Using Sentiment Analysis to Understand Public Opinion
In today's fast-paced digital world, understanding public opinion has never been more critical. The ability to gauge how people feel about issues, brands, and policies can significantly impact decision-making processes across various sectors. Enter sentiment analysis, a powerful tool that allows businesses, governments, and organizations to dive deep into the emotional undertones of public discourse. By employing sophisticated algorithms and data analytics, sentiment analysis helps to uncover the collective feelings of a population, transforming raw text data into actionable insights.
But what exactly is sentiment analysis? At its core, it is a computational technique designed to determine the emotional tone behind a series of words. This method helps us understand attitudes, opinions, and emotions expressed in text data, whether from social media posts, customer reviews, or news articles. Imagine being able to sift through thousands of tweets or comments and pinpoint whether the overall sentiment is positive, negative, or neutral. This capability is not just fascinating; it’s incredibly valuable for shaping strategies in various fields.
As we delve deeper into the importance of sentiment analysis, it becomes clear that its applications are vast and varied. In the realm of marketing, businesses leverage sentiment analysis to gauge consumer reactions to products and campaigns. This real-time feedback allows them to tailor their marketing strategies, ensuring they resonate with their audience. For instance, if a new product launch receives a flurry of negative comments online, companies can pivot quickly, addressing concerns and improving customer engagement.
Moreover, sentiment analysis plays a critical role in politics. Politicians and political analysts utilize it to track public opinion on policies and candidates. By analyzing sentiment data, they can adjust their communication strategies to better connect with voters. Imagine a politician who, after analyzing social media sentiment, discovers a growing concern among constituents regarding healthcare policies. This insight empowers them to address the issue directly, fostering trust and engagement.
Despite its advantages, sentiment analysis is not without challenges. One major hurdle is the detection of sarcasm and contextual nuances in language. A statement like "Oh great, another meeting" could be interpreted as positive if taken literally, but sentiment analysis must recognize the underlying sarcasm. Additionally, the need for language-specific models is crucial to ensure the accuracy of interpretation across different languages and dialects.
As we look towards the future, the evolution of sentiment analysis is promising. With advancements in artificial intelligence and machine learning, we can expect enhanced accuracy and broader applications across various fields. This evolution will not only improve our understanding of public sentiment but also ensure that insights are accessible and relevant across diverse populations.
- What is sentiment analysis? Sentiment analysis is a computational technique used to determine the emotional tone behind a series of words, helping to understand attitudes, opinions, and emotions expressed in text data.
- How is sentiment analysis used in marketing? Businesses use sentiment analysis to gauge consumer reactions to products and campaigns, allowing them to tailor marketing strategies based on real-time feedback.
- What challenges does sentiment analysis face? Some challenges include sarcasm detection, context understanding, and the need for language-specific models to ensure accuracy in interpretation.
- What is the future of sentiment analysis? The future of sentiment analysis is expected to incorporate advancements in AI and machine learning, enhancing accuracy and broadening its applications.
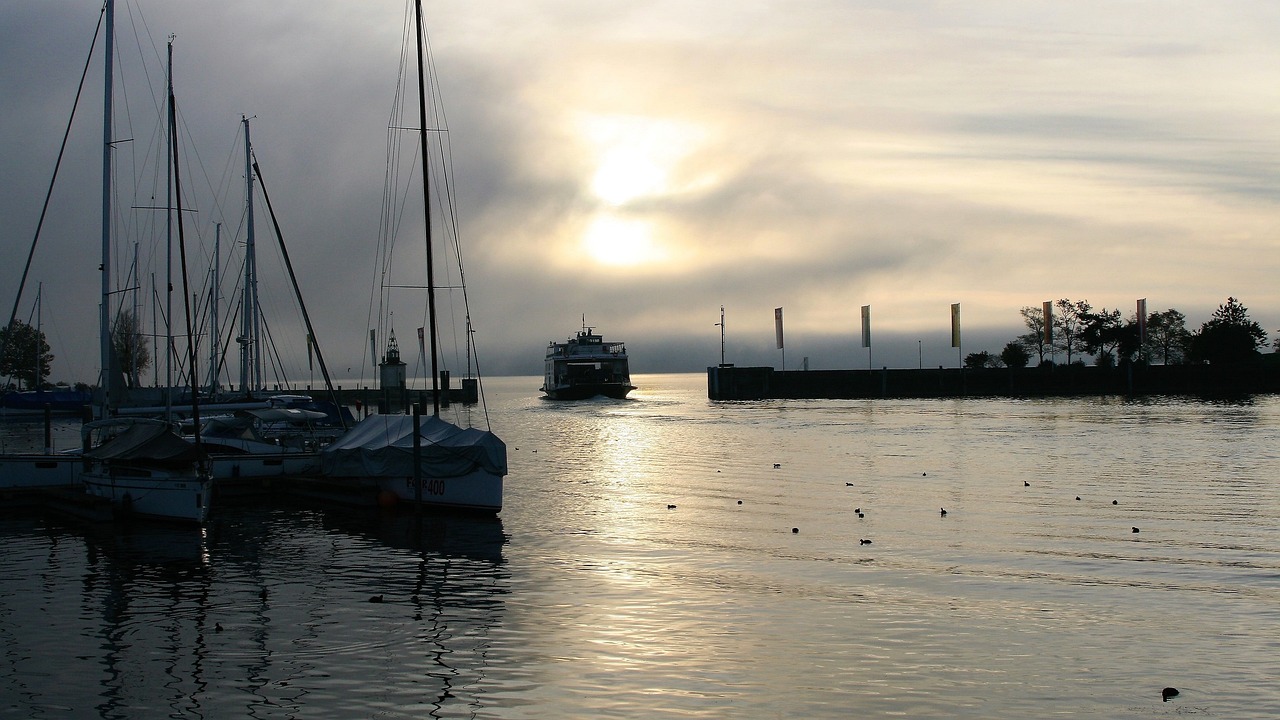
What is Sentiment Analysis?
Sentiment analysis, often referred to as opinion mining, is a fascinating computational technique that dives deep into the emotional tone behind a series of words. Imagine being able to sift through mountains of text data—whether it's tweets, reviews, or comments—and extract the underlying feelings of the writers. This is precisely what sentiment analysis accomplishes. By employing various algorithms and natural language processing (NLP) techniques, it helps us understand not just what people are saying, but how they truly feel about a subject.
This methodology is akin to having a digital emotional radar that can detect nuances in language. For instance, consider the difference between the phrases "I love this product!" and "I hate that product!" The first phrase radiates positivity, while the latter is steeped in negativity. Sentiment analysis helps to categorize such sentiments into positive, negative, or neutral, enabling businesses and organizations to gauge public opinion effectively.
But how does it work? At its core, sentiment analysis involves several steps:
- Data Collection: Gathering text data from various sources such as social media, reviews, and forums.
- Preprocessing: Cleaning the data to remove any noise, such as irrelevant characters or formatting issues.
- Sentiment Detection: Utilizing algorithms to classify the emotional tone of the text.
- Analysis and Interpretation: Analyzing the results to draw meaningful insights about public sentiment.
To illustrate, let's look at a simple example of how sentiment analysis can be applied:
Text Sample | Sentiment |
---|---|
I am thrilled with the new features! | Positive |
The service was terrible and disappointing. | Negative |
It was okay, nothing special. | Neutral |
In today’s digital age, the sheer volume of text produced daily is staggering. From social media chatter to customer reviews, sentiment analysis serves as a powerful tool for extracting valuable insights from this data deluge. By understanding the emotional landscape of their audience, businesses can tailor their strategies to better meet customer needs and expectations. It's like having a compass that guides decision-making by revealing the collective feelings of a population.
In summary, sentiment analysis is more than just a buzzword; it's a vital instrument for interpreting public opinion and emotional trends in a world overflowing with information. As we continue to explore its applications and implications, it becomes increasingly clear that understanding sentiment is essential for anyone looking to connect meaningfully with their audience.
