The Future of AI in Fraud Detection
As we step into an era dominated by technology, the role of artificial intelligence (AI) in various sectors is becoming increasingly pivotal, especially in the realm of fraud detection. Fraud is a pervasive issue that can cripple businesses and erode consumer trust. With the rise of digital transactions and online services, fraudsters are becoming more sophisticated, making traditional detection methods obsolete. Enter AI—a game changer in the fight against fraud. This article dives into how AI is transforming fraud detection, exploring its applications, benefits, challenges, and what the future holds for this dynamic intersection of technology and security.
To grasp the significance of AI in fraud detection, it's essential to understand what AI is. In simple terms, AI refers to the simulation of human intelligence in machines that are programmed to think and learn. In the context of fraud detection, AI leverages its ability to analyze vast amounts of data, identify patterns, and recognize anomalies that might indicate fraudulent activities. Imagine having a digital detective that tirelessly sifts through countless transactions, flagging those that don't quite fit the norm. That's the power of AI at work.
Utilizing AI for fraud prevention comes with a host of advantages. First and foremost, AI systems can process and analyze data at lightning speed, far surpassing human capabilities. This means organizations can detect fraud in real-time, significantly reducing potential losses. Moreover, AI's ability to learn from historical data allows it to improve its detection rates over time. With each interaction, the system becomes smarter, more efficient, and more accurate.
One of the standout features of AI in fraud detection is its capacity for real-time monitoring. This means that as transactions occur, AI systems are on the lookout for any suspicious activities. For instance, if a credit card is suddenly used in a different country just minutes after a transaction in the home country, AI can flag this immediately. This proactive approach not only helps in detecting fraud but also in minimizing the damage it can cause.
At the heart of AI's effectiveness in fraud detection are machine learning algorithms. These algorithms are designed to learn from past data and improve their predictive capabilities over time. For example, a machine learning model can be trained on thousands of historical transactions, identifying which patterns are indicative of fraud. As it processes more data, it becomes increasingly adept at spotting irregularities. Here’s a quick overview of some commonly used algorithms:
Algorithm | Description |
---|---|
Decision Trees | Breaks down data into branches to make decisions based on various conditions. |
Neural Networks | Mimics human brain functions to recognize complex patterns. |
Support Vector Machines | Finds the optimal boundary between different classes of data. |
Another exciting aspect of AI in fraud detection is predictive analytics. This technique allows organizations to anticipate fraudulent behaviors before they even occur. By analyzing trends and patterns from historical data, AI can forecast potential fraud scenarios, enabling businesses to implement preventive measures proactively. Think of it as having a crystal ball that not only shows you what's happening now but also what might happen in the future.
Despite the myriad benefits, implementing AI in fraud detection isn't without its challenges. Organizations often grapple with data privacy concerns, as the collection and analysis of personal data can lead to ethical dilemmas. Additionally, integrating AI systems with existing infrastructures can be complex and may require significant investment. Lastly, the demand for skilled personnel who can manage and interpret AI outputs is ever-increasing, placing another hurdle in the path of successful implementation.
Looking ahead, the landscape of AI in fraud detection is set to evolve dramatically. We can expect advancements in technology that will enhance the capabilities of AI systems. The rise of deep learning is particularly noteworthy, as it allows for even deeper analysis of data, uncovering insights that traditional methods might miss. Furthermore, the collaboration between AI systems and human analysts is becoming increasingly important, as this hybrid approach can significantly elevate fraud detection effectiveness.
While AI is powerful, it’s not infallible. The importance of combining AI’s capabilities with human expertise cannot be overstated. Humans bring intuition, contextual understanding, and ethical considerations to the table, which AI lacks. This collaboration can lead to more comprehensive fraud detection strategies, ensuring that both technology and human insight work hand in hand.
As AI technologies continue to develop, so too does the regulatory landscape surrounding them. Organizations must navigate a complex web of compliance and ethical considerations when deploying AI in fraud detection. Staying abreast of regulations ensures that companies not only protect themselves from legal repercussions but also maintain consumer trust.
- What is the primary benefit of using AI in fraud detection?
AI offers real-time monitoring and analysis, allowing for quicker identification and response to fraudulent activities. - How does machine learning improve fraud detection?
Machine learning algorithms learn from historical data, enhancing their ability to recognize patterns and anomalies over time. - What challenges do organizations face when implementing AI?
Challenges include data privacy concerns, integration issues, and the need for skilled personnel. - Why is collaboration between AI and humans important?
Human expertise complements AI's capabilities, leading to more effective fraud detection strategies.
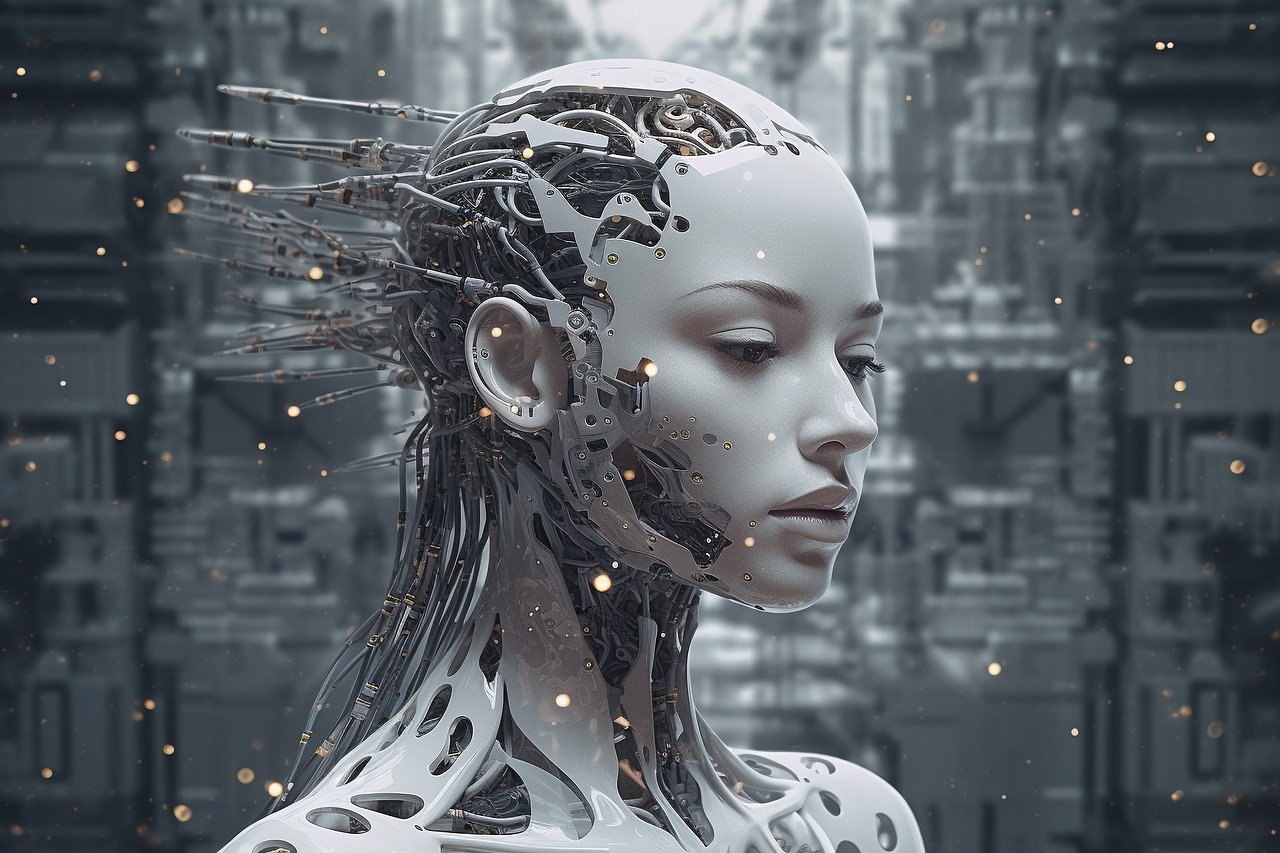
Understanding AI and Fraud Detection
Artificial Intelligence (AI) has become a game changer in various sectors, and one of the most impactful applications is in fraud detection. But what exactly is AI? In simple terms, AI refers to the simulation of human intelligence in machines that are programmed to think and learn like humans. This capability allows organizations to sift through massive datasets, uncovering patterns and anomalies that would be nearly impossible for a human analyst to detect in a reasonable timeframe.
