The Role of Data Science in Climate Modeling
Data science is not just a buzzword; it's a game-changer in the realm of climate modeling. Imagine trying to predict the weather without any data—it's like trying to navigate a ship through a stormy sea without a compass. With the increasing urgency of climate change, the integration of data science techniques into climate modeling has become critical. These techniques are revolutionizing how we understand and predict the Earth's climate, enabling scientists to develop effective strategies for mitigating the impacts of climate change.
At its core, climate modeling involves simulating the Earth's climate system to forecast future conditions. Traditional models relied heavily on mathematical equations and assumptions, but now, with the advent of data science, we have the ability to analyze vast datasets and uncover patterns that were previously hidden. This transformation allows for more accurate predictions and a deeper understanding of climate dynamics. The marriage of data science and climate science is akin to adding a powerful engine to a ship—it not only enhances speed but also improves navigation, making it easier to reach our destination.
As we delve deeper into this topic, we will explore how data collection techniques, machine learning algorithms, and advanced data processing methods are reshaping climate modeling. From satellite observations to ground-based sensors, the data we collect is becoming more precise and abundant. This wealth of information is crucial for building reliable models that can predict future climate scenarios with greater accuracy.
In the following sections, we will break down the components of climate models, the significance of accurate data collection, and how machine learning is enhancing our predictive capabilities. Each of these elements plays a vital role in our understanding of climate change and our ability to respond effectively. So, buckle up as we embark on this enlightening journey through the fascinating world of data science and climate modeling!
Climate models are the backbone of climate science, acting as sophisticated tools that simulate the interactions within the Earth's climate system. By utilizing various types of models, scientists can predict how different factors—such as greenhouse gas emissions, solar radiation, and volcanic activity—affect global temperatures and weather patterns. There are several types of climate models, including:
- Energy Balance Models (EBMs): Simple models that calculate energy inputs and outputs to predict temperature changes.
- General Circulation Models (GCMs): Complex models that simulate atmospheric and oceanic circulation, providing detailed climate projections.
- Earth System Models (ESMs): Comprehensive models that incorporate biological and chemical processes alongside physical interactions.
Each type of model has its strengths and weaknesses, but collectively, they provide invaluable insights into climate dynamics. By understanding how these models work, we can better appreciate the importance of data science in refining their accuracy and reliability.
Accurate data is the lifeblood of effective climate modeling. Without it, predictions would be little more than educated guesses. Various data collection methods play a crucial role in climate science, including:
- Satellite Observations: Satellites provide a bird's-eye view of the Earth, capturing data on temperature, humidity, and atmospheric composition.
- Ground-Based Sensors: These sensors collect localized data, allowing for a more granular understanding of climate variations.
Both satellite observations and ground-based data are essential for validating and improving climate models. The interplay between these data sources ensures that we have a comprehensive view of our planet's climate system, paving the way for more accurate predictions and effective climate strategies.
Remote sensing technology is a cornerstone of modern climate science. By utilizing satellite imagery, scientists can gather vast amounts of data from the Earth's surface, enabling them to monitor changes in land use, vegetation, and sea levels. This information is critical for understanding how human activities and natural processes impact our climate.
Recent advancements in satellite technology have significantly improved the accuracy and frequency of data collection. Newer satellites can capture high-resolution images and provide near-real-time data, allowing scientists to track climate changes as they happen. This enhanced capability not only improves our understanding of climate patterns but also aids in disaster preparedness and response.
While satellites offer a broad perspective, ground-based observation networks provide critical data that helps validate satellite findings. These networks consist of weather stations and monitoring sites that collect data on temperature, precipitation, and other climatic factors. The collaboration between ground-based and satellite data is essential for improving the accuracy of climate models.
Once data is collected, it must be processed and analyzed to extract meaningful insights. Data processing techniques, such as data cleaning and normalization, are essential for transforming raw data into a usable format. Advanced analytical methods, including statistical analysis and visualization tools, help scientists interpret complex datasets and identify trends and anomalies.
Machine learning is a powerful tool that is increasingly being integrated into climate modeling. By leveraging algorithms that can learn from data, scientists can improve predictions and identify complex patterns in climate data that traditional methods might overlook. This integration of machine learning enhances our ability to forecast climate changes and adapt to their impacts.
Predictive modeling using machine learning helps forecast climate changes by analyzing historical data and identifying trends. Specific algorithms, such as regression analysis and neural networks, are applied to make predictions about future climate scenarios. This approach not only improves accuracy but also allows for more nuanced predictions that account for various influencing factors.
Quantifying uncertainty is vital for reliable climate projections. Data science techniques assist in assessing and managing uncertainties in climate models, providing a clearer picture of potential outcomes. By understanding the range of possible scenarios, policymakers can make more informed decisions regarding climate action and adaptation strategies.
Q: How does data science improve climate modeling?
A: Data science enhances climate modeling by providing advanced analytical tools and techniques that help process vast amounts of data, improving prediction accuracy and identifying complex patterns.
Q: What types of data are used in climate modeling?
A: Climate modeling utilizes data from various sources, including satellite observations, ground-based sensors, and historical climate records.
Q: Why is uncertainty quantification important in climate science?
A: Uncertainty quantification is essential because it helps scientists understand the range of possible climate outcomes, allowing for better decision-making and planning in response to climate change.
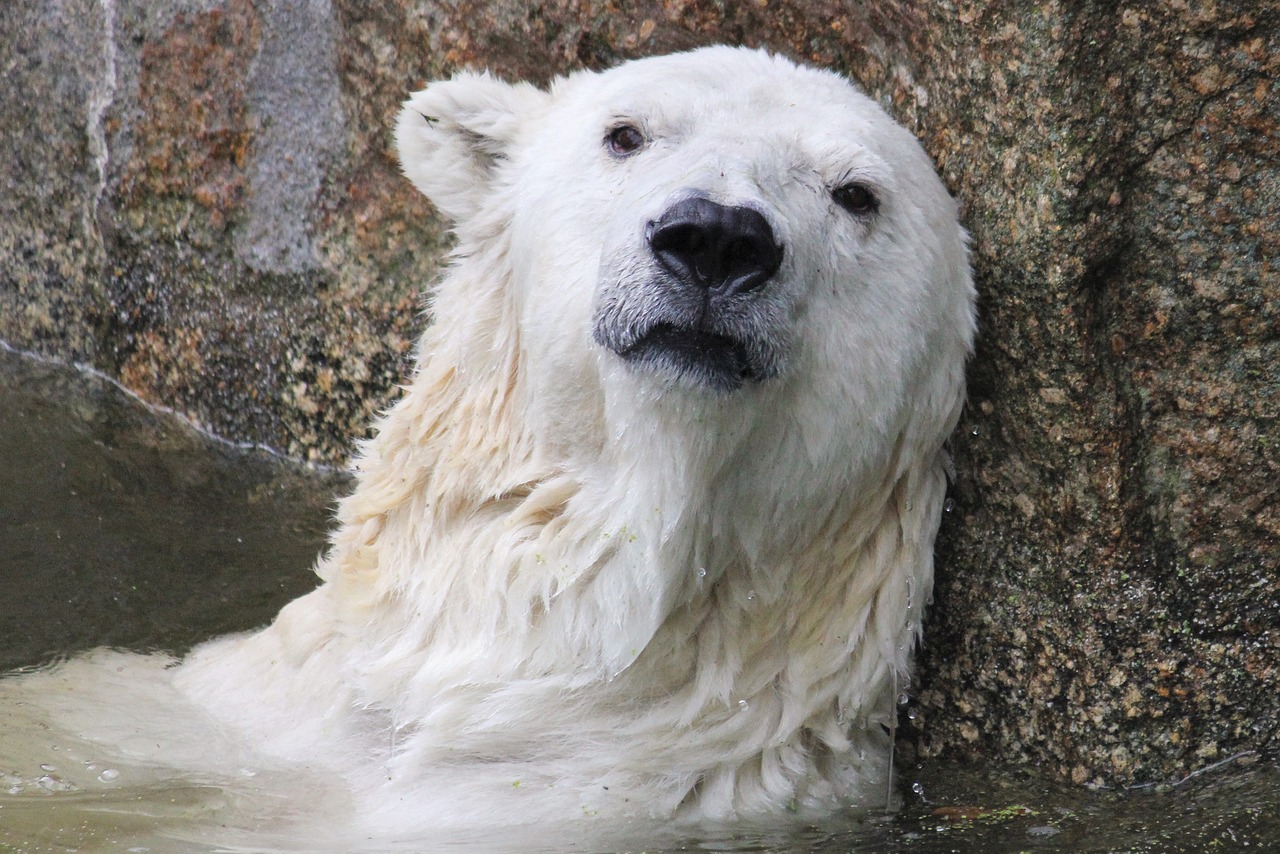
Understanding Climate Models
Climate models are like the crystal balls of the scientific world—they help us peek into the future of our planet's climate. By simulating the Earth's climate system, these models allow scientists to predict future climate conditions based on various scenarios. Imagine trying to forecast the weather without any data; it would be nearly impossible! That's why climate models are crucial for understanding the intricate dynamics that govern our atmosphere, oceans, and land surfaces.
