How to Integrate Quantitative and Qualitative Data
In today's data-driven world, the ability to effectively combine quantitative and qualitative data is a game-changer for researchers, business analysts, and decision-makers alike. But what does it really mean to integrate these two types of data? Imagine trying to put together a jigsaw puzzle where one piece is a numerical statistic and another piece is a vivid personal story. Alone, each piece tells only part of the story, but together, they create a complete picture. This article dives deep into the methods of integration, showcasing how the fusion of numbers and narratives can lead to richer insights and more informed decisions.
To start, let’s break down the essence of both data types. Quantitative data is all about numbers, metrics, and measurable facts. It’s the backbone of statistical analysis, providing a solid foundation for understanding trends and patterns. On the flip side, qualitative data dives into the human experience—capturing emotions, opinions, and the intricate details that numbers alone can’t convey. When we combine these two, we don’t just see the surface; we uncover the underlying reasons and motivations that drive behaviors and outcomes.
Why should we even care about integrating these data types? Well, the answer is simple: the world is complex, and so are the questions we seek to answer. By merging quantitative and qualitative data, we can enhance our research quality and depth. This integration allows us to validate findings, fill in gaps, and ultimately develop a more comprehensive understanding of the topics we’re exploring. Think of it as using both a microscope and a telescope; each tool provides a different perspective, but together, they reveal a much broader view.
Moreover, the advantages of using mixed methods are numerous. For instance, integrating these data types can improve the validity of research findings. When qualitative insights support quantitative results, it creates a more robust argument. Additionally, this approach provides richer insights, allowing researchers to uncover nuances that might otherwise go unnoticed. It’s like adding color to a black-and-white photo—suddenly, the image pops with life and detail.
However, let’s not sugarcoat it—integrating quantitative and qualitative data comes with its own set of challenges. Methodological differences can create obstacles, and ensuring data compatibility isn’t always straightforward. For example, while quantitative data often follows strict protocols and statistical methods, qualitative data can be more subjective and open to interpretation. Navigating these differences requires careful planning and a clear understanding of both data types.
As we explore techniques for integration, several methods stand out. Triangulation is a popular approach, where researchers use multiple data sources to validate findings. Data transformation is another technique, allowing researchers to convert qualitative insights into quantifiable metrics. Additionally, leveraging software tools can streamline the integration process, making it easier to analyze and visualize combined data sets.
In conclusion, the integration of quantitative and qualitative data is not just a research trend; it's a necessity in our increasingly complex world. By embracing both data types, we can unlock deeper insights and make more informed decisions. As we move forward in this article, we will delve into real-world case studies and applications across various fields, illustrating the power of this integration in action.
- What is the difference between quantitative and qualitative data?
Quantitative data is numerical and can be measured statistically, while qualitative data is descriptive and captures experiences and opinions. - Why is data integration important?
Integrating data types enhances research quality, provides a comprehensive understanding, and improves the validity of findings. - What are some techniques for integrating data?
Techniques include triangulation, data transformation, and the use of software tools for analysis.
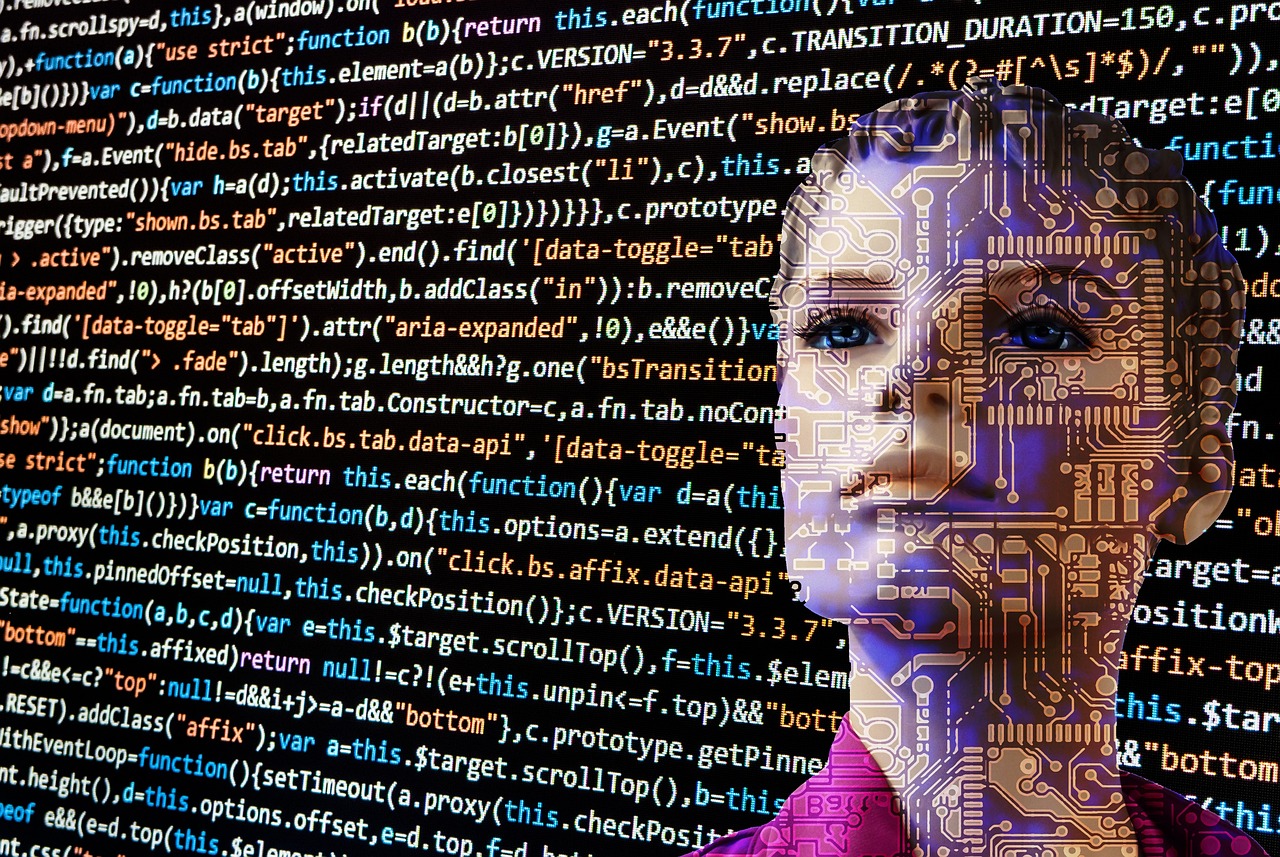
Understanding Quantitative Data
Quantitative data is like the backbone of statistical analysis; it consists of numerical information that can be measured and analyzed in a systematic way. Imagine trying to understand a crowd at a concert: while you might enjoy the music, quantitative data allows you to measure the number of attendees, their age ranges, and even how much they spent on merchandise. This kind of data is characterized by its ability to provide clear, objective insights that can be easily communicated and compared.