When it comes to fraud detection, AI utilizes advanced algorithms to analyze transaction data, customer behavior, and historical patterns. By doing so, it can pinpoint suspicious activities that suggest fraudulent behavior. For example, if a credit card is suddenly used in a different country, AI can flag this as unusual based on the user's typical behavior. This ability to analyze data in real-time is what sets AI apart from traditional fraud detection methods.
AI systems can be trained using machine learning, a subset of AI that enables these systems to improve their detection rates over time. By feeding the system historical data about past fraud cases, it learns to recognize the signs of fraud more accurately. This is akin to teaching a child to recognize different types of fruits by showing them various examples until they can identify them independently.
The beauty of AI in fraud detection lies in its ability to adapt. As fraudsters become more sophisticated, AI systems can evolve alongside them. They can analyze trends and adapt their algorithms to counteract new methods of fraud, essentially staying one step ahead. This dynamic capability makes AI a crucial ally in the fight against fraud.
Moreover, AI's potential is not limited to just identifying fraud; it also plays a significant role in preventing it. By employing predictive analytics, organizations can foresee potential fraudulent activities before they even happen. This proactive approach is vital in minimizing losses and safeguarding assets.
In summary, the integration of AI into fraud detection systems is transforming how organizations address fraudulent activities. With its ability to analyze data patterns, learn from historical cases, and predict future incidents, AI is not just a tool but a powerful partner in the quest for security and trust in financial transactions.
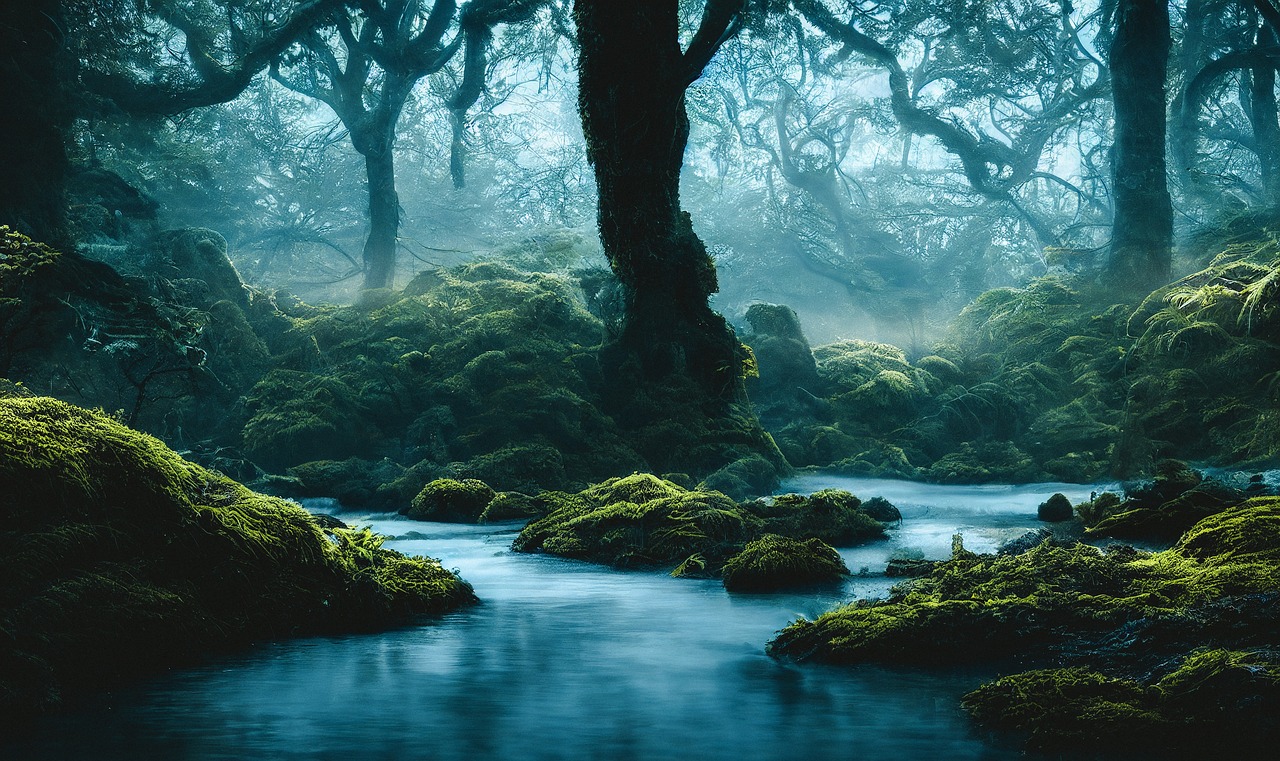
Benefits of AI in Fraud Prevention
Artificial Intelligence (AI) has revolutionized the way organizations combat fraud, introducing a plethora of benefits that enhance both efficiency and effectiveness. One of the most significant advantages of AI in fraud prevention is its unparalleled accuracy. Traditional methods often rely on rule-based systems that can miss subtle patterns indicative of fraudulent activity. In contrast, AI systems utilize sophisticated algorithms that learn from vast datasets, enabling them to identify anomalies that human analysts might overlook. This capability not only increases the detection rate but also reduces the number of false positives, allowing businesses to focus their resources more effectively.
Another key benefit is the efficiency that AI brings to the table. In a world where transactions occur at lightning speed, the ability to process and analyze data in real-time is crucial. AI systems can sift through millions of transactions per second, flagging suspicious activities almost instantaneously. This real-time monitoring means that organizations can respond to potential fraud as it happens, significantly minimizing potential losses. Imagine a security guard who can monitor every inch of a sprawling complex simultaneously—this is the kind of vigilance AI offers in the fight against fraud.
Moreover, AI's ability to handle vast amounts of data is a game-changer. In today's digital landscape, businesses generate an overwhelming volume of transactional data daily. AI technologies can not only manage this data but also extract meaningful insights from it. For instance, machine learning algorithms can detect trends over time, learning from historical data to improve their predictive capabilities. This means that as the system processes more data, it becomes increasingly adept at identifying fraudulent behaviors before they escalate.
To illustrate the benefits further, consider the following table that summarizes the key advantages of AI in fraud prevention:
Benefit | Description |
---|---|
Increased Accuracy | AI systems reduce false positives and improve detection rates by learning from complex data patterns. |
Real-Time Monitoring | AI can analyze transactions as they occur, allowing for immediate response to suspicious activities. |
Data Handling | AI can process vast amounts of data, providing insights that enhance predictive capabilities. |
In addition to these benefits, AI systems also utilize predictive analytics. This technology allows organizations to anticipate fraudulent activities before they occur. By analyzing historical data and identifying patterns, AI can forecast potential threats, enabling businesses to implement preventive measures proactively. It's like having a weather forecast that not only tells you it's going to rain but also advises you to carry an umbrella to avoid getting soaked!
