Understanding Recent Advances in Computational Biology
In the ever-evolving landscape of science, computational biology stands out as a beacon of innovation and promise. This interdisciplinary field merges biology, computer science, and mathematics, creating a powerful toolkit for researchers to tackle some of the most complex biological questions. With the recent advances in technology, particularly in areas like machine learning and high-throughput sequencing, we are witnessing a revolution in how we understand life at a molecular level. Imagine being able to sift through millions of genetic sequences in the blink of an eye—this is not just a dream; it’s happening right now!
The impact of computational biology extends far beyond the laboratory bench. It is reshaping the way we approach genomics, drug discovery, and even personalized medicine. For instance, thanks to sophisticated algorithms and computational models, researchers can now identify genetic mutations associated with diseases much faster than ever before. This acceleration in research not only enhances our understanding of complex conditions but also paves the way for developing targeted therapies that can significantly improve patient outcomes.
One of the most exciting aspects of computational biology is its ability to analyze vast datasets. In the past, studying biological data was akin to searching for a needle in a haystack, but now, with advanced computational techniques, we can quickly pinpoint that needle. This capability is crucial in fields such as genomics, where the sheer volume of data generated by sequencing technologies can be overwhelming. The integration of artificial intelligence into these processes allows for more nuanced insights, enabling researchers to make connections that were previously impossible.
However, as we embrace these advancements, it’s essential to acknowledge the challenges that come along with them. Issues such as data integration and the need for standardized methodologies are critical hurdles that researchers must overcome to ensure the reliability and reproducibility of their findings. The landscape of computational biology is not just about harnessing the latest technologies; it’s also about creating frameworks that enable effective collaboration and innovation across disciplines.
As we look to the future, the potential for computational biology seems limitless. With the integration of big data analytics and ongoing advancements in bioinformatics tools, we are on the cusp of breakthroughs that could redefine our understanding of biology and medicine. The prospect of interdisciplinary collaborations further enhances this field, bringing together experts from various domains to foster innovation and tackle pressing health challenges.
In summary, the advances in computational biology represent a transformative shift in our approach to biological research. By leveraging cutting-edge technologies and fostering collaboration, we can unlock new insights that will not only enhance our understanding of life but also lead to practical applications that improve health outcomes worldwide. The journey is just beginning, and the future holds exciting possibilities for those willing to explore the depths of this fascinating field.
- What is computational biology? Computational biology is an interdisciplinary field that uses computational techniques to analyze and model biological systems and data.
- How does computational biology impact drug discovery? It streamlines the identification of drug candidates and optimizes their development through simulations and modeling.
- What role does machine learning play in computational biology? Machine learning algorithms help analyze large datasets, identify patterns, and make predictions in biological research.
- What are the challenges faced in computational biology? Key challenges include data integration, computational resource limitations, and the need for standardized methodologies.
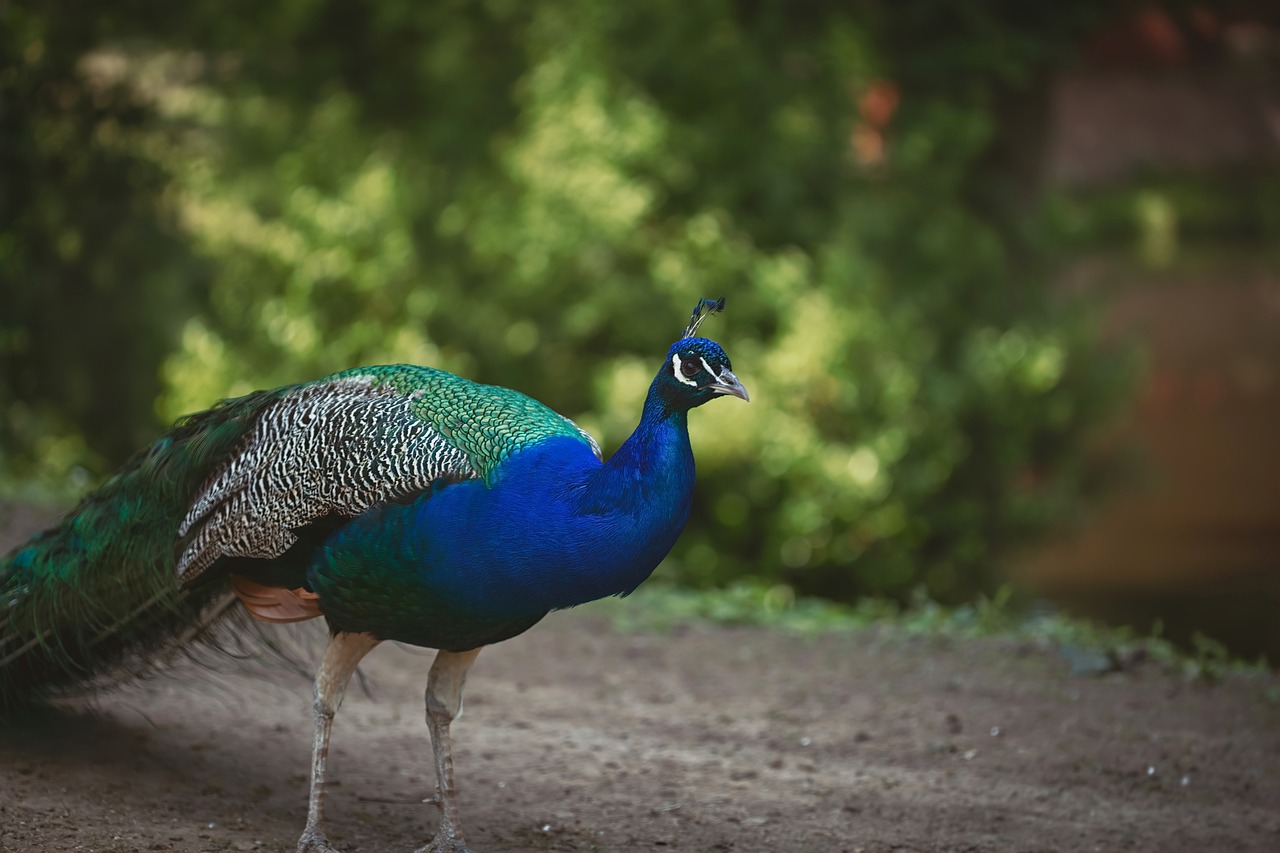
Key Technologies in Computational Biology
In the rapidly evolving world of computational biology, several key technologies are spearheading groundbreaking advancements that are reshaping our understanding of biological systems. These technologies not only enhance research capabilities but also pave the way for innovative applications across various fields. At the forefront of this revolution are machine learning, artificial intelligence, and high-throughput sequencing, each playing a pivotal role in transforming how scientists approach biological data.