There are several types of quantitative data, primarily categorized into discrete and continuous data. Discrete data refers to counts that can be expressed in whole numbers, like the number of people in a room or the number of cars in a parking lot. Continuous data, on the other hand, can take any value within a given range, such as height, weight, or temperature. This distinction is crucial when selecting the appropriate statistical methods for analysis.
Collecting quantitative data can be accomplished through various methods, including surveys, experiments, and observational studies. Surveys often utilize structured questionnaires with closed-ended questions, allowing for easy quantification of responses. For instance, a survey might ask participants to rate their satisfaction on a scale from 1 to 10. Experiments, meanwhile, involve manipulating variables to observe outcomes, yielding numerical results that can be statistically analyzed. Observational studies collect data by observing subjects in their natural environment, which can then be quantified for further analysis.
To illustrate the different types of quantitative data and their collection methods, consider the following table:
Type of Data | Description | Common Collection Methods |
---|---|---|
Discrete | Countable items or categories | Surveys, Polls |
Continuous | Measurable quantities | Experiments, Observational Studies |
Understanding quantitative data is essential for integrating it with qualitative data. By grasping the numerical aspects of research, you can better appreciate how these two data types can complement each other. For instance, while quantitative data might tell you that 70% of customers prefer Product A over Product B, qualitative data can provide the context and reasons behind that preference, enriching your overall understanding.
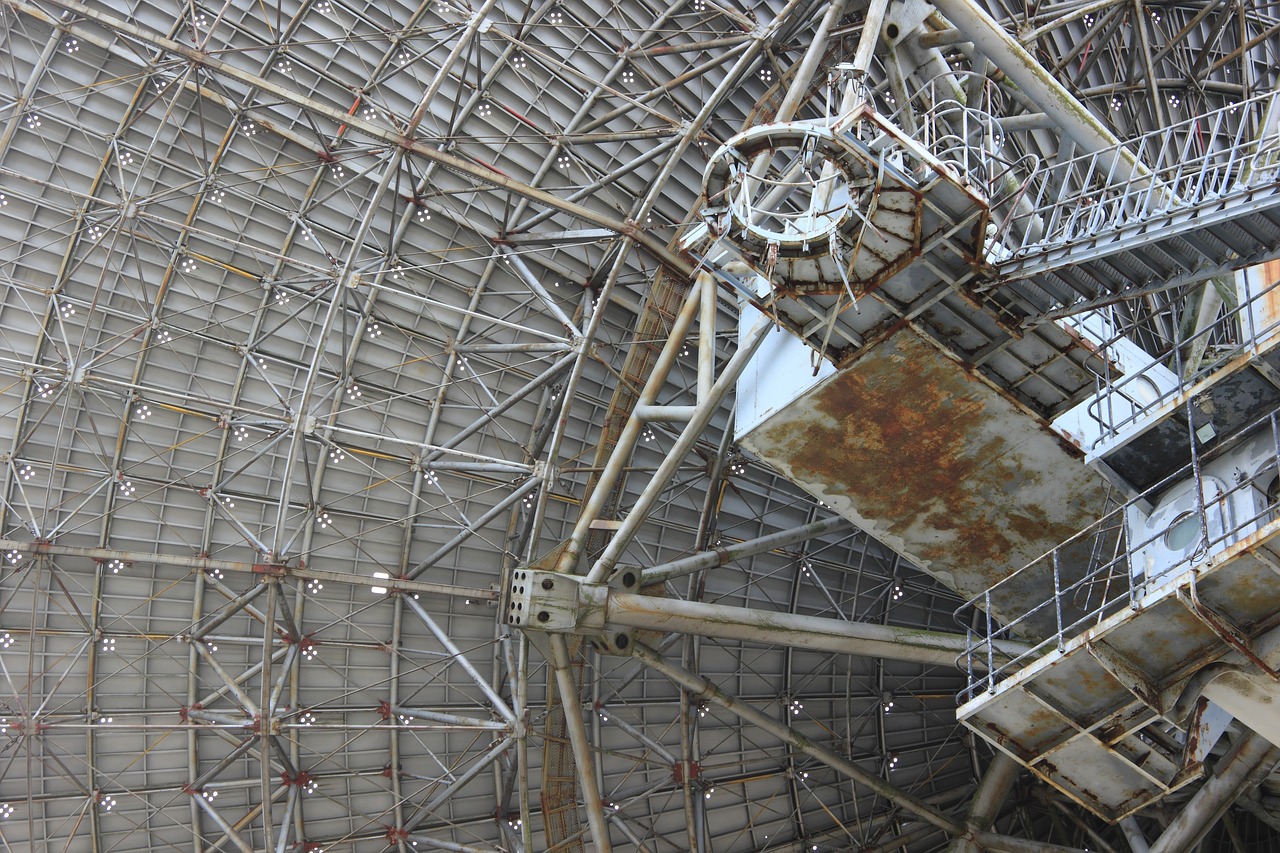
Understanding Qualitative Data
Qualitative data is all about the stories, the nuances, and the rich details that numbers alone can't capture. Unlike quantitative data, which is focused on measurable quantities, qualitative data dives into the depths of human experience, shedding light on emotions, opinions, and perceptions. Imagine trying to understand a beautiful painting just by counting the colors used; you would miss the essence of the artwork. Similarly, qualitative data provides context and meaning to the numerical data, enriching our understanding of complex phenomena.
Characteristics of qualitative data include its subjectivity and contextual richness. This type of data often comes from interviews, focus groups, and open-ended surveys, where respondents can express themselves freely. It can take various forms such as text, audio recordings, images, or even videos. The beauty of qualitative data lies in its ability to capture the nuances of human experience, which often cannot be quantified. For instance, a survey might reveal that 70% of people prefer a certain product, but qualitative interviews can uncover why they feel that way, providing insights into their motivations and desires.
Some common methods of collecting qualitative data include:
- Interviews: One-on-one conversations that allow for deep exploration of a participant's thoughts and feelings.
- Focus Groups: Guided discussions with a group of people to gather diverse perspectives on a topic.
- Observations: Watching how people behave in natural settings to gather insights into their actions and interactions.
- Content Analysis: Analyzing existing texts or media to identify patterns, themes, or meanings.
Qualitative data is invaluable in fields such as social sciences, marketing, and healthcare, where understanding human behavior and motivations is crucial. For example, in healthcare, patient interviews can reveal how they experience a particular illness, which can lead to improved treatment approaches. In marketing, understanding consumer sentiment through qualitative research can help brands tailor their messaging and product offerings, creating a deeper connection with their audience.
In summary, qualitative data is a powerful tool that complements quantitative data by providing context, depth, and a human touch. By integrating both types of data, researchers can achieve a more holistic understanding of their subjects, leading to richer insights and more informed decisions.
- What is the main difference between qualitative and quantitative data?
Qualitative data is descriptive and focuses on understanding human experiences, while quantitative data is numerical and focuses on measuring and analyzing statistical information. - How can qualitative data improve research outcomes?
By providing context and depth, qualitative data enriches the findings of quantitative research, leading to a more comprehensive understanding of the research topic. - What are some common methods for collecting qualitative data?
Common methods include interviews, focus groups, observations, and content analysis.