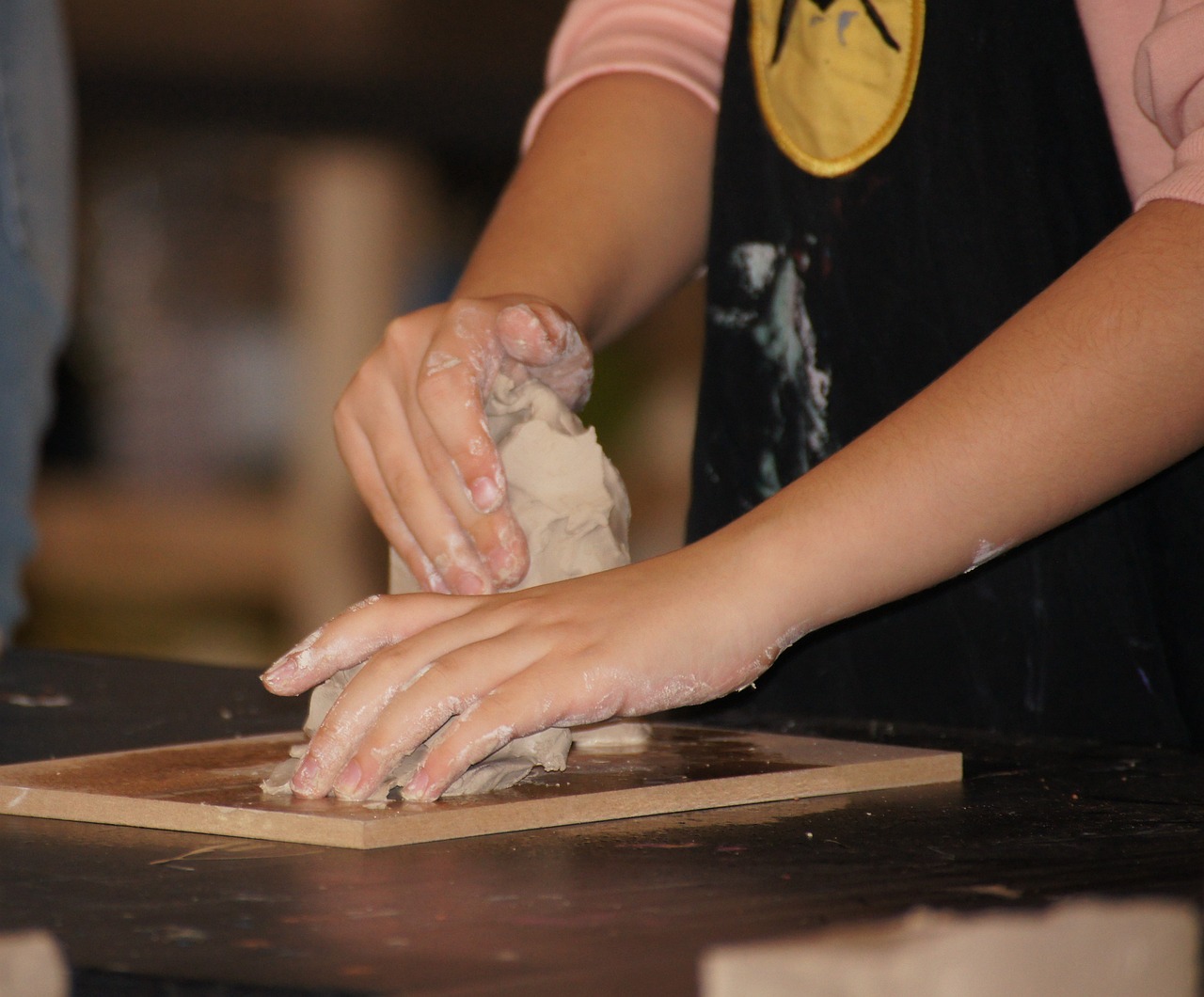
Importance of Sentiment Analysis in Public Opinion
Understanding public sentiment is crucial for businesses and governments alike. In today's fast-paced digital world, where opinions are shared in real-time across social media platforms and online forums, sentiment analysis serves as a powerful tool to gauge the collective feelings of a population. By analyzing the emotional tone behind words, organizations can make informed decisions that resonate with their audience. Imagine a ship navigating through stormy seas; sentiment analysis acts as the compass, guiding businesses and policymakers towards calmer waters.
For businesses, sentiment analysis can unlock a treasure trove of insights. It allows them to:
- Enhance Customer Engagement: By understanding how customers feel about their products or services, businesses can tailor their marketing strategies to better meet consumer expectations.
- Improve Product Development: Feedback derived from sentiment analysis can inform product improvements, ensuring that offerings align with consumer desires.
- Monitor Brand Reputation: Companies can quickly respond to negative sentiments, mitigating potential damage and fostering loyalty among their customer base.
On the other hand, governments and political entities can utilize sentiment analysis to track public opinion on policies and candidates. This capability allows them to adjust their strategies and communication to better connect with voters. For instance, during an election campaign, a political party can analyze social media sentiments to identify which issues resonate most with the electorate. This data-driven approach can significantly enhance campaign effectiveness.
Furthermore, sentiment analysis can aid in policy formulation by providing insights into public concerns and priorities. Imagine a city government considering a new public transportation initiative. By analyzing public sentiment, they can gauge community support and identify potential objections before rolling out the plan, ultimately leading to more successful implementations.
In summary, the importance of sentiment analysis in understanding public opinion cannot be overstated. It empowers organizations to make data-driven decisions, enhances customer relationships, and fosters a deeper connection between governments and their constituents. As we continue to navigate an increasingly interconnected world, the ability to comprehend and respond to public sentiment will remain a vital asset.
- What is sentiment analysis? Sentiment analysis is a computational technique used to determine the emotional tone behind a series of words, helping to understand attitudes and opinions expressed in text data.
- How can businesses use sentiment analysis? Businesses can leverage sentiment analysis to enhance customer engagement, improve product development, and monitor brand reputation.
- Why is sentiment analysis important for governments? It helps governments track public opinion on policies and candidates, allowing for more effective communication and policy formulation.
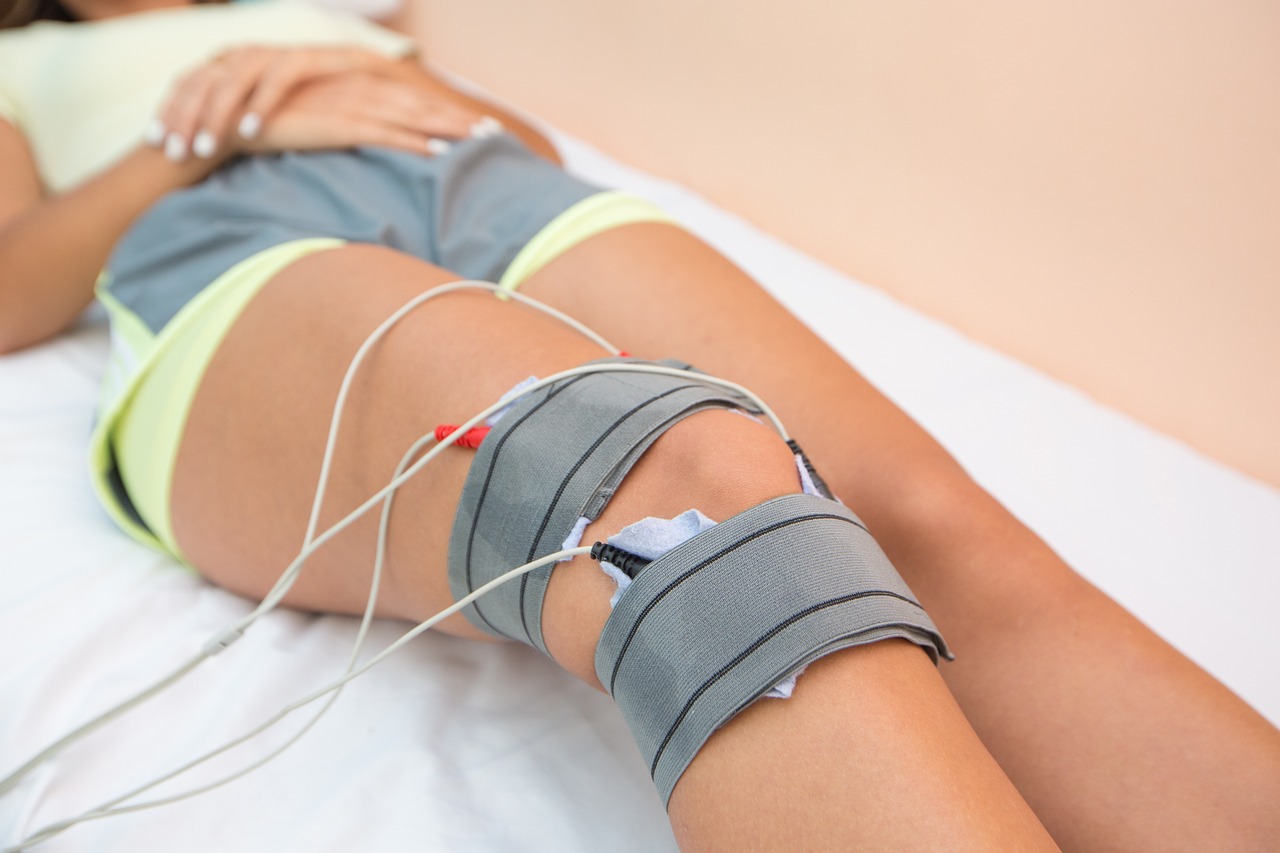
Applications in Marketing
In today's fast-paced digital landscape, understanding consumer sentiment is more crucial than ever. Businesses are leveraging sentiment analysis to gain insights into how their customers feel about their products and services. Imagine launching a new product and having the ability to gauge public reaction almost instantly—this is the power of sentiment analysis. By analyzing social media posts, reviews, and customer feedback, companies can quickly identify trends and adjust their strategies accordingly.