Machine learning has emerged as a game-changer in computational biology. By leveraging algorithms that can learn and adapt from data, researchers can analyze vast datasets more efficiently than ever before. Imagine trying to find a needle in a haystack; machine learning algorithms act as powerful magnets, helping to sift through mountains of genetic information to uncover hidden patterns and insights. This technology is particularly useful in identifying genetic mutations and predicting their potential impacts on health.
Similarly, the integration of artificial intelligence into biological research is revolutionizing the way we interpret complex biological phenomena. AI systems can process and analyze data at speeds and accuracies that far exceed human capabilities. For instance, AI-driven tools can assist in the interpretation of genomic sequences, enabling researchers to pinpoint variations that may contribute to diseases. This capability not only accelerates research but also enhances the precision of findings, leading to more informed conclusions.
Another crucial technology is high-throughput sequencing, which allows scientists to sequence DNA and RNA at an unprecedented scale. This technology has democratized access to genomic data, making it possible to conduct large-scale studies that were previously unfeasible. High-throughput sequencing is akin to having a high-speed camera that captures every detail of a fast-moving event, providing a comprehensive view of genetic information. The implications for research are immense, allowing for the identification of genetic markers associated with diseases, which can lead to earlier diagnoses and targeted therapies.
To illustrate the impact of these technologies, consider the following table that summarizes their key features and applications:
Technology | Key Features | Applications |
---|---|---|
Machine Learning | Data-driven algorithms, pattern recognition | Genetic mutation analysis, disease prediction |
Artificial Intelligence | Automated data processing, predictive analytics | Genomic sequence interpretation, drug discovery |
High-Throughput Sequencing | Rapid sequencing, large-scale data generation | Population genomics, personalized medicine |
In summary, the integration of these key technologies in computational biology is not just enhancing our research capabilities; it's fundamentally changing the way we understand life itself. With machine learning, artificial intelligence, and high-throughput sequencing at our disposal, we are entering an era where the possibilities for discovery are virtually limitless. As we continue to harness these technologies, we can expect to see even more exciting breakthroughs that will propel the field of biology into new frontiers.
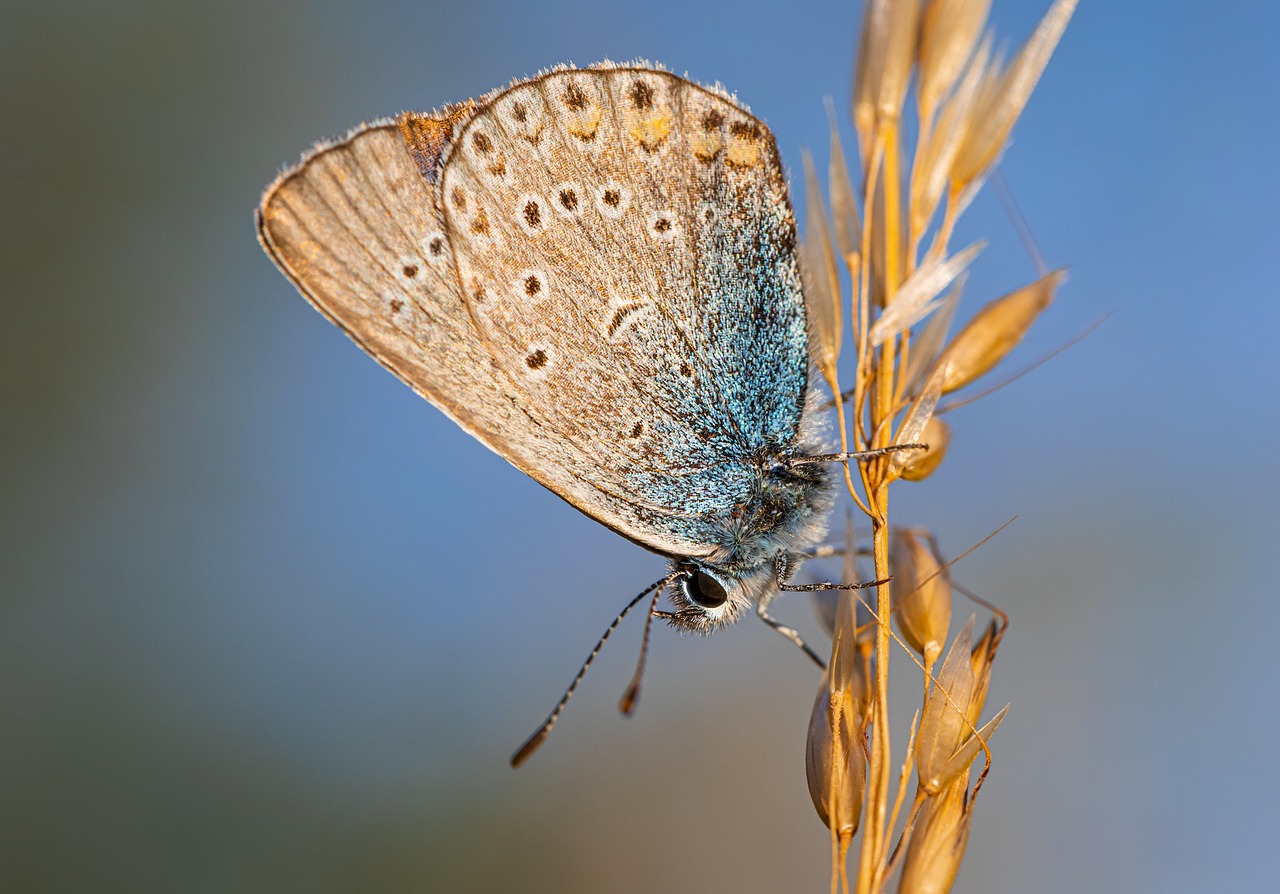
Applications in Genomics
In recent years, the field of genomics has experienced a seismic shift thanks to the advancements in computational biology. Imagine trying to find a needle in a haystack, but instead of just one needle, you have thousands! That's essentially what researchers face when sifting through vast amounts of genetic data. Yet, with the power of computational tools, this once-daunting task has become more manageable and efficient. By harnessing machine learning algorithms and high-throughput sequencing technologies, scientists are now able to analyze complex genetic information quickly and accurately.