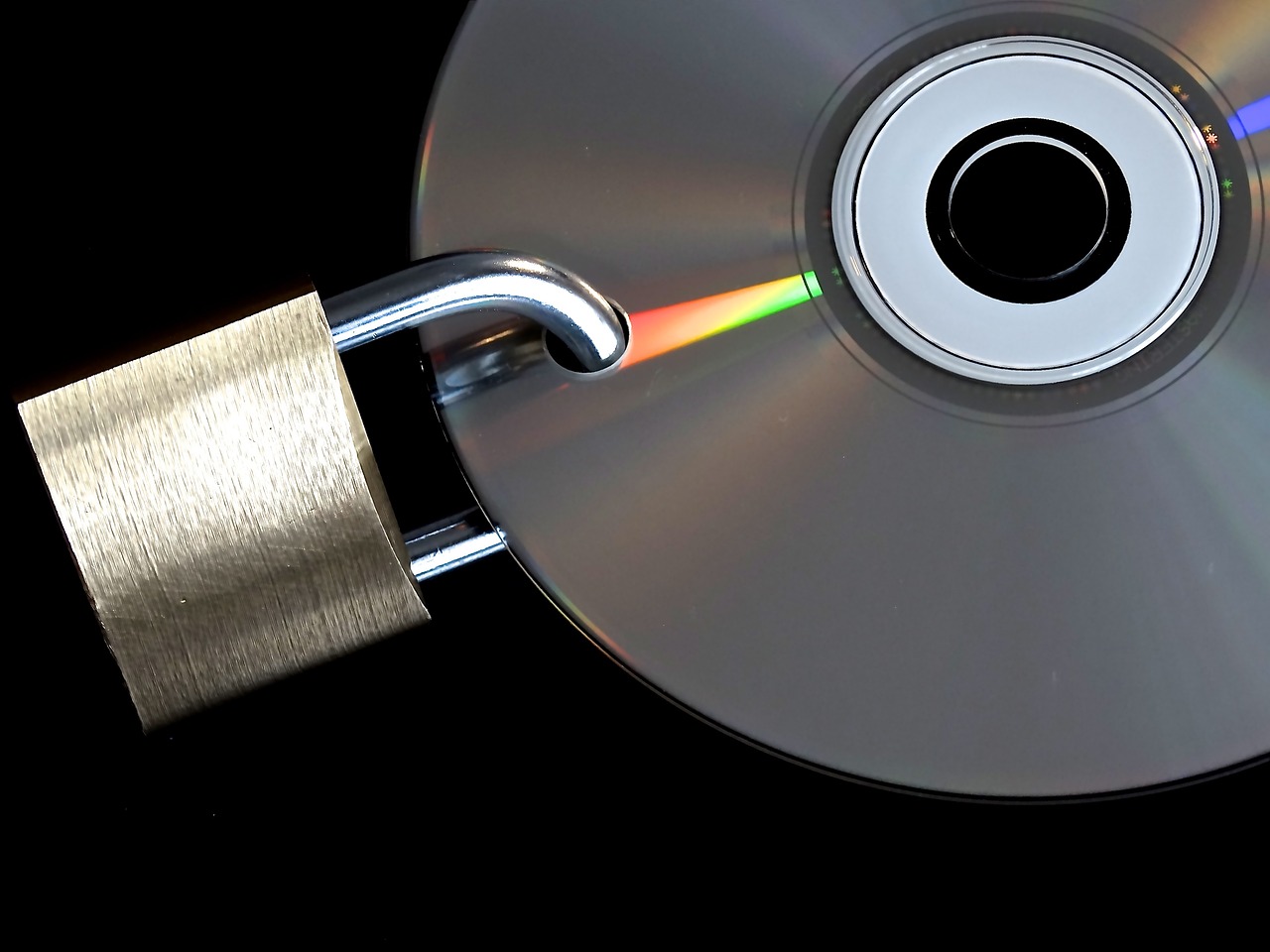
The Importance of Data Integration
When it comes to research, the phrase "the whole is greater than the sum of its parts" couldn't be more applicable. Integrating quantitative and qualitative data is like creating a beautiful tapestry; each thread contributes to a richer, more vibrant picture. By combining these two distinct forms of data, researchers can uncover insights that neither could reveal alone. Think of it as having a two-dimensional view versus a three-dimensional perspective—data integration allows for a fuller understanding of complex issues.
Why is data integration so crucial? For starters, it enhances the quality and depth of research findings. Quantitative data provides the hard facts—numbers, percentages, and statistics—that can paint a clear picture of trends and patterns. However, without the context provided by qualitative data, those numbers can often feel cold and lifeless. Qualitative data, on the other hand, offers rich narratives and personal experiences that breathe life into the statistics. When combined, they create a compelling story that resonates with audiences and decision-makers alike.
Furthermore, integrating these data types can lead to more informed decision-making. Organizations that leverage both quantitative and qualitative insights can better understand their target audiences, identify gaps in their services, and tailor their strategies accordingly. For example, a business might analyze sales data to see which products are performing well, but without qualitative feedback from customers, they might miss out on why those products are successful or what improvements can be made.
Moreover, the integration of data types can also enhance the validity of research findings. When quantitative results are supported by qualitative evidence, it adds an additional layer of credibility. This is particularly important in fields like social sciences, where human behavior is often complex and multifaceted. By employing mixed methods, researchers can cross-validate their findings, ensuring that conclusions drawn are not only statistically significant but also reflective of real-world experiences.
To illustrate the importance of data integration, consider a hypothetical study on public health. A researcher might collect quantitative data on the incidence of a disease in a community, but without qualitative interviews with affected individuals, they may overlook critical factors such as social stigma or access to care. By integrating these data types, the researcher can develop a more comprehensive understanding of the public health issue, leading to more effective interventions.
In conclusion, the integration of quantitative and qualitative data is not just a methodological choice; it's a necessity for anyone looking to delve deeper into their research. The richness that comes from blending numbers with narratives opens doors to insights that can change the way we understand and approach complex issues. As we continue to navigate an increasingly data-driven world, embracing this integration will be key to unlocking the full potential of our findings.
- What is data integration? Data integration refers to the process of combining quantitative and qualitative data to provide a more comprehensive understanding of a research topic.
- Why should I integrate data types? Integrating data types enhances the depth and quality of research findings, leading to more informed decision-making.
- What are some common challenges of data integration? Common challenges include methodological differences, data compatibility issues, and the complexity of analyzing combined data.
- Can you give an example of data integration? A public health study that combines disease incidence statistics (quantitative) with interviews from affected individuals (qualitative) is a great example of data integration.
