How AI Will Enhance Predictive Analytics in Business
The world of business is changing rapidly, and at the heart of this transformation is artificial intelligence (AI). As companies strive to stay ahead of the competition, they are increasingly turning to AI to enhance their predictive analytics capabilities. But what does this really mean? Well, imagine having a crystal ball that not only predicts the future but also learns from past data to improve its accuracy. This is the essence of AI in predictive analytics, where data-driven decisions become more than just a possibility—they become a reality.
At its core, predictive analytics involves analyzing historical data to forecast future outcomes. However, traditional methods often fall short due to limitations in processing power and the inability to recognize complex patterns. Here’s where AI steps in, acting like a supercharged engine that processes vast amounts of data at lightning speed. With AI, businesses can uncover insights that would have otherwise remained hidden, enabling them to make informed decisions based on real-time data.
One of the most exciting aspects of AI-enhanced predictive analytics is its ability to adapt and learn. Just like a seasoned detective piecing together clues, AI algorithms can analyze data trends over time, adjusting their predictions as new information emerges. This means that businesses are not just reacting to market changes but are proactively shaping their strategies based on anticipated trends. Imagine the competitive edge this provides—companies can pivot quickly, ensuring they’re always a step ahead.
Moreover, AI doesn’t just improve the speed of data analysis; it significantly enhances accuracy. Traditional forecasting methods often rely on static models that can become outdated quickly. In contrast, AI leverages machine learning techniques to refine predictive models continually. For instance, as more data becomes available, AI systems can adjust their algorithms, leading to more precise predictions and insights. This is particularly crucial in today’s fast-paced business environment, where even a slight miscalculation can have significant repercussions.
To illustrate the impact of AI on predictive analytics, consider the following table that outlines the key benefits:
Benefit | Description |
---|---|
Increased Efficiency | Automates data analysis processes, saving time and resources. |
Enhanced Accuracy | Utilizes advanced algorithms to reduce errors in forecasting. |
Real-Time Insights | Provides immediate access to data trends, enabling faster decision-making. |
Personalization | Allows businesses to tailor strategies to meet customer needs effectively. |
As we delve deeper into the applications of AI in predictive analytics, it becomes clear that the potential is vast. From improving customer experiences to optimizing supply chain management, AI is revolutionizing how businesses operate. By harnessing the power of AI, companies can not only predict future trends but also create a more agile and responsive business model.
In conclusion, the integration of AI into predictive analytics is not just a trend; it's a fundamental shift in how businesses approach decision-making. With its ability to analyze vast datasets, uncover hidden insights, and adapt to new information, AI is paving the way for a future where businesses can thrive in uncertainty. So, are you ready to embrace this change and unlock the full potential of your data?
- What is predictive analytics? - Predictive analytics involves using historical data to forecast future outcomes, helping businesses make informed decisions.
- How does AI improve predictive analytics? - AI enhances predictive analytics by automating data analysis, increasing accuracy, and uncovering deeper insights from complex datasets.
- What are some applications of AI in business? - AI can be used in various areas, including customer experience personalization, supply chain optimization, and real-time decision-making.
- What future trends can we expect in predictive analytics? - Future trends may include increased automation, better integration of AI technologies, and improved capabilities for analyzing unstructured data.

The Role of AI in Data Analysis
In today's fast-paced business environment, the ability to analyze data effectively is more crucial than ever. Artificial Intelligence (AI) plays a transformative role in data analysis, acting as a powerful ally for businesses aiming to harness their data for strategic advantage. By automating data processing, AI not only increases efficiency but also enhances accuracy, allowing companies to derive insights that were previously hidden in vast amounts of information.
Imagine trying to find a needle in a haystack. This is akin to traditional data analysis, where sifting through countless records can be overwhelming. However, with AI, businesses can swiftly navigate through this data haystack, pinpointing critical insights and trends that can inform decision-making. The integration of AI technologies means that businesses can now analyze data in real-time, leading to faster and more informed decisions. This capability is especially vital in industries where timing is everything, such as finance or retail.
Moreover, AI enhances data analysis by utilizing advanced algorithms that can learn from data patterns over time. This process involves machine learning, where systems improve their performance as they are exposed to more data. For instance, AI can recognize patterns in customer behavior, enabling businesses to tailor their marketing strategies effectively. The shift from manual analysis to AI-driven insights not only saves time but also significantly reduces the likelihood of human error.
Furthermore, AI can analyze unstructured data—such as text, images, and videos—which traditional data analysis methods often struggle with. This capability opens up new avenues for understanding customer sentiments and preferences. For example, businesses can analyze social media interactions to gauge public opinion about their products or services, allowing for proactive adjustments to their strategies.
In summary, the role of AI in data analysis is not just about making processes faster; it’s about unlocking the full potential of data. By automating routine tasks, enhancing accuracy, and providing deeper insights, AI empowers businesses to make data-driven decisions that can significantly impact their success. As we continue to embrace this technology, the possibilities for innovation and growth are limitless.
- What is AI's primary function in data analysis? AI automates data processing, enhances accuracy, and uncovers insights, enabling informed decision-making.
- How does AI improve forecasting? Through advanced algorithms and machine learning, AI refines predictive models for more precise forecasts.
- Can AI analyze unstructured data? Yes, AI can effectively analyze unstructured data such as text and images, providing valuable insights.
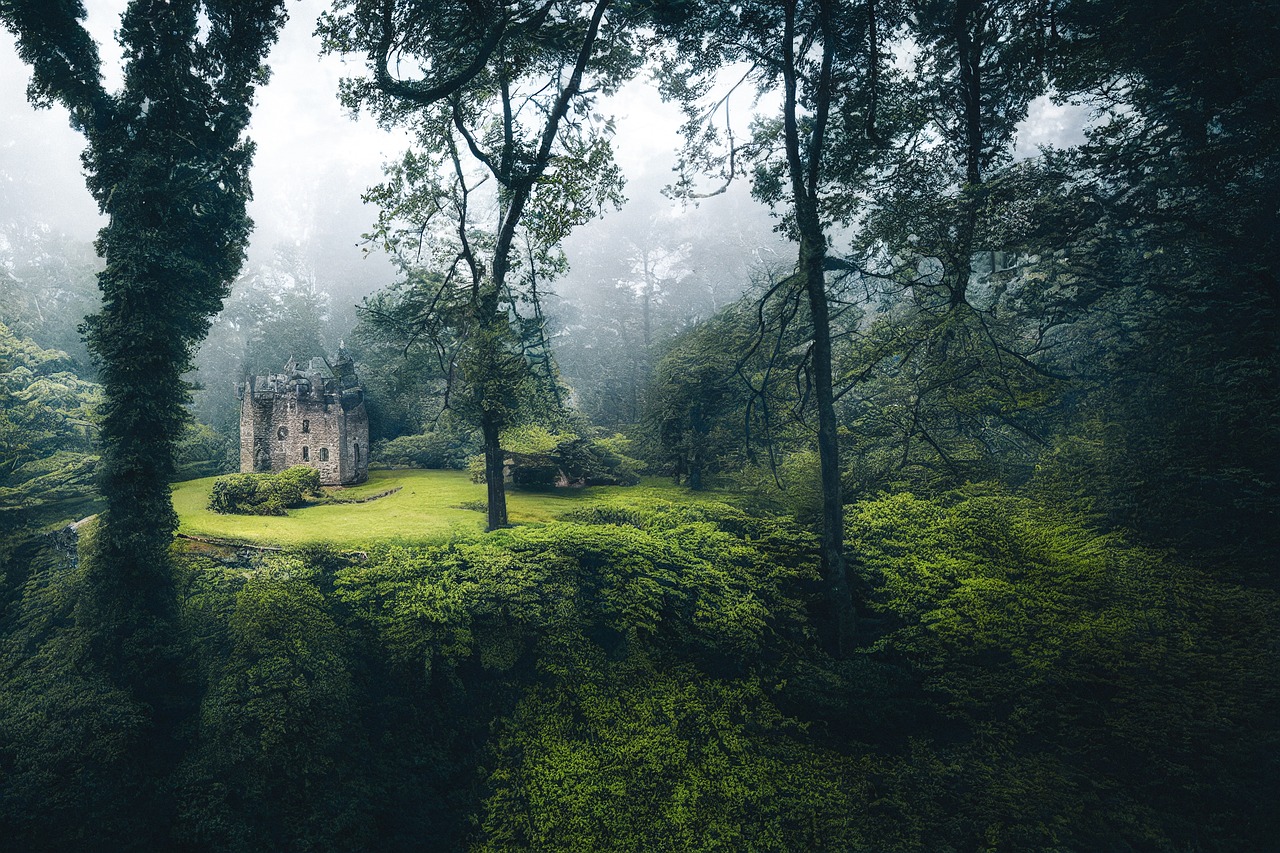
Improving Forecasting Accuracy
In the fast-paced world of business, the ability to predict future trends accurately is akin to having a crystal ball. With the advent of artificial intelligence (AI), companies are now equipped with powerful tools that significantly enhance their forecasting capabilities. By employing advanced algorithms and machine learning techniques, AI allows businesses to analyze complex datasets and extract valuable insights that were previously hidden. This not only leads to improved accuracy in predictions but also empowers organizations to make informed decisions that drive growth and success.