One of the most significant advantages of sentiment analysis in marketing is its ability to provide real-time feedback. For instance, if a company launches a new advertising campaign, sentiment analysis can help determine whether the audience is responding positively or negatively. This immediate insight allows marketers to pivot their strategies without missing a beat. Instead of waiting for traditional metrics like sales figures to roll in, businesses can proactively address concerns or amplify successful messaging.
Moreover, sentiment analysis can enhance customer engagement by personalizing marketing efforts. By understanding the emotions behind customer interactions, businesses can tailor their communications to resonate more deeply with their audience. For example, if sentiment analysis reveals that customers are feeling frustrated with a particular aspect of a service, a company can create targeted campaigns to address those issues, showing that they are listening and care about their customers' experiences.
Here are a few key applications of sentiment analysis in marketing:
- Product Development: Insights from sentiment analysis can guide product improvements or new feature developments based on customer feedback.
- Brand Monitoring: Companies can track how their brand is perceived over time, allowing them to respond to negative sentiment before it escalates.
- Competitor Analysis: By analyzing sentiment around competitors, businesses can identify gaps in the market and opportunities for differentiation.
To illustrate the impact of sentiment analysis in marketing, consider the following table that summarizes a hypothetical case study:
Brand | Campaign | Sentiment Before | Sentiment After | Change in Sales |
---|---|---|---|---|
Brand A | Summer Launch | Negative (30%) | Positive (75%) | +40% |
Brand B | Winter Sale | Neutral (50%) | Positive (80%) | +25% |
This table shows how Brand A was able to shift its sentiment from negative to positive through targeted adjustments in their marketing strategy, resulting in a significant increase in sales. Such examples highlight the transformative power of sentiment analysis, as businesses can not only understand but also influence public opinion.
In conclusion, the applications of sentiment analysis in marketing are vast and varied. From product development to brand monitoring, the insights gained from analyzing consumer sentiment can lead to more effective strategies and stronger customer relationships. As companies continue to embrace this technology, we can expect to see even more innovative uses that will shape the future of marketing.
1. What is sentiment analysis?
Sentiment analysis is a computational technique used to determine the emotional tone behind a series of words, helping to understand attitudes, opinions, and emotions expressed in text data.
2. How can businesses use sentiment analysis?
Businesses can use sentiment analysis to gauge consumer reactions to products, tailor marketing strategies, monitor brand reputation, and enhance customer engagement.
3. What are the challenges of sentiment analysis?
Challenges include sarcasm detection, context understanding, and the need for language-specific models to ensure accuracy in interpretation.
4. Is sentiment analysis only useful for marketing?
No, sentiment analysis is also widely used in politics, social research, and customer service to understand public opinion and improve communication strategies.
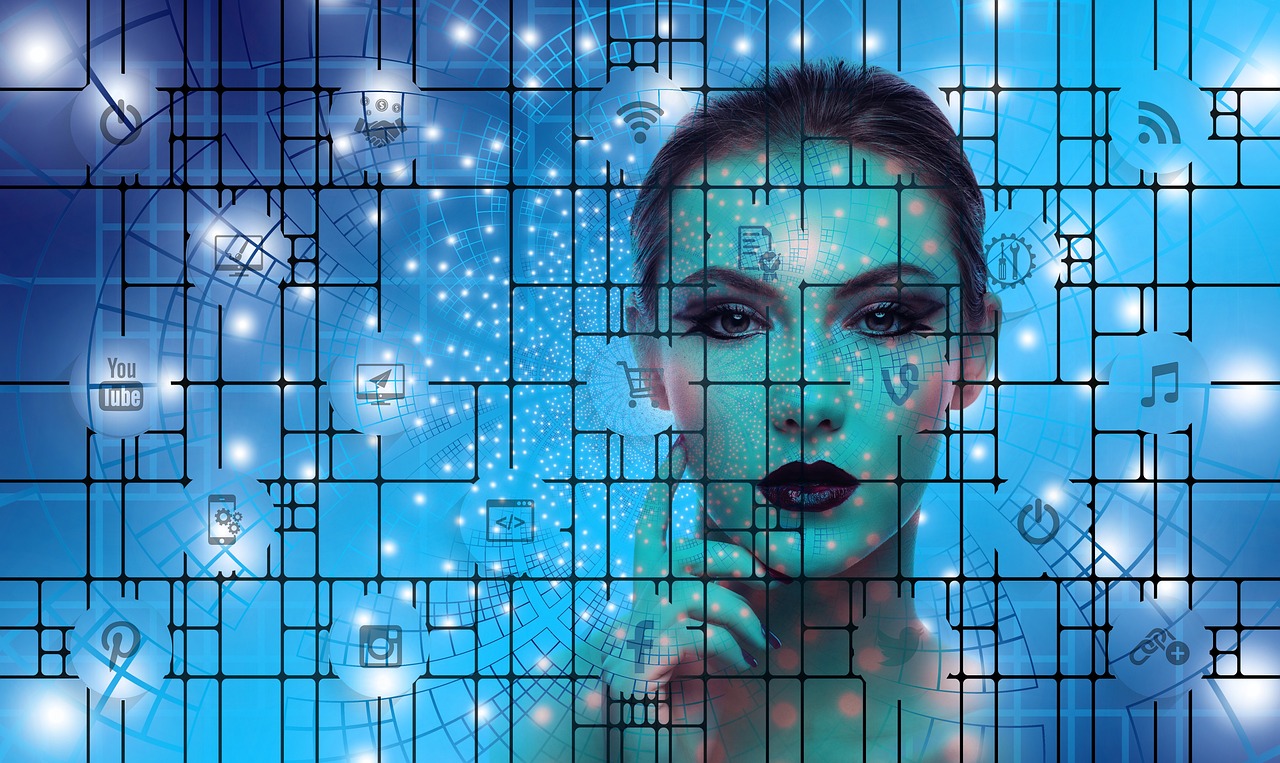
Case Studies in Brand Management
In the fast-paced world of brand management, sentiment analysis has emerged as a powerful tool for companies looking to navigate the complexities of consumer opinions. One notable case is that of Nike, which has successfully harnessed sentiment analysis to respond to public feedback during its marketing campaigns. By monitoring social media platforms and online forums, Nike was able to identify key sentiments surrounding their new product launches. For instance, during the launch of a controversial ad campaign, sentiment analysis revealed a significant amount of negative feedback stemming from a specific demographic. Instead of ignoring this backlash, Nike took immediate action, addressing the concerns directly through targeted communications and adjustments to their marketing strategy. This proactive approach not only mitigated potential damage to their brand reputation but also fostered a sense of loyalty among their consumers.