In conclusion, the integration of AI in fraud prevention is not just a trend—it's a necessity for organizations aiming to safeguard their assets and maintain customer trust. With its ability to provide increased accuracy, efficiency, and predictive insights, AI stands as a formidable ally in the ongoing battle against fraud.
- How does AI improve fraud detection accuracy?
AI improves accuracy by analyzing complex data patterns and learning from historical data, which helps in identifying anomalies that traditional methods may miss. - Can AI systems operate in real-time?
Yes, AI systems can monitor transactions in real-time, allowing organizations to respond to suspicious activities immediately. - What role does predictive analytics play in fraud prevention?
Predictive analytics helps organizations anticipate fraudulent behaviors by analyzing trends from historical data, enabling proactive measures to be taken.
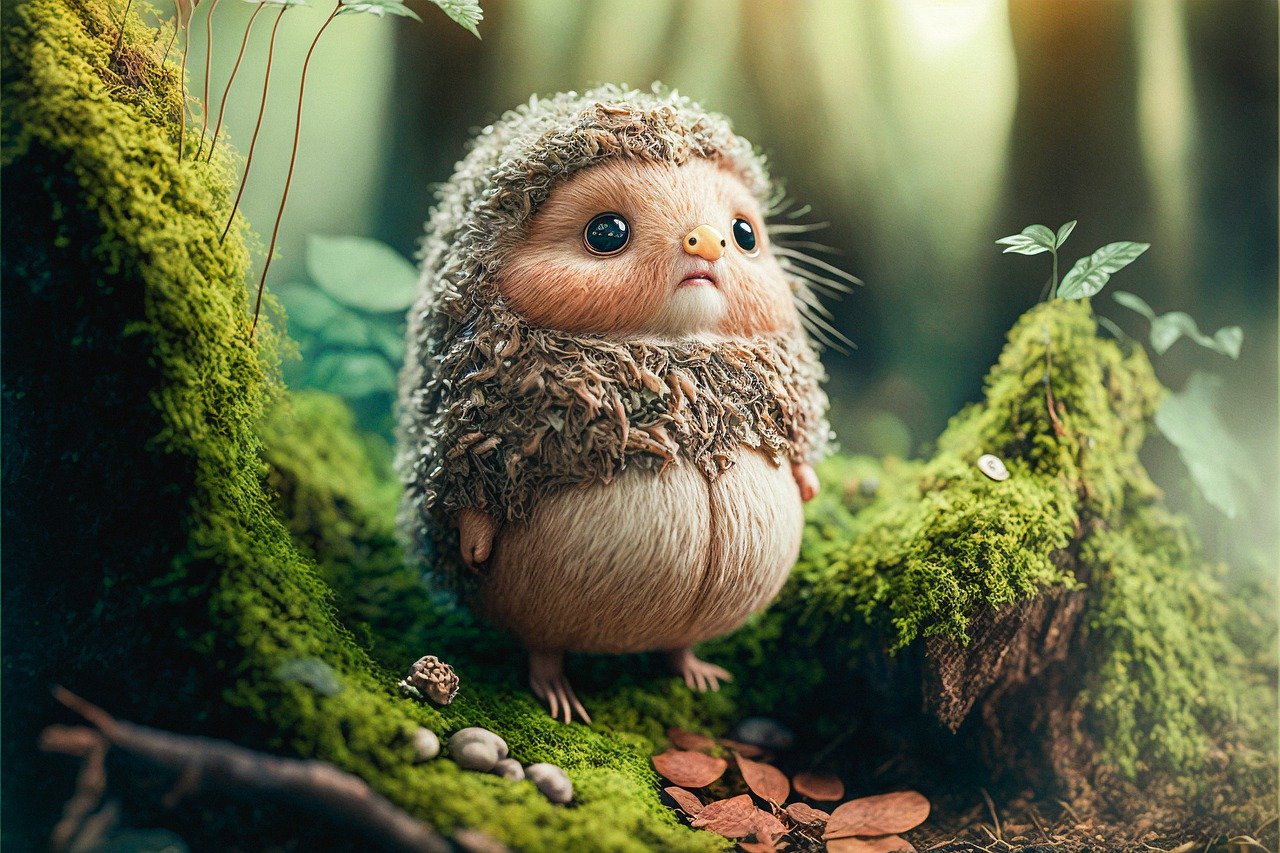
Real-Time Monitoring
Imagine walking into a store and having a personal security guard who knows your shopping habits inside and out. This is essentially what with artificial intelligence (AI) offers businesses in the realm of fraud detection. By leveraging advanced algorithms and machine learning, organizations can monitor transactions as they happen, identifying suspicious activities almost instantaneously. This capability is like having a vigilant eye that never blinks, ensuring that any anomalies are caught before they escalate into significant losses.
The beauty of real-time monitoring lies in its ability to process vast amounts of data at lightning speed. For instance, consider a financial institution that processes thousands of transactions every minute. Traditional methods of fraud detection may involve manual checks or delayed alerts, which can allow fraudulent activities to slip through the cracks. However, with AI, every transaction is analyzed against a backdrop of historical data and behavioral patterns. If something seems off—like a sudden spike in spending or a transaction from an unusual location—the system can flag it immediately. This not only helps in mitigating risks but also enhances customer trust, as clients feel more secure knowing their transactions are being monitored continuously.
Moreover, the integration of real-time monitoring with AI enables organizations to employ sophisticated techniques such as anomaly detection. This approach allows the system to learn and adapt over time, becoming more adept at recognizing what constitutes 'normal' behavior for each user. For example, if a customer typically makes small purchases at local stores, but suddenly attempts to buy high-ticket items overseas, the AI can recognize this deviation and trigger an alert. This proactive stance is crucial in today’s fast-paced digital environment, where fraudsters are constantly evolving their tactics.
In addition to immediate alerts, real-time monitoring can also provide valuable insights into trends and patterns over time. By analyzing data continuously, organizations can create a comprehensive profile of customer behavior, allowing them to refine their fraud detection strategies further. This not only helps in preventing fraud but also optimizes the overall customer experience by reducing false positives—those frustrating moments when legitimate transactions are mistakenly flagged as fraudulent.
To illustrate the effectiveness of real-time monitoring, consider the following table that outlines the key benefits of implementing AI-driven monitoring systems in fraud detection:
Benefit | Description |
---|---|
Immediate Alerts | Fraudulent transactions are flagged as they occur, enabling swift action. |
Reduced False Positives | Enhanced accuracy leads to fewer legitimate transactions being incorrectly flagged. |
Continuous Learning | AI systems evolve with changing fraud patterns, improving detection over time. |
Enhanced Customer Trust | Customers feel safer knowing their transactions are monitored effectively. |
In conclusion, real-time monitoring powered by AI is transforming the landscape of fraud detection. It provides organizations with the tools they need to stay one step ahead of fraudsters, ensuring that they can respond quickly and effectively to threats. As technology continues to advance, the potential for even more sophisticated monitoring systems will only grow, making it an exciting area to watch in the future of fraud prevention.
- What is real-time monitoring in fraud detection?
Real-time monitoring refers to the continuous analysis of transactions as they occur, allowing organizations to detect and respond to fraudulent activities immediately. - How does AI improve fraud detection accuracy?
AI improves fraud detection accuracy by learning from historical data and identifying patterns, which helps reduce false positives and enhances the overall detection rate. - Can real-time monitoring prevent all types of fraud?
While real-time monitoring significantly reduces the risk of fraud, it may not prevent all types, especially those that are highly sophisticated or involve insider threats. - What industries benefit most from AI in fraud detection?
Industries such as finance, e-commerce, insurance, and telecommunications benefit greatly from AI-driven fraud detection due to the high volume of transactions and the potential for significant losses.