There are several types of climate models, each serving a specific purpose and offering unique insights. The most common types include:
- Energy Balance Models (EBMs): These are the simplest models that focus on the balance between incoming solar energy and outgoing heat from the Earth. They provide a broad overview of climate changes.
- Atmospheric General Circulation Models (AGCMs): These models simulate the atmospheric processes and are more complex, incorporating factors like wind patterns and temperature variations.
- Coupled Models: These sophisticated models link the atmosphere, oceans, and land surfaces, allowing for a more comprehensive understanding of climate interactions.
The significance of these models cannot be overstated. They help scientists comprehend how different factors, such as greenhouse gas emissions or volcanic eruptions, influence climate dynamics. For instance, by using coupled models, researchers can predict how rising sea levels might impact coastal communities or how changes in temperature could affect global ecosystems.
Moreover, climate models are essential for developing effective climate change mitigation strategies. They provide policymakers with the necessary data to make informed decisions about reducing emissions, investing in renewable energy, and preparing for climate-related disasters. Without these models, we would be navigating the complexities of climate change blindfolded.
In conclusion, understanding climate models is key to grasping the challenges posed by climate change. They are not just tools for prediction; they are vital for crafting a sustainable future. As we continue to refine these models with advanced data science techniques, our ability to foresee and respond to climate-related issues will only improve, paving the way for a more resilient planet.
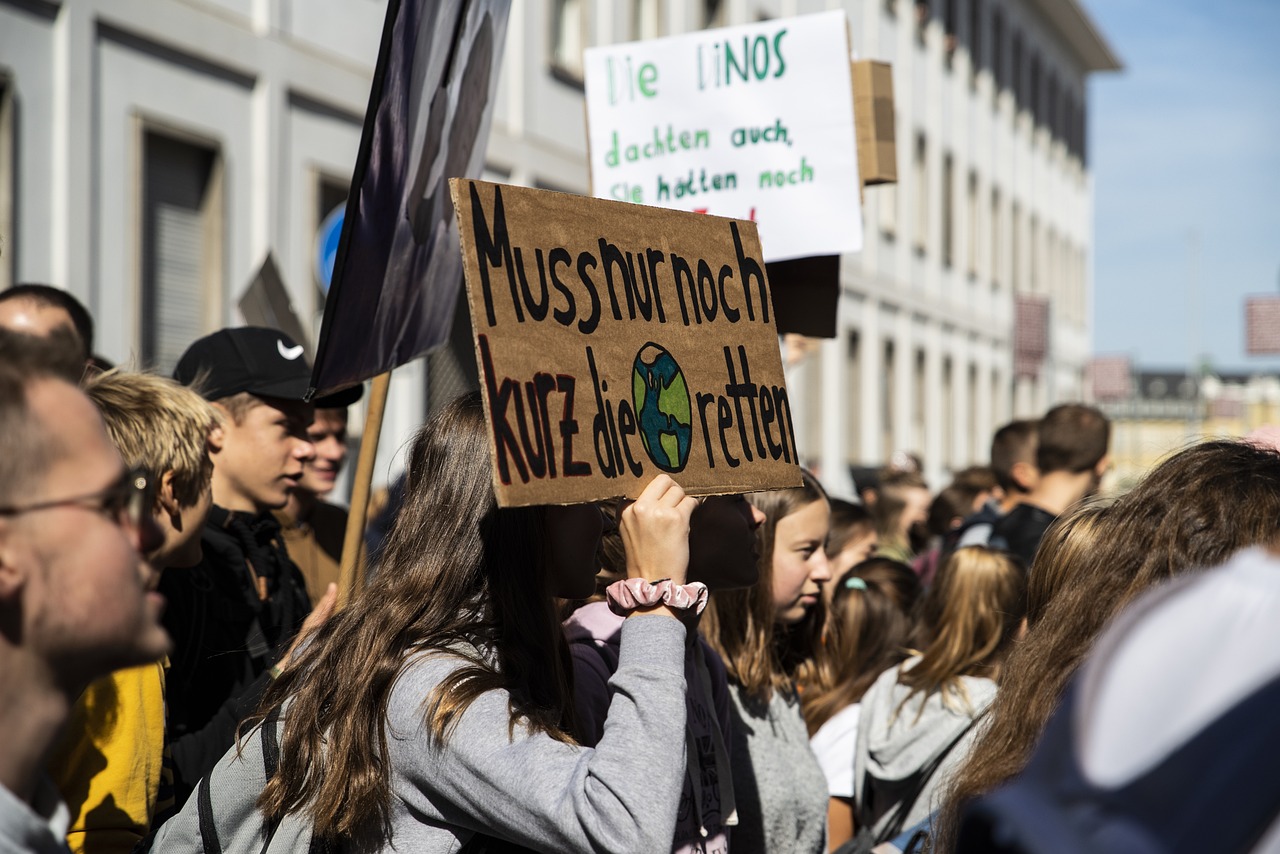
Data Collection Techniques
When it comes to understanding our planet's climate, accurate data is like the compass guiding us through a dense fog. Without it, our predictions and strategies would be akin to navigating without a map. are the backbone of climate science, enabling researchers to gather vital information about atmospheric conditions, temperature fluctuations, and other environmental factors. These techniques can be broadly categorized into two main types: remote sensing and ground-based observations.
Remote sensing involves the use of satellite technology to gather data from above. Imagine a bird's-eye view of the Earth that captures everything from cloud patterns to land surface temperatures. This method allows scientists to collect vast amounts of data over large areas, which is essential for understanding global climate trends. For instance, satellite imagery can reveal changes in ice cover, deforestation, and even urban heat islands. The data collected from these satellites is not just numbers; they tell a story about our planet's health and the impact of human activities.
Remote sensing technology has revolutionized how we monitor the Earth's climate. By utilizing satellites equipped with advanced sensors, scientists can capture detailed images and data across different wavelengths. This technology allows for the collection of information such as:
- Temperature measurements: Satellites can measure the temperature of the Earth's surface, providing critical data for climate models.
- Atmospheric composition: Remote sensing can track greenhouse gases like carbon dioxide and methane, which are crucial for understanding climate change.