Imagine trying to navigate a ship through foggy waters without a compass. That's how many businesses operate without robust forecasting methods. AI acts as that compass, guiding companies through the uncertainty of market fluctuations and consumer behavior. With AI, forecasting is no longer a guessing game; it's a science backed by data. For instance, AI can analyze historical sales data, current market conditions, and even social media trends to predict future sales with remarkable precision. This ability to synthesize vast amounts of information in real-time is what sets AI apart from traditional forecasting methods.
One of the key advantages of AI in forecasting is its use of machine learning techniques. These techniques, including regression analysis and neural networks, play a crucial role in refining predictive models. For example, regression analysis helps businesses understand the relationships between various factors, such as pricing strategies, marketing campaigns, and customer demographics. By identifying these correlations, businesses can adjust their strategies to optimize sales and enhance customer satisfaction.
Machine learning is the backbone of AI-driven forecasting. By employing algorithms that learn from historical data, businesses can create models that continually improve over time. Here are a few ways machine learning contributes to better forecasting:
- Regression Analysis: This technique helps businesses quantify the impact of different variables on sales, allowing for more accurate predictions.
- Neural Networks: These mimic the human brain's functioning, enabling the identification of complex patterns in data that traditional methods might miss.
As businesses integrate these machine learning techniques into their forecasting processes, they not only enhance accuracy but also gain a competitive edge. The ability to anticipate market trends and consumer behaviors allows companies to be proactive rather than reactive, ensuring they stay ahead of the curve.
Moreover, the integration of big data with AI further amplifies forecasting accuracy. With access to an immense volume of data from various sources—such as sales records, social media interactions, and even weather patterns—businesses can perform more granular analyses. This wealth of information enables them to identify emerging trends and shifts in consumer preferences, leading to more precise forecasts.
In conclusion, improving forecasting accuracy through AI is not just about using advanced technology; it's about transforming how businesses operate. By leveraging AI's capabilities, companies can make smarter decisions, optimize their strategies, and ultimately achieve greater success in an ever-evolving marketplace.
1. How does AI improve forecasting accuracy?
AI enhances forecasting accuracy by analyzing large datasets using advanced algorithms and machine learning techniques, enabling businesses to uncover insights and predict future trends with greater precision.
2. What are some common machine learning techniques used in forecasting?
Common machine learning techniques include regression analysis and neural networks, which help businesses identify relationships between variables and recognize complex patterns in data.
3. Can AI help with real-time forecasting?
Yes, AI can facilitate real-time forecasting by processing data as it becomes available, allowing businesses to respond quickly to changes in market conditions and consumer behavior.
4. What role does big data play in predictive analytics?
Big data provides a vast amount of information that, when integrated with AI, allows businesses to conduct deeper analyses and make more accurate predictions.
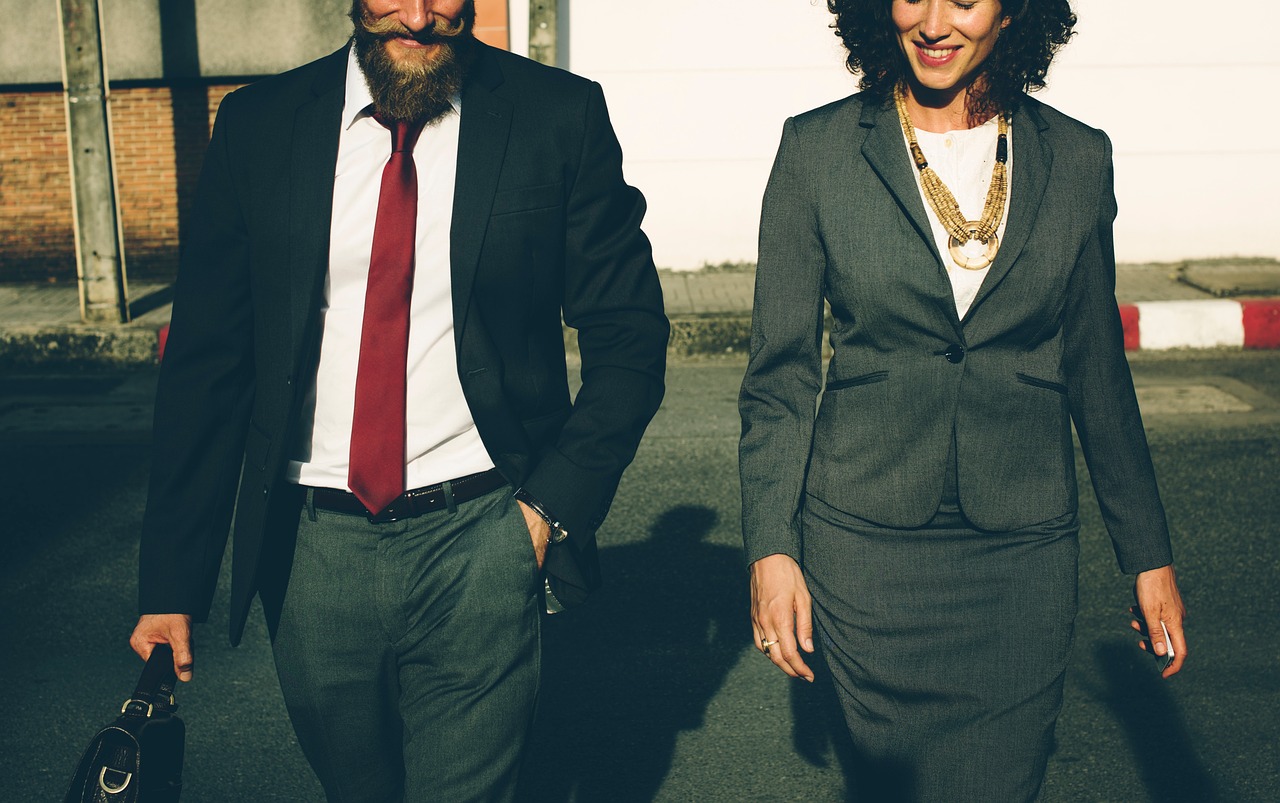
Machine Learning Techniques
When we talk about , we’re diving into the heart of predictive analytics. These techniques are not just fancy algorithms; they are the backbone of how businesses can make sense of the massive amounts of data they collect every day. Imagine having a crystal ball that not only predicts the future but does so with incredible accuracy. That’s what machine learning brings to the table!
