced technology that can analyze medical images and patient data faster than the blink of an eye. That’s the power of AI. By leveraging sophisticated algorithms, AI systems can quickly sift through vast amounts of data, providing clinicians with insights that were previously unimaginable.
One of the most striking features of AI-assisted diagnostics is its ability to enhance accuracy. Traditional diagnostic methods can sometimes lead to human error, whether due to fatigue, oversight, or misinterpretation of data. AI, on the other hand, works tirelessly, analyzing patterns and anomalies in data that might escape human notice. For instance, in radiology, AI algorithms can detect subtle changes in X-rays or MRIs that could indicate the early stages of cancer, potentially saving lives through earlier intervention.
Furthermore, AI-assisted diagnostics do not operate in isolation; they complement the expertise of healthcare professionals. Think of AI as a highly skilled assistant that provides additional insights, allowing doctors to make more informed decisions. This collaboration between human intuition and machine precision creates a powerful synergy that enhances the overall diagnostic process.
Additionally, AI can significantly reduce the time it takes to arrive at a diagnosis. In emergency situations, every second counts. With AI, clinicians can quickly analyze data and make faster decisions, which can be crucial for patient outcomes. For example, AI systems can triage patients based on their symptoms and medical history, ensuring that those in critical need receive immediate attention.
However, it is essential to remember that while AI brings remarkable benefits, it is not without challenges. The integration of AI into diagnostics requires careful consideration of ethical implications, data privacy, and the need for ongoing human oversight. As we embrace this technology, we must ensure that it is used responsibly and effectively, maintaining the trust between patients and healthcare providers.
In conclusion, AI-assisted diagnostics are paving the way for a future where healthcare is not only more efficient but also more precise. By harnessing the power of AI, we can enhance the diagnostic process, ultimately leading to better patient outcomes and a more effective healthcare system.
- What is AI-assisted diagnostics?
AI-assisted diagnostics refers to the use of artificial intelligence technologies to support healthcare professionals in diagnosing diseases more accurately and efficiently. - How does AI improve diagnostic accuracy?
AI analyzes vast datasets and medical images to identify patterns and anomalies that may not be readily apparent to human clinicians, thus enhancing diagnostic accuracy. - Are there any risks associated with AI-assisted diagnostics?
Yes, there are risks such as data privacy concerns, potential algorithm bias, and the need for human oversight to ensure ethical practices. - Can AI completely replace human doctors?
No, AI is designed to assist and enhance the capabilities of healthcare professionals, not to replace them. The combination of human expertise and AI technology leads to the best outcomes.

AI in Financial Decision-Making
Artificial Intelligence is making waves in the financial sector, and it’s not just a ripple; it’s a full-blown tsunami of change! Imagine a world where decisions are not solely based on gut feelings or outdated data but on real-time analytics and predictive modeling. That’s the power of AI in financial decision-making. With the ability to sift through mountains of data in seconds, AI algorithms can analyze market trends and consumer behavior with remarkable accuracy. This capability allows investors and financial institutions to make informed decisions that can significantly impact their bottom line.
One of the most exciting aspects of AI in finance is its ability to enhance risk management. By leveraging AI, financial institutions can identify potential risks before they become major issues. For example, AI systems can analyze historical data to predict future market fluctuations, enabling firms to adjust their strategies proactively. This not only helps in mitigating risks but also in capitalizing on opportunities as they arise. Think of it like having a crystal ball that provides insights into the financial future—who wouldn’t want that?
Moreover, AI can automate routine tasks, allowing financial professionals to focus on more strategic initiatives. For instance, AI-powered chatbots can handle customer inquiries, freeing up human agents to tackle more complex issues. This not only improves efficiency but also enhances the customer experience. Imagine calling your bank and being greeted by a friendly AI that understands your needs and provides immediate assistance. It’s a win-win situation!
To illustrate the impact of AI on financial decision-making, let's take a look at a table that highlights some key applications:
Application | Description | Benefits |
---|---|---|
Fraud Detection | AI systems analyze transaction patterns to identify anomalies. | Early detection of fraudulent activities, reducing losses. |
Portfolio Management | AI algorithms optimize investment portfolios based on risk tolerance. | Maximized returns with minimized risks. |
Credit Scoring | AI evaluates creditworthiness using alternative data sources. | More accurate assessments leading to better loan approvals. |
However, while the benefits of AI in financial decision-making are substantial, it’s essential to approach this technology with caution. The algorithms that drive AI are only as good as the data fed into them. If the data is biased or flawed, the outcomes can be misleading. Therefore, it’s crucial for financial institutions to continually monitor and refine their AI systems to ensure they are making fair and accurate decisions.