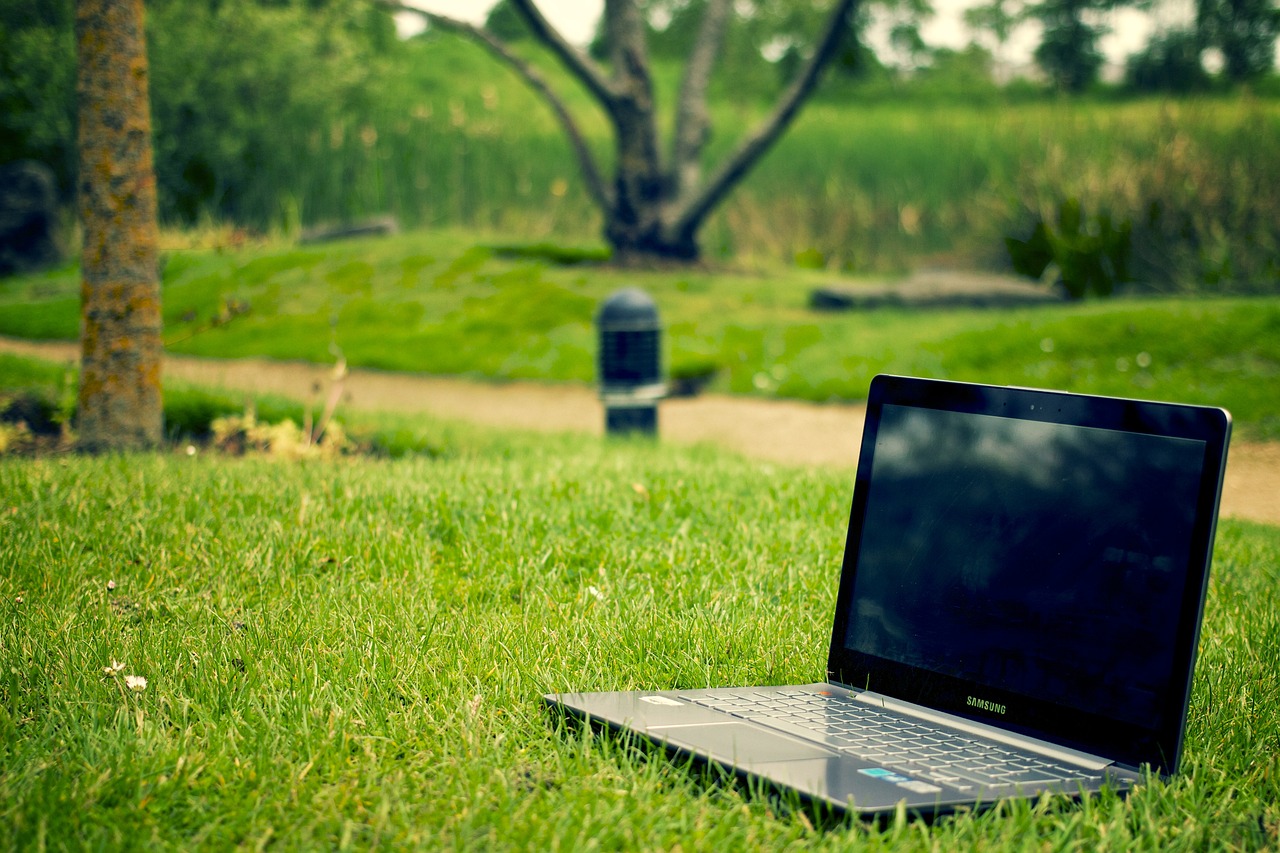
Benefits of Mixed Methods
When it comes to research, the age-old debate of quantitative vs. qualitative data often leaves many scratching their heads. But what if I told you that the magic happens when you combine the two? That’s right! Mixed methods research brings together the best of both worlds, allowing for a deeper and more nuanced understanding of complex issues. Imagine trying to solve a puzzle: you have the edge pieces (quantitative data) that give you the structure, and then you have the colorful middle pieces (qualitative data) that fill in the details. Together, they create a complete picture.
One of the most significant advantages of mixed methods is the increased validity of research findings. When quantitative data is supported by qualitative insights, it adds a layer of credibility to the results. For instance, a survey might show that 70% of consumers prefer a particular product, but qualitative interviews can reveal why they feel that way. This combination not only strengthens the findings but also provides context that numbers alone cannot convey.
Moreover, mixed methods allow researchers to explore complex phenomena from multiple angles. By leveraging both data types, researchers can uncover patterns that might remain hidden when using a single approach. For example, in education research, quantitative data might show test scores, while qualitative data can shed light on student engagement and motivation. Together, these insights can inform better teaching strategies and policies.
Another benefit is the ability to address research questions more comprehensively. Sometimes, a single approach may not be sufficient to tackle a research question fully. By employing mixed methods, researchers can triangulate their findings, leading to a more robust understanding of the subject matter. This is particularly useful in fields like social sciences and health research, where human behavior and experiences are inherently complex.
Additionally, mixed methods foster collaboration among researchers from different disciplines. When quantitative and qualitative researchers come together, they can share their expertise and perspectives, leading to innovative solutions and richer analyses. This interdisciplinary approach not only enhances the quality of research but also encourages a more holistic view of the issues at hand.
In conclusion, embracing mixed methods in research is like adding spices to a dish; it enhances flavor and depth. By integrating quantitative and qualitative data, researchers can achieve a more comprehensive understanding of their subjects, leading to more informed decisions and impactful outcomes. So, if you’re embarking on a research journey, consider mixing it up!
- What are mixed methods in research? Mixed methods research involves using both quantitative and qualitative data to provide a more comprehensive understanding of a research question.
- Why should I use mixed methods? Using mixed methods can enhance the validity of your findings, uncover complex patterns, and provide a richer context for your research.
- Can mixed methods be applied in any field? Yes! Mixed methods can be applied across various fields, including social sciences, business research, healthcare, and education.
- What are some challenges of using mixed methods? Challenges include methodological differences, data compatibility issues, and the need for expertise in both data types.