One of the most effective methods in this realm is regression analysis. This technique helps businesses understand the relationships between various factors. For example, it can reveal how changes in marketing spend impact sales or how customer satisfaction correlates with product features. By using regression analysis, companies can identify which variables are most influential, allowing them to focus their efforts where they matter most.
Then we have neural networks, which are a bit like the brain's wiring. These networks are designed to recognize patterns in data, making them ideal for complex tasks such as image recognition or natural language processing. Think of neural networks as a sophisticated detective, sifting through heaps of data to find hidden clues that can inform business decisions. With their ability to learn from vast datasets, they can enhance predictive modeling by identifying trends that traditional methods might overlook.
Moreover, let’s not forget the importance of big data integration in machine learning. When businesses combine big data with machine learning techniques, they unlock a treasure trove of insights. This integration allows for deeper analysis, leading to more accurate forecasts. Imagine trying to find a needle in a haystack; big data is that haystack, and machine learning is your magnet, pulling out valuable insights effortlessly.
To illustrate the impact of these techniques, consider the following table that highlights the differences between regression analysis and neural networks:
Feature | Regression Analysis | Neural Networks |
---|---|---|
Complexity | Less complex, easier to interpret | Highly complex, harder to interpret |
Data Requirements | Works well with smaller datasets | Requires large datasets for training |
Use Cases | Sales forecasting, trend analysis | Image recognition, natural language processing |
In conclusion, machine learning techniques like regression analysis and neural networks are essential tools in the predictive analytics toolbox. They enable businesses to harness the power of data, providing insights that lead to informed decision-making. As we continue to evolve in this digital age, the businesses that leverage these techniques will undoubtedly hold the key to success.
- What is machine learning? Machine learning is a subset of artificial intelligence that enables systems to learn from data and improve their performance over time without being explicitly programmed.
- How does regression analysis work? Regression analysis examines the relationship between dependent and independent variables to predict outcomes based on input data.
- What are neural networks used for? Neural networks are used for complex data analysis tasks, including image and speech recognition, as well as predictive modeling.
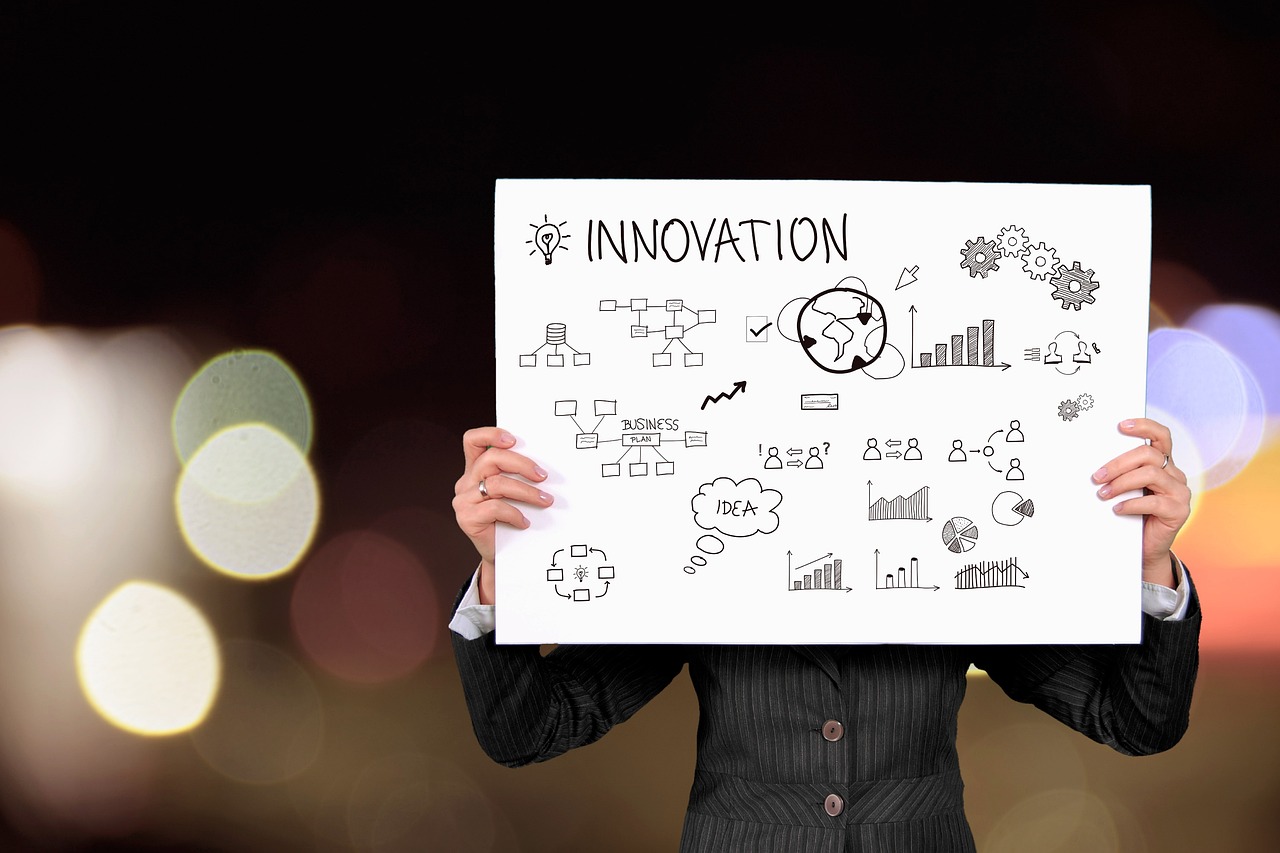
Regression Analysis in Business
Regression analysis is a powerful statistical method that plays a crucial role in the decision-making processes of businesses. By examining the relationships between different variables, regression analysis helps organizations understand how various factors influence key performance indicators such as sales, customer satisfaction, and operational efficiency. Imagine trying to piece together a puzzle; regression analysis provides the framework to connect the dots, revealing insights that might otherwise remain obscured.
At its core, regression analysis allows businesses to identify trends and make predictions based on historical data. For instance, a retail company may want to understand how advertising spend affects sales revenue. By employing regression analysis, they can quantify the relationship between these variables, enabling them to allocate their marketing budget more effectively. This insight not only leads to better financial outcomes but also fosters a culture of data-driven decision-making within the organization.
Moreover, the beauty of regression analysis lies in its versatility. There are various types of regression techniques, such as:
- Linear Regression: This is the simplest form, where the relationship between two variables is modeled as a straight line. It's particularly useful for understanding direct correlations.
- Multiple Regression: This technique analyzes multiple independent variables to see how they collectively impact a dependent variable. For example, a company might study how factors like pricing, marketing efforts, and product quality jointly influence customer satisfaction.
- Logistic Regression: Often used in binary outcome scenarios, logistic regression helps businesses predict outcomes such as whether a customer will buy a product or not based on various predictors.
To illustrate the impact of regression analysis in a business context, consider the following table that summarizes how different industries utilize regression techniques:
Industry | Application of Regression Analysis |
---|---|
Retail | Predicting sales based on marketing spend and seasonal trends. |
Finance | Assessing risk factors and predicting stock prices based on economic indicators. |
Healthcare | Understanding the impact of treatment types on patient recovery rates. |
Manufacturing | Evaluating how production levels affect operational costs and efficiency. |
In conclusion, regression analysis serves as a vital tool that empowers businesses to make informed decisions by revealing the intricate relationships between various factors. By leveraging this analytical technique, organizations can not only enhance their understanding of market dynamics but also improve their strategic planning efforts. As the business landscape continues to evolve, the ability to analyze and interpret data through regression will remain an invaluable asset.