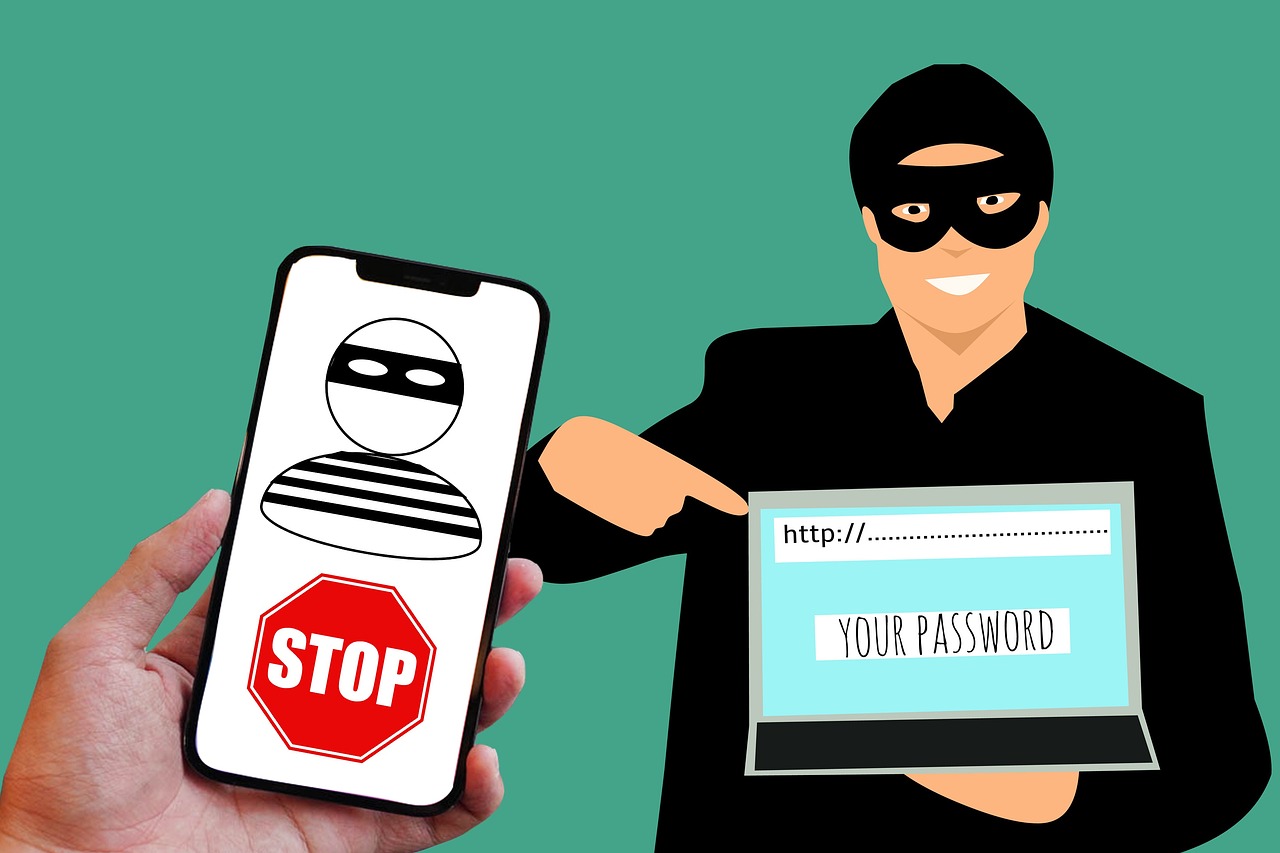
Machine Learning Algorithms
When it comes to fraud detection, machine learning algorithms are the backbone of the technology that helps organizations combat fraudulent activities. These algorithms are designed to analyze vast amounts of data, recognize patterns, and identify anomalies that could signify fraudulent behavior. Think of them as the detectives of the digital world, tirelessly sifting through mountains of transactions to spot the criminals hiding in plain sight.
There are several types of machine learning algorithms that play a crucial role in fraud detection. Some of the most effective include:
- Supervised Learning: This involves training the algorithm on a labeled dataset, where the outcomes (fraudulent or not) are known. The model learns to predict outcomes based on this training data, making it highly effective for identifying known fraud patterns.
- Unsupervised Learning: Unlike supervised learning, this method works with unlabeled data. It tries to identify hidden patterns or groupings within the data. This is particularly useful for detecting new or unknown types of fraud that have not been previously documented.
- Reinforcement Learning: This type of learning involves algorithms that learn to make decisions by receiving rewards or penalties based on their actions. In fraud detection, it can continuously improve its detection strategies based on real-time feedback.
Each of these algorithms has its strengths and weaknesses, and the choice of which to use often depends on the specific context of the fraud detection task at hand. For instance, supervised learning is excellent for established fraud patterns, while unsupervised learning shines when new fraud tactics emerge that haven’t been seen before.
Moreover, as these algorithms process more data, they become increasingly adept at identifying subtle signs of fraud that human analysts might miss. This capability is enhanced by the use of ensemble methods, which combine multiple algorithms to improve accuracy and reduce the likelihood of false positives. Imagine having a team of detectives, each with their unique skills, working together to crack a case; that’s what ensemble methods do for fraud detection.
In summary, machine learning algorithms are not just tools; they are essential partners in the fight against fraud. By leveraging their capabilities, organizations can significantly enhance their fraud detection efforts, ensuring they stay one step ahead of fraudsters. As technology continues to evolve, we can expect even more sophisticated algorithms to emerge, further transforming the landscape of fraud detection.
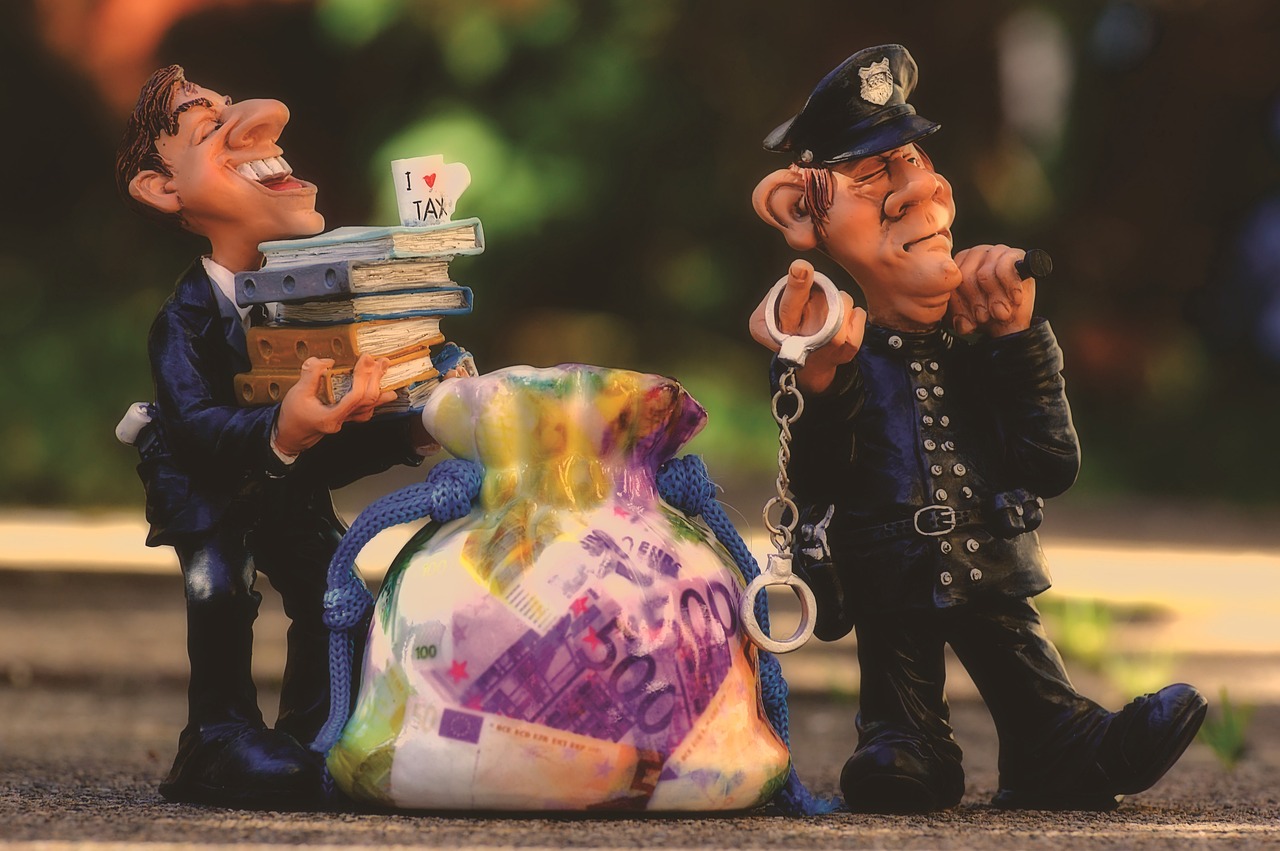
Predictive Analytics
Predictive analytics is a powerful tool that leverages the vast capabilities of artificial intelligence to forecast potential fraudulent activities before they even occur. Imagine having a crystal ball that not only reveals what might happen but also allows organizations to take proactive measures. By analyzing historical data and recognizing patterns, predictive analytics can identify behaviors that are indicative of fraud. This not only enhances the ability to prevent fraud but also significantly reduces the time and resources spent on investigating false positives.