- Land use changes: By monitoring land cover changes over time, scientists can assess the impact of human activities on climate.
Recent advancements in satellite technology have significantly enhanced our ability to collect and analyze climate data. For example, new satellites are equipped with higher resolution sensors that can capture images with unprecedented detail. This improvement allows for more accurate monitoring of changes in the Earth's climate. Additionally, the frequency of data collection has increased, enabling near real-time monitoring of environmental changes. With these advancements, scientists can detect and respond to climate changes more swiftly than ever before.
While remote sensing provides a macro view of the Earth’s climate, ground-based observations offer a micro perspective that is equally important. These observations involve a network of sensors and monitoring stations strategically placed around the globe to collect data on various climate parameters. Ground-based observations are essential for validating satellite data and ensuring its accuracy. For instance, weather stations record temperature, humidity, and precipitation levels, providing essential data that can help refine climate models. The integration of both remote sensing and ground-based data creates a comprehensive picture of climate dynamics.
In conclusion, the synergy between remote sensing and ground-based observations forms a robust framework for climate data collection. Accurate data is the lifeblood of climate modeling, enabling scientists to make informed predictions about future climate scenarios. As technology continues to advance, the potential for more precise and comprehensive data collection will only grow, paving the way for better climate change mitigation strategies.
Q: What is remote sensing in climate science?
A: Remote sensing involves collecting data about the Earth's climate from satellites, allowing scientists to monitor large areas and gather information on various climate parameters.
Q: How do ground-based observations complement satellite data?
A: Ground-based observations provide detailed, localized data that can validate and enhance the accuracy of satellite data, ensuring a more comprehensive understanding of climate dynamics.
Q: Why is accurate data collection important for climate modeling?
A: Accurate data is crucial for building reliable climate models, which help predict future climate conditions and inform effective climate change mitigation strategies.
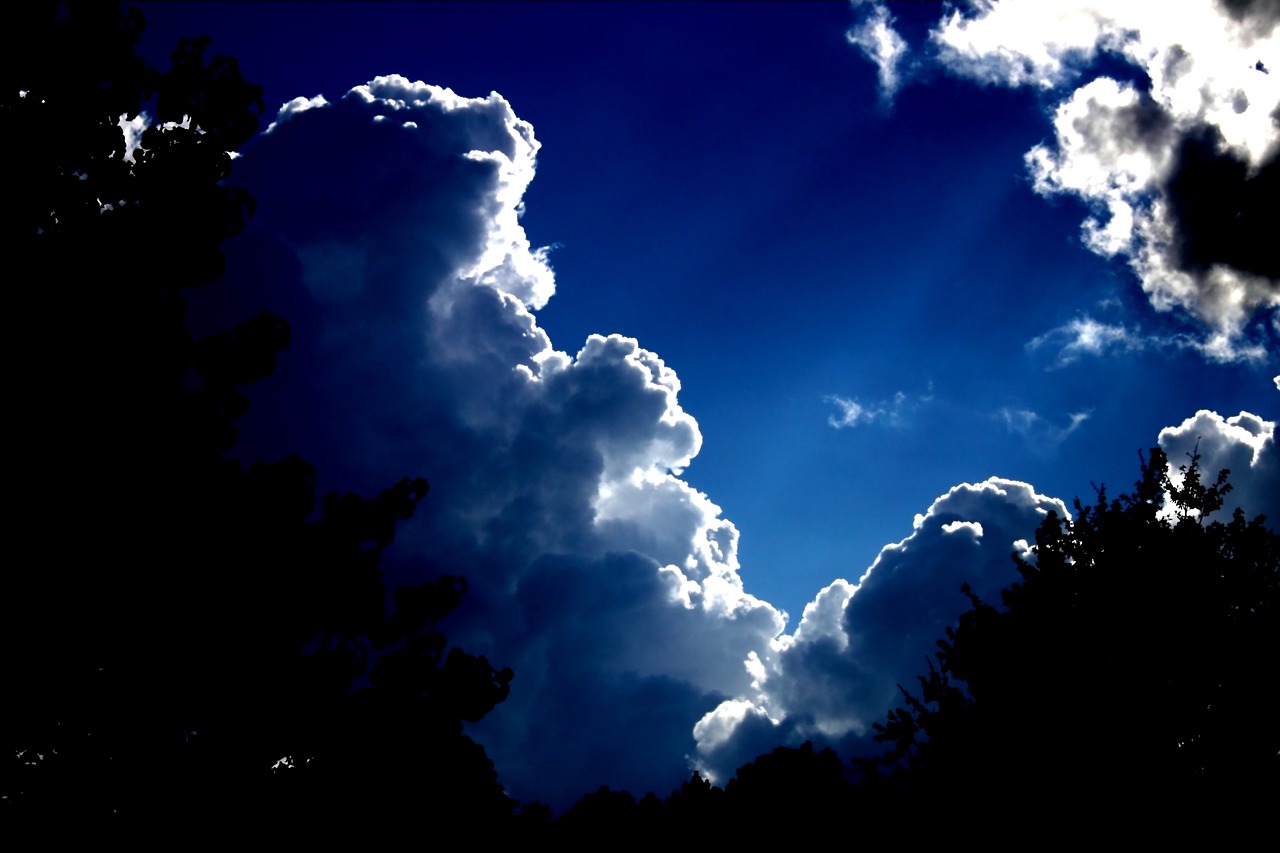
Remote Sensing
Remote sensing is a game changer in the realm of climate science, enabling researchers to gather extensive data about the Earth's surface without the need for physical presence. Imagine being able to observe the entire planet from space! This technology employs various sensors mounted on satellites and aircraft to capture detailed images and data across multiple wavelengths. The beauty of remote sensing lies in its ability to provide a comprehensive view of climate dynamics over vast areas and long periods, which is essential for understanding complex climate patterns.
One of the key advantages of remote sensing is its capacity to monitor changes in land use, vegetation cover, and surface temperatures. For instance, satellite imagery can reveal how urban expansion affects local climates or how deforestation contributes to global warming. By analyzing these images, scientists can track the health of ecosystems and assess the impact of climate change on biodiversity. The data collected through remote sensing serves as a crucial input for climate models, allowing for more accurate predictions and assessments of future scenarios.
Moreover, remote sensing technology has evolved significantly over the years. Recent advancements have led to the development of high-resolution sensors capable of capturing data with incredible precision. This has enhanced our ability to monitor atmospheric conditions, ocean temperatures, and even ice sheet dynamics. The table below illustrates some of the prominent satellite missions and their contributions to climate modeling:
Satellite Mission | Launch Year | Key Contributions |
---|---|---|
MODIS (Terra and Aqua) | 1999, 2002 | Monitoring vegetation, land surface temperature, and ocean color |
Sentinel-1 | 2014 | Radar imaging for land subsidence and deforestation |
Sentinel-2 | 2015 | High-resolution optical imagery for agriculture and forestry |
ICESat-2 | 2018 | Monitoring ice sheet elevation and sea level rise |
In conclusion, remote sensing is not just a technological marvel; it is a vital tool that enhances our understanding of climate change. By providing real-time data and long-term trends, remote sensing allows scientists to make informed decisions and craft effective climate policies. As we continue to grapple with the challenges posed by climate change, the insights gained from remote sensing will undoubtedly play a pivotal role in shaping our responses and strategies for mitigation.