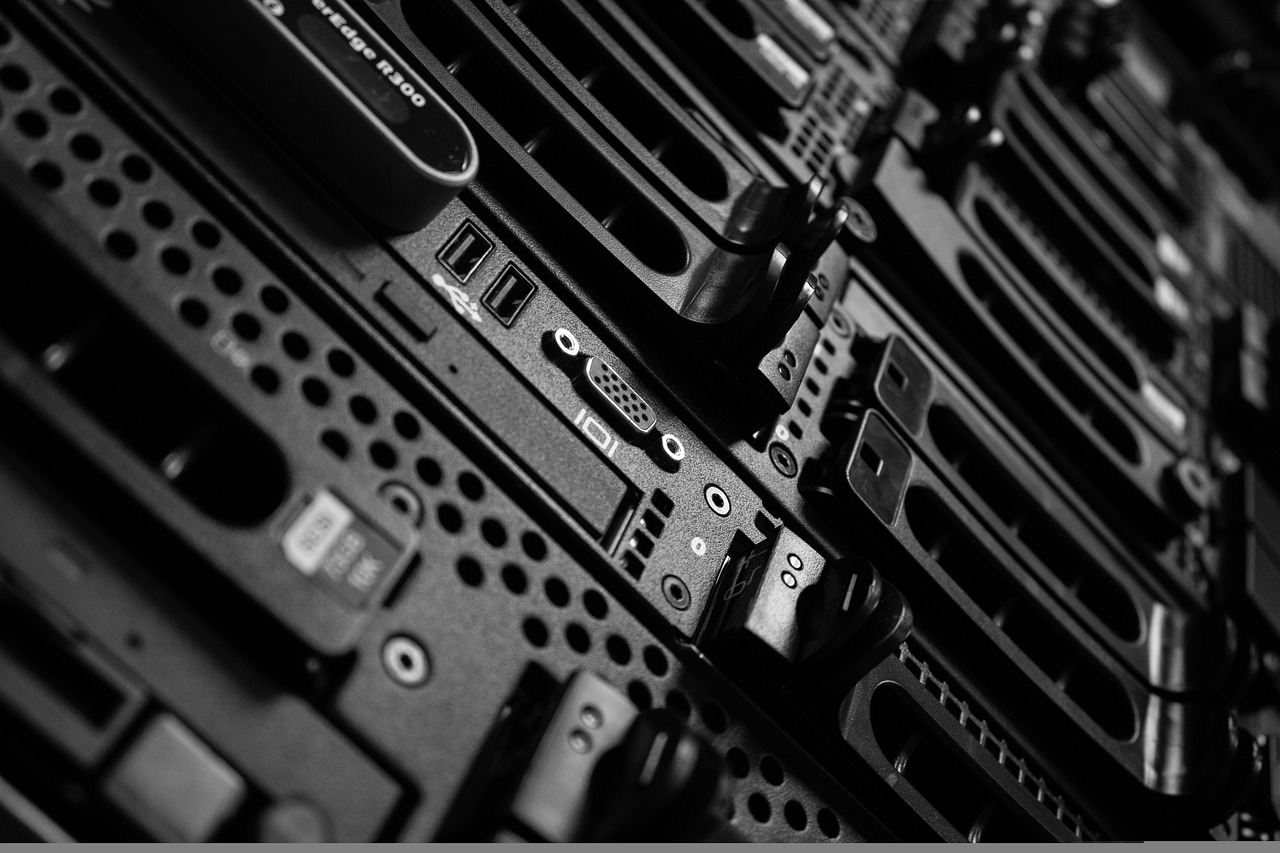
Challenges in Integration
Integrating quantitative and qualitative data is not just a walk in the park; it comes with its own set of challenges that can make even the most seasoned researchers scratch their heads in confusion. One of the primary hurdles is the methodological differences between the two data types. Quantitative data is all about numbers, statistics, and measurable outcomes, while qualitative data dives deep into human experiences, emotions, and opinions. This fundamental difference can create a disconnect when trying to merge the two, leading to potential misinterpretations.
Moreover, data compatibility issues often arise. Have you ever tried fitting a square peg into a round hole? That’s what it can feel like when attempting to reconcile differing data formats. For instance, quantitative data might be presented in numerical tables, while qualitative data could be in the form of interview transcripts or open-ended survey responses. This disparity can complicate the integration process, making it difficult to draw coherent conclusions.
Another challenge lies in the subjectivity of qualitative data. Unlike quantitative data, which is typically objective and can be easily replicated, qualitative data is often influenced by the researcher’s perspective. This subjectivity can introduce bias, making it harder to align findings from both data types. Researchers must be vigilant, employing strategies to minimize bias and ensure that their interpretations are as accurate as possible.
Additionally, the time and resource demands of integrating these data types can be significant. Collecting, analyzing, and interpreting both quantitative and qualitative data requires a substantial investment of time and effort. Researchers may find themselves overwhelmed, especially when juggling multiple projects or tight deadlines. This can lead to rushed analyses, which may compromise the quality of the research.
To navigate these challenges successfully, researchers can employ various strategies, such as training in mixed methods and utilizing software tools designed for data integration. These tools can streamline the process, making it easier to handle the complexities of combining different data types. By acknowledging and addressing these challenges head-on, researchers can unlock the full potential of data integration, leading to richer insights and a more comprehensive understanding of their research topics.
- What is data integration? Data integration is the process of combining different types of data, such as quantitative and qualitative, to provide a more comprehensive understanding of a research topic.
- Why is integrating quantitative and qualitative data important? It enhances the depth and quality of research, allowing for a more nuanced perspective on complex issues.
- What are some common challenges in data integration? Methodological differences, data compatibility issues, subjectivity, and resource demands are some of the key challenges faced.
- How can researchers overcome these challenges? By employing mixed methods training, utilizing data integration software, and being mindful of biases, researchers can navigate these hurdles effectively.
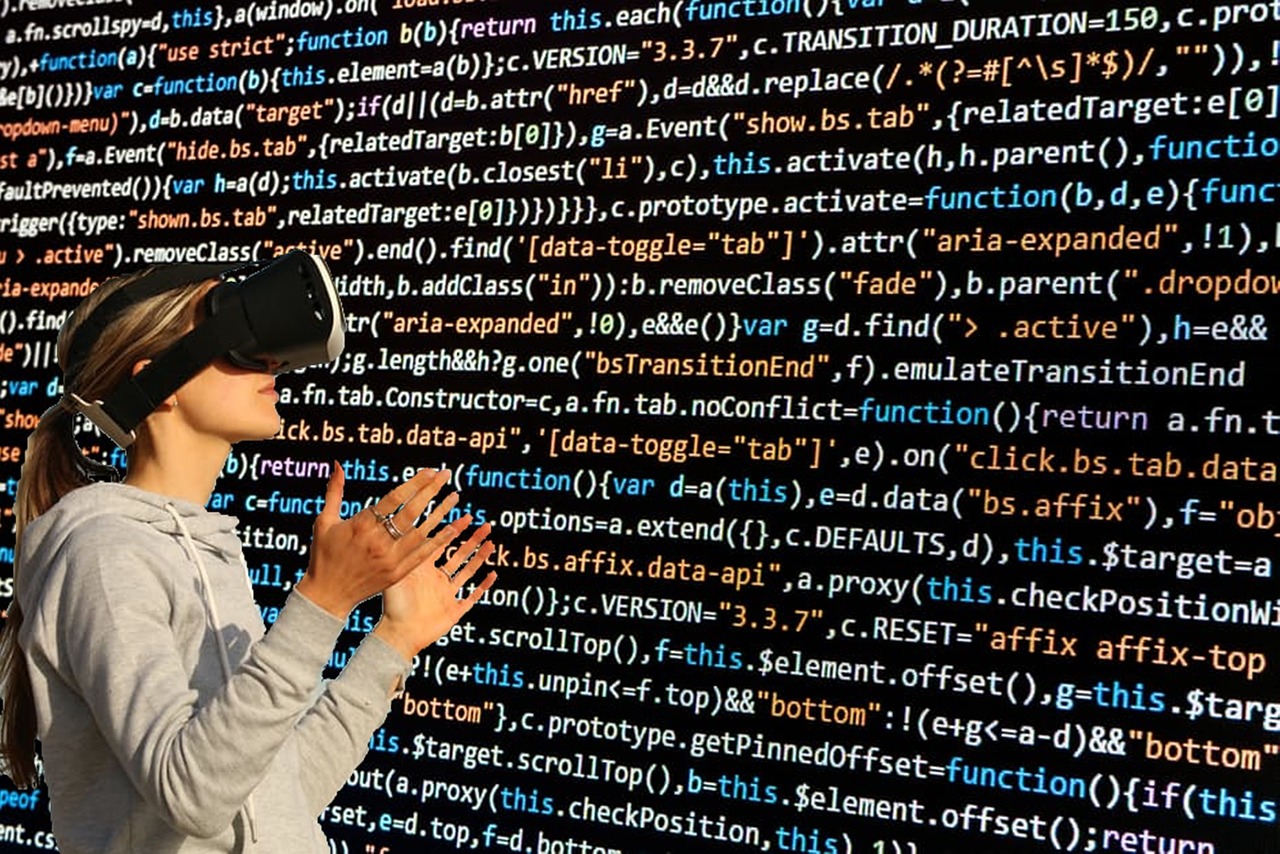
Techniques for Data Integration
Integrating quantitative and qualitative data is not just a beneficial practice; it’s an art that can significantly enhance the depth and breadth of research findings. To achieve this integration effectively, researchers can employ several techniques that facilitate a seamless blend of numerical and narrative data. One of the most widely used methods is triangulation. This technique involves using multiple data sources or methods to cross-verify results, ensuring a more robust outcome. For instance, a researcher studying consumer behavior might combine survey data (quantitative) with in-depth interviews (qualitative) to confirm trends and insights.