At the heart of predictive analytics are sophisticated algorithms that sift through mountains of data to find correlations and anomalies. These algorithms are trained on past incidents of fraud, learning from them to create models that predict future occurrences. For instance, if a particular transaction pattern has led to fraud in the past, the system can flag similar transactions in real-time, allowing organizations to act swiftly. This predictive capability is akin to having a seasoned detective on the case, always vigilant and ready to alert the team at the first sign of trouble.
Moreover, the effectiveness of predictive analytics is enhanced by its ability to continuously learn and adapt. As new data comes in, the algorithms refine their models, improving their accuracy over time. This dynamic learning process means that organizations are not just relying on static rules; they are equipped with a system that evolves alongside emerging fraud tactics. In a world where fraudsters are constantly honing their techniques, this adaptability is crucial.
To illustrate the impact of predictive analytics in fraud detection, consider the following table that summarizes its key advantages:
Advantage | Description |
---|---|
Proactive Detection | Allows organizations to identify and mitigate potential fraud before it happens. |
Reduced False Positives | Improves accuracy in identifying genuine fraudulent activities, minimizing unnecessary investigations. |
Continuous Improvement | Adapts to new data and emerging fraud patterns, enhancing detection capabilities over time. |
In conclusion, predictive analytics is not just a buzzword; it's a game-changer in the realm of fraud detection. By harnessing the power of AI, organizations can stay one step ahead of fraudsters, ensuring their operations remain secure and efficient. With the right predictive tools in place, businesses can transform their approach to fraud prevention, making it more strategic and effective than ever before.
Frequently Asked Questions
- What is predictive analytics in fraud detection? Predictive analytics involves using historical data and algorithms to forecast potential fraudulent activities before they occur.
- How does predictive analytics improve fraud detection? By identifying patterns and anomalies in data, predictive analytics allows organizations to proactively address potential fraud, reducing the chances of losses.
- Can predictive analytics adapt to new fraud tactics? Yes, predictive analytics systems continuously learn from new data, improving their models and adapting to emerging fraud techniques.
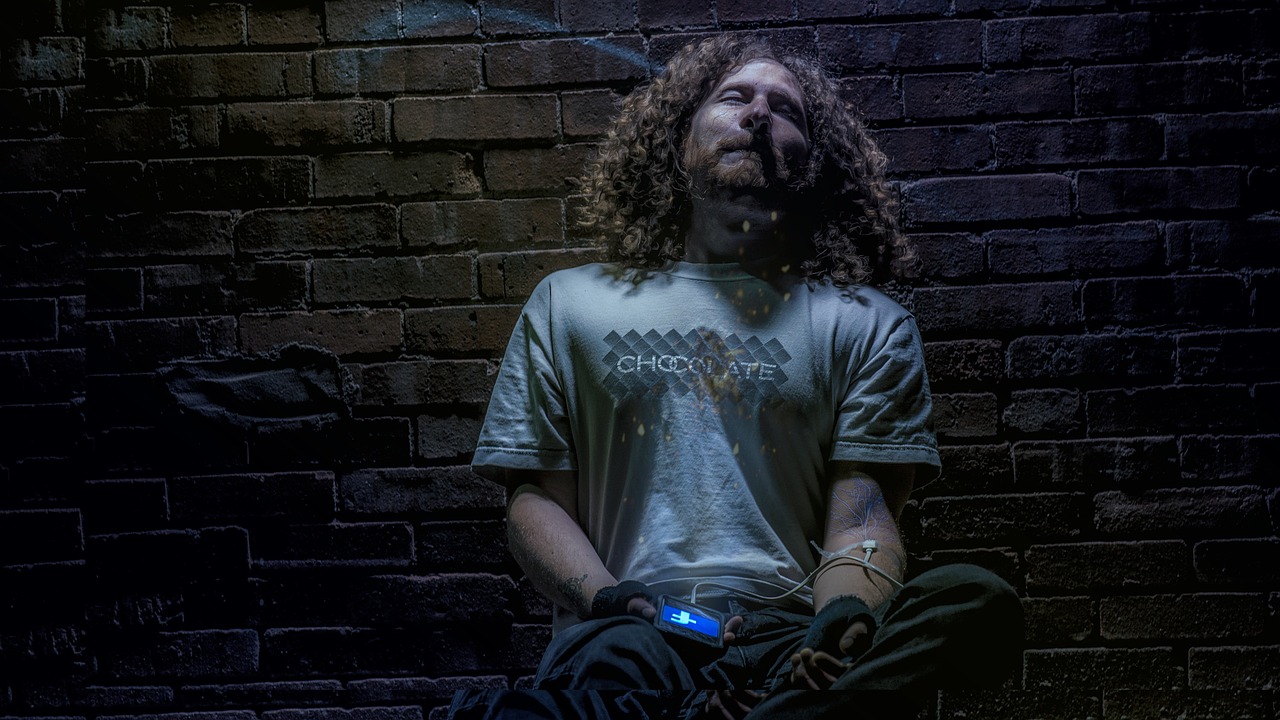
Challenges in Implementing AI
Implementing artificial intelligence (AI) in fraud detection is not without its hurdles. While the potential benefits are immense, organizations often encounter several significant challenges that can impede the successful integration of AI technologies. One of the primary issues is data privacy concerns. With the increasing scrutiny on how data is collected, stored, and used, companies must navigate a complex landscape of regulations such as GDPR and CCPA. This can make it difficult to gather the necessary data for training AI models, as organizations must ensure they are compliant with these legal frameworks.
Another challenge is the integration of AI systems with existing infrastructure. Many organizations have legacy systems that may not be compatible with modern AI solutions. This can lead to increased costs and extended timelines as businesses work to upgrade or replace outdated technology. Additionally, there is often a lack of understanding among stakeholders about how AI can be effectively utilized in fraud detection, which can create resistance to adopting these innovative solutions.