In conclusion, AI is reshaping the landscape of financial decision-making, providing tools that enhance accuracy, efficiency, and risk management. As we move forward, the integration of AI into finance will likely continue to grow, making it an exciting area to watch. So, whether you’re an investor, a financial analyst, or just someone interested in the future of finance, embracing AI could be the key to unlocking new opportunities.
- How does AI improve financial decision-making? AI enhances financial decision-making by providing real-time analytics, automating routine tasks, and improving risk management through predictive modeling.
- What are some applications of AI in finance? Applications include fraud detection, portfolio management, and credit scoring, each contributing to more informed and efficient decision-making.
- Are there risks associated with AI in finance? Yes, risks include algorithmic bias and reliance on flawed data, which can lead to inaccurate outcomes. Continuous monitoring and refinement are necessary to mitigate these risks.
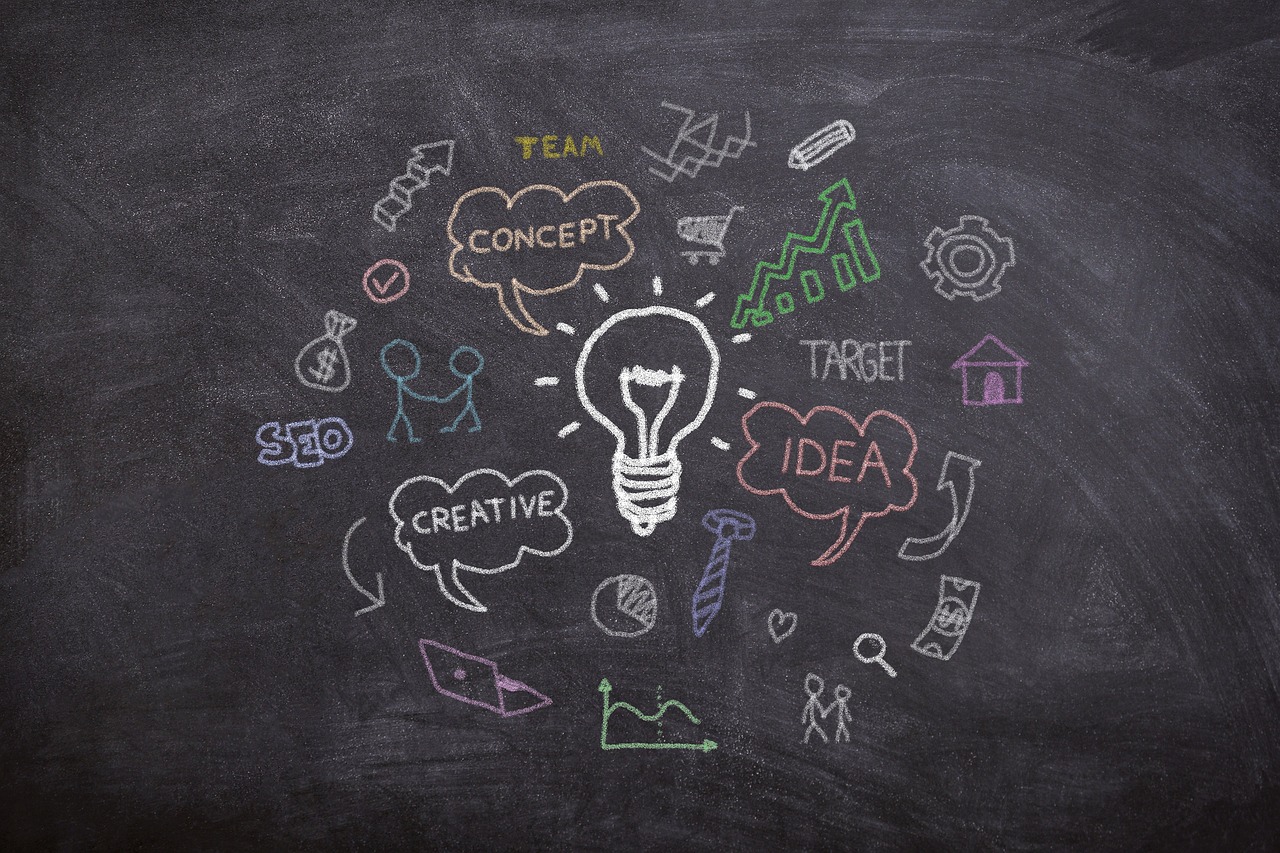
Challenges of Implementing AI in Decision-Making
As we dive deeper into the world of artificial intelligence (AI), it's essential to recognize that with great power comes great responsibility. While AI offers remarkable opportunities for enhancing decision-making processes, it also presents a plethora of challenges that organizations must navigate. One of the most pressing concerns is data privacy and security. In an age where information is invaluable, protecting sensitive data is paramount. Organizations need to ensure that they comply with stringent regulations, such as GDPR, to maintain trust and integrity with their users. The mishandling of data can lead to catastrophic consequences, not just for the organization but also for the individuals whose information is compromised.