Another effective technique is data transformation, which involves converting qualitative data into quantifiable formats. This can be achieved through coding qualitative responses into numerical values, allowing them to be analyzed statistically. For example, responses from open-ended survey questions can be categorized into themes and then assigned a numerical score based on frequency or sentiment. This transformation not only enriches the data but also makes it easier to integrate with existing quantitative datasets.
Moreover, leveraging software tools can streamline the integration process significantly. Tools like NVivo or MAXQDA are designed to handle mixed methods research, allowing researchers to organize, analyze, and visualize both qualitative and quantitative data in one platform. These tools often come equipped with features that facilitate data coding, pattern recognition, and even statistical analysis, making them invaluable for researchers who wish to maintain a cohesive workflow.
In addition to these techniques, it’s crucial to maintain a clear methodological framework throughout the integration process. This framework should outline how data will be collected, analyzed, and interpreted. By establishing a clear plan, researchers can avoid common pitfalls such as data incompatibility or misinterpretation of results. Furthermore, documenting the integration process ensures transparency and reproducibility, which are essential in academic research.
Ultimately, the key to successful data integration lies in understanding the strengths and limitations of both quantitative and qualitative data. By employing these techniques thoughtfully, researchers can create a more nuanced and comprehensive understanding of their research topics, leading to richer insights and more informed conclusions.
- What is the best method for integrating quantitative and qualitative data?
There is no one-size-fits-all answer, as the best method depends on the specific research context. However, triangulation and data transformation are commonly effective techniques. - Can software tools really help with data integration?
Absolutely! Tools like NVivo and MAXQDA are designed to facilitate the integration of mixed methods, making analysis more efficient and organized. - What challenges might arise during data integration?
Common challenges include methodological differences between data types, compatibility issues, and the potential for misinterpretation of results.
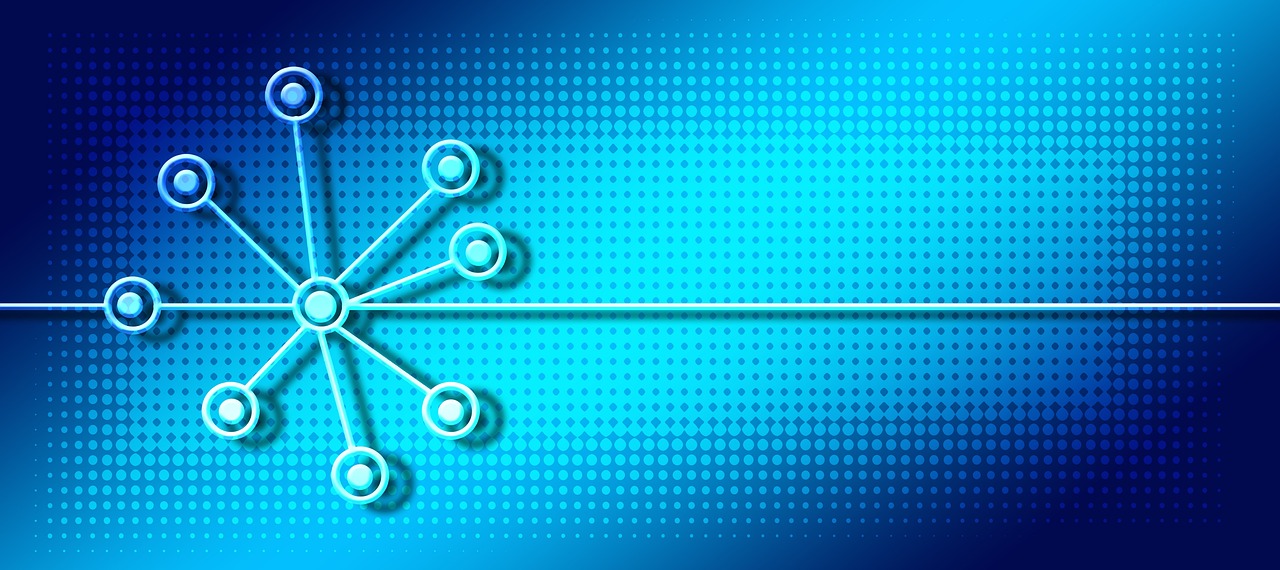
Case Studies of Successful Integration
When it comes to integrating quantitative and qualitative data, the real-world applications can be both enlightening and inspiring. Let’s delve into some fascinating case studies that showcase how organizations and researchers have successfully blended these two data types to achieve remarkable outcomes. One notable example comes from the field of healthcare, where a hospital system sought to improve patient satisfaction ratings. By combining quantitative data from patient surveys (numerical ratings of their experiences) with qualitative data gathered from open-ended interview responses, the hospital was able to identify specific areas for improvement. They discovered that while patients rated their care highly in numerical terms, their comments revealed underlying issues related to communication and empathy. This integration allowed the hospital to implement targeted training programs, resulting in a significant increase in patient satisfaction scores.
Another compelling case study is found in the realm of education. A university conducted a mixed-methods research project to evaluate the effectiveness of a new teaching strategy. The quantitative aspect involved standardized test scores, while the qualitative component included student interviews and classroom observations. The results were enlightening; while test scores showed a modest improvement, the qualitative data painted a richer picture of student engagement and enthusiasm. This comprehensive understanding led the university to adopt the teaching strategy more widely, showcasing how integrating both data types can lead to informed decision-making.
In the business sector, a retail company analyzed consumer behavior by merging sales data (quantitative) with customer feedback (qualitative). They utilized advanced analytics to identify trends in purchasing patterns, but it was the qualitative insights that revealed why customers preferred certain products over others. The combination of these data types enabled the company to refine its marketing strategies effectively, tailoring promotions to resonate with customer sentiments. This case illustrates how integration not only enhances understanding but also drives strategic initiatives that can lead to increased revenue.