One of the most significant breakthroughs in genomics is the ability to identify mutations that contribute to various diseases. For instance, through the use of bioinformatics, researchers can compare genetic sequences from healthy individuals to those with specific diseases. This comparative analysis allows them to pinpoint mutations that may be responsible for conditions like cancer or hereditary disorders. The implications of this are profound; not only does it enhance our understanding of disease mechanisms, but it also opens doors for developing targeted therapies.
Consider the following key applications of computational biology in genomics:
- Genome Annotation: Computational tools help in predicting the functions of genes and their products, which is crucial for understanding biological processes.
- Variant Calling: This involves identifying genetic variants from sequencing data, which is essential for personalized medicine.
- Population Genomics: By analyzing genetic data across populations, researchers can uncover insights into evolutionary biology and human migration patterns.
Furthermore, the integration of big data analytics has revolutionized how researchers approach genomic studies. With the ability to process and analyze large datasets, scientists can now uncover patterns and correlations that were previously hidden. This has led to the discovery of new biomarkers for diseases, enabling earlier diagnosis and better treatment options.
Moreover, the collaboration between computational biologists and experimental scientists has become increasingly vital. By combining computational predictions with laboratory experiments, researchers can validate their findings and refine their hypotheses. This synergy not only accelerates the pace of discovery but also enhances the reliability of the results.
As we move forward, the applications of computational biology in genomics will continue to expand. With ongoing advancements in technology and methodology, we can expect to see even more innovative solutions to complex biological questions. The future of genomics is bright, and computational biology is at the heart of this exciting journey.
- What is computational biology? Computational biology is an interdisciplinary field that uses computational techniques to analyze and interpret biological data.
- How does computational biology impact genomics? It enables researchers to analyze large genetic datasets efficiently, leading to discoveries about disease mechanisms and potential treatments.
- What technologies are used in computational biology? Key technologies include machine learning, artificial intelligence, and high-throughput sequencing.
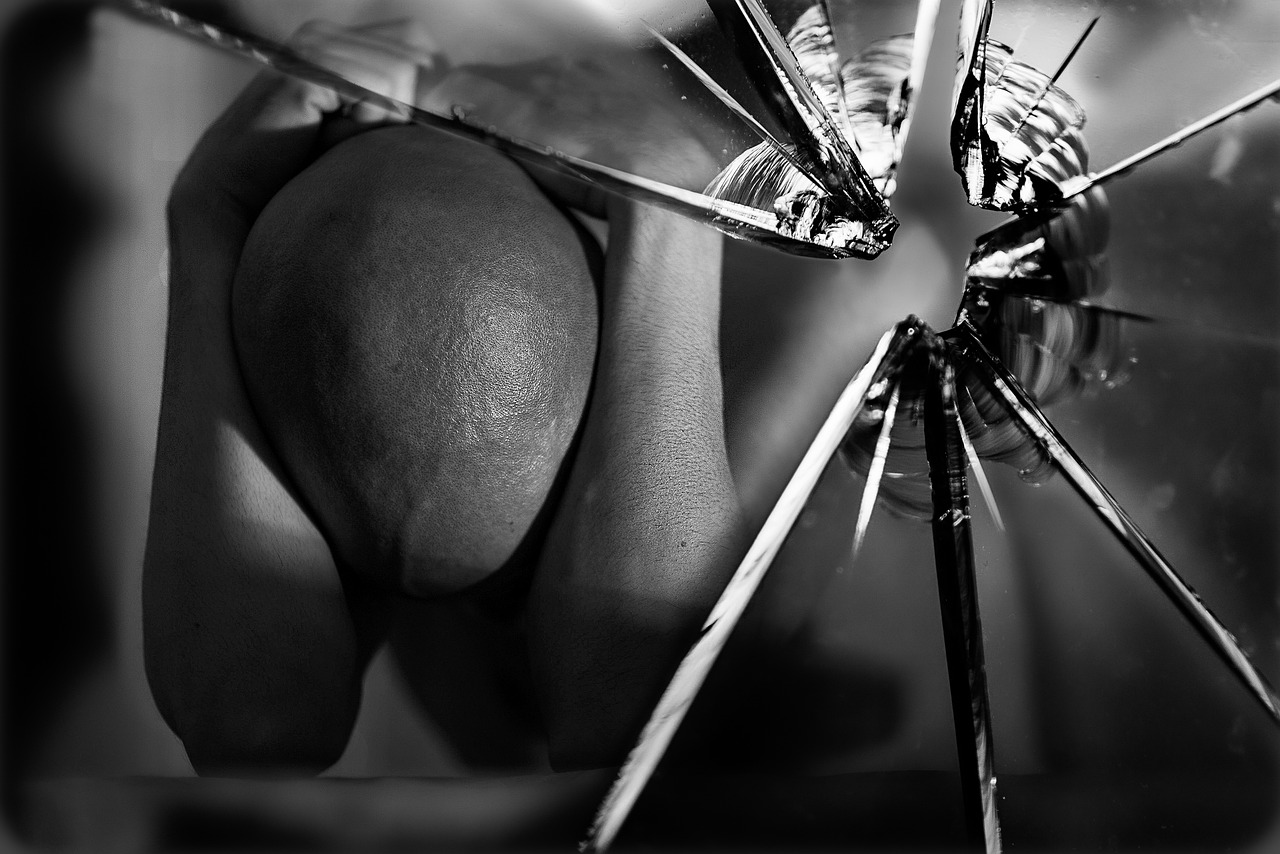
Drug Discovery Innovations
In the fast-paced world of pharmaceuticals, drug discovery has undergone a remarkable transformation thanks to advancements in computational biology. Traditionally a lengthy and costly process, drug discovery now leverages cutting-edge technologies to enhance efficiency and effectiveness. Imagine a world where researchers can predict how a molecule will behave in the human body before even synthesizing it in the lab. This is not science fiction; it’s the reality brought about by computational innovations!