Moreover, the integration of AI systems can inadvertently lead to algorithmic bias. This bias can stem from various sources, including the data used to train the algorithms and the design of the algorithms themselves. For instance, if an AI model is trained on historical data that reflects societal biases, it may perpetuate these biases in its decision-making processes. This raises ethical concerns and necessitates ongoing evaluations and adjustments to mitigate potential discrimination and inaccuracies. Organizations must be vigilant in ensuring that their AI systems promote fairness and equity, rather than reinforce existing biases.
Additionally, the need for human oversight cannot be overstated. AI systems, while powerful, are not infallible. They can make mistakes or misinterpret data, leading to poor decision-making. Therefore, human oversight is crucial to ensure that AI recommendations align with ethical standards and organizational goals. This human-AI collaboration can also foster a culture of accountability, where decisions are not solely reliant on machines but are informed by human judgment and expertise.
Another challenge lies in the integration of AI technologies into existing workflows. Many organizations struggle with the transition from traditional decision-making processes to AI-driven ones. This can lead to resistance from employees who may feel threatened by the technology or unsure of how to adapt. To overcome this, organizations should invest in training and development programs that empower their workforce to leverage AI tools effectively. By fostering a culture of innovation and continuous learning, companies can alleviate fears and encourage a more seamless integration of AI into their operations.
In summary, while the potential of AI in decision-making is vast, organizations must tread carefully. Addressing data privacy concerns, combating algorithmic bias, ensuring human oversight, and facilitating smooth integration are all critical steps in harnessing the true power of AI. It’s a balancing act that requires diligence, transparency, and a commitment to ethical practices.
- What are the main challenges of implementing AI in decision-making?
The primary challenges include data privacy and security, algorithmic bias, the necessity of human oversight, and the integration of AI technologies into existing workflows. - How can organizations address data privacy concerns?
Organizations should implement robust data protection measures, comply with regulations like GDPR, and maintain transparency with users about how their data is used. - What is algorithmic bias, and why is it a concern?
Algorithmic bias occurs when AI systems reflect historical biases present in training data, leading to unfair or discriminatory outcomes. It is a concern because it can perpetuate inequality and undermine trust in AI systems. - Why is human oversight important in AI decision-making?
Human oversight ensures that AI recommendations are aligned with ethical standards and organizational goals, and it helps to catch errors or misinterpretations made by AI systems.