In the social sciences, researchers studying community health interventions employed a mixed-methods approach to evaluate the impact of their programs. They collected quantitative data through health metrics and surveys while also conducting focus groups to gather personal stories and experiences from community members. This dual approach allowed researchers to quantify improvements in health outcomes while also understanding the social dynamics and individual experiences that contributed to these changes. The success of this integration provided a model for future community health initiatives.
These case studies exemplify the power of integrating quantitative and qualitative data across various fields. By leveraging both data types, organizations can gain a more nuanced understanding of their subjects, leading to better-informed decisions and more effective outcomes. As we continue to explore the benefits of data integration, it becomes clear that the future of research and analysis lies in the harmonious blend of numbers and narratives.
- What is the main benefit of integrating quantitative and qualitative data?
Integrating these data types provides a more comprehensive understanding of research topics, allowing for richer insights and informed decision-making. - Can you give an example of a field that benefits from data integration?
Fields like healthcare, education, and business research have all seen significant advantages from combining quantitative and qualitative data. - What challenges might arise when integrating these data types?
Common challenges include methodological differences, data compatibility issues, and the need for specialized skills to analyze mixed methods effectively.
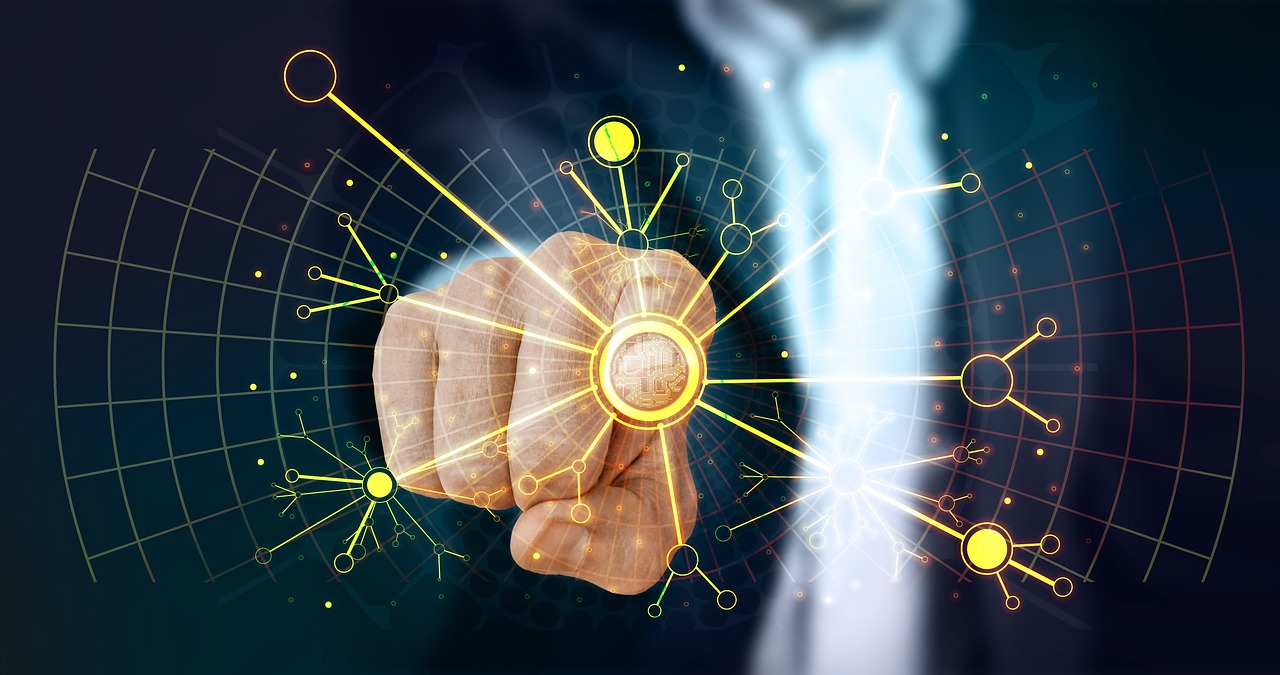
Applications in Social Sciences
In the realm of social sciences, the integration of quantitative and qualitative data is not just beneficial; it's essential. Researchers in fields such as sociology, psychology, and anthropology often grapple with complex social phenomena that cannot be adequately understood through numbers alone. Imagine trying to gauge the emotional impact of a community program solely by attendance figures. While those numbers are important, they only tell part of the story. To truly grasp the effectiveness of such programs, one must delve into the experiences and feelings of the participants involved.
For instance, consider a study aimed at understanding the effects of a new educational initiative in underprivileged communities. Researchers might collect quantitative data through standardized test scores to measure academic performance. However, to enrich their findings, they could also conduct interviews or focus groups with students, teachers, and parents. This qualitative data can provide insights into the barriers to learning, the motivations behind student engagement, and the overall sentiment towards the educational changes. By weaving together these two data types, researchers can paint a more comprehensive picture of the initiative's impact.
Moreover, integrating these data forms allows for a more nuanced analysis of social issues. For example, a researcher examining the relationship between poverty and mental health might use surveys to collect quantitative data on income levels and mental health diagnoses. However, to understand the lived experiences of those affected, they could conduct in-depth interviews to explore how poverty shapes individuals' mental health. This combination not only enhances the validity of the research but also enables researchers to uncover patterns and themes that numeric data alone might miss.
In practice, the applications of integrated data methods in social sciences can be categorized into several key areas:
- Policy Development: By understanding both the statistics and personal stories behind social issues, policymakers can create more effective and empathetic solutions.
- Community Engagement: Engaging with community members through qualitative methods fosters trust and ensures that their voices are heard in the research process.
- Program Evaluation: Evaluating social programs through a mixed-methods lens allows for a more holistic understanding of their effectiveness and areas for improvement.
Ultimately, the integration of quantitative and qualitative data in social sciences is akin to assembling a jigsaw puzzle. Each piece—whether a statistic or a personal narrative—contributes to a larger, more vibrant picture of human behavior and societal trends. By embracing both data types, researchers can not only enhance their analyses but also advocate for meaningful change in society.
- What is the main benefit of integrating quantitative and qualitative data?
Integrating these data types provides a more comprehensive understanding of research topics, leading to richer insights and more informed conclusions. - Can qualitative data be quantified?
Yes, qualitative data can often be coded and quantified to some extent, allowing researchers to analyze patterns and trends statistically. - How do researchers ensure the reliability of mixed methods?
Researchers often use triangulation, which involves comparing and cross-validating results from different data sources to enhance reliability.