Moreover, the need for skilled personnel cannot be overstated. AI and machine learning require a specific set of skills that many organizations may not currently possess. Finding professionals who are not only well-versed in AI technologies but also understand the intricacies of fraud detection can be a daunting task. This skills gap can delay the implementation process and hinder the effectiveness of AI systems.
To further illustrate these challenges, consider the following table that summarizes the key obstacles faced by organizations:
Challenge | Description |
---|---|
Data Privacy Concerns | Compliance with regulations like GDPR and CCPA makes data collection difficult. |
Integration Issues | Legacy systems may not be compatible with new AI technologies, leading to increased costs. |
Skilled Personnel Shortage | Finding professionals with expertise in both AI and fraud detection is challenging. |
In addition to these challenges, organizations must also consider the ethical implications of deploying AI technologies. As AI systems become more autonomous, there is a growing concern about the potential for bias in decision-making processes. If AI models are trained on biased data, they may inadvertently perpetuate existing inequalities, leading to unfair treatment of certain groups. Thus, organizations must be vigilant in ensuring that their AI systems are designed and implemented in an ethical manner.
In conclusion, while the integration of AI in fraud detection holds great promise, organizations must carefully navigate the myriad challenges that come with it. From data privacy and integration issues to the need for skilled personnel and ethical considerations, the road to successful AI implementation is fraught with obstacles. However, with the right strategies and a commitment to overcoming these challenges, businesses can harness the power of AI to significantly enhance their fraud detection capabilities.
- What are the primary challenges in implementing AI for fraud detection?
Organizations face data privacy concerns, integration issues with legacy systems, and a shortage of skilled personnel. - How does data privacy affect AI implementation?
Compliance with regulations like GDPR can limit data availability for training AI models. - Why is ethical consideration important in AI?
To prevent bias in decision-making and ensure fair treatment across all demographics.
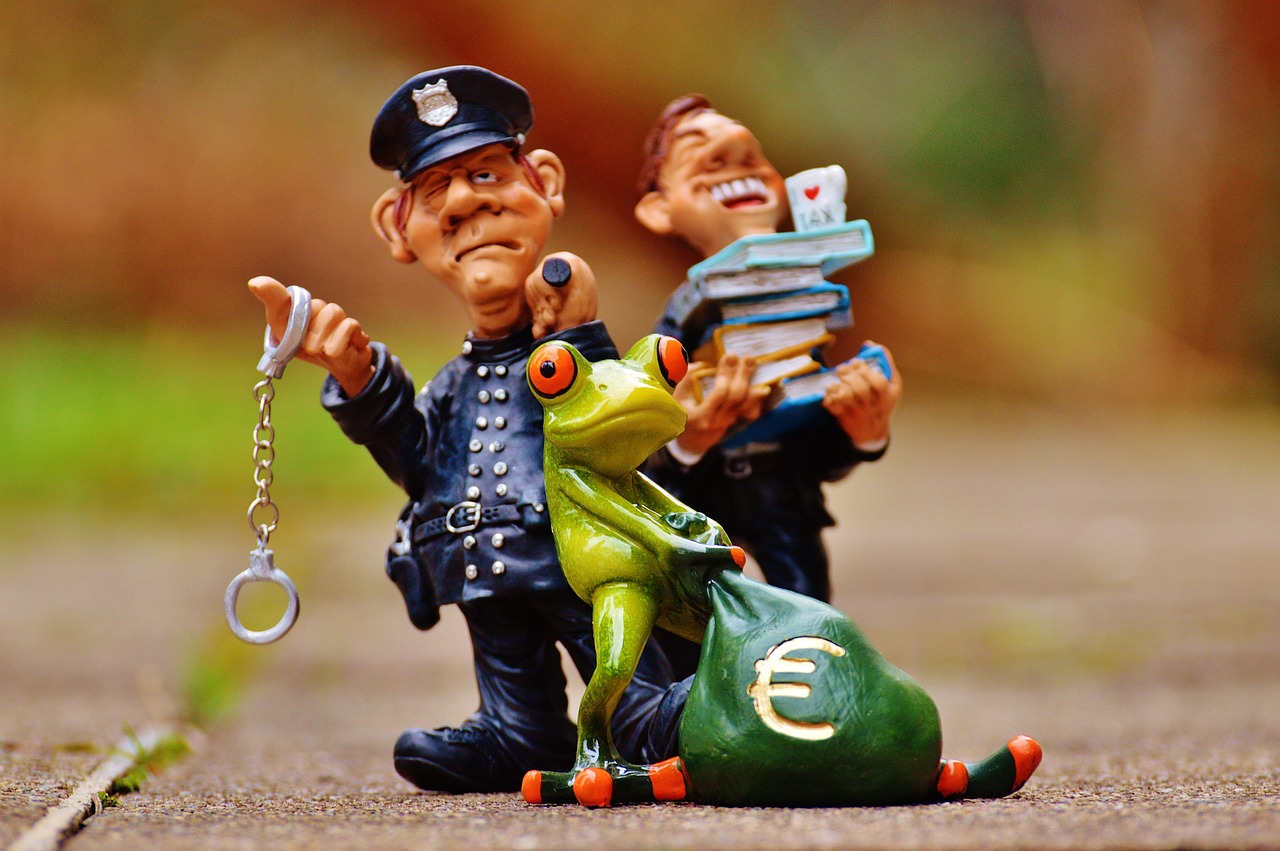
Future Trends in AI Fraud Detection
The landscape of fraud detection is rapidly evolving, driven by the relentless advancements in artificial intelligence (AI). As we look towards the future, several trends are beginning to take shape, promising to revolutionize how organizations combat fraud. One of the most exciting developments is the rise of deep learning technologies. These sophisticated algorithms are capable of processing vast amounts of data and identifying complex patterns that traditional methods might miss. Imagine having a digital detective that learns from every transaction, becoming smarter and more effective over time!
Another trend gaining traction is the increased collaboration between AI systems and human analysts. While AI can analyze data at lightning speed, human intuition and experience are irreplaceable when it comes to understanding the nuances of fraud. This hybrid approach allows organizations to leverage the strengths of both AI and human expertise, resulting in a more robust fraud detection strategy. Think of it as a dynamic duo, where AI handles the heavy lifting of data analysis, while humans provide the critical thinking and contextual understanding needed to make informed decisions.
Moreover, as AI technologies continue to mature, we can expect to see a greater emphasis on predictive analytics. This involves not just reacting to fraudulent activities but anticipating them before they occur. By analyzing historical data and identifying emerging trends, organizations can develop proactive measures to thwart potential fraud. It’s like having a crystal ball that helps businesses stay one step ahead of fraudsters!
However, with great power comes great responsibility. As the use of AI in fraud detection expands, so does the need for regulatory compliance. Organizations must navigate the evolving landscape of data privacy laws and ethical considerations surrounding AI deployment. This will require a concerted effort to ensure that AI systems are not only effective but also transparent and fair in their operations. Companies that prioritize ethical AI will not only enhance their fraud detection capabilities but also build trust with their customers.
To summarize, the future of AI in fraud detection is bright, characterized by:
- Deep Learning Technologies: Enhancing pattern recognition and anomaly detection.
- Collaboration with Human Expertise: Merging AI's analytical power with human intuition.
- Predictive Analytics: Anticipating fraud before it happens.
- Regulatory Compliance: Navigating the ethical landscape of AI usage.
As organizations embrace these trends, they will not only improve their fraud detection capabilities but also foster a culture of innovation and trust. The journey may have its challenges, but the potential rewards are enormous, paving the way for a safer and more secure digital environment.
Q1: How does AI improve fraud detection?