One of the key innovations driving this revolution is molecular modeling. By using computational models, scientists can simulate the interactions between drug candidates and their biological targets. This process significantly reduces the time and resources needed for experimental testing. For instance, using computer-aided drug design (CADD), researchers can identify the most promising compounds from vast libraries of potential drugs, effectively narrowing down candidates for further testing. This not only accelerates the discovery process but also minimizes the chances of failure in later stages.
Moreover, the integration of machine learning algorithms has opened new avenues for drug discovery. These algorithms can analyze complex biological data to identify patterns and correlations that might not be apparent to human researchers. For example, machine learning can predict which drug compounds are likely to succeed based on historical data from previous studies. This predictive capability is invaluable, as it allows for more informed decision-making and smarter allocation of resources.
Another exciting development is the application of high-throughput screening techniques, which enable researchers to rapidly test thousands of compounds simultaneously. When combined with computational methods, high-throughput screening can lead to the identification of effective drug candidates in a fraction of the time it would take using traditional methods. This synergy between computational tools and laboratory techniques is revolutionizing the pace at which new therapies are developed.
To illustrate the impact of these innovations, consider the following table that outlines some of the key technologies in drug discovery:
Technology | Description | Impact |
---|---|---|
Molecular Modeling | Simulates molecular interactions to predict drug efficacy. | Reduces time and costs in identifying drug candidates. |
Machine Learning | Analyzes data to identify patterns and predict outcomes. | Enhances decision-making and resource allocation. |
High-Throughput Screening | Tests thousands of compounds at once. | Accelerates the identification of potential drugs. |
In addition to these technologies, computational biology also facilitates the optimization of drug candidates. Once potential drugs are identified, computational tools can help refine their chemical structures to enhance efficacy and reduce side effects. This iterative process of design, test, and refine is crucial in developing safe and effective medications.
However, it’s essential to acknowledge that while these innovations are groundbreaking, they also come with challenges. The reliance on computational models necessitates a robust understanding of biological systems, and the accuracy of predictions can vary based on the quality of data and algorithms used. Researchers must remain vigilant in validating their findings through experimental methods to ensure that computational predictions translate into real-world results.
In conclusion, the innovations in drug discovery driven by computational biology are not just enhancing the speed and efficiency of developing new therapies; they are also paving the way for more personalized treatments. As we continue to explore the potential of these technologies, the future of drug discovery looks promising, with the potential to revolutionize healthcare as we know it.
Q1: How does computational biology impact drug discovery?
A1: Computational biology enhances drug discovery by using technologies like molecular modeling and machine learning to predict drug interactions and streamline the identification of potential drug candidates.
Q2: What is high-throughput screening?
A2: High-throughput screening is a method that allows researchers to test thousands of compounds simultaneously to identify effective drug candidates quickly.
Q3: Are there challenges associated with computational drug discovery?
A3: Yes, challenges include the need for high-quality data, the accuracy of computational models, and the requirement for validation through experimental methods.