Q: What is the primary purpose of regression analysis in business?
A: The primary purpose of regression analysis is to understand the relationships between different variables, allowing businesses to make informed decisions and predict future outcomes based on historical data.
Q: Can regression analysis be used in any industry?
A: Yes, regression analysis is versatile and can be applied across various industries, including retail, finance, healthcare, and manufacturing, to analyze and predict trends.
Q: What are some common types of regression analysis?
A: Common types include linear regression, multiple regression, and logistic regression, each serving different purposes based on the nature of the data and the analysis required.
Q: How does regression analysis enhance decision-making?
A: By providing insights into how different factors influence outcomes, regression analysis helps businesses allocate resources more effectively and develop strategies that align with market trends.
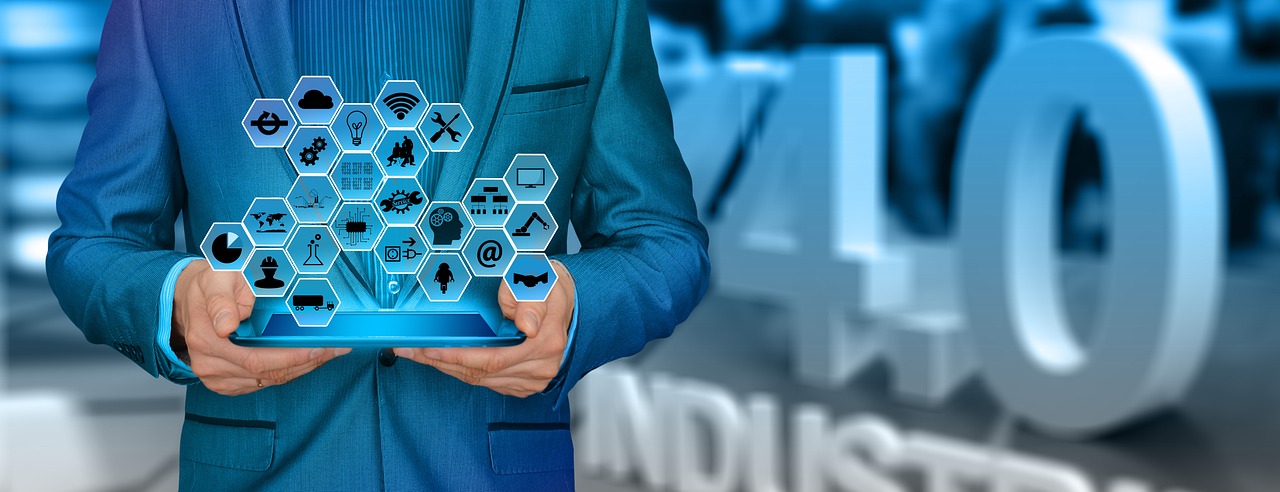
Neural Networks and Their Applications
Neural networks are a fascinating aspect of artificial intelligence that mimic the intricate workings of the human brain. By utilizing interconnected nodes, or "neurons," these systems can process vast amounts of data and identify complex patterns that traditional algorithms might overlook. This capability makes neural networks incredibly powerful for various applications across different industries.
One of the most prominent applications of neural networks is in image recognition. For instance, tech giants like Google and Facebook employ these networks to tag people in photos automatically. The neural network analyzes the pixels in an image, identifies patterns, and matches them with known features of individuals. This technology has not only revolutionized social media but has also found its way into security systems, where facial recognition is critical.
Another significant application is in natural language processing (NLP). Companies like Amazon and Apple use neural networks to enhance their virtual assistants, Alexa and Siri, respectively. These systems learn from vast datasets of spoken language, allowing them to understand and respond to user queries with remarkable accuracy. This capability is essential for providing a seamless user experience, as it enables the assistants to comprehend context, tone, and even slang.
In the realm of financial services, neural networks play a crucial role in fraud detection. By analyzing transaction patterns, these networks can identify anomalies that may indicate fraudulent activity. For example, if a user typically makes small purchases in their hometown but suddenly makes a large purchase overseas, the neural network can flag this as suspicious and alert the financial institution. This proactive approach not only protects consumers but also saves businesses significant amounts of money.
Moreover, neural networks are making waves in the field of healthcare by assisting in diagnostics. For instance, deep learning models can analyze medical images, such as X-rays and MRIs, to detect diseases like cancer at an early stage. This capability significantly enhances the accuracy and speed of diagnosis, ultimately leading to better patient outcomes. In fact, a study published in a reputable medical journal revealed that neural networks outperformed human radiologists in detecting certain types of tumors.
As we look to the future, the applications of neural networks are only expected to expand. With advancements in technology and an increasing amount of data available, businesses across various sectors will continue to harness the power of these networks. Whether it's through enhancing customer service, streamlining operations, or improving product recommendations, the potential is vast.
- What are neural networks? Neural networks are computational models inspired by the human brain, designed to recognize patterns and make predictions based on data.
- How are neural networks used in business? They are utilized for applications such as image recognition, natural language processing, fraud detection, and diagnostics in healthcare.
- What industries benefit from neural networks? Industries like technology, finance, healthcare, and retail are leveraging neural networks to enhance their operations and customer experiences.
- Will neural networks replace human jobs? While they may automate certain tasks, neural networks are more likely to augment human capabilities rather than completely replace jobs.
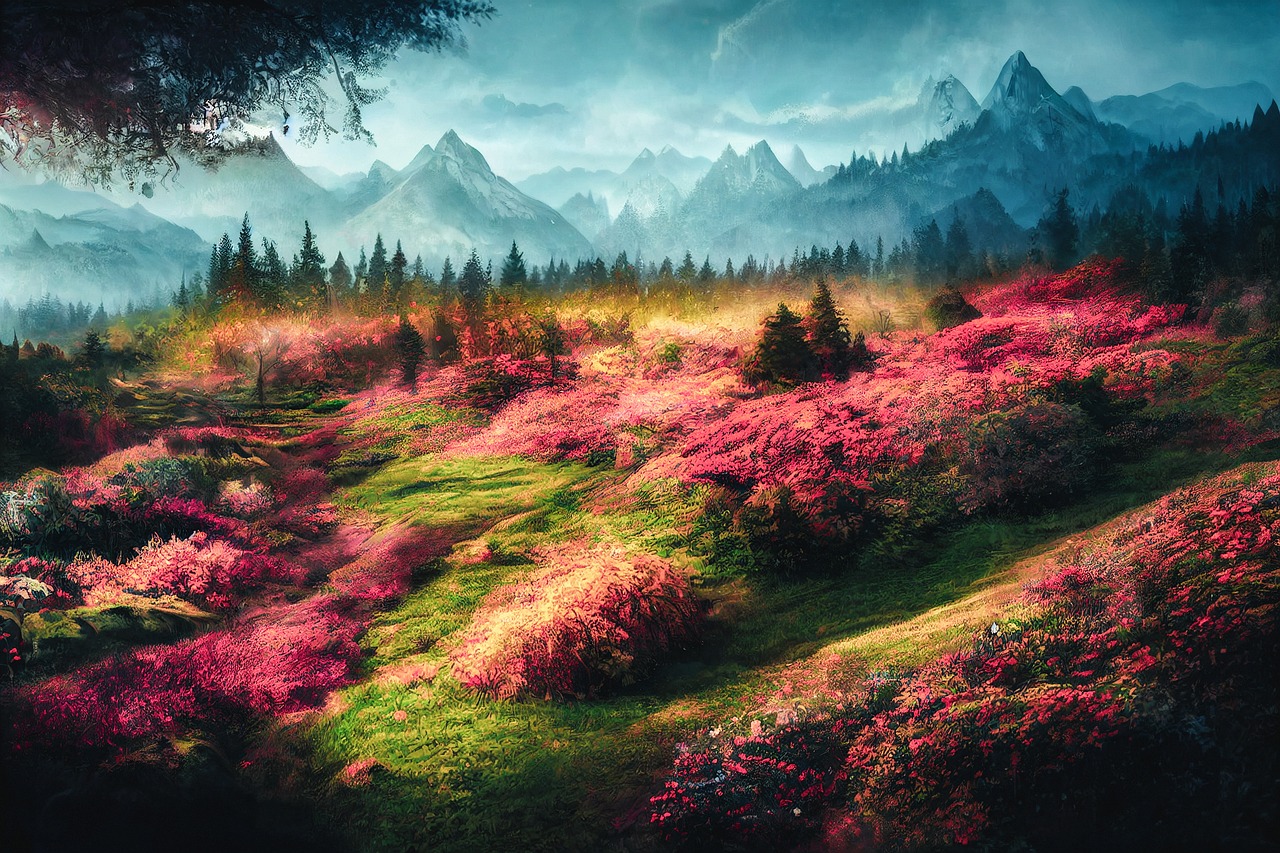
Big Data Integration
In today's digital landscape, the term big data is often thrown around, but what does it really mean for businesses? Well, imagine trying to find a needle in a haystack. Now, what if that haystack were made of millions of needles, each representing valuable insights? This is where comes into play, acting as the powerful magnet that pulls those needles together, transforming raw data into actionable intelligence.