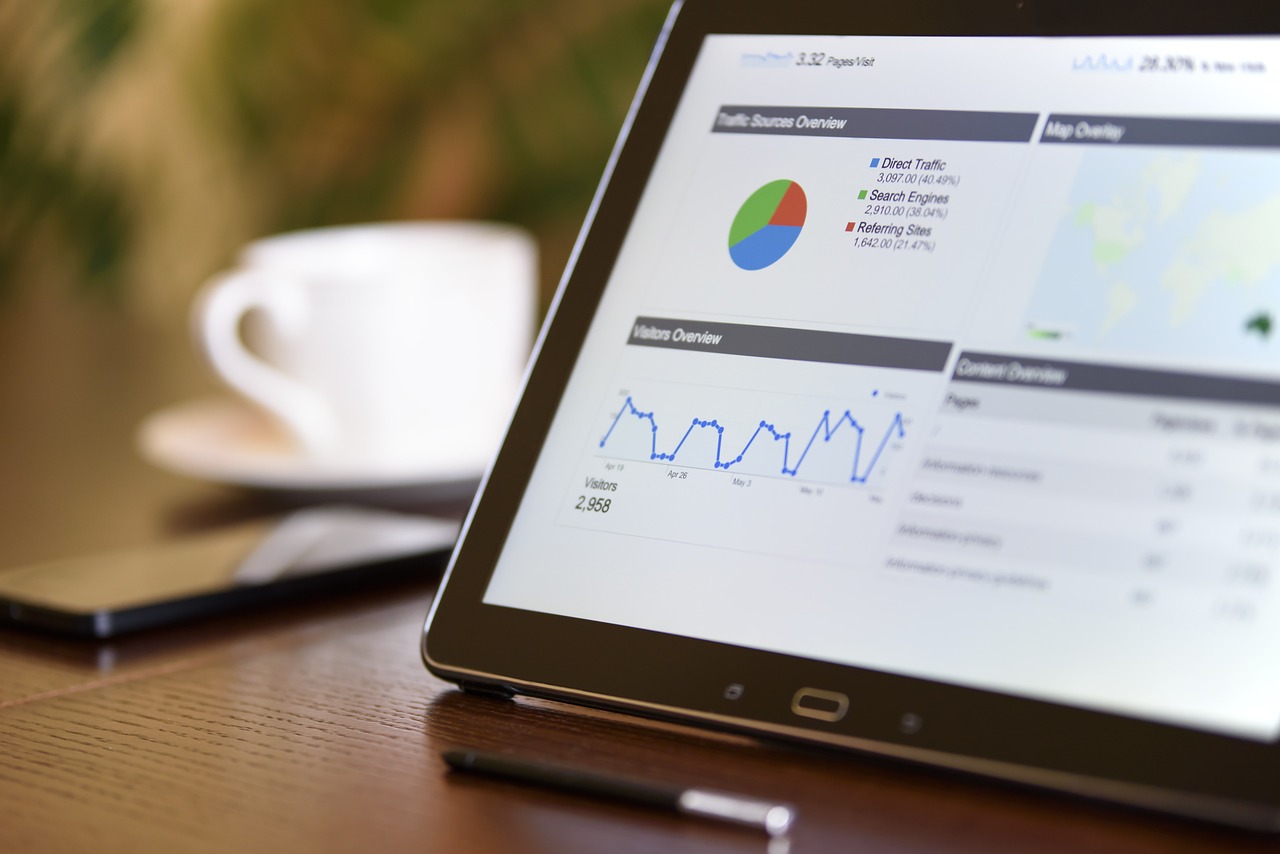
Satellite Technology Advancements
In recent years, the realm of satellite technology has witnessed remarkable advancements that have significantly enhanced our ability to monitor and understand the climate. These innovations not only improve the accuracy of the data we collect but also increase the frequency with which we can gather this information. Imagine a world where we can track changes in the Earth's climate in real-time; that world is becoming a reality thanks to cutting-edge satellite technology.
One of the most noteworthy advancements is the development of high-resolution imaging satellites. These satellites are equipped with sensors that can capture images with incredible detail, allowing scientists to observe changes in land use, vegetation cover, and even urban development. For instance, the Sentinel satellites from the European Space Agency provide data that is crucial for monitoring environmental changes. With their ability to capture images at various wavelengths, these satellites help us understand not just the surface changes but also the health of our ecosystems.
Moreover, the frequency of data collection has improved dramatically. Older satellites might only provide data every few days or weeks, but modern satellites can deliver updates multiple times a day. This is particularly important when tracking rapidly evolving phenomena such as hurricanes or wildfires. The ability to monitor these events in near real-time allows for quicker responses and better preparedness, ultimately saving lives and resources.
Another significant advancement is the integration of machine learning algorithms with satellite data. By utilizing machine learning, scientists can analyze vast amounts of data more efficiently than ever before. These algorithms can identify patterns and anomalies in climate data that might be missed by human analysts. For example, they can detect subtle changes in temperature or precipitation patterns over time, providing insights that are crucial for accurate climate modeling.
To illustrate the impact of these advancements, consider the following table that summarizes key improvements in satellite technology:
Advancement | Description | Impact on Climate Modeling |
---|---|---|
High-Resolution Imaging | Increased detail in capturing environmental changes | Better understanding of land use and ecosystem health |
Increased Data Frequency | Real-time monitoring capabilities | Enhanced response to natural disasters |
Machine Learning Integration | Efficient analysis of large datasets | Improved pattern recognition and anomaly detection |
As we continue to innovate and refine satellite technology, the potential for more accurate and timely climate modeling grows exponentially. The combination of high-resolution data, rapid collection frequencies, and advanced analytical techniques paves the way for a deeper understanding of our planet's climate system. This not only aids scientists in their research but also equips policymakers with the information needed to develop effective climate change mitigation strategies.
- What are climate models? Climate models are simulations that help scientists predict future climate conditions based on various data inputs.
- How do satellites contribute to climate science? Satellites provide critical data on temperature, precipitation, and land use changes, which are essential for accurate climate modeling.
- What is machine learning's role in climate modeling? Machine learning helps analyze large datasets and identify complex patterns, improving the accuracy of climate predictions.