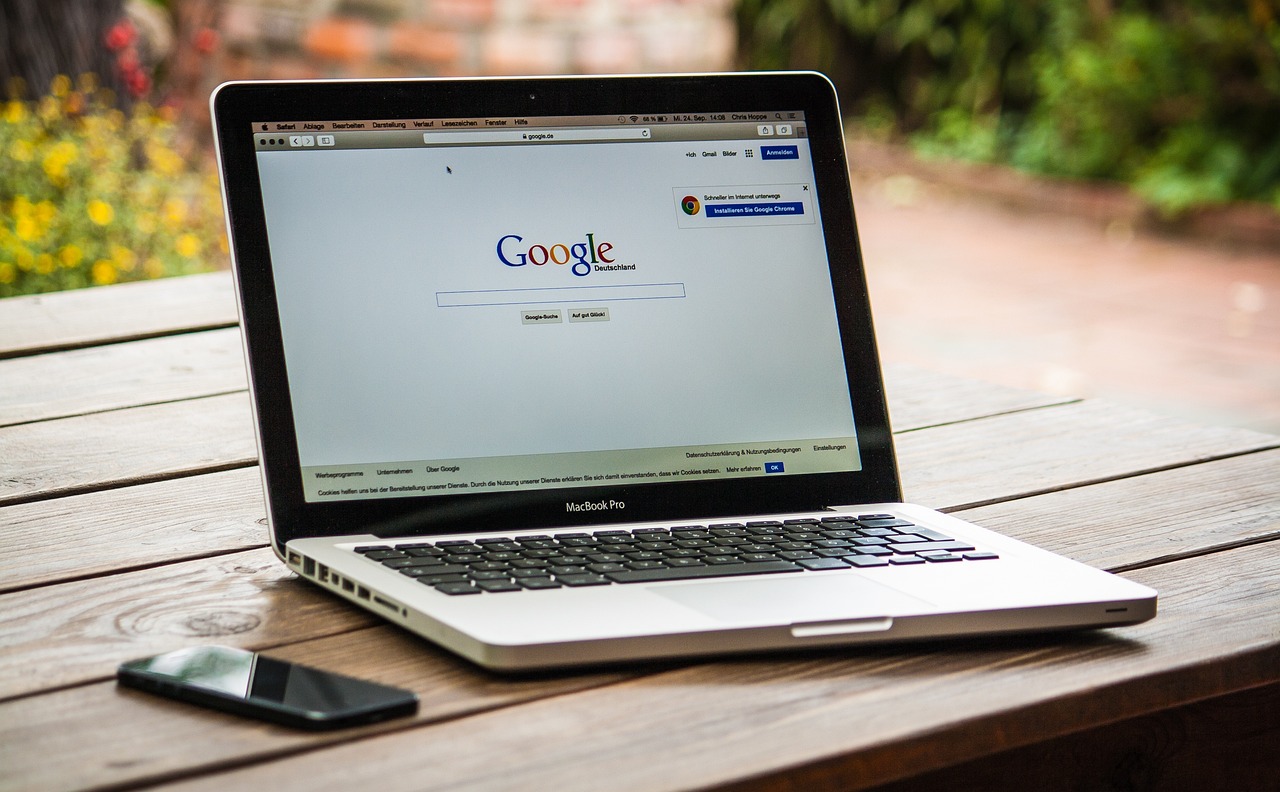
Personalized Medicine Approaches
In the ever-evolving landscape of healthcare, personalized medicine stands out as a revolutionary approach that tailors medical treatment to the individual characteristics of each patient. Imagine a world where your healthcare is as unique as your DNA; this is the promise of personalized medicine. By leveraging the power of computational biology, researchers and clinicians are now able to analyze vast amounts of genetic data, paving the way for treatments that are not just effective but also specifically designed for you.
At the heart of personalized medicine is the concept of genomic profiling. This involves sequencing a patient’s genome to identify specific genetic variations that may influence their response to certain medications or their susceptibility to particular diseases. For instance, individuals with a specific mutation in the BRCA1 gene may be at a higher risk for breast cancer, and understanding this can lead to proactive measures, including tailored screening protocols and preventive treatments.
Moreover, the integration of machine learning algorithms in analyzing genomic data allows for the identification of patterns that were previously undetectable. These algorithms can sift through millions of data points, uncovering correlations between genetic markers and treatment outcomes. This means that doctors can predict which therapies will be most effective for their patients, significantly improving the chances of successful treatment.
Another exciting aspect of personalized medicine is the development of targeted therapies. Unlike traditional treatments that often take a one-size-fits-all approach, targeted therapies are designed to attack specific molecular targets associated with certain diseases. For example, in cancer treatment, drugs like Herceptin are used specifically for patients whose tumors overexpress the HER2 protein. This not only enhances the efficacy of the treatment but also minimizes side effects, leading to a better overall patient experience.
Furthermore, personalized medicine extends beyond just genetics. It encompasses a wide range of factors, including lifestyle, environment, and even microbiome composition. By considering these elements, healthcare providers can develop comprehensive treatment plans that address the whole person rather than just the disease. This holistic approach is crucial in managing chronic conditions such as diabetes or heart disease, where lifestyle modifications play a significant role in treatment success.
To illustrate the impact of personalized medicine, let's take a look at a few key examples:
Disease | Genetic Marker | Personalized Treatment |
---|---|---|
Breast Cancer | BRCA1/BRCA2 | Preventive mastectomy or targeted therapies |
Non-Small Cell Lung Cancer | EGFR mutations | EGFR inhibitors like Erlotinib |
Melanoma | BRAF mutations | BRAF inhibitors such as Vemurafenib |
As we look to the future, the potential of personalized medicine continues to expand. The integration of big data analytics and artificial intelligence will drive even greater advancements, enabling healthcare providers to refine their approaches further. However, it’s essential to navigate this exciting terrain with caution, considering the ethical implications and ensuring that patient privacy is always a priority.
In summary, personalized medicine represents a paradigm shift in healthcare, moving towards a model that recognizes the uniqueness of each patient. By harnessing the insights provided by computational biology, we stand on the brink of a new era in medicine—one where treatments are not just prescribed but are tailored to fit the intricate tapestry of our individual biological makeup.
- What is personalized medicine?
Personalized medicine is an approach that tailors medical treatment to the individual characteristics of each patient, often based on genetic information. - How does genomic profiling work?
Genomic profiling involves sequencing a patient’s DNA to identify genetic variations that may affect their response to medications or disease susceptibility. - What are targeted therapies?
Targeted therapies are drugs designed to specifically target molecular changes in certain diseases, leading to more effective and less harmful treatments. - What role does computational biology play in personalized medicine?
Computational biology helps analyze complex biological data, allowing for insights that inform personalized treatment plans.