Big data integration involves the process of combining data from various sources to create a unified view that enhances predictive analytics. By leveraging AI, businesses can sift through vast amounts of data—structured and unstructured—to extract meaningful patterns and trends. This not only improves the accuracy of predictions but also unveils hidden opportunities that can propel a business forward.
Consider the retail industry as a prime example. Retailers collect data from numerous channels, including point-of-sale systems, online transactions, social media interactions, and customer feedback. Integrating this data allows them to:
- Understand customer preferences and behavior
- Optimize inventory management
- Enhance marketing strategies
Moreover, with the integration of big data, predictive models become more robust. AI algorithms can analyze historical data alongside real-time inputs, resulting in predictions that are not only timely but also highly relevant. This capability is particularly critical in industries like finance, where market conditions can change in the blink of an eye.
To illustrate the impact of big data integration, let’s take a look at a simple table that summarizes its benefits:
Benefit | Description |
---|---|
Enhanced Decision-Making | Access to comprehensive data allows for more informed and strategic decisions. |
Increased Efficiency | Streamlining data processes reduces time and resource wastage. |
Competitive Advantage | Businesses can anticipate market trends and stay ahead of competitors. |
In conclusion, the integration of big data with AI technologies is revolutionizing predictive analytics. It empowers businesses to harness the full potential of their data, turning potential chaos into clarity. As we move forward, those who effectively integrate big data into their predictive models will not only survive but thrive in an increasingly competitive landscape.
- What is big data integration? Big data integration is the process of combining data from multiple sources to create a cohesive view that enhances analytics and decision-making.
- How does AI improve predictive analytics? AI enhances predictive analytics by automating data analysis, improving accuracy, and uncovering insights that can drive business strategies.
- Why is real-time data important? Real-time data allows businesses to make swift decisions, adapt to market changes, and respond to customer needs effectively.
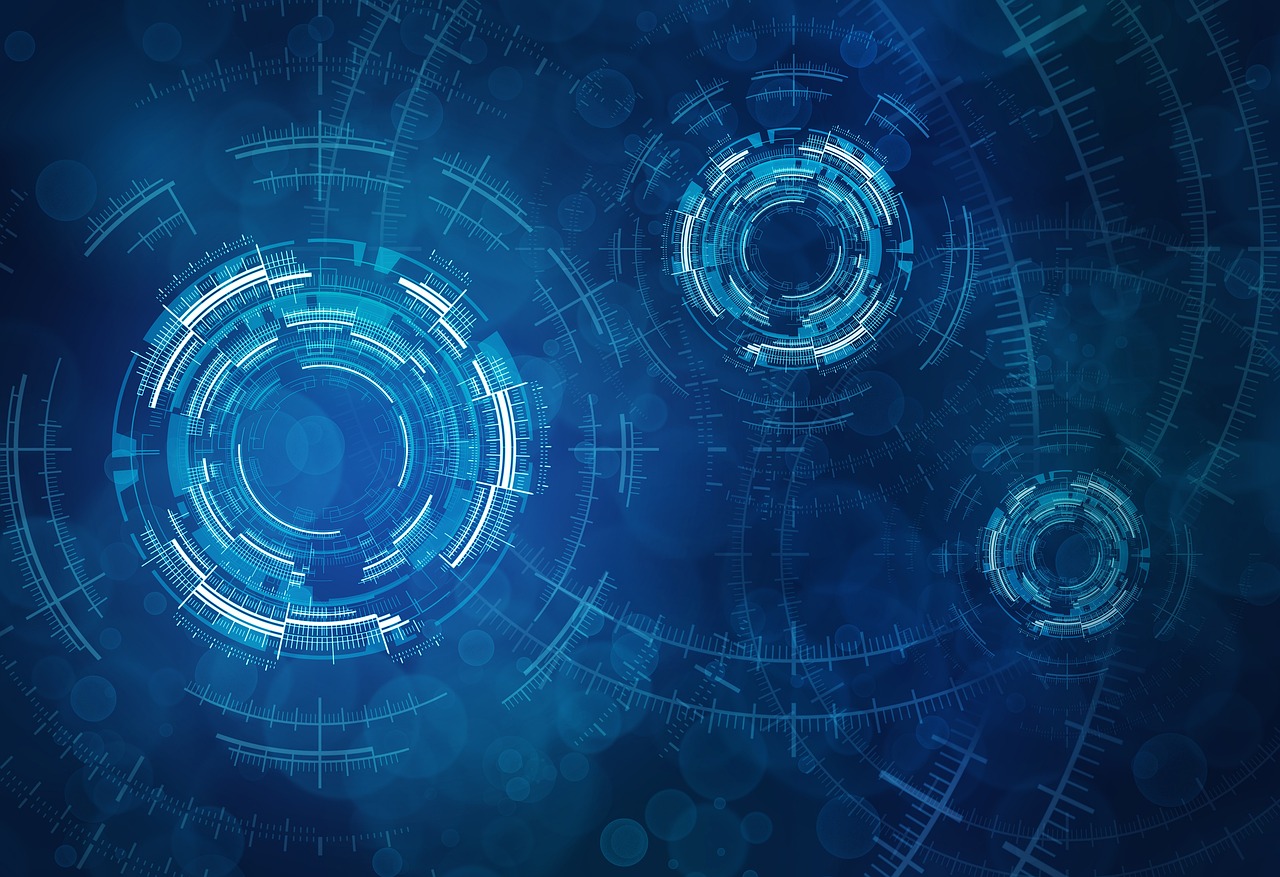
Real-Time Decision Making
In today's fast-paced business environment, the ability to make real-time decisions is more crucial than ever. With the integration of artificial intelligence (AI) into predictive analytics, companies can now analyze data as it comes in, allowing them to respond to market changes and consumer behavior almost instantly. Imagine being able to adjust your marketing strategy on the fly, based on real-time data about customer preferences and purchasing patterns. This level of agility is not just beneficial; it is essential for survival in a competitive landscape.