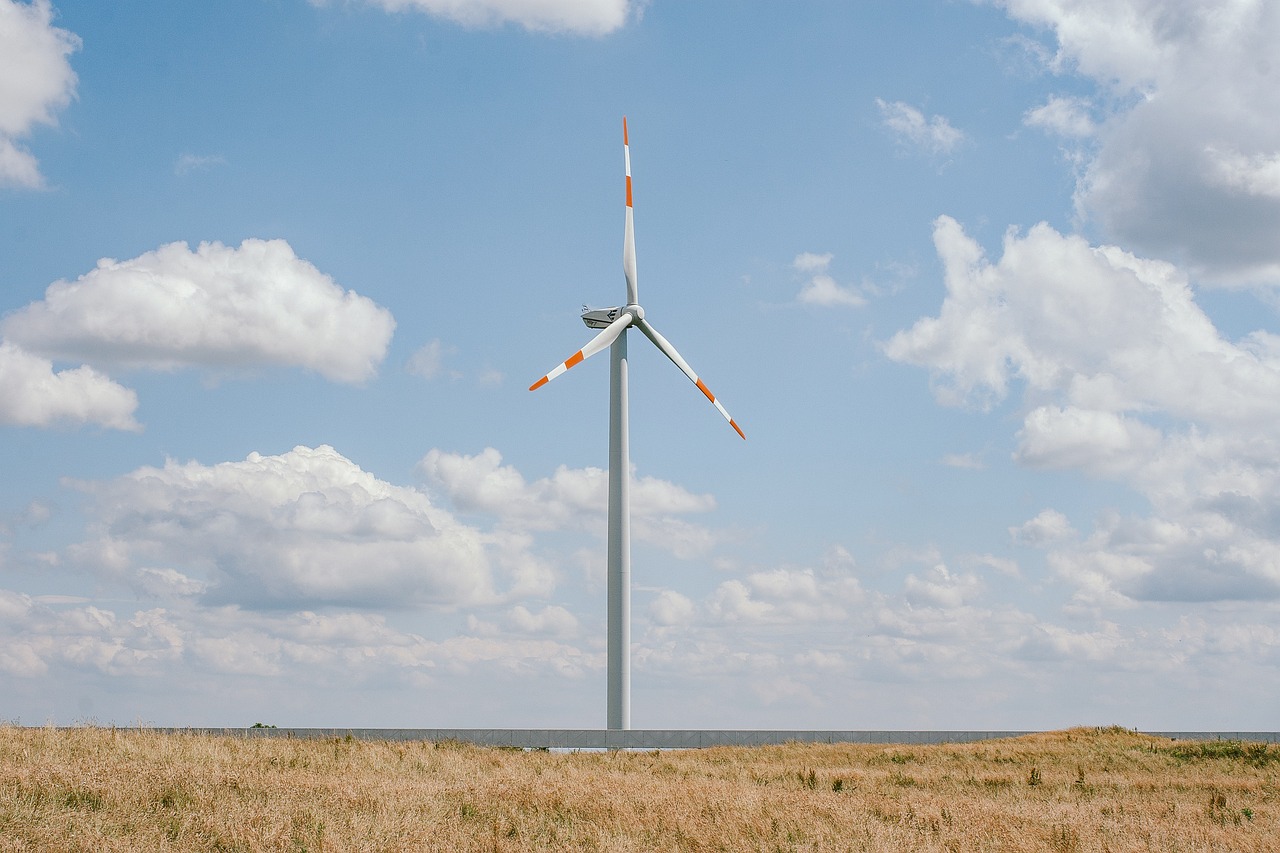
Ground-Based Observations
Ground-based observations are an indispensable part of climate science, providing crucial data that complements satellite measurements. While satellites can capture broad swathes of the Earth's surface, ground-based sensors offer localized insights that are vital for understanding microclimates and specific weather phenomena. Imagine trying to paint a detailed portrait of a landscape by only viewing it from the sky; you would miss the intricate details that ground-level perspectives reveal. This is where ground-based observations come into play, filling in the gaps and ensuring that our climate models are as accurate as possible.
One of the primary advantages of ground-based observations is their ability to validate and calibrate satellite data. Without these on-the-ground measurements, we would be left with a less accurate picture of climate dynamics. For instance, ground stations equipped with advanced sensors can measure temperature, humidity, wind speed, and other meteorological variables with high precision. This data is then used to adjust satellite readings, ensuring that the information we rely on is as close to reality as possible.
Furthermore, ground-based observations can capture phenomena that satellites may overlook. For example, the intricate interactions of urban heat islands, local vegetation, and topography can significantly influence climate patterns in specific areas. Ground stations can monitor these interactions in real-time, providing invaluable data that can be incorporated into climate models. The role of citizen science has also become increasingly important, with volunteers setting up weather stations in their backyards to contribute to a larger network of data collection. This grassroots approach not only enhances data availability but also fosters community engagement in climate science.
To illustrate the impact of ground-based observations, consider the following table that summarizes the key features and benefits:
Feature | Benefit |
---|---|
High Precision Measurements | Ensures accurate data for model calibration |
Localized Data Collection | Captures microclimate variations |
Validation of Satellite Data | Improves overall data reliability |
Community Engagement | Encourages public participation in climate monitoring |
In summary, ground-based observations are not just a supplementary tool; they are a vital component of climate science that enhances our understanding of the complex interactions within the Earth's climate system. By integrating these observations into climate models, scientists can create more robust predictions and develop effective strategies to mitigate the impacts of climate change. Without them, our understanding of climate dynamics would be significantly hindered, leaving us with a fragmented view of our planet's changing climate.
- What are ground-based observations? Ground-based observations refer to data collected from sensors and instruments located on the Earth's surface, providing localized insights into climate conditions.
- How do ground-based observations improve climate models? They validate and calibrate satellite data, capture localized phenomena, and provide high-precision measurements that enhance the accuracy of climate predictions.
- Can citizen scientists contribute to ground-based observations? Absolutely! Citizen scientists can set up weather stations and share their data, contributing to a larger network of climate monitoring efforts.
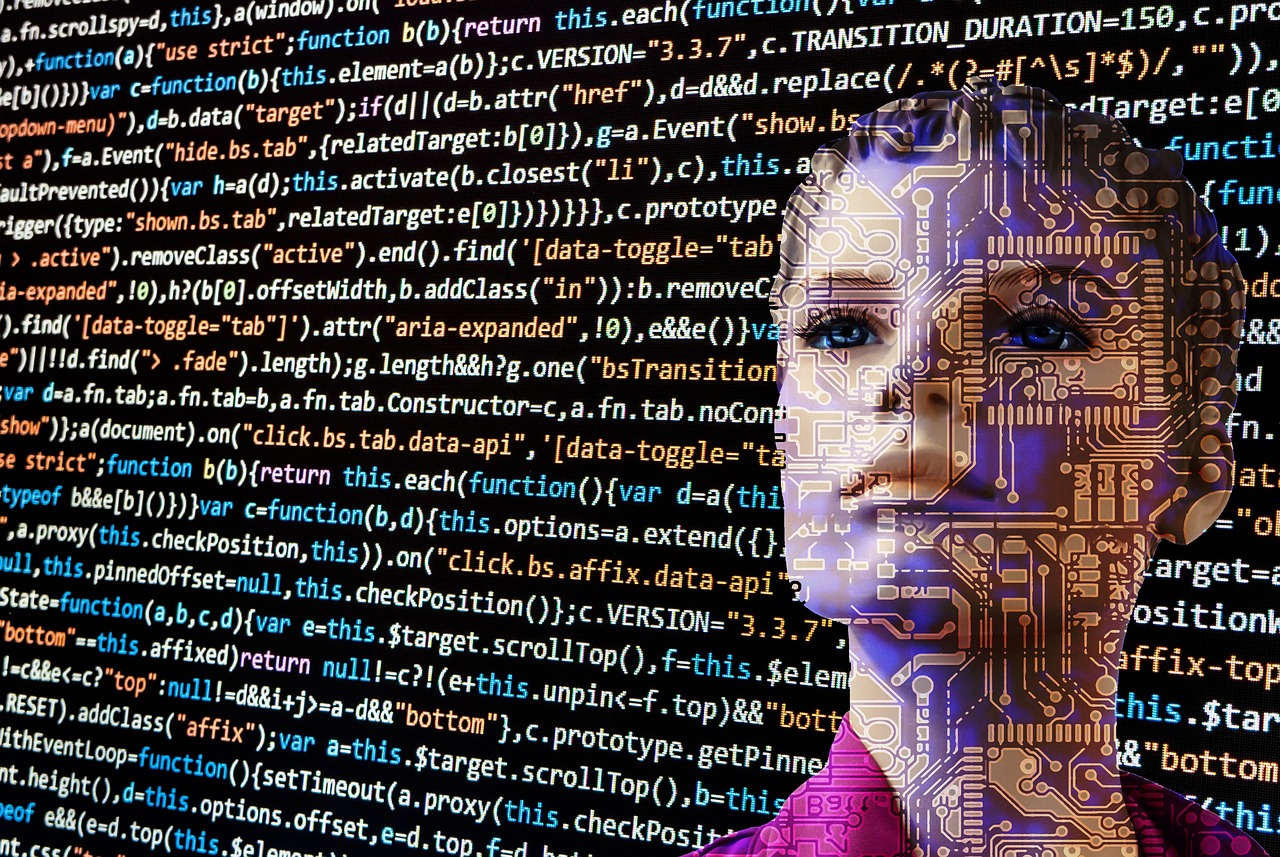
Data Processing and Analysis
Data processing and analysis are at the heart of climate science, transforming raw data into actionable insights that can guide policy decisions and climate action. Imagine trying to find a needle in a haystack; that’s what dealing with massive datasets can feel like. However, with the right techniques, scientists can sift through this information with precision, uncovering patterns and trends that are crucial for understanding our planet's changing climate.