Another compelling example comes from Coca-Cola, which utilized sentiment analysis to enhance its customer engagement strategies. By analyzing consumer sentiment across various channels, Coca-Cola discovered that their audience had a strong emotional connection to sustainability initiatives. In response, the company launched a campaign focused on environmental responsibility, aligning their messaging with the prevailing sentiments of their consumers. This alignment not only boosted their brand image but also resulted in a significant increase in sales, demonstrating how effectively understanding public sentiment can lead to tangible business outcomes.
Furthermore, a tech giant like Apple has also embraced sentiment analysis to maintain its competitive edge. During the launch of new products, Apple employs sentiment analysis tools to gauge public reaction in real-time. This allows them to make necessary adjustments to their marketing efforts and product features based on consumer feedback. For example, when a new iPhone model received mixed reviews regarding its battery life, Apple quickly addressed the issue by enhancing battery performance in subsequent updates. This responsiveness not only improved customer satisfaction but also reinforced Apple's commitment to quality and innovation.
These case studies illustrate that sentiment analysis is not just a buzzword; it is a strategic asset that can significantly influence brand management. Companies that effectively leverage this tool can foster stronger connections with their audience, adapt to changing sentiments, and ultimately drive their business success. As brands continue to navigate the digital landscape, the ability to listen and respond to consumer emotions will be more critical than ever.
In conclusion, the integration of sentiment analysis in brand management is transforming how companies interact with their consumers. By understanding the emotional undercurrents of public opinion, brands like Nike, Coca-Cola, and Apple are not only enhancing their marketing strategies but also building lasting relationships with their audiences. The future of brand management will undoubtedly be shaped by those who can effectively harness the power of sentiment analysis.
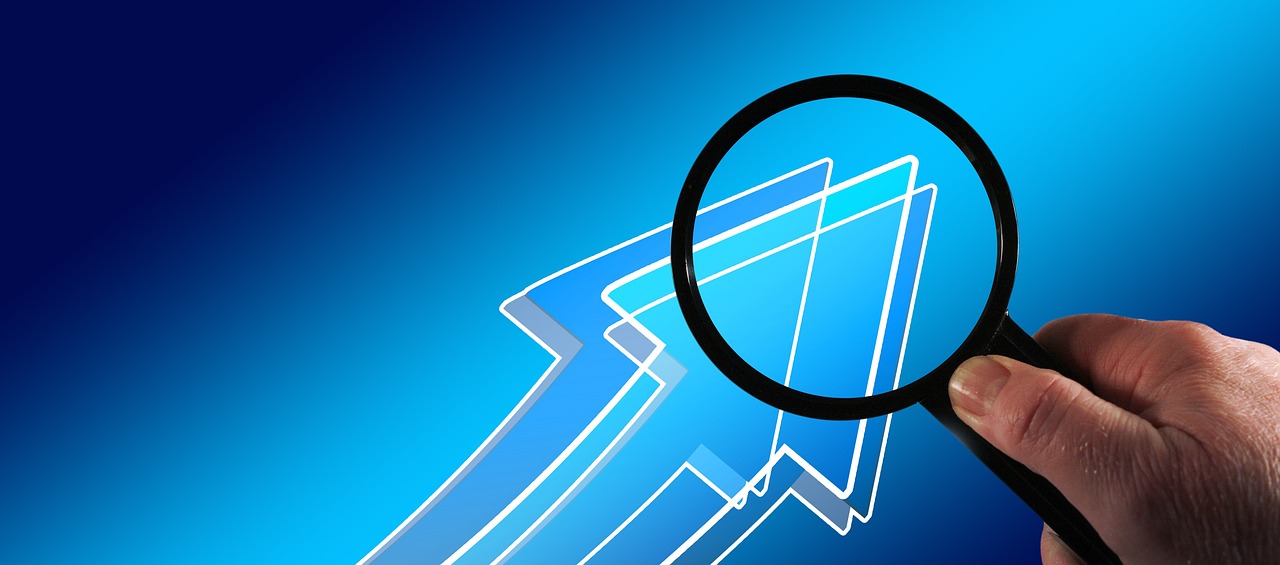
Impact on Advertising Strategies
In the ever-evolving world of advertising, sentiment analysis has emerged as a game-changer, transforming how brands connect with their audiences. Imagine trying to hit a target blindfolded; that’s what advertising was like before sentiment analysis came into play. Now, with the ability to gauge public feelings towards products and campaigns, advertisers can fine-tune their messaging to hit the bullseye every time. By analyzing social media conversations, reviews, and other forms of consumer feedback, brands gain invaluable insights into what resonates with their audience.
One of the most significant impacts of sentiment analysis on advertising strategies is the ability to create tailored content. Advertisers can now craft campaigns that align with the emotional tone of their target demographic. For instance, if sentiment analysis reveals that a large portion of the audience feels nostalgic about a particular theme, brands can incorporate that sentiment into their advertising to create a stronger emotional connection. This approach not only enhances engagement but also fosters a sense of trust and loyalty among consumers.
Moreover, sentiment analysis allows brands to monitor the effectiveness of their campaigns in real-time. Imagine launching a new product and being able to see, almost instantly, how consumers are reacting to it. This immediate feedback loop enables advertisers to pivot their strategies quickly, optimizing their campaigns based on actual consumer sentiment rather than relying solely on historical data or assumptions. As a result, brands can allocate their marketing budgets more efficiently, focusing on strategies that yield the best return on investment.
To illustrate this further, consider the following table showcasing how sentiment analysis can influence different advertising strategies:
Strategy | Sentiment Analysis Impact |
---|---|
Content Creation | Tailors messaging to evoke positive emotions, increasing engagement. |
Ad Placement | Identifies platforms where target audiences express favorable sentiments. |
Campaign Adjustment | Allows for real-time modifications based on audience reactions. |
Brand Positioning | Enhances brand image by aligning with consumer sentiments and trends. |
Additionally, sentiment analysis helps brands to identify potential issues before they escalate. By keeping a pulse on public opinion, advertisers can proactively address negative sentiments, turning potential crises into opportunities for engagement. For example, if a product receives unfavorable feedback, brands can respond swiftly with clarifications or improvements, demonstrating their commitment to customer satisfaction. This level of responsiveness not only mitigates damage but also strengthens brand loyalty.
In conclusion, the impact of sentiment analysis on advertising strategies cannot be overstated. It empowers brands to create more meaningful connections with their audiences, optimize their campaigns in real-time, and respond proactively to consumer feedback. As we move forward, it’s clear that leveraging sentiment analysis will be crucial for any brand looking to stay ahead in the competitive advertising landscape.
- What is sentiment analysis? Sentiment analysis is a computational technique used to determine the emotional tone behind a series of words, helping to understand attitudes, opinions, and emotions expressed in text data.
- How does sentiment analysis benefit advertising? It allows advertisers to tailor their messaging, monitor campaign effectiveness in real-time, and respond proactively to consumer feedback.
- Can sentiment analysis detect sarcasm? While it's a challenge, advancements in technology are improving the ability to detect sarcasm and context in sentiment analysis.
- Is sentiment analysis applicable across different languages? Yes, but it requires language-specific models to ensure accuracy in interpretation.