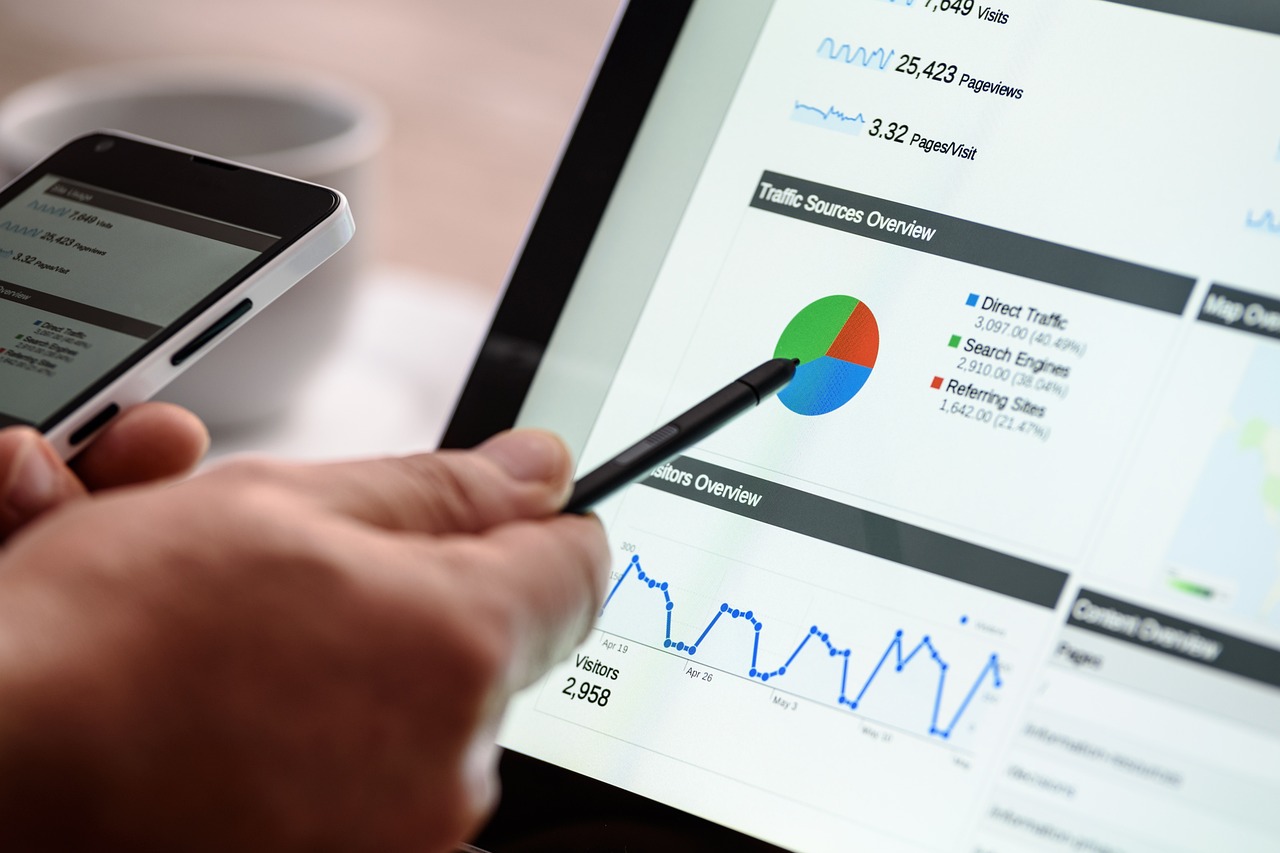
Applications in Business Research
In the fast-paced world of business, understanding consumer behavior is crucial for success. Integrating quantitative and qualitative data provides businesses with a comprehensive view of their market, enabling them to make informed decisions. By combining numerical data—such as sales figures or market share—with insights from customer interviews and surveys, companies can uncover trends and patterns that might otherwise remain hidden.
For instance, consider a company that sells athletic wear. By analyzing sales data (quantitative), they might notice a spike in sales during the summer months. However, to fully understand the reasons behind this trend, they could conduct focus groups or customer interviews (qualitative). These discussions might reveal that customers prefer to exercise outdoors during warmer weather, prompting the company to launch a targeted marketing campaign for their summer collection.
Moreover, the integration of these data types allows businesses to tailor their products and services more effectively. A well-rounded approach can lead to:
- Enhanced Customer Insights: Understanding not just what customers are buying, but why they are making those choices.
- Improved Product Development: Utilizing feedback from customers to innovate and refine offerings.
- Strategic Marketing: Developing campaigns that resonate with the target audience based on a deeper understanding of their preferences.
To illustrate this further, let’s look at a table comparing traditional research methods with mixed methods:
Aspect | Traditional Research | Mixed Methods |
---|---|---|
Data Type | Quantitative only | Quantitative and Qualitative |
Insights | Statistical trends | In-depth understanding of consumer motivations |
Flexibility | Less adaptable | Highly adaptable to changing market conditions |
Outcome | Generalized findings | Tailored strategies based on comprehensive data |
In conclusion, the integration of quantitative and qualitative data in business research not only enriches the understanding of consumer behavior but also enhances the overall strategic approach of organizations. By leveraging the strengths of both data types, businesses can navigate the complexities of the market more effectively, ultimately leading to greater success and customer satisfaction.
- What is the main benefit of integrating quantitative and qualitative data?
Integrating these data types allows for a more comprehensive understanding of consumer behavior, leading to better decision-making. - How can businesses collect qualitative data?
Businesses can gather qualitative data through interviews, focus groups, and open-ended survey questions. - Are there any challenges in integrating these data types?
Yes, challenges can include methodological differences and ensuring data compatibility.
Frequently Asked Questions
- What is the difference between quantitative and qualitative data?
Quantitative data is all about numbers, measurable and statistically analyzable, like survey results or sales figures. On the other hand, qualitative data dives into the why and how, capturing experiences and opinions, often through interviews or open-ended surveys. Think of quantitative data as the backbone of your research, while qualitative data adds the flesh and blood, giving it life!
- Why is integrating both data types important?
Integrating quantitative and qualitative data enhances the depth and quality of research. It allows you to validate findings, explore underlying reasons, and gain a comprehensive view of the topic at hand. Imagine trying to solve a puzzle; quantitative data gives you the pieces, while qualitative data helps you see the bigger picture!
- What are some common challenges in data integration?
One major challenge is the methodological differences between the two data types, which can lead to compatibility issues. For instance, quantitative data might require strict numerical formats, while qualitative data is more fluid and subjective. Navigating these differences can be tricky, but overcoming them is key to successful integration!
- Can you give examples of techniques for data integration?
Absolutely! Some effective techniques include triangulation, where you cross-verify data from different sources, and data transformation, which involves converting qualitative insights into quantifiable metrics. Additionally, using specialized software tools can streamline the integration process, making it easier to manage and analyze combined datasets.
- How is data integration applied in social sciences?
In social sciences, integrating both data types allows researchers to analyze complex social phenomena more deeply. For instance, a study on community health might combine statistical data on disease prevalence with personal stories from affected individuals, providing a richer understanding of the issue.
- What benefits does data integration offer in business research?
Data integration in business research offers comprehensive insights into consumer behavior, helping companies make informed strategic decisions. By combining sales data with customer feedback, businesses can tailor their marketing strategies more effectively, ensuring they meet the needs and preferences of their target audience.