AI-powered predictive analytics tools process vast amounts of data in real time, enabling businesses to gain insights that were previously out of reach. For instance, retailers can monitor inventory levels and sales trends continuously, adjusting their stock and marketing efforts dynamically. This leads to a more efficient operation, reducing waste and maximizing profits. The ability to react swiftly can be the difference between capitalizing on a fleeting trend and missing out entirely.
Furthermore, real-time decision making enhances customer experience. By analyzing customer interactions as they happen, businesses can tailor their offerings to meet immediate needs. For example, if a customer shows interest in a specific product online, AI can trigger personalized marketing messages or special offers, increasing the likelihood of conversion. This level of personalization fosters a deeper connection with customers, driving loyalty and repeat business.
To illustrate the impact of real-time decision making, consider the following table that highlights key benefits:
Benefit | Description |
---|---|
Increased Agility | Businesses can adapt to changes in consumer behavior and market trends swiftly. |
Enhanced Customer Experience | Personalized interactions lead to improved customer satisfaction and loyalty. |
Optimized Operations | Efficient resource allocation and inventory management reduce costs. |
Competitive Advantage | Real-time insights allow businesses to stay ahead of competitors. |
In summary, leveraging AI for real-time decision making is not just a trend; it’s a game changer for businesses across various sectors. Companies that embrace this technology will find themselves better equipped to navigate the complexities of the modern marketplace, ultimately leading to greater success and sustainability.
- What is real-time decision making? Real-time decision making involves analyzing data and making decisions instantly, allowing businesses to respond quickly to changes in the market or consumer behavior.
- How does AI enhance predictive analytics? AI automates data analysis, improves accuracy, and uncovers hidden insights, enabling businesses to make informed decisions based on real-time data.
- What industries benefit from real-time decision making? Many industries, including retail, finance, healthcare, and logistics, can benefit from real-time decision making to optimize operations and improve customer experiences.
- Can small businesses leverage AI for real-time decision making? Absolutely! With the availability of affordable AI tools, small businesses can also harness the power of real-time data analysis to enhance their operations.
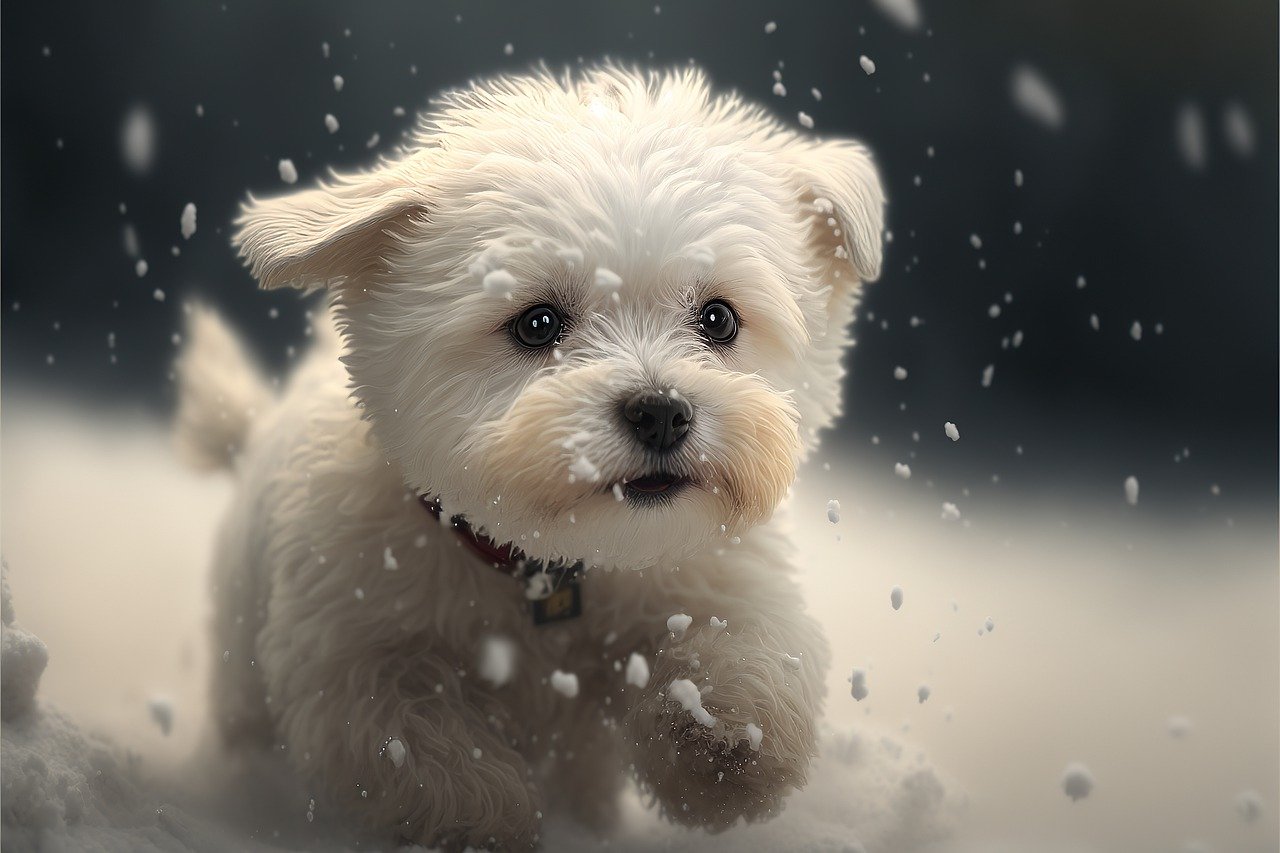
Impact on Customer Experience
In today's fast-paced digital world, customer experience is paramount to business success. With the integration of AI-powered predictive analytics, companies can transform how they interact with their customers. Imagine walking into a store where the staff knows your preferences and can recommend products tailored just for you—that's the kind of personalized experience AI can facilitate. By analyzing vast amounts of data, businesses can anticipate customer needs, preferences, and behaviors, leading to a more engaging and satisfying experience.
One of the key benefits of utilizing predictive analytics in customer experience is the ability to create personalized marketing campaigns. Instead of sending generic advertisements, businesses can tailor their messages based on individual customer profiles. For instance, if a customer frequently buys sports equipment, they might receive promotions for the latest gear or exclusive offers on related products. This level of personalization not only increases the likelihood of a purchase but also fosters a deeper connection between the brand and the consumer.
Moreover, AI can analyze customer feedback and sentiment in real-time, allowing businesses to make immediate adjustments to their strategies. This responsiveness can significantly enhance customer satisfaction. For example, if a company notices a spike in negative reviews about a specific product, they can quickly investigate and address the issue before it escalates. This proactive approach not only mitigates potential damage to the brand's reputation but also shows customers that their opinions are valued.
Another fascinating aspect of AI in customer experience is its capability to predict customer churn. By analyzing patterns in customer behavior, businesses can identify which customers are likely to disengage and take action to retain them. This might involve targeted offers, personalized outreach, or improved service options. According to recent studies, businesses that leverage predictive analytics for churn prevention can reduce customer turnover by as much as 15% to 20%.
Finally, the integration of AI can enhance customer service through chatbots and virtual assistants. These tools can provide instant support, answer queries, and even guide customers through complex processes. The efficiency of AI-driven customer service not only saves time for customers but also allows human agents to focus on more complex issues that require a personal touch. In fact, research indicates that over 70% of customers prefer interacting with an AI chatbot for quick inquiries, further illustrating the impact of AI on customer experience.