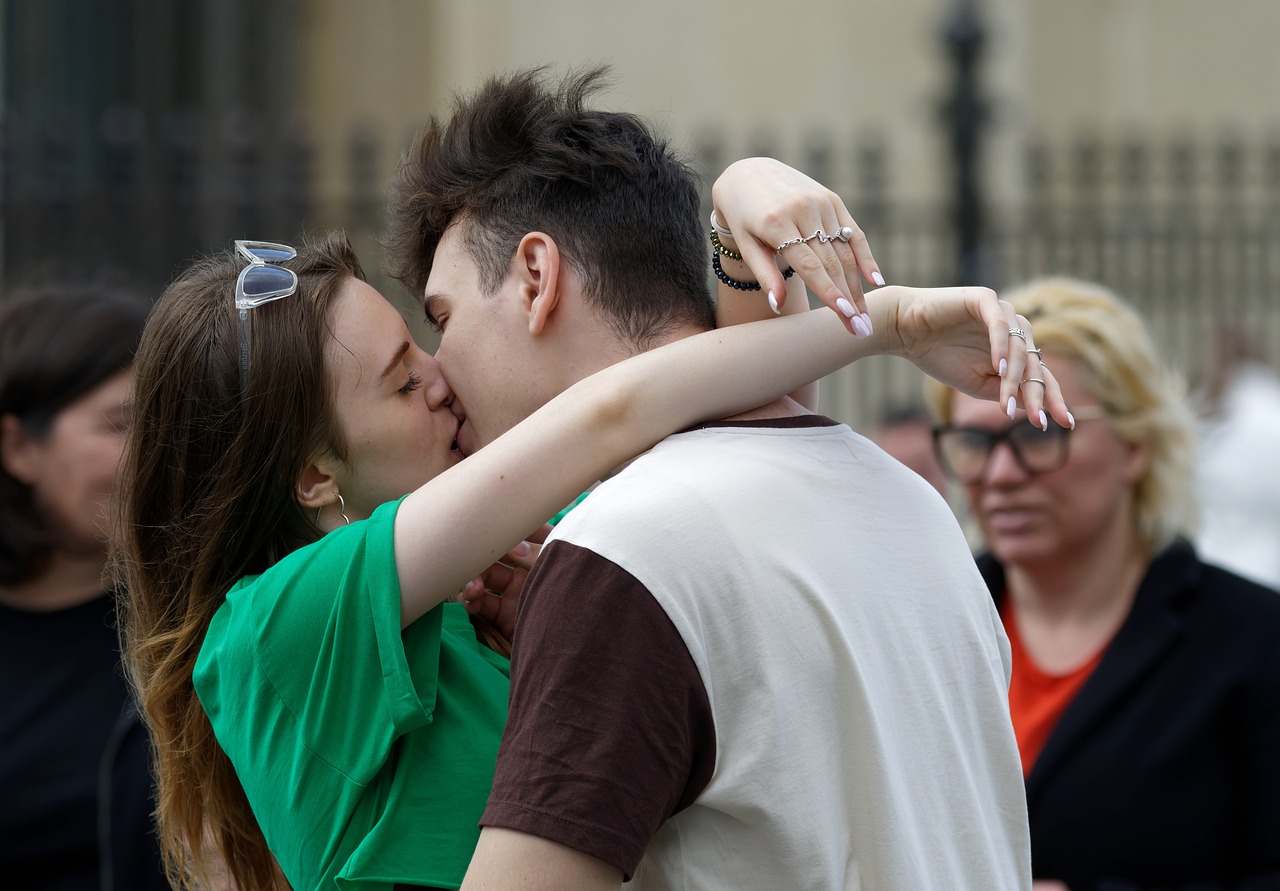
Data Privacy and Security
In today's digital age, where data is often referred to as the new oil, have become paramount concerns, especially when integrating AI into decision-making processes. Organizations are inundated with vast amounts of sensitive information, ranging from personal health records to financial details. As AI systems analyze this data to deliver insights, the risk of data breaches and unauthorized access looms large. This reality raises a critical question: how can organizations ensure that they protect sensitive information while leveraging the power of AI?
First and foremost, organizations must implement robust data governance frameworks. This includes establishing clear policies regarding data collection, storage, and usage. By doing so, they can ensure that data is only accessed by authorized personnel and is used in compliance with legal regulations such as GDPR or HIPAA. Additionally, employing encryption methods can safeguard data both in transit and at rest, acting as a formidable barrier against potential cyber threats.
Moreover, organizations should conduct regular audits and assessments of their AI systems. These evaluations help identify vulnerabilities and ensure that data protection measures are up to date. It's essential to adopt a proactive approach rather than a reactive one. After all, as the saying goes, "An ounce of prevention is worth a pound of cure." By staying ahead of potential risks, businesses can maintain customer trust and avoid the hefty fines associated with data breaches.
Another crucial aspect is training employees on data privacy protocols. Even the most secure systems can be compromised by human error. Therefore, organizations should invest in comprehensive training programs that educate staff about the importance of data security and the best practices for handling sensitive information. This can include:
- Understanding the significance of strong passwords and two-factor authentication.
- Recognizing phishing attempts and other social engineering tactics.
- Knowing the proper procedures for reporting suspicious activities.
Finally, transparency with customers is vital. Organizations should communicate openly about how their data is being used, what measures are in place to protect it, and how individuals can exercise control over their data. This not only builds trust but also empowers customers to make informed decisions about their personal information.