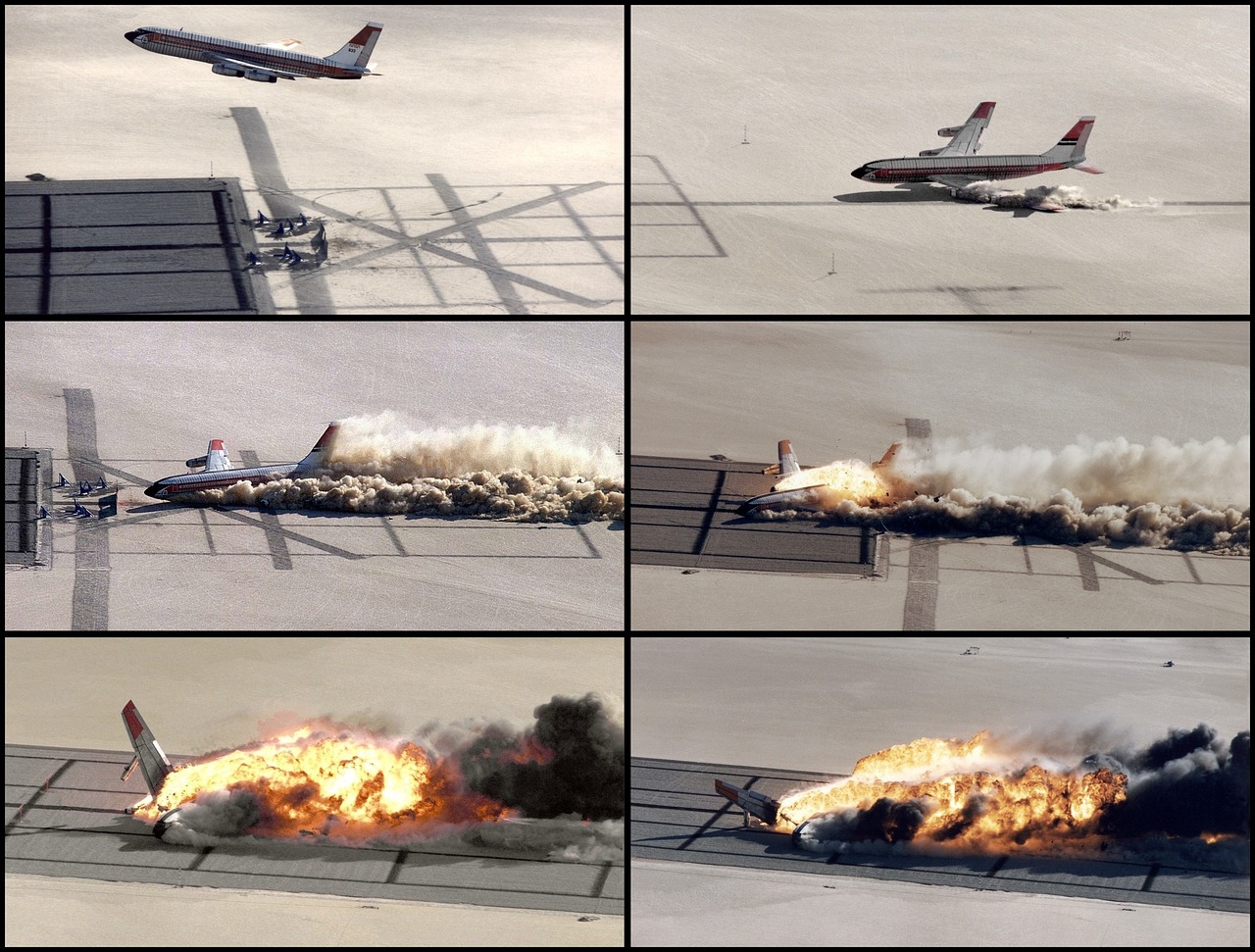
Applications in Politics
In the fast-paced world of politics, understanding public sentiment is akin to having a compass in a dense fog. Politicians and political analysts are increasingly turning to sentiment analysis as a powerful tool to navigate the complex landscape of voter opinions. By analyzing social media posts, news articles, and public forums, they can gain insights into how policies and candidates are perceived by the public. This data-driven approach allows them to tailor their strategies and communication efforts to resonate with voters more effectively.
One of the most fascinating aspects of sentiment analysis in politics is its ability to provide real-time feedback. Imagine a politician delivering a speech and, within minutes, they can gauge how well their message was received through social media reactions. This immediacy enables them to adjust their messaging on the fly, ensuring that they connect with their audience in a meaningful way. For instance, if a candidate's stance on a particular issue is met with negative sentiment, they can pivot their approach, addressing concerns and reframing their message to better align with public opinion.
Additionally, sentiment analysis can be instrumental during election campaigns. By monitoring trends in voter sentiment over time, campaigns can identify key issues that resonate with the electorate. This allows them to focus their efforts where they matter most, ensuring that their platforms reflect the collective concerns of the population. For example, if sentiment analysis reveals a growing concern over healthcare, candidates can prioritize this issue in their campaign materials and public appearances.
Moreover, sentiment analysis can help in identifying not just what the public thinks, but also the emotions behind those thoughts. Are voters feeling hopeful, frustrated, or angry? Understanding these emotional nuances can provide valuable context that goes beyond mere approval ratings. It can help campaigns craft messages that not only inform but also inspire action. For instance, a campaign that taps into feelings of hope and optimism may mobilize supporters more effectively than one that simply lists policy proposals.
However, it’s essential to recognize that sentiment analysis is not without its challenges in the political realm. The presence of sarcasm and irony in political discourse can complicate the accuracy of sentiment detection. A tweet that seems negative on the surface may actually be a humorous critique, and without the right context, sentiment analysis tools might misinterpret it. Therefore, it's crucial for political analysts to continually refine their tools and methodologies to ensure they capture the true sentiment of the public.
In summary, the applications of sentiment analysis in politics are vast and varied. From shaping campaign strategies to understanding voter emotions, this innovative approach is redefining how politicians engage with their constituents. As technology continues to evolve, we can expect sentiment analysis to become even more integral to political decision-making, providing deeper insights and fostering a more responsive political landscape.
- What is sentiment analysis? Sentiment analysis is a computational technique used to determine the emotional tone behind a series of words, helping to understand attitudes, opinions, and emotions expressed in text data.
- How is sentiment analysis used in politics? Politicians use sentiment analysis to track public opinion on policies and candidates, enabling them to adjust strategies and communication to better connect with voters.
- What are the challenges of sentiment analysis? Challenges include sarcasm detection, context understanding, and the need for language-specific models to ensure accuracy in interpretation.
- What is the future of sentiment analysis? The future of sentiment analysis is likely to involve advancements in artificial intelligence and machine learning, enhancing accuracy and broadening its applications across various fields.
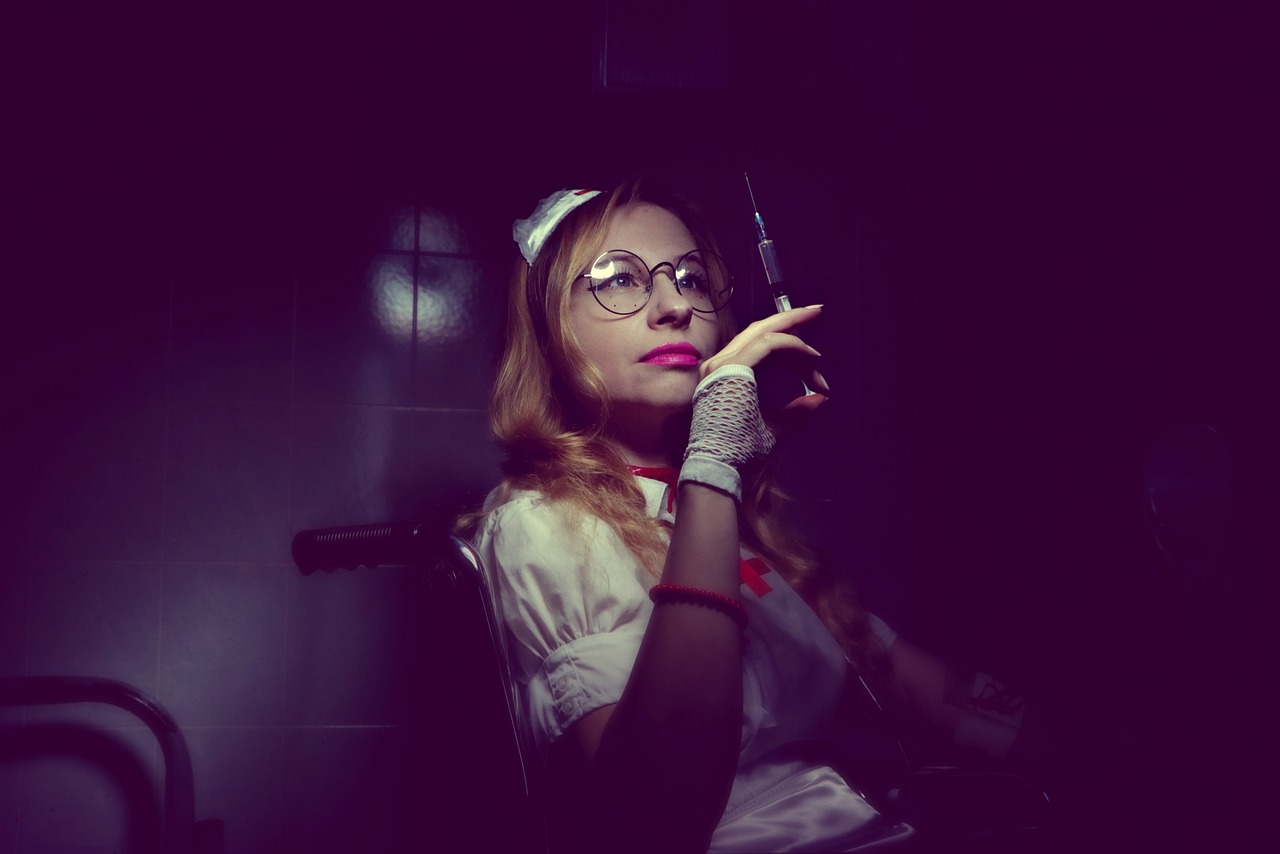
Challenges in Sentiment Analysis
Sentiment analysis, while a powerful tool for understanding public opinion, is not without its challenges. One of the primary hurdles is the detection of sarcasm. Imagine reading a tweet that says, "Oh great, another rainy day!" While the words may seem positive, the underlying sentiment is anything but. This nuance can easily trip up algorithms that are designed to classify text based on surface-level cues. To effectively harness sentiment analysis, it’s crucial to develop systems that can understand the context in which words are used, allowing for a more accurate interpretation of emotions.