In conclusion, the impact of AI on customer experience is profound and multifaceted. By leveraging predictive analytics, businesses can create personalized interactions, respond swiftly to feedback, anticipate customer needs, and enhance service efficiency. As we continue to embrace AI technology, the potential for improving customer experiences will only grow, making it an essential component of modern business strategy.
- How does AI improve customer service? AI improves customer service by providing instant support through chatbots, analyzing customer feedback in real-time, and predicting customer needs.
- Can predictive analytics really reduce customer churn? Yes, businesses that utilize predictive analytics can identify customers at risk of churning and implement targeted strategies to retain them.
- What are the benefits of personalized marketing? Personalized marketing increases customer engagement, improves conversion rates, and fosters brand loyalty by tailoring messages to individual preferences.
- How does AI analyze customer feedback? AI uses natural language processing to analyze sentiment in customer reviews and feedback, allowing businesses to make data-driven decisions.
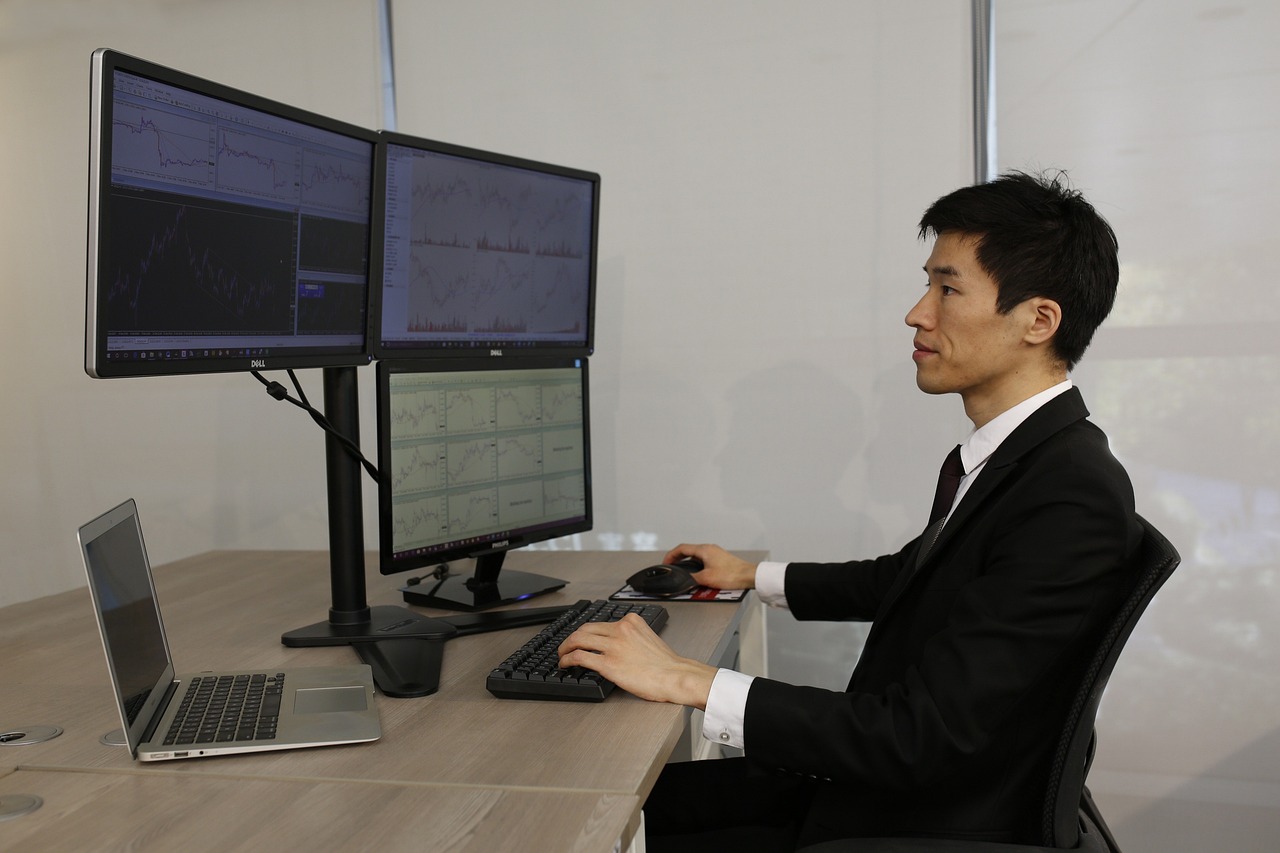
Optimizing Supply Chain Management
In today's fast-paced business world, is more critical than ever. With the integration of artificial intelligence into predictive analytics, companies can now streamline their operations like never before. Imagine a world where businesses can foresee demand fluctuations, adjust their inventory levels accordingly, and enhance their logistics—all in real-time! This is not just a dream; it’s the reality that AI brings to the table.
One of the most significant advantages of using AI in supply chain management is its ability to analyze vast amounts of data quickly. By leveraging machine learning algorithms, companies can predict customer demand with remarkable accuracy. This means that instead of relying on historical data alone, businesses can consider various factors such as market trends, seasonal variations, and even social media sentiment to make informed decisions.
For instance, let’s say a retailer experiences a sudden increase in demand for winter jackets due to an unexpected cold snap. An AI-driven system can analyze weather forecasts, historical sales data, and even social media buzz to predict this surge. As a result, the retailer can adjust their inventory levels proactively, ensuring they have enough stock to meet the demand without overcommitting resources.
Moreover, AI optimizes logistics by identifying the most efficient routes and methods for transportation. By analyzing traffic patterns, weather conditions, and shipment schedules, AI can suggest the best paths for delivery trucks, minimizing delays and reducing costs. This level of efficiency not only saves money but also enhances customer satisfaction by ensuring timely deliveries.
To illustrate the impact of AI on supply chain management, consider the following table that compares traditional methods with AI-enhanced strategies:
Aspect | Traditional Methods | AI-Enhanced Methods |
---|---|---|
Demand Forecasting | Based on historical data | Incorporates real-time data and trends |
Inventory Management | Reactive approach | Proactive adjustments based on predictions |
Logistics Optimization | Static routing | Dynamic routing based on real-time analysis |
Customer Satisfaction | Variable delivery times | Consistent, timely deliveries |
As you can see, the integration of AI into supply chain management transforms how businesses operate. It allows for a seamless flow of information and a more agile response to market changes. In a competitive landscape, companies that embrace these technologies will not only survive but thrive.
In conclusion, optimizing supply chain management through AI-driven predictive analytics is a game-changer for businesses. It empowers them to make data-driven decisions, enhance operational efficiency, and ultimately deliver a better experience for their customers. As we look to the future, the potential for AI in this realm is limitless, and companies that invest in these technologies will undoubtedly see significant returns.
- What is predictive analytics?
Predictive analytics involves using statistical algorithms and machine learning techniques to identify the likelihood of future outcomes based on historical data. - How does AI improve supply chain management?
AI enhances supply chain management by providing real-time insights, improving demand forecasting, optimizing logistics, and enabling proactive inventory management. - Can small businesses benefit from AI in their supply chains?
Absolutely! AI tools are becoming increasingly accessible, allowing small businesses to leverage advanced analytics for better decision-making and efficiency. - What are some challenges of implementing AI in supply chains?
Challenges may include data integration issues, the initial costs of technology, and the need for skilled personnel to manage AI systems.