In summary, while AI offers incredible opportunities for enhancing decision-making, it also presents significant challenges regarding data privacy and security. By prioritizing robust governance frameworks, conducting regular audits, training employees, and maintaining transparency with customers, organizations can navigate these challenges effectively. The balance between leveraging AI's potential and safeguarding sensitive information is delicate, but with the right strategies in place, it is achievable.
- What are the main concerns regarding data privacy in AI?
Organizations must worry about data breaches, unauthorized access, and compliance with regulations. - How can organizations protect sensitive data?
Implementing strong data governance, encryption, regular audits, and employee training are essential steps. - Why is transparency important in data handling?
Transparency builds trust with customers and allows them to make informed decisions about their personal data.
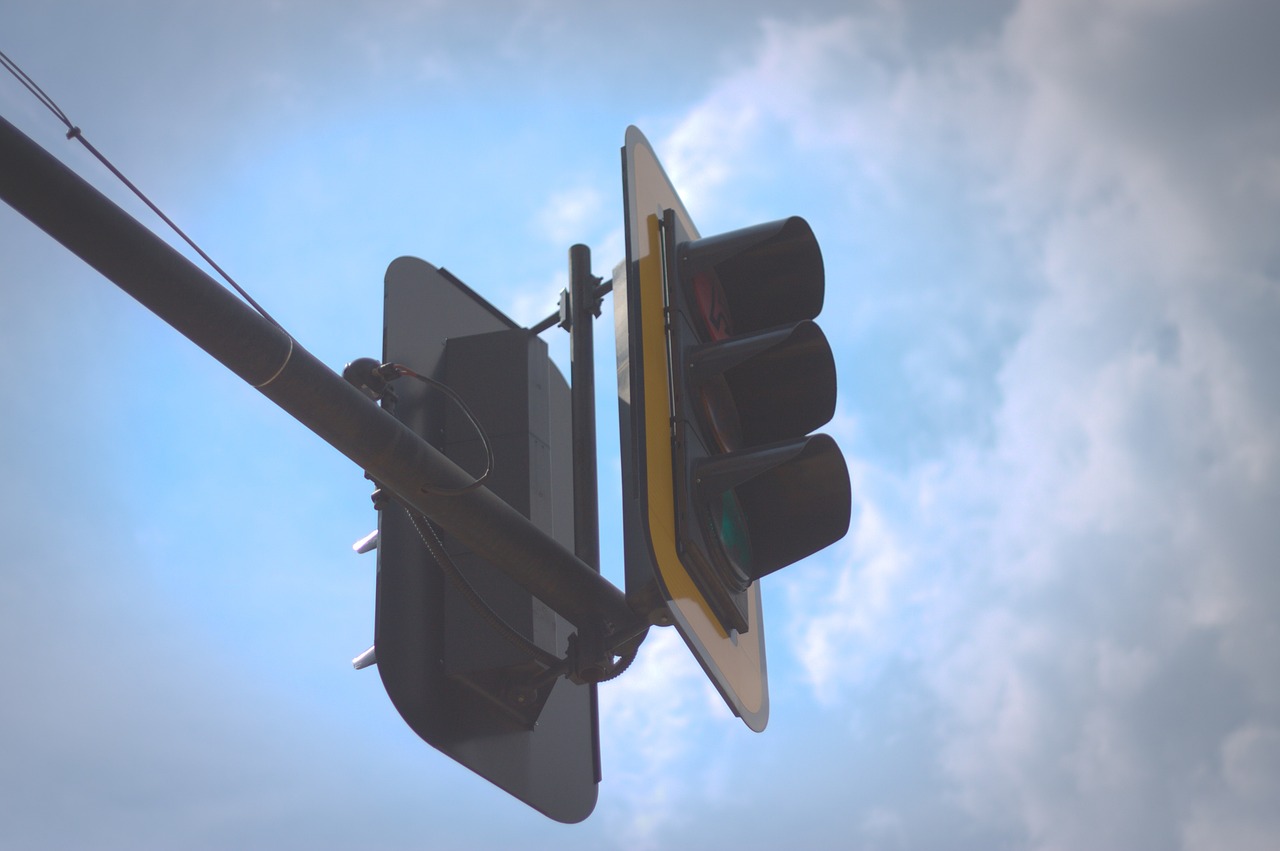
Addressing Algorithmic Bias
In the realm of artificial intelligence, algorithmic bias poses a significant challenge that can skew decision-making processes and lead to unfair outcomes. This bias often arises from the data used to train AI models, which may reflect historical inequalities or societal prejudices. For instance, if an AI system is trained on data that predominantly features one demographic, it may not perform as well for individuals from different backgrounds, inadvertently perpetuating existing disparities. Addressing algorithmic bias is not just a technical issue; it’s a moral imperative.