Another significant challenge is the need for language-specific models. Different languages have unique structures, idioms, and cultural references that can alter the meaning of phrases. For instance, a phrase that is considered complimentary in one language might be offensive in another. This linguistic diversity means that a one-size-fits-all approach to sentiment analysis is insufficient. Developers must invest time and resources into creating tailored models that can accurately interpret sentiments across various languages and dialects.
Furthermore, data bias poses a substantial risk to the reliability of sentiment analysis. If the training data used to develop sentiment analysis algorithms is skewed towards a particular demographic, the results can reflect those biases, leading to misinterpretations. For example, if a dataset predominantly features opinions from a specific age group or socio-economic status, the algorithm may overlook or misrepresent the sentiments of other groups. To combat this issue, it’s essential to use diverse datasets that capture a wide range of perspectives, ensuring that the analysis is representative and fair.
In addition to these challenges, the rapid evolution of language, especially with the rise of slang and internet jargon, complicates sentiment analysis further. New phrases and expressions can emerge overnight, and keeping sentiment analysis tools updated to recognize these changes is a continuous task. Developers need to implement adaptive learning techniques that allow algorithms to learn and evolve with language trends, ensuring that their analyses remain relevant and accurate.
Lastly, the integration of emotional context into sentiment analysis is crucial. Words can carry different meanings depending on the emotional state of the speaker. For instance, the statement "I'm fine" can express contentment or conceal frustration, depending on the delivery and context. Therefore, sentiment analysis tools must be equipped to evaluate not just the words, but also the emotional undertones that accompany them. This level of sophistication requires advanced machine learning techniques and a deep understanding of human emotions.
In summary, while sentiment analysis offers invaluable insights into public opinion, it faces significant challenges that need to be addressed for optimal performance. By focusing on sarcasm detection, language-specific models, data bias, evolving language trends, and emotional context, developers can enhance the accuracy and applicability of sentiment analysis across various fields.
- What is sentiment analysis? Sentiment analysis is a computational technique used to determine the emotional tone behind a series of words, helping to understand attitudes, opinions, and emotions expressed in text data.
- How is sentiment analysis used in marketing? Businesses use sentiment analysis to gauge consumer reactions to products and campaigns, allowing them to tailor their marketing strategies and improve customer engagement based on real-time feedback.
- What challenges does sentiment analysis face? Key challenges include sarcasm detection, language-specific models, data bias, evolving language trends, and the need for emotional context in interpretation.
- How can sentiment analysis impact political strategies? Politicians use sentiment analysis to track public opinion on policies and candidates, enabling them to adjust strategies and communication to better connect with voters.
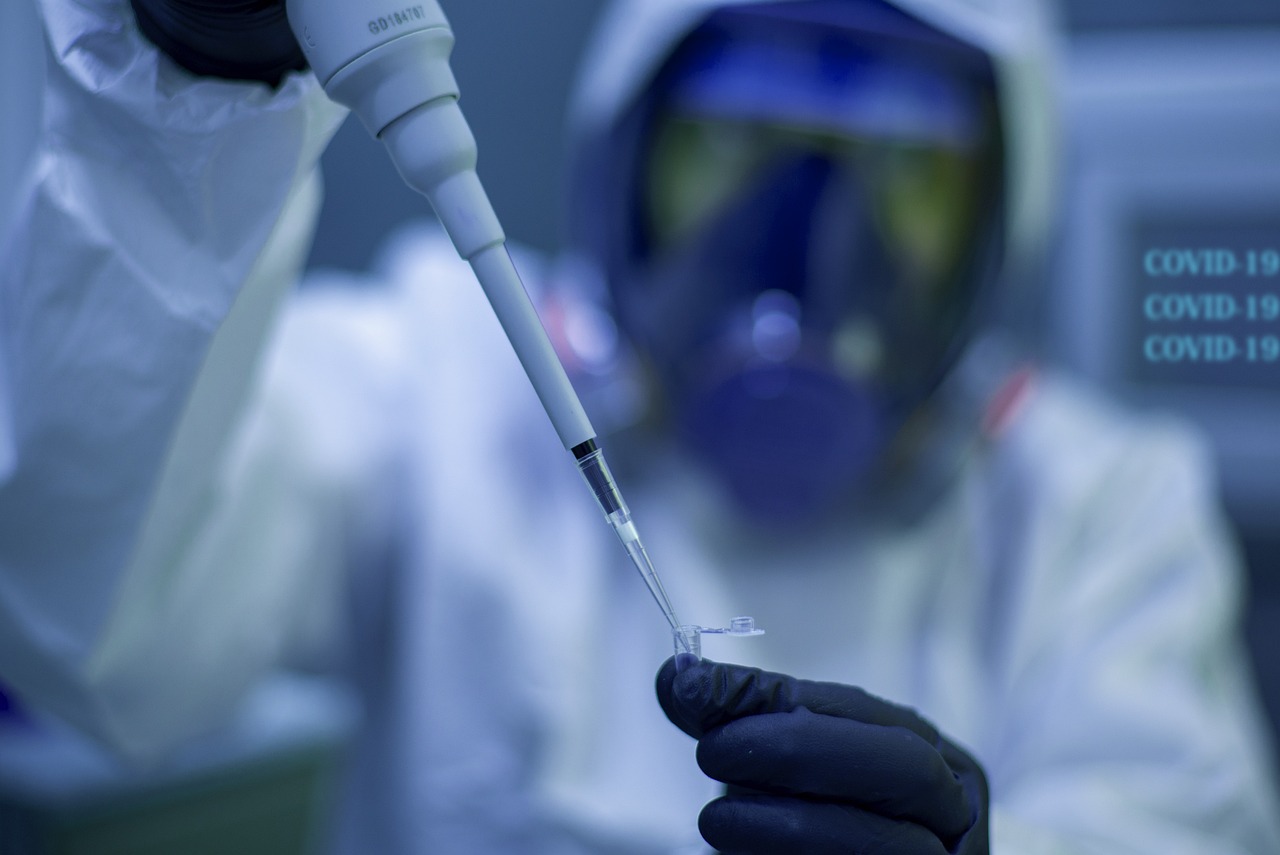
Overcoming Language Barriers
In today’s interconnected world, language barriers pose significant challenges for sentiment analysis. Imagine trying to understand a conversation in a language you don’t speak; it’s like trying to solve a puzzle with missing pieces. For sentiment analysis to be effective globally, it must accurately interpret various languages and dialects. This requires sophisticated algorithms that can navigate the nuances of different linguistic structures and cultural contexts.
One of the primary hurdles is the diversity of languages. Each language has its own set of idioms, slang, and emotional expressions, making it essential for sentiment analysis tools to be tailored to specific languages. For instance, a phrase that conveys excitement in one language might not translate the same way in another. Therefore, developing language-specific models is crucial for ensuring accurate sentiment interpretation.