One of the primary methods used in data processing involves statistical analysis. This technique allows researchers to summarize and interpret the vast amounts of data collected from various sources. By employing statistical models, scientists can identify correlations between different climate variables, such as temperature, precipitation, and greenhouse gas emissions. For instance, a study might reveal that increases in CO2 levels correlate with rising global temperatures, which is vital for predicting future climate scenarios.
Moreover, the advent of big data technologies has revolutionized how we handle climate data. Tools like Hadoop and Spark enable the processing of large datasets across distributed systems, making it faster and more efficient. This capability is particularly important when dealing with data from remote sensing satellites and ground-based sensors that generate terabytes of information daily. In fact, a recent analysis showed that using big data processing techniques reduced the time needed to process climate data by over 50%, allowing scientists to focus more on interpreting the results rather than managing the data.
Visualization is another critical aspect of data analysis. It’s one thing to have numbers and graphs; it’s another to see them in a comprehensible format. Advanced visualization tools help scientists and policymakers alike to grasp complex climate data easily. For instance, interactive maps can show temperature changes over time, making it easier to communicate findings to the public and stakeholders. These visual aids can be powerful in advocating for climate action, as they illustrate the urgency of the situation in a way that raw data cannot.
Additionally, data integration plays a crucial role in enhancing the reliability of climate models. By combining data from various sources—such as satellite imagery, weather stations, and ocean buoys—scientists can create a more comprehensive picture of the climate system. This multi-faceted approach helps in validating models and ensuring that predictions are based on robust data. For example, integrating ocean temperature data with atmospheric models can provide insights into phenomena like El Niño, which significantly impacts global weather patterns.
In conclusion, the processes of data processing and analysis are indispensable in climate science. They not only help in managing the overwhelming amounts of data but also enhance our understanding of climate dynamics. As technology continues to evolve, the tools and methods for data analysis will become even more sophisticated, paving the way for more accurate climate modeling and effective strategies for mitigating climate change.
- What is the importance of data processing in climate science? Data processing helps transform raw data into meaningful insights, allowing scientists to identify trends and make predictions about climate changes.
- How do big data technologies impact climate modeling? Big data technologies enable the efficient processing of large datasets, significantly reducing the time needed for data analysis and improving the accuracy of climate models.
- What role does visualization play in climate data analysis? Visualization helps communicate complex data in an understandable format, making it easier for policymakers and the public to grasp the urgency of climate issues.
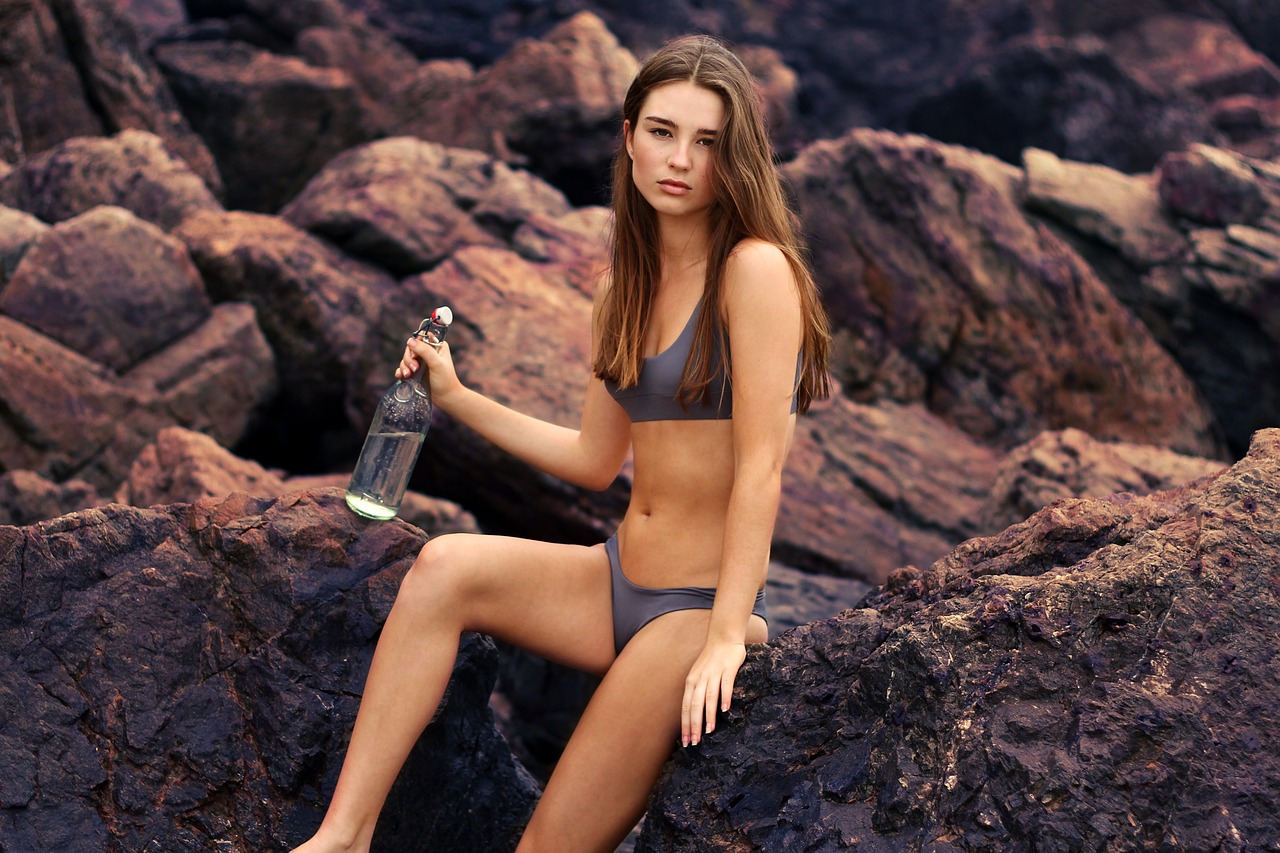
Machine Learning in Climate Science
As we dive deeper into the intersection of machine learning and climate science, it becomes increasingly clear that these advanced algorithms are not just tools; they are powerful allies in our quest to understand and mitigate the impacts of climate change. Imagine trying to predict the weather without any data—it's nearly impossible! But with machine learning, we can analyze vast datasets and uncover patterns that were previously hidden from our view.
Machine learning techniques can process and analyze data at a speed and scale that human analysts simply cannot match. For instance, algorithms can sift through historical climate data, satellite imagery, and even social media posts to predict future climate scenarios. This capability is crucial, especially when we consider the complexity of climate systems. By leveraging machine learning, scientists can enhance their predictive modeling, which is essential for developing effective climate policies.
One of the most exciting applications of machine learning in climate science is in predictive modeling. This involves using historical data to train algorithms that can forecast future climate conditions. For example, a model might analyze temperature and precipitation data over the past century to make predictions about next summer's weather. The algorithms can identify trends and correlations that might be too subtle for traditional statistical methods to detect. This leads to more accurate forecasts, which can inform everything from agricultural planning to disaster preparedness.
Moreover, machine learning excels in identifying complex patterns within climate data. For instance, researchers have used neural networks—an advanced type of machine learning model—to analyze how various factors like greenhouse gas emissions, solar radiation, and ocean currents interact to affect global temperatures. These insights can help scientists understand not just what changes are occurring, but why they are happening, allowing for more effective intervention strategies.