A1: AI enhances fraud detection by analyzing large datasets quickly, identifying patterns, and learning from historical data to improve accuracy over time.
Q2: What role do human analysts play in AI fraud detection?
A2: Human analysts provide essential context, intuition, and decision-making skills that complement AI's data processing capabilities, creating a more effective fraud detection system.
Q3: Are there any ethical concerns with using AI in fraud detection?
A3: Yes, there are concerns regarding data privacy, transparency, and potential biases in AI algorithms, which necessitate careful regulatory compliance and ethical considerations.
Q4: What is predictive analytics in fraud detection?
A4: Predictive analytics uses historical data to identify trends and anticipate fraudulent behavior before it occurs, allowing organizations to take preventive measures.
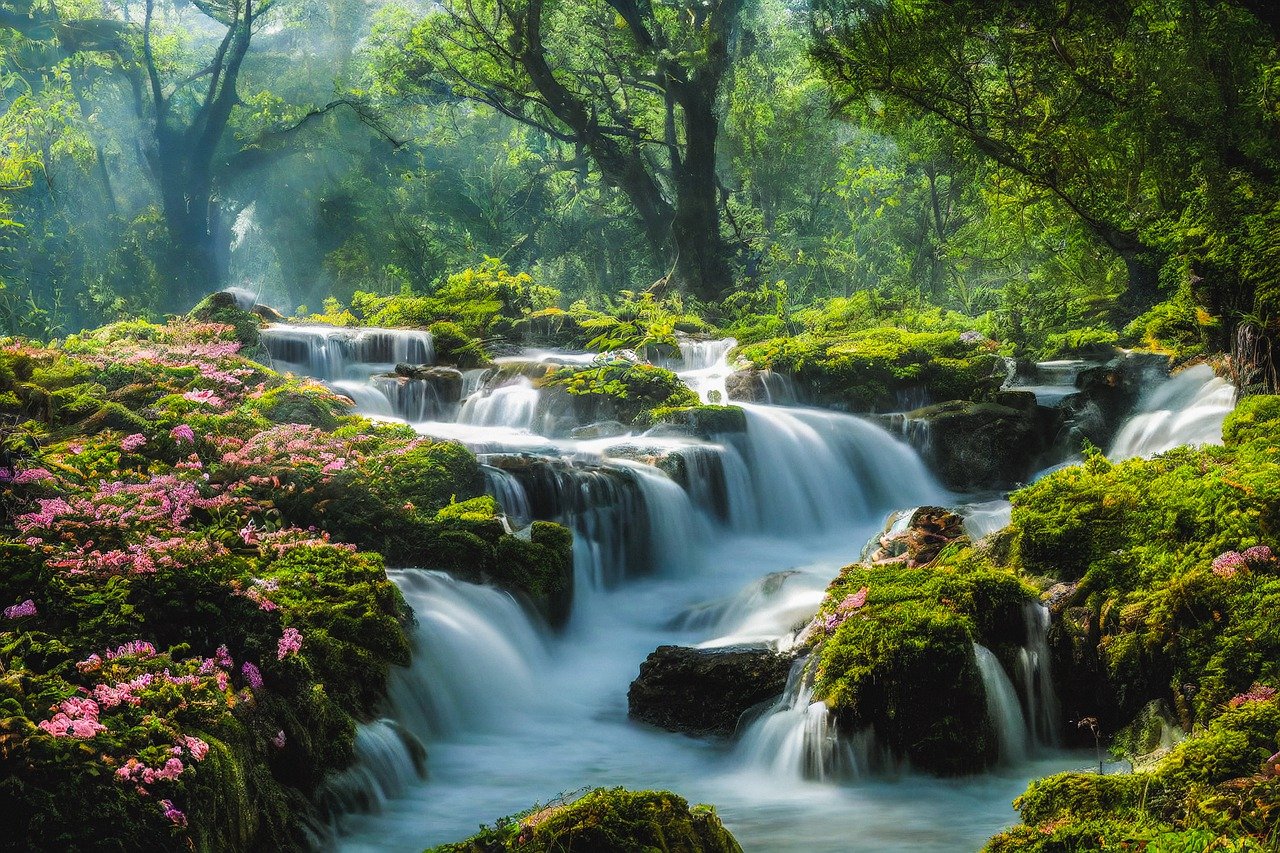
Collaboration with Human Expertise
In the ever-evolving landscape of fraud detection, the synergy between artificial intelligence and human expertise is becoming increasingly vital. While AI brings impressive capabilities to the table, such as speed and the ability to analyze vast amounts of data, it is the nuanced understanding of human analysts that ultimately enhances the effectiveness of fraud detection systems. Imagine AI as a powerful engine, capable of processing information at lightning speed, but it still requires a skilled driver—human expertise—to navigate the complex terrain of fraud detection.
One of the most significant advantages of this collaboration is the ability to interpret the context behind the data. AI can identify patterns and anomalies, but it often lacks the ability to understand the nuances of human behavior. For instance, a sudden spike in transactions from a particular account might raise a red flag. However, a human analyst can delve deeper into the situation, uncovering legitimate reasons behind the activity, such as a customer making a large purchase for a special occasion. This human touch can prevent unnecessary alarm and enhance customer satisfaction.
Moreover, the integration of human insights into AI systems can significantly improve the training of machine learning algorithms. By feeding AI systems with contextual knowledge and real-world experiences, human experts can help refine the algorithms, making them more effective in detecting fraudulent activities. For example, when analysts identify new fraud tactics, they can provide feedback to the AI systems, which can then adapt and evolve, learning from these insights to better anticipate future threats.
Furthermore, the collaborative approach fosters a culture of continuous improvement. Human experts can regularly assess the AI's performance, identifying areas where it excels and where it may fall short. This ongoing evaluation not only enhances the AI's accuracy but also ensures that the fraud detection system remains agile and responsive to emerging threats. In this dynamic environment, it’s crucial for organizations to embrace a hybrid model that leverages both AI's computational prowess and human intuition.
To illustrate the impact of this collaboration, consider the following table that outlines the key benefits of integrating human expertise with AI in fraud detection:
Benefit | Description |
---|---|
Contextual Understanding | Human analysts provide insights into the behavioral patterns behind data anomalies. |
Algorithm Training | Experts help refine AI models by sharing real-world experiences and feedback. |
Continuous Improvement | Regular assessments by humans ensure AI systems adapt to new fraud tactics. |
Enhanced Decision-Making | Combining AI speed with human judgment leads to better fraud detection outcomes. |
In conclusion, the future of fraud detection lies in the harmonious collaboration between AI and human expertise. By harnessing the strengths of both, organizations can create robust fraud detection systems that not only identify threats with remarkable speed but also understand the context behind them. This partnership not only enhances the effectiveness of fraud prevention efforts but also builds trust with customers, ensuring a safer and more secure financial environment.
- How does AI improve fraud detection? AI improves fraud detection by analyzing large datasets quickly, identifying patterns, and flagging anomalies that may indicate fraudulent activity.
- Why is human expertise important in AI fraud detection? Human expertise is crucial because it provides context and understanding of complex situations that AI may not fully grasp, ensuring more accurate decision-making.
- What are the challenges of integrating AI with human expertise? Challenges include ensuring effective communication between AI systems and human analysts, as well as maintaining a balance between automated processes and human oversight.