Moreover, context matters. The same word can carry different meanings based on context, tone, or even cultural background. For example, the word "sick" can be interpreted positively in slang, while it has a negative connotation in a literal sense. To overcome these challenges, sentiment analysis systems need to incorporate context-aware algorithms that can discern meaning based on surrounding words and phrases.
To illustrate this point, consider the following table that highlights some of the common challenges faced in sentiment analysis across different languages:
Language | Challenge | Solution |
---|---|---|
Spanish | Use of gendered nouns and adjectives | Develop models that account for grammatical gender |
Mandarin | Tonal variations affecting meaning | Integrate tonal analysis into sentiment algorithms |
Arabic | Dialectal differences | Create region-specific sentiment models |
English | Slang and idiomatic expressions | Incorporate contemporary slang dictionaries |
By investing in language-specific sentiment analysis tools and continually refining them, developers can ensure that insights are not only accessible but also relevant across diverse populations. This is akin to building a bridge that connects different cultures and perspectives, allowing for a more comprehensive understanding of global sentiments.
Ultimately, overcoming language barriers in sentiment analysis is not just about technology; it’s about fostering a deeper connection among people. As we continue to break down these barriers, the potential for more informed decision-making and enhanced communication becomes limitless.
- What is sentiment analysis? Sentiment analysis is a computational technique used to determine the emotional tone behind a series of words, helping to understand attitudes, opinions, and emotions expressed in text data.
- Why is sentiment analysis important? It is crucial for businesses and governments as it aids in decision-making processes, marketing strategies, and policy formulation based on the collective feelings of a population.
- What are some challenges in sentiment analysis? Challenges include sarcasm detection, context understanding, and the need for language-specific models to ensure accuracy in interpretation.
- How can language barriers be overcome in sentiment analysis? By developing language-specific models and context-aware algorithms, sentiment analysis can accurately interpret various languages and dialects.
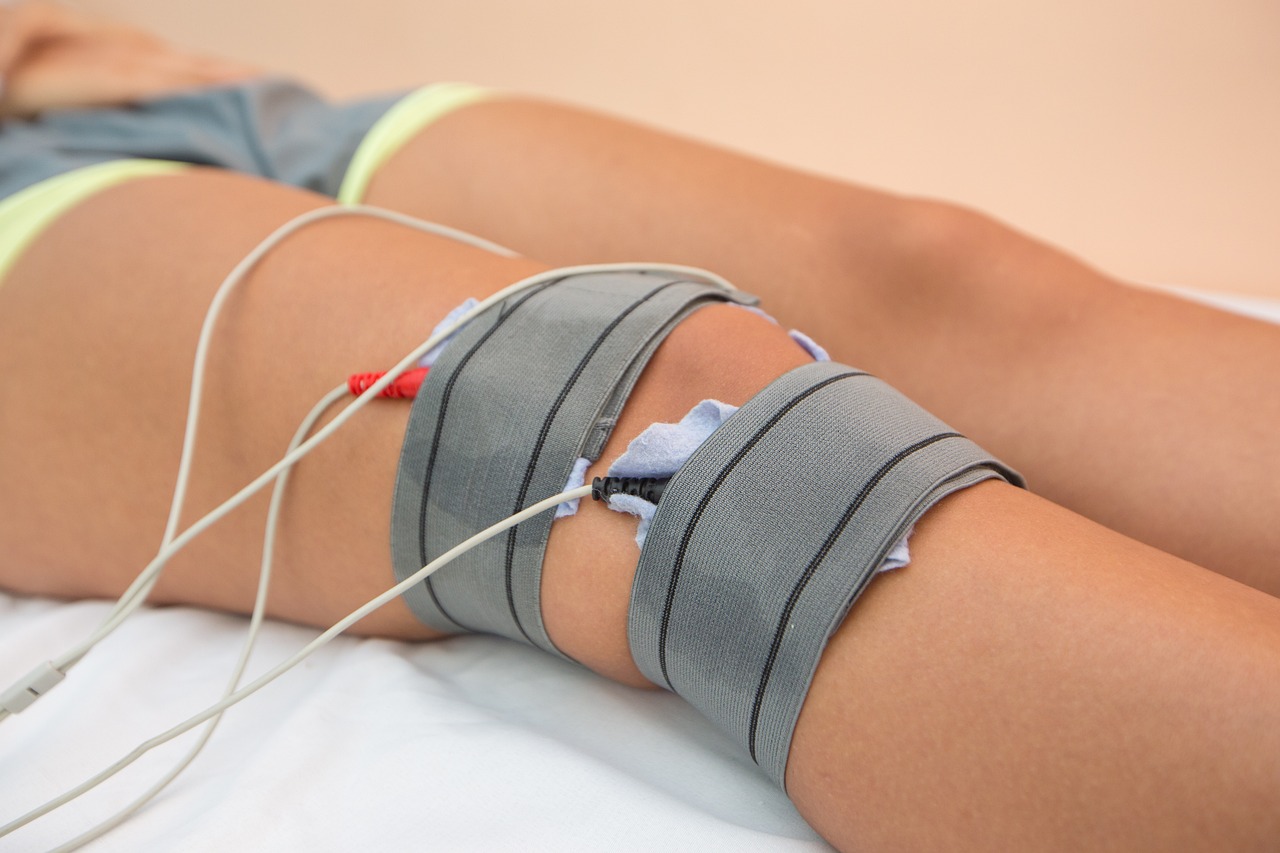
Addressing Data Bias
Data bias is a significant hurdle in the realm of sentiment analysis, and it's one that cannot be overlooked. When we talk about bias, we're referring to the systematic favoritism that can distort our understanding of public sentiment. Imagine trying to get a clear picture of a crowd's mood, but only listening to a select few voices; that’s essentially what happens when we rely on biased data. If the datasets used for training sentiment analysis models do not accurately represent the diversity of the population, the insights derived can be misleading and potentially harmful.
One of the primary reasons bias creeps into sentiment analysis is the lack of representation in the data. For instance, if a model is trained predominantly on English-speaking data from a specific region, it may struggle to accurately interpret sentiments from non-English speakers or those from different cultural backgrounds. This is why it's crucial to utilize a diverse array of datasets that encompass various demographics, languages, and cultural contexts. By doing so, we can ensure that the models we develop are more inclusive and capable of understanding the nuances of different sentiments.
To tackle data bias, organizations can adopt several strategies:
- Utilizing Diverse Data Sources: This involves sourcing data from various platforms, regions, and demographic groups to create a more balanced dataset.
- Regularly Updating Datasets: As language and sentiment evolve over time, it’s essential to continually refresh the datasets used for training models to reflect current trends and vernacular.
- Algorithm Refinement: Constantly refining algorithms to detect and mitigate bias is key. This can include implementing fairness constraints during model training.
Moreover, the role of human oversight cannot be underestimated. By incorporating human judgment in the analysis process, organizations can catch potential biases that automated systems might miss. This hybrid approach not only enhances the accuracy of sentiment analysis but also builds trust in the outcomes. In essence, addressing data bias is not just about improving technical performance; it’s about ensuring that the insights we derive genuinely reflect the sentiments of the entire population.
1. What is data bias in sentiment analysis?
Data bias refers to the skewed representation in the datasets used for training sentiment analysis models, which can lead to inaccurate interpretations of public sentiment.
2. How can organizations reduce data bias?
Organizations can reduce data bias by utilizing diverse data sources, regularly updating their datasets, and refining algorithms to ensure fair representation.
3. Why is human oversight important in sentiment analysis?
Human oversight is crucial because it allows for the identification of biases that automated systems might overlook, enhancing the accuracy and trustworthiness of the analysis.
4. What are the implications of biased sentiment analysis?
Biased sentiment analysis can lead to misguided business strategies, ineffective marketing campaigns, and flawed policy decisions, ultimately affecting the well-being of the population being analyzed.