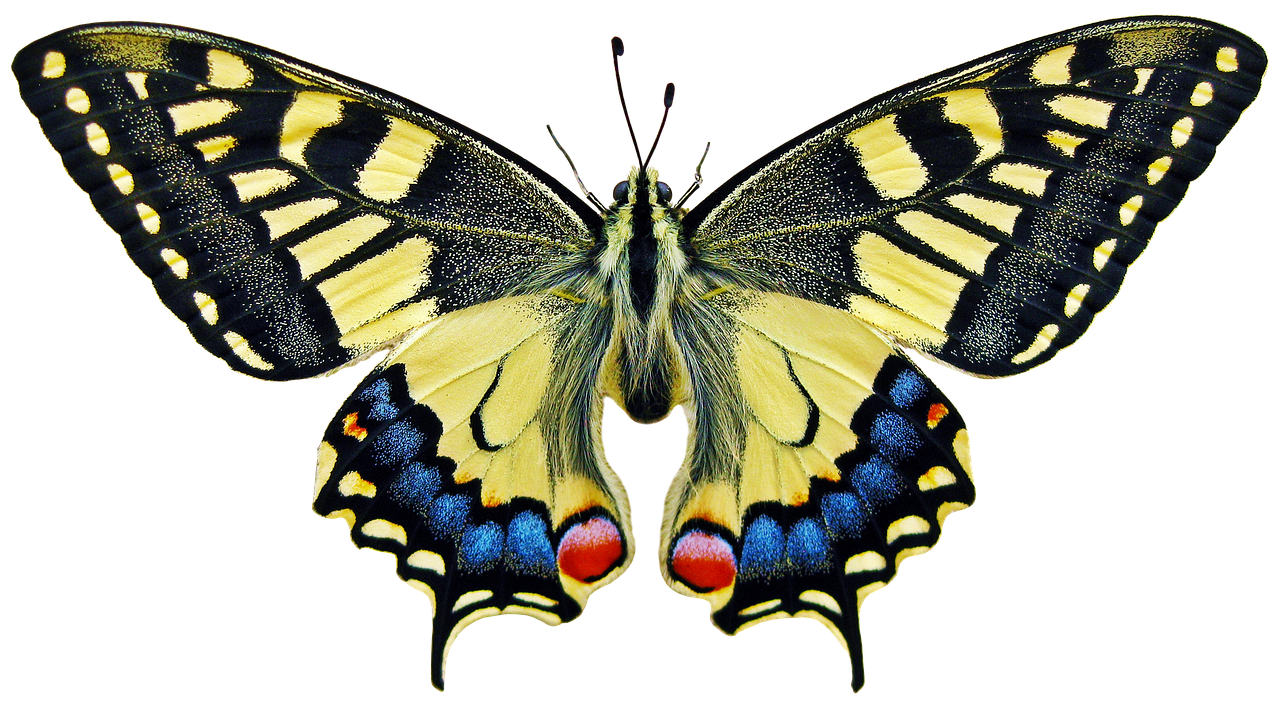
Systems Biology and Network Analysis
Systems biology is like the orchestra of biological research, where each instrument plays a unique role, yet they harmonize to create a beautiful symphony of life. This field focuses on the complex interactions within biological systems, emphasizing how various components—genes, proteins, and metabolites—work together to influence cellular functions. By leveraging computational models, researchers can visualize these interactions, leading to a deeper understanding of cellular processes and disease mechanisms.
At the heart of systems biology lies network analysis. Imagine a city map, where each intersection represents a gene and the roads signify the interactions between them. By studying these networks, scientists can identify critical pathways that may lead to diseases, much like tracing the routes of traffic jams to discover the cause of congestion. This approach has proven invaluable in unraveling the complexities of diseases such as cancer, where multiple genes and pathways are involved.
One of the most exciting aspects of systems biology is its ability to integrate data from various sources. For instance, researchers can combine genomic data with proteomic and metabolomic data to create a comprehensive picture of a biological system. This integration allows for a more holistic view, enabling scientists to pinpoint how changes in one component can ripple through the entire system. Such insights are crucial for developing targeted therapies and understanding the underlying mechanisms of diseases.
To illustrate the impact of systems biology, consider the following table, which summarizes key applications in different areas of research:
Application Area | Key Insights | Impact |
---|---|---|
Cancer Research | Identifying tumorigenic pathways | Enhanced targeted therapies |
Cardiovascular Diseases | Understanding heart function and failure mechanisms | Improved prevention strategies |
Neurobiology | Mapping neural networks and their functions | Advancements in treating neurological disorders |
Moreover, systems biology facilitates the development of predictive models that can simulate biological processes. This capability is akin to having a crystal ball that helps researchers foresee how a biological system will respond to various stimuli, such as drugs or environmental changes. By predicting outcomes, scientists can design more effective experiments and therapies, ultimately accelerating the pace of discovery.
Despite its potential, systems biology also faces challenges. Data integration remains a significant hurdle, as researchers must navigate the complexities of combining diverse datasets while ensuring accuracy and reliability. Furthermore, the computational resources required for large-scale simulations can be daunting, often necessitating collaborations with computational scientists and engineers.
In conclusion, systems biology and network analysis are transforming our understanding of biological systems. By embracing a holistic approach and utilizing advanced computational tools, researchers are uncovering the intricate webs of life, paving the way for groundbreaking discoveries and innovations in healthcare.
- What is systems biology? Systems biology is an interdisciplinary field that focuses on the complex interactions within biological systems, integrating data from various sources to understand cellular functions and disease mechanisms.
- How does network analysis contribute to systems biology? Network analysis helps visualize and understand the interactions between genes and proteins, identifying critical pathways that influence biological processes and diseases.
- What are the challenges faced in systems biology? Key challenges include data integration, computational resource limitations, and the need for standardized methodologies to ensure the reliability of results.