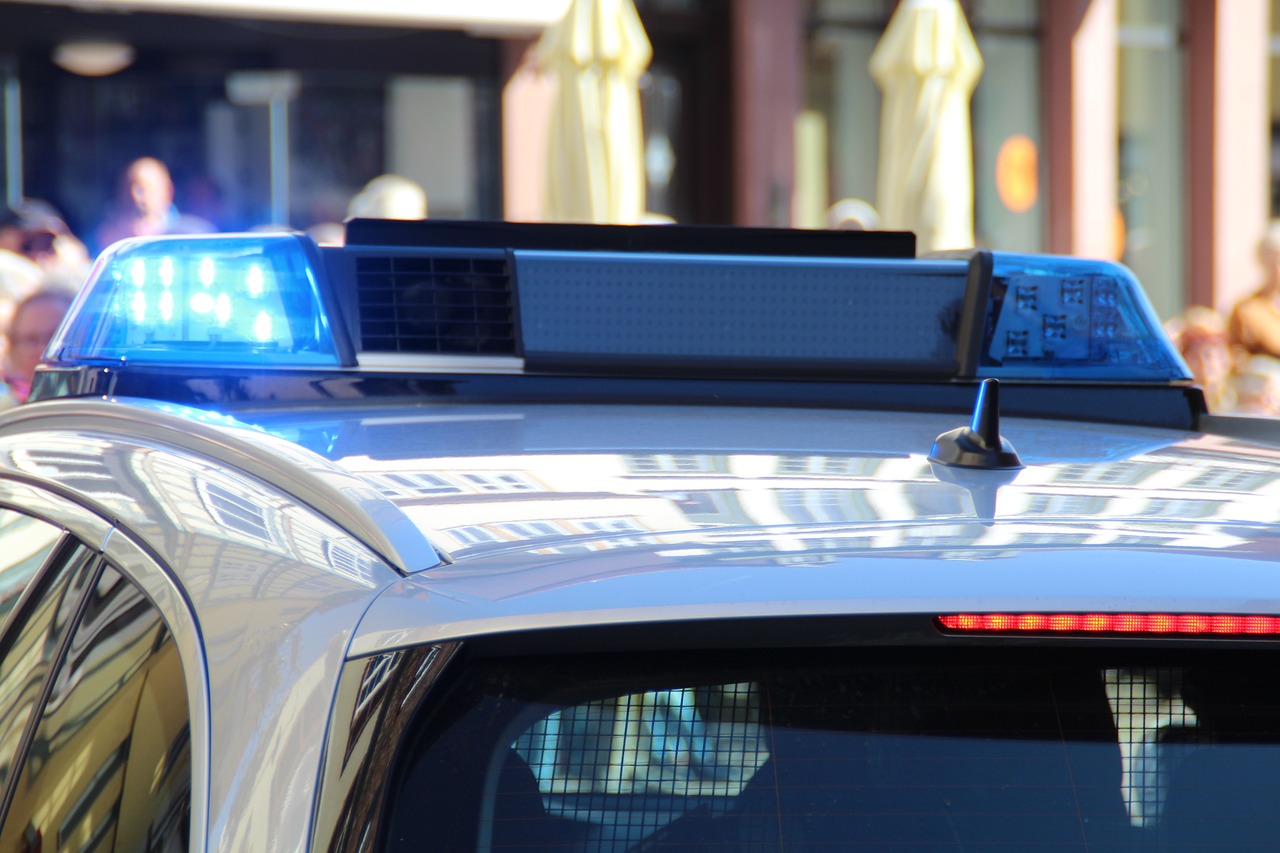
Regulatory Considerations
As the landscape of fraud detection evolves with the integration of artificial intelligence, regulatory considerations become increasingly critical. Organizations must navigate a complex web of regulations that govern the use of AI technologies. These regulations aim to protect consumer data, ensure fairness in algorithms, and maintain transparency in AI operations. The challenge lies in balancing innovation with compliance, as businesses strive to implement cutting-edge solutions while adhering to legal standards.
One of the primary regulatory concerns is data privacy. With AI systems processing vast amounts of sensitive information, organizations must comply with regulations such as the General Data Protection Regulation (GDPR) in Europe and the California Consumer Privacy Act (CCPA) in the United States. These laws mandate that companies obtain explicit consent from users before collecting their data and provide mechanisms for users to access, modify, or delete their information.
Furthermore, the need for algorithmic accountability is gaining traction. As AI systems become more autonomous, regulators are scrutinizing how decisions are made. Companies must ensure that their algorithms do not perpetuate bias or discrimination, which can lead to unfair treatment of certain groups. This is particularly relevant in fraud detection, where biased algorithms could unfairly target specific demographics, resulting in reputational damage and legal repercussions.
To address these concerns, organizations are encouraged to adopt a transparent approach to AI deployment. This involves documenting the decision-making processes of AI systems and providing stakeholders with insights into how these systems function. By fostering transparency, companies can build trust with consumers and regulators alike, ensuring that their AI applications are seen as ethical and responsible.
Moreover, collaboration with regulatory bodies is essential for developing industry standards. Engaging in dialogue with regulators allows organizations to stay ahead of compliance requirements and contribute to shaping the future of AI regulations. This proactive approach not only mitigates risks but also positions companies as leaders in ethical AI practices.
In summary, as organizations leverage AI for fraud detection, they must remain vigilant about regulatory considerations. By prioritizing data privacy, algorithmic accountability, transparency, and collaboration with regulators, businesses can navigate the complexities of compliance while harnessing the full potential of AI technologies.
- What are the main regulatory challenges in AI fraud detection? Organizations face challenges such as data privacy concerns, algorithmic bias, and the need for transparency in their AI systems.
- How can companies ensure compliance with data privacy regulations? Companies should implement robust data management practices, obtain user consent, and provide options for users to control their data.
- Why is algorithmic accountability important in fraud detection? Ensuring that AI algorithms are fair and unbiased is crucial to prevent discrimination and maintain consumer trust.
- What role do regulators play in the AI landscape? Regulators establish guidelines and standards that help ensure ethical use of AI technologies while protecting consumers' rights.
Frequently Asked Questions
- What is AI in fraud detection?
AI in fraud detection refers to the use of artificial intelligence technologies to identify and prevent fraudulent activities. By analyzing vast amounts of data, AI can detect patterns and anomalies that may indicate fraud, allowing organizations to respond quickly and effectively.
- How does AI improve fraud prevention?
AI improves fraud prevention by enhancing accuracy and efficiency. It can process large volumes of transactions in real-time, identifying suspicious activities that human analysts might miss. This capability not only reduces false positives but also speeds up the response to potential threats.
- What are machine learning algorithms?
Machine learning algorithms are a subset of AI that allows systems to learn from data and improve over time. In fraud detection, these algorithms analyze historical transaction data to recognize patterns and predict future fraudulent behavior, continuously refining their accuracy as they receive more data.
- What role does predictive analytics play?
Predictive analytics uses historical data and statistical algorithms to forecast future outcomes. In the context of fraud detection, it helps organizations anticipate fraudulent activities before they happen, enabling proactive measures to mitigate risks and prevent losses.
- What challenges do organizations face when implementing AI?
Organizations may encounter several challenges when implementing AI in fraud detection, including data privacy concerns, integration with existing systems, and the need for skilled personnel to manage and interpret AI outputs. Addressing these challenges is crucial for successful deployment.
- What are the future trends in AI for fraud detection?
Future trends in AI for fraud detection include advancements in deep learning technologies, increased collaboration between AI systems and human analysts, and a growing focus on ethical considerations and regulatory compliance as organizations seek to leverage AI responsibly.
- How important is collaboration with human expertise?
Collaboration with human expertise is vital in enhancing the effectiveness of AI in fraud detection. While AI can process data and identify patterns, human analysts bring contextual understanding and critical thinking skills that are essential for making informed decisions based on AI findings.
- What are the regulatory considerations for AI in fraud detection?
As AI technologies evolve, so do the regulatory frameworks governing their use. Organizations must stay informed about compliance requirements and ethical standards to ensure that their AI applications in fraud detection are both effective and responsible.