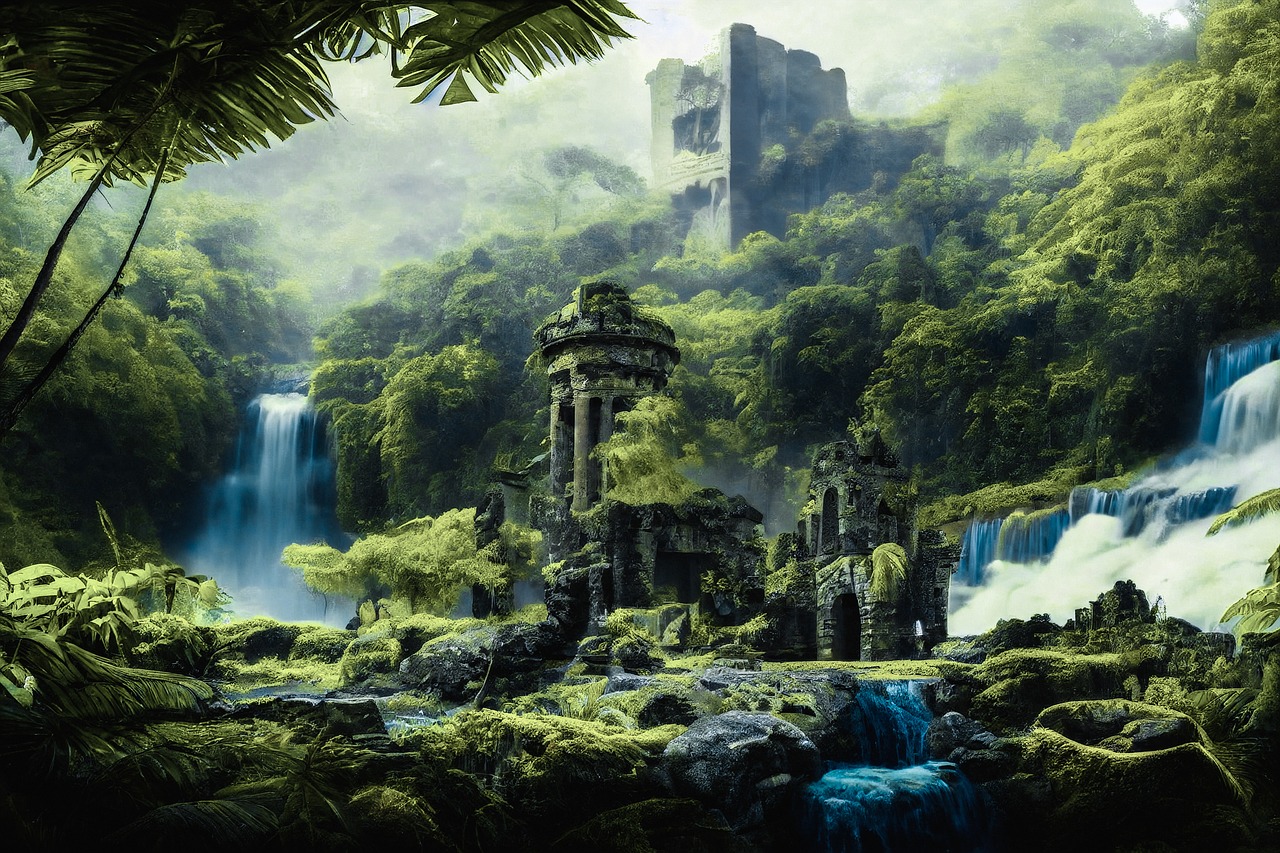
Future Trends in Predictive Analytics
The landscape of predictive analytics is constantly evolving, and as we look ahead, several exciting trends are set to shape its future. First and foremost, the integration of artificial intelligence will become even more profound. As businesses continue to harness the power of AI, predictive analytics will transition from traditional methods to more advanced, automated systems that can analyze data in real-time. This shift not only enhances the speed of data processing but also improves the accuracy of predictions, allowing companies to stay ahead of the curve.
Moreover, the rise of edge computing will play a critical role. By processing data closer to the source, businesses can make faster decisions based on real-time analytics. Imagine a retail store that uses predictive analytics to adjust inventory levels on the fly based on customer behavior detected by sensors. This kind of real-time responsiveness will be a game changer, allowing businesses to react swiftly to market changes and consumer demands.
Another fascinating trend is the increasing focus on unstructured data. While structured data has been the backbone of predictive analytics, unstructured data from sources like social media, customer reviews, and IoT devices is becoming invaluable. Businesses that can effectively analyze this type of data will gain deeper insights into customer sentiments and market trends. For instance, a company could analyze social media chatter to predict shifts in consumer preferences before they become apparent in sales data.
Additionally, the use of natural language processing (NLP) will enhance the capabilities of predictive analytics. With NLP, businesses can analyze text data to extract meaningful insights, such as understanding customer feedback or identifying emerging trends from news articles. This will empower companies to make more informed decisions based on comprehensive data analysis.
As we move forward, we can also expect to see a greater emphasis on ethical AI in predictive analytics. With increasing scrutiny on data privacy and algorithmic bias, businesses will need to ensure that their predictive models are fair and transparent. This includes implementing robust governance frameworks to manage data usage and model development, ultimately building trust with consumers.
Trend | Description |
---|---|
AI Integration | Enhanced automation and accuracy in data analysis. |
Edge Computing | Real-time data processing for quicker decision-making. |
Unstructured Data Analysis | Leveraging data from social media and IoT for deeper insights. |
Natural Language Processing | Analyzing text data for customer sentiment and trends. |
Ethical AI | Focus on fairness, transparency, and data privacy. |
In conclusion, the future of predictive analytics is bright and full of potential. With advancements in technology and a growing emphasis on ethical practices, businesses that adapt to these trends will not only enhance their decision-making capabilities but also secure a competitive edge in their respective markets. The question is, are you ready to embrace these changes and leverage the power of predictive analytics to drive your business forward?
- What is predictive analytics? Predictive analytics involves using statistical algorithms and machine learning techniques to identify the likelihood of future outcomes based on historical data.
- How does AI enhance predictive analytics? AI enhances predictive analytics by automating data analysis, improving accuracy, and uncovering insights that help businesses make informed decisions.
- What role does big data play in predictive analytics? Big data provides the vast amount of information needed for accurate predictions, allowing for deeper insights and better forecasting.
- Why is real-time decision-making important? Real-time decision-making allows businesses to quickly respond to market changes and consumer preferences, giving them a competitive advantage.
Frequently Asked Questions
- What is predictive analytics?
Predictive analytics is a branch of advanced analytics that uses historical data, statistical algorithms, and machine learning techniques to identify the likelihood of future outcomes. It's like having a crystal ball that helps businesses foresee trends and make informed decisions.
- How does AI improve predictive analytics?
AI enhances predictive analytics by automating data analysis processes, increasing accuracy, and uncovering hidden insights. This allows businesses to make decisions based on real-time data, ultimately leading to more effective strategies.
- What role do machine learning techniques play in predictive analytics?
Machine learning techniques, such as regression analysis and neural networks, are crucial for refining predictive models. They enable businesses to analyze large datasets, improving the precision of predictions and insights.
- Can predictive analytics help improve customer experience?
Absolutely! By leveraging predictive analytics, businesses can personalize customer experiences, anticipate needs, and enhance engagement. This leads to increased customer loyalty and satisfaction, making customers feel valued and understood.
- How does AI optimize supply chain management?
AI-driven predictive analytics optimizes supply chain management by accurately forecasting demand, reducing inventory costs, and improving logistics. This ensures that businesses operate efficiently and can respond swiftly to market changes.
- What future trends can we expect in predictive analytics?
The future of predictive analytics will likely see increased automation, greater AI integration, and enhanced capabilities for analyzing unstructured data. These advancements will continue to shape the business landscape and improve decision-making processes.