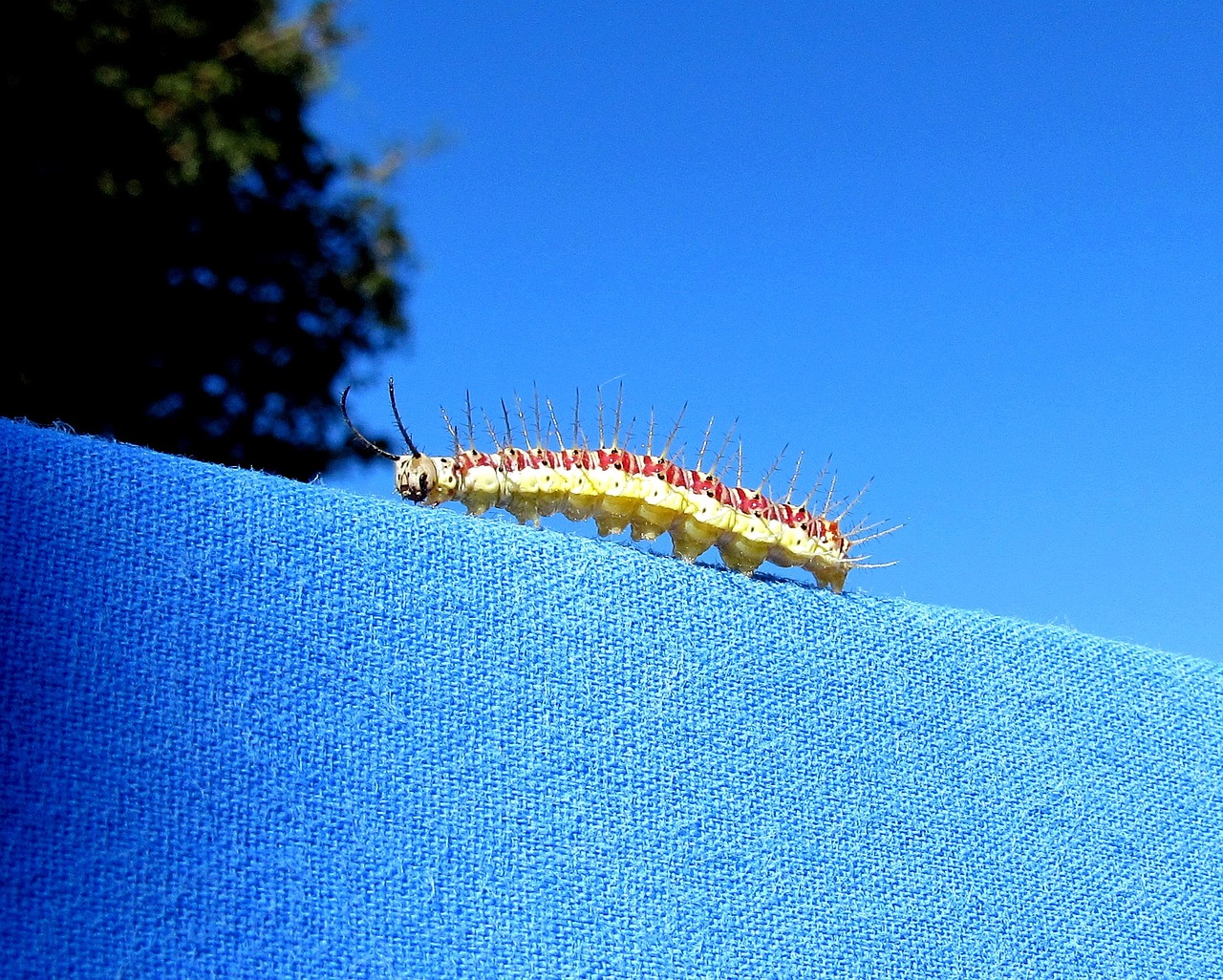
Challenges in Computational Biology
As the field of computational biology continues to evolve, it faces a myriad of challenges that can impede progress and innovation. One of the most pressing issues is the sheer volume of data being generated. With advancements in technologies like high-throughput sequencing, researchers are now able to collect vast amounts of genetic information. However, managing, analyzing, and interpreting this data can be a daunting task. The complexity of biological systems means that simple analyses often fall short, necessitating the development of advanced computational techniques.
Another significant challenge lies in data integration. In computational biology, researchers often work with data from various sources, including genomics, proteomics, and metabolomics. Each of these data types may come from different platforms and formats, making it difficult to create a cohesive analysis. The lack of standardized methodologies further complicates this issue, leading to inconsistencies and potential errors in research findings.
Additionally, computational resource limitations pose a substantial barrier. High-performance computing is essential for processing large datasets and running complex simulations. Unfortunately, not all research institutions have access to the necessary computational power. This disparity can create a gap in research capabilities, where only well-funded labs can fully exploit the potential of computational biology.
Moreover, there is a growing need for reproducibility and reliability in computational research. As the field progresses, ensuring that results can be replicated is crucial. The introduction of new algorithms and models, while exciting, can lead to variability in outcomes. Researchers must be diligent in documenting their methodologies and sharing their code to foster a culture of transparency and reproducibility.
In summary, while computational biology holds incredible potential for advancing our understanding of biological processes, it is not without its challenges. Addressing issues related to data management, integration, computational resources, and reproducibility will be essential for the field to reach its full potential. By overcoming these hurdles, researchers can pave the way for groundbreaking discoveries that could revolutionize medicine and biotechnology.
- What are the main challenges in computational biology? The main challenges include data volume, data integration, computational resource limitations, and the need for reproducibility.
- How can researchers address data integration issues? Researchers can adopt standardized methodologies and utilize advanced software tools designed for data integration.
- Why is reproducibility important in computational biology? Reproducibility ensures that research findings are reliable and can be validated by others, which is critical for scientific advancement.