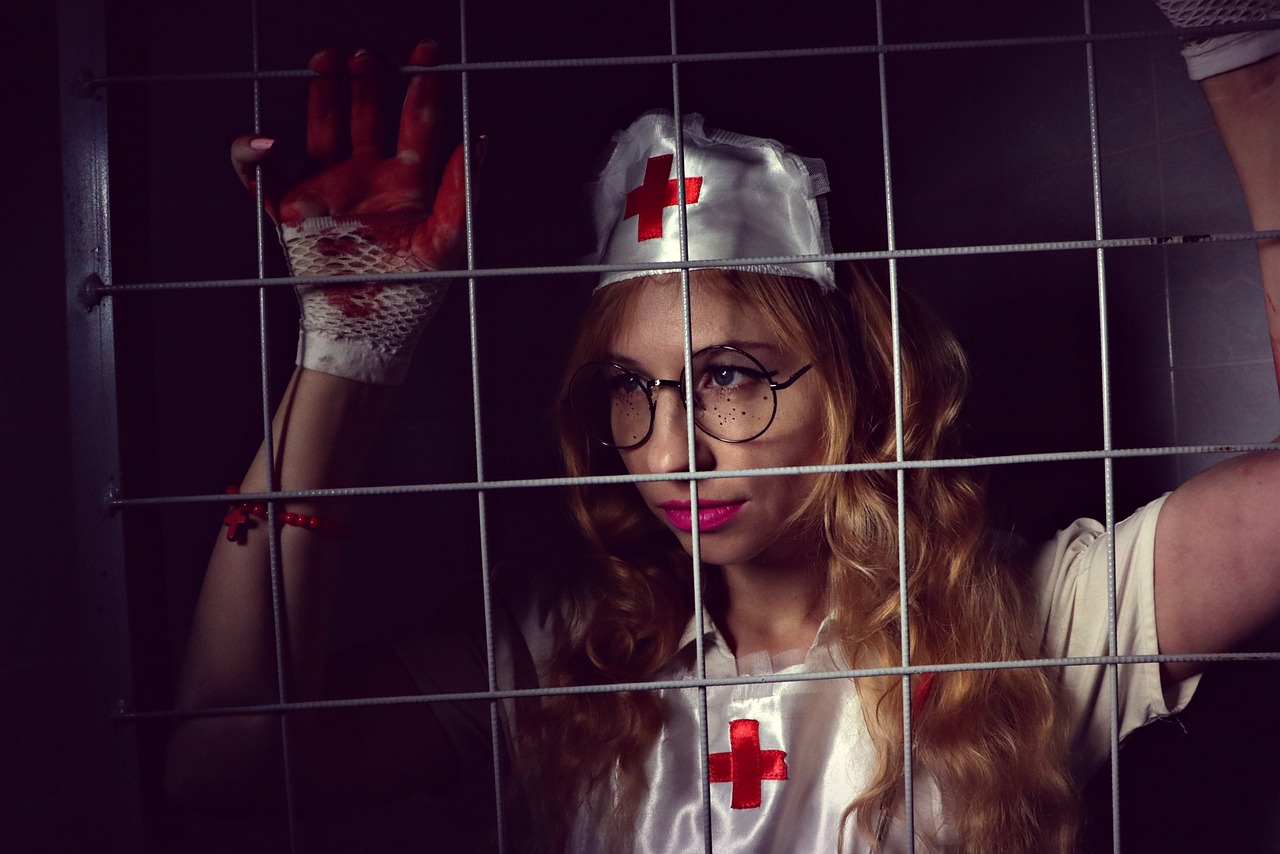
The Future of Sentiment Analysis
As we gaze into the crystal ball of technology, it's clear that the future of sentiment analysis is not just bright, but also filled with exciting possibilities. With the rapid advancements in artificial intelligence and machine learning, we can expect sentiment analysis tools to become increasingly sophisticated. Imagine a world where algorithms can not only detect emotions from text but also understand the nuances of human conversation, including sarcasm and irony. This evolution will allow businesses, governments, and organizations to tap into the collective consciousness of society like never before.
One of the most thrilling prospects is the integration of real-time sentiment analysis into various platforms. Picture this: during a live event, sentiment analysis tools could analyze tweets, comments, and posts instantly, providing a live feed of public opinion. This could revolutionize how brands respond to their audience, allowing for immediate adjustments in marketing strategies or public relations efforts. The potential for instant feedback could lead to a more dynamic relationship between consumers and businesses, fostering a sense of community and engagement.
Moreover, as sentiment analysis becomes more refined, we can expect it to play a crucial role in political landscapes. Political campaigns could leverage these insights to craft messages that resonate deeply with voters, ensuring that their platforms align with the current sentiments of the public. This could lead to a more informed electorate, where decisions are based on genuine understanding rather than mere rhetoric. However, with great power comes great responsibility; the ethical implications of using sentiment analysis in politics will need careful consideration.
In addition to these advancements, the globalization of sentiment analysis tools is another area ripe for growth. As businesses expand their reach across borders, the demand for sentiment analysis that can accurately interpret various languages and cultural contexts will soar. This means developing sophisticated algorithms that can understand the subtleties of different dialects and social nuances. By overcoming language barriers, sentiment analysis can provide valuable insights into diverse populations, ensuring that no voice goes unheard.
To illustrate the potential advancements in sentiment analysis, consider the following table that outlines key areas of expected growth:
Area of Growth | Description |
---|---|
AI Integration | Utilizing advanced AI to improve emotional detection and contextual understanding. |
Real-Time Analysis | Providing instant feedback during live events and campaigns for immediate response. |
Global Adaptation | Creating tools that understand multiple languages and cultural contexts. |
Ethical Frameworks | Establishing guidelines to ensure responsible use of sentiment analysis in politics and marketing. |
As we move forward, it’s essential to address data bias, which can skew results and lead to misinterpretations. The future of sentiment analysis hinges on the development of diverse datasets and algorithms that represent all demographic groups fairly. This will not only enhance the accuracy of sentiment analysis but also ensure that it serves as a tool for inclusivity rather than exclusion.
In conclusion, the future of sentiment analysis is poised to transform the way we understand and engage with public opinion. With innovations on the horizon, businesses and governments alike will have unprecedented access to insights that can drive meaningful change. As we embrace these advancements, the challenge will be to harness the power of sentiment analysis responsibly, ensuring that it enriches our understanding of human emotions and fosters a more connected society.
- What is sentiment analysis? Sentiment analysis is a computational technique used to determine the emotional tone behind words, helping to understand attitudes and opinions expressed in text data.
- How can businesses benefit from sentiment analysis? Businesses can use sentiment analysis to gauge consumer reactions, tailor marketing strategies, and improve customer engagement based on real-time feedback.
- What challenges does sentiment analysis face? Challenges include sarcasm detection, context understanding, and the need for language-specific models to ensure accuracy.
- What is the future of sentiment analysis? The future includes advancements in AI, real-time analysis, global adaptation, and addressing data bias to improve accuracy and inclusivity.
Frequently Asked Questions
- What is sentiment analysis and why is it important?
Sentiment analysis is a computational technique used to identify and extract subjective information from text. It's crucial because it helps businesses and governments understand public opinion, which can guide decision-making, marketing strategies, and policy formulation.
- How do businesses use sentiment analysis?
Businesses utilize sentiment analysis to gauge consumer reactions to their products and campaigns. By analyzing feedback, they can tailor their marketing strategies, enhance customer engagement, and even manage their brand reputation effectively.
- Can sentiment analysis be applied in politics?
Absolutely! Politicians and analysts use sentiment analysis to monitor public opinion on various policies and candidates. This insight allows them to adjust their communication strategies to better resonate with voters and enhance their connection with the electorate.
- What are some challenges faced in sentiment analysis?
Sentiment analysis faces several challenges, including detecting sarcasm, understanding context, and the need for language-specific models. These challenges can impact the accuracy of the analysis and the insights derived from it.
- How can language barriers be overcome in sentiment analysis?
To address language barriers, developers need to create sentiment analysis tools that accurately interpret multiple languages and dialects. This ensures that insights are relevant and accessible across diverse populations, enhancing the global applicability of the analysis.
- What is data bias and how does it affect sentiment analysis?
Data bias occurs when the datasets used for sentiment analysis do not represent the entire population fairly. This can skew results and lead to inaccurate interpretations. It's essential to use diverse datasets and refine algorithms to mitigate this issue.
- What does the future hold for sentiment analysis?
The future of sentiment analysis looks promising, with advancements in technology. Incorporating artificial intelligence and machine learning is expected to enhance the accuracy of sentiment analysis, broadening its applications in various fields.