However, as with all powerful tools, machine learning comes with its challenges. One of the most critical issues in climate modeling is uncertainty quantification. Climate systems are inherently complex, and predicting their behavior involves a degree of uncertainty. Machine learning can assist in assessing and managing these uncertainties by providing probabilistic forecasts rather than deterministic ones. This means that instead of saying, "It will rain tomorrow," a machine-learning model might say, "There is a 70% chance of rain tomorrow." This nuanced approach helps policymakers make more informed decisions, as they can weigh the risks and probabilities associated with various climate scenarios.
In summary, the integration of machine learning into climate science is revolutionizing how we understand and respond to climate change. By enhancing predictive capabilities and uncovering complex relationships within data, machine learning is not just improving our forecasts but also empowering us to take meaningful action in the face of one of the greatest challenges of our time.
- What is machine learning? Machine learning is a branch of artificial intelligence that focuses on the development of algorithms that can learn from and make predictions based on data.
- How does machine learning improve climate modeling? By analyzing large datasets, machine learning can identify patterns and make more accurate predictions about future climate conditions.
- What are the challenges of using machine learning in climate science? The main challenges include uncertainty quantification and the complexity of climate systems, which can lead to difficulties in making precise predictions.
- Can machine learning help mitigate climate change? Yes, by improving our understanding of climate dynamics and enhancing predictive capabilities, machine learning can inform better climate policies and strategies.
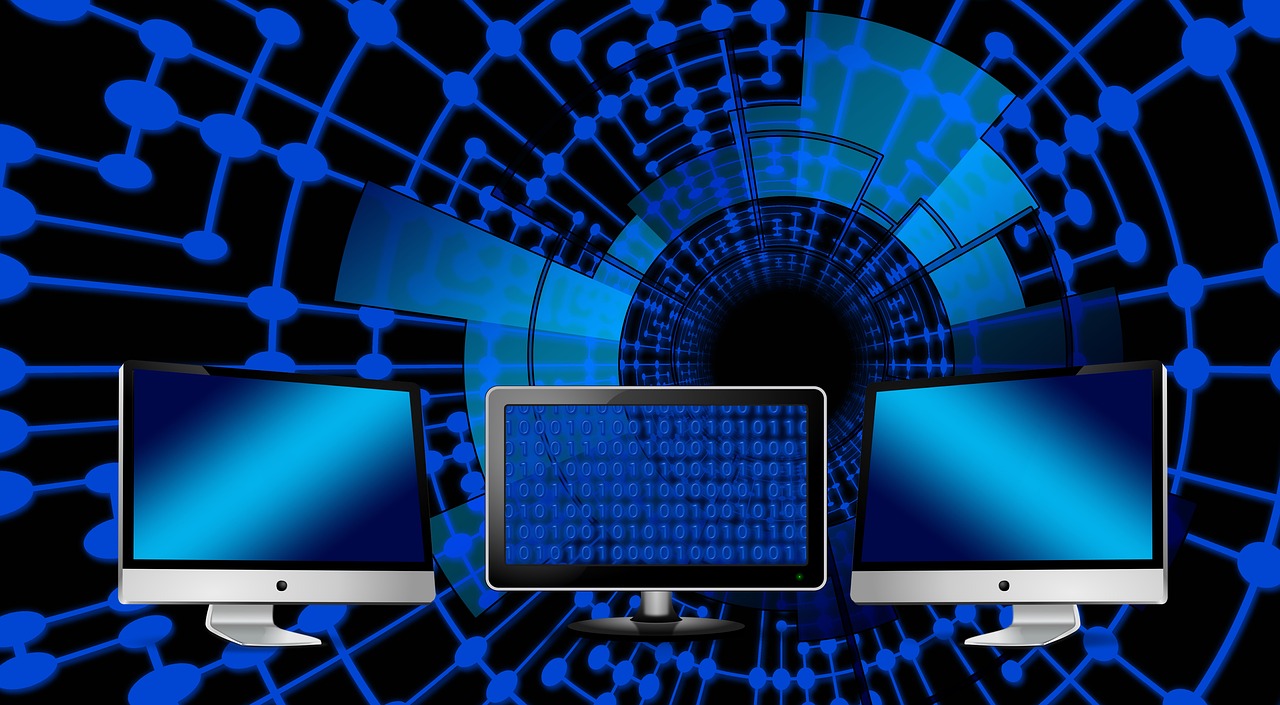
Predictive Modeling
Predictive modeling has emerged as a game-changer in the realm of climate science, allowing researchers to forecast climate changes with remarkable precision. At its core, predictive modeling uses historical data to identify patterns and trends, which then inform future projections. Think of it as a weather forecast that evolves from mere guesswork into a sophisticated analysis of data. By employing a variety of machine learning algorithms, scientists can sift through vast amounts of climate data, extracting meaningful insights that help us understand potential future scenarios.
One of the most common algorithms used in predictive modeling is the Random Forest, which operates by creating multiple decision trees and aggregating their outputs to enhance accuracy. This method is particularly effective for handling complex datasets with numerous variables, such as temperature, precipitation, and greenhouse gas concentrations. Another powerful tool is the Support Vector Machine (SVM), which excels in classification tasks, helping scientists categorize climate data into distinct patterns that can indicate shifts in climate behavior.
Moreover, predictive modeling is not just about crunching numbers; it’s about making informed decisions. For example, when predicting the likelihood of extreme weather events, predictive models can provide insights that are crucial for disaster preparedness. By analyzing past occurrences of hurricanes, droughts, and floods, these models can help communities strategize and implement effective mitigation measures. Imagine being able to predict a storm's path with high accuracy—this capability not only saves lives but also reduces economic losses.
In addition to traditional algorithms, deep learning techniques are gaining traction in climate modeling. These advanced neural networks can process unstructured data, such as satellite images, to identify subtle changes in climate patterns. By leveraging these technologies, scientists can enhance the granularity of their models, leading to more localized and accurate predictions. As we continue to refine these models, the potential for understanding and combating climate change becomes increasingly promising.
However, it's essential to recognize that predictive modeling comes with its own set of challenges. The accuracy of predictions is heavily reliant on the quality of data fed into the models. Inconsistent or incomplete data can lead to misleading forecasts, which is why ongoing efforts to improve data collection and processing are vital. Furthermore, the inherent uncertainty in climate systems means that while predictive models can provide valuable insights, they should be viewed as tools to guide decision-making rather than absolute certainties.
In summary, predictive modeling stands at the forefront of climate science, offering a pathway to understanding the complexities of our planet's changing climate. By harnessing the power of machine learning and advanced data analysis techniques, we can not only predict future climate scenarios but also empower communities to take proactive steps in addressing climate change. As we look to the future, the integration of these technologies will undoubtedly play a pivotal role in shaping effective climate policies and strategies.
- What is predictive modeling? Predictive modeling is a statistical technique that uses historical data to forecast future outcomes, particularly in climate science.
- How does machine learning improve predictive modeling? Machine learning algorithms can analyze large datasets, identify complex patterns, and enhance the accuracy of climate predictions.
- What are some common algorithms used in predictive modeling? Common algorithms include Random Forest, Support Vector Machine (SVM), and deep learning techniques.
- Why is data quality important in predictive modeling? The accuracy of predictions relies on the quality of data; inconsistent or incomplete data can lead to misleading forecasts.
- How can predictive modeling aid in climate change mitigation? By providing insights into potential future climate scenarios, predictive modeling helps communities prepare for and adapt to climate change impacts.