To effectively tackle this issue, organizations must adopt a multi-faceted approach. First and foremost, diversifying training data is crucial. By ensuring that the data used to train AI systems is representative of various demographics, organizations can minimize the risk of bias. This may involve collecting additional data from underrepresented groups or employing techniques to augment existing datasets.
Moreover, continuous monitoring and evaluation of AI systems are essential. Organizations should implement regular audits to assess the performance of their algorithms across different demographics. This can help identify any discrepancies in outcomes and allow for timely adjustments. For example, if an AI hiring tool is found to favor one gender over another, it’s vital to revisit the training data and algorithms to rectify this bias.
Another effective strategy is to involve diverse teams in the development of AI systems. When teams consist of individuals from various backgrounds, perspectives, and experiences, they are more likely to recognize potential biases and address them proactively. This collaborative approach fosters an environment of accountability and encourages ethical practices in AI development.
Finally, transparency in AI decision-making processes is imperative. Organizations should strive to be open about how their algorithms function, including the data sources and methodologies used. This transparency not only builds trust with users but also allows for external scrutiny, which can help identify and rectify biases that may have been overlooked. By fostering a culture of openness, organizations can ensure that their AI systems are held to high ethical standards.
In conclusion, addressing algorithmic bias requires a concerted effort from all stakeholders involved in AI development and deployment. By diversifying data, implementing regular audits, involving diverse teams, and maintaining transparency, organizations can create AI systems that are not only effective but also fair and equitable for all users.
- What is algorithmic bias? Algorithmic bias refers to systematic and unfair discrimination in AI decision-making processes, often stemming from biased training data.
- How can organizations reduce algorithmic bias? Organizations can reduce bias by diversifying training data, conducting regular audits, involving diverse teams, and ensuring transparency in AI processes.
- Why is addressing algorithmic bias important? It is vital to address algorithmic bias to ensure fairness, equity, and trust in AI systems, preventing the perpetuation of existing societal inequalities.
Frequently Asked Questions
- What is the role of AI in decision-making?
AI plays a transformative role in decision-making by providing data-driven insights that enhance efficiency and accuracy. It helps businesses and professionals analyze vast amounts of data quickly, leading to better-informed decisions across various sectors.
- How does AI improve business decisions?
AI improves business decisions by enabling organizations to adopt data-driven strategies. This means they can analyze customer behavior, market trends, and operational efficiencies to make more accurate and timely decisions, ultimately driving growth and competitiveness.
- Can AI assist in healthcare decision-making?
Absolutely! AI assists healthcare professionals in diagnosing diseases, personalizing treatment plans, and predicting patient outcomes. By analyzing patient data, AI enhances the quality of care and operational efficiency within healthcare systems.
- What is predictive analytics in healthcare?
Predictive analytics in healthcare uses AI to anticipate patient needs and manage resources effectively. By identifying trends and patterns in patient data, healthcare providers can reduce costs and improve patient care outcomes.
- What challenges does AI face in decision-making?
AI faces several challenges in decision-making, including data privacy concerns, algorithm bias, and the need for human oversight. These challenges must be addressed to ensure ethical practices and maintain trust in AI systems.
- How important is data privacy in AI?
Data privacy is crucial when implementing AI systems. Organizations must safeguard sensitive information to comply with regulations and maintain trust with their customers. Failing to protect data can lead to significant repercussions.
- What is algorithmic bias, and why is it a concern?
Algorithmic bias refers to the unintended discrimination that can arise from AI algorithms. It’s a concern because biased algorithms can lead to unfair decisions, impacting individuals and communities. Continuous evaluation and adjustment of algorithms are necessary to mitigate these risks.
- How does AI enhance financial decision-making?
In finance, AI analyzes market trends and consumer behavior to provide insights that help investors and financial institutions make informed decisions. This capability enables better risk management and investment strategies, ultimately leading to improved financial outcomes.