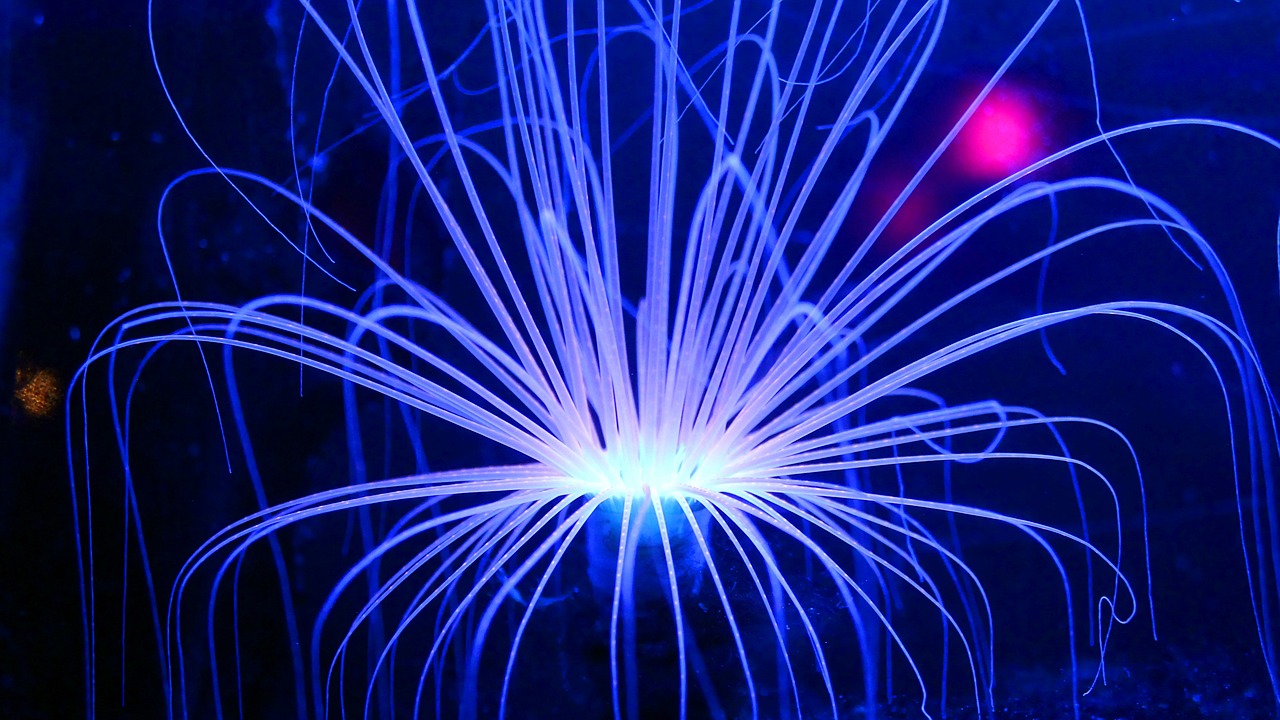
Future Trends and Directions
As we stand at the crossroads of biological research and computational technology, the future of computational biology appears incredibly promising. With the rapid pace of innovation, several trends are shaping the landscape, pushing the boundaries of what we know and can achieve in this field. One of the most exciting trends is the integration of big data analytics. As more biological data is generated, the ability to analyze this data efficiently becomes paramount. Researchers are now employing advanced algorithms to sift through massive datasets, revealing insights that were previously hidden in the noise.
Another significant direction is the advancement of bioinformatics tools. These tools are evolving to become more user-friendly and accessible, allowing researchers from various backgrounds to harness the power of computational biology without needing a deep understanding of programming or complex algorithms. This democratization of technology means that more scientists can contribute to the field, leading to a surge in innovative solutions and discoveries.
Moreover, the potential for interdisciplinary collaborations is greater than ever. As computational biology intersects with fields like artificial intelligence, engineering, and social sciences, we can expect groundbreaking innovations. For instance, the use of machine learning in predicting disease outcomes or understanding biological processes is becoming increasingly common. This synergy not only enhances research outcomes but also fosters a culture of collaboration that can lead to unexpected breakthroughs.
To illustrate these trends, consider the following table that summarizes the key areas of development:
Trend | Description |
---|---|
Big Data Analytics | Utilizing advanced algorithms to analyze vast datasets for hidden insights. |
Bioinformatics Tools | Creating user-friendly tools that enable broader participation in research. |
Interdisciplinary Collaborations | Bringing together diverse fields to foster innovative solutions and discoveries. |
Additionally, we are witnessing a shift towards more personalized approaches in computational biology. With the growing emphasis on precision medicine, researchers are utilizing computational tools to tailor treatments based on individual genetic profiles. This not only enhances the effectiveness of therapies but also minimizes adverse effects, making healthcare more efficient and patient-centered.
As we look to the horizon, it is essential to remain aware of the ethical implications that accompany these advancements. The responsible use of data, ensuring privacy, and maintaining consent are critical as we navigate this new terrain. The balance between innovation and ethics will define the success and acceptance of computational biology in the future.
In conclusion, the future of computational biology is not just about technological advancements; it’s about how these innovations will transform research, healthcare, and our understanding of life itself. As we embrace these changes, we can anticipate a world where computational biology plays a pivotal role in solving some of humanity's most pressing challenges.
- What is computational biology? Computational biology is an interdisciplinary field that uses computational techniques to analyze and model biological data.
- How does computational biology impact healthcare? It enables personalized medicine, improves drug discovery, and enhances our understanding of genetic diseases.
- What are the key technologies used in computational biology? Key technologies include machine learning, artificial intelligence, and high-throughput sequencing.
- What are the ethical considerations in computational biology? Ethical considerations include data privacy, informed consent, and the responsible use of genetic information.

Ethical Considerations in Computational Biology
As we dive deeper into the world of computational biology, the ethical implications of its advancements become increasingly significant. With the ability to analyze genetic data and manipulate biological systems, researchers must tread carefully to navigate the moral landscape that accompanies these powerful tools. Have you ever considered the potential consequences of unlocking the secrets hidden in our DNA? The implications stretch far beyond the laboratory, impacting society at large.
One of the primary concerns in computational biology is data privacy. As genetic information becomes more accessible, the risk of misuse escalates. Imagine a world where your genetic data is used without your consent, leading to discrimination in healthcare, employment, or insurance. This is not just a hypothetical scenario; it raises real questions about who