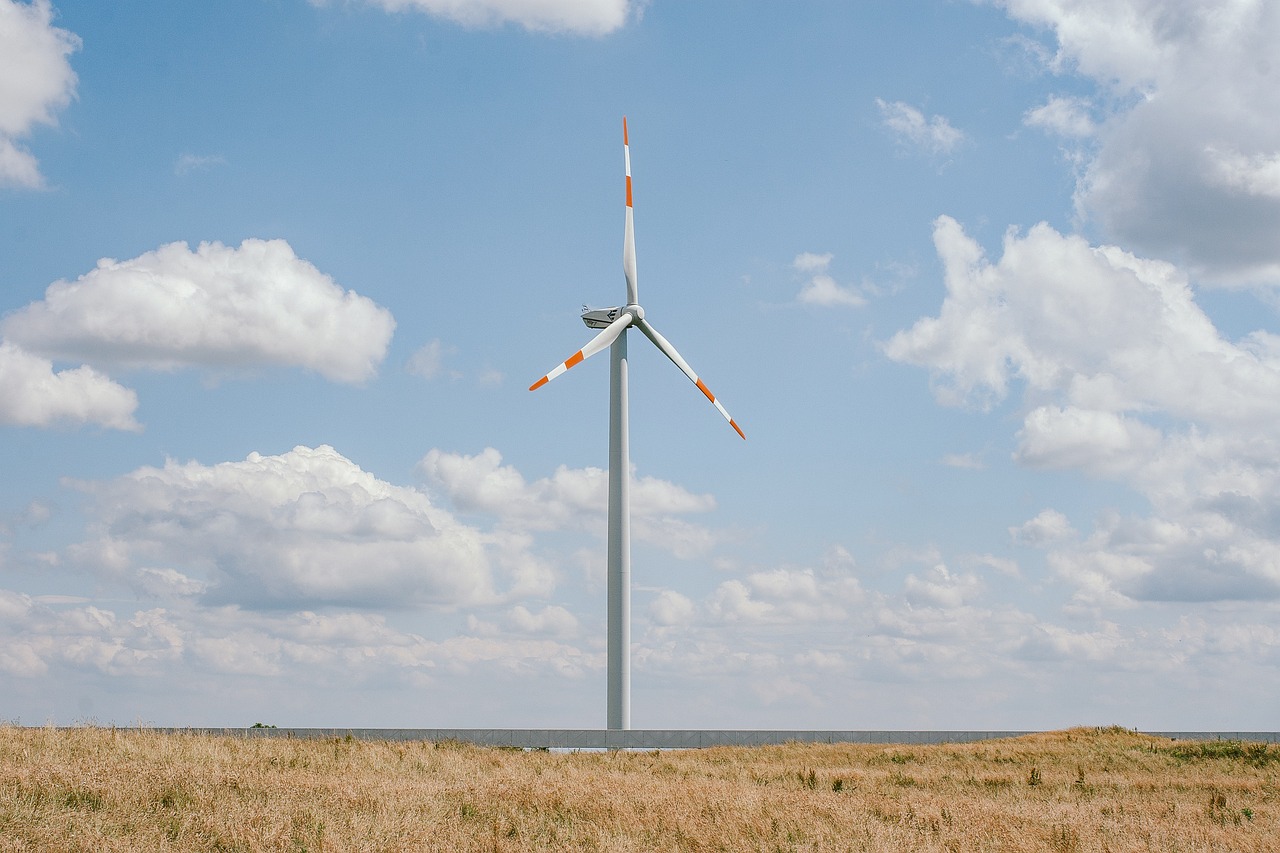
Uncertainty Quantification
In the realm of climate modeling, plays a pivotal role in ensuring that the predictions made by scientists are not only reliable but also actionable. Imagine you're trying to predict the weather for your weekend plans; the accuracy of that forecast can greatly influence whether you pack an umbrella or head out in shorts. Similarly, in climate science, understanding the uncertainties involved helps researchers and policymakers make informed decisions regarding climate change mitigation strategies.
Uncertainty can arise from various sources, including model parameters, input data, and the inherent variability of the climate system itself. For instance, when we consider the inputs to a climate model, such as greenhouse gas emissions or land use changes, each of these factors can have a range of possible values. This variability can lead to different outcomes in model predictions, which is why quantifying uncertainty is crucial.
Data scientists employ several techniques to assess and quantify these uncertainties. One common method is the use of Monte Carlo simulations, which involve running the climate model multiple times with varying inputs to create a distribution of possible outcomes. This approach allows scientists to visualize the range of potential future climates, much like how a weather forecast might provide a range of temperatures rather than a single value.
Another important aspect of uncertainty quantification is the development of confidence intervals. By calculating these intervals, researchers can express the degree of certainty associated with their predictions. For example, a model might predict that global temperatures will rise by 2°C, but with a confidence interval of ±0.5°C. This means that while a 2°C increase is likely, there is still a chance that the actual increase could be as low as 1.5°C or as high as 2.5°C.
Furthermore, uncertainty quantification is not just about understanding what we know; it also involves recognizing what we don’t know. This is where the concept of model validation comes into play. By comparing model predictions with real-world observations, scientists can identify discrepancies and refine their models accordingly. This iterative process of validation and adjustment helps to minimize uncertainties over time, leading to more robust climate projections.
In summary, uncertainty quantification is an essential component of climate modeling that helps bridge the gap between complex scientific data and practical decision-making. By employing sophisticated techniques to assess uncertainties, researchers can provide clearer insights into potential climate futures, ultimately guiding policies and actions aimed at combating climate change.
- What is uncertainty quantification in climate modeling?
Uncertainty quantification refers to the process of identifying and assessing the uncertainties present in climate model predictions, helping to improve the reliability of these forecasts. - Why is uncertainty important in climate predictions?
Understanding uncertainty helps scientists and policymakers make informed decisions regarding climate change mitigation and adaptation strategies. - How do scientists quantify uncertainty?
Scientists use methods such as Monte Carlo simulations and confidence intervals to assess and communicate the range of possible outcomes in climate predictions.
Frequently Asked Questions
- What are climate models and why are they important?
Climate models are sophisticated tools that simulate the Earth's climate system. They help scientists predict future climate conditions by analyzing various factors such as temperature, precipitation, and atmospheric pressure. Understanding these models is crucial because they enable us to grasp the dynamics of climate change and devise effective strategies for mitigation.
- How is data collected for climate modeling?
Data collection for climate modeling involves a mix of methods, including satellite observations and ground-based sensors. These techniques are essential for gathering accurate information about the Earth's climate. For instance, satellite technology allows for the collection of vast amounts of data from space, while ground-based observations help validate and enhance the accuracy of satellite data.
- What role does remote sensing play in climate science?
Remote sensing is a game-changer in climate science. It involves using satellite imagery to collect data about the Earth's surface without direct contact. This technology provides critical insights into climate patterns, allowing scientists to monitor changes over time and make informed predictions about future climate scenarios.
- How does machine learning enhance climate modeling?
Machine learning brings a new level of sophistication to climate modeling. By leveraging algorithms, it can analyze complex climate data, identify patterns, and improve predictive accuracy. This means that forecasts about climate changes become more reliable, helping policymakers and researchers make better-informed decisions.
- What is predictive modeling in the context of climate science?
Predictive modeling refers to the use of statistical techniques and machine learning algorithms to forecast future climate conditions. This approach allows scientists to anticipate changes based on historical data and current trends, offering a clearer picture of what the future may hold in terms of climate impacts.
- Why is uncertainty quantification important in climate projections?
Uncertainty quantification is vital because it addresses the inherent unpredictability in climate modeling. By assessing and managing these uncertainties, scientists can provide more robust climate projections, helping stakeholders understand the range of possible outcomes and prepare for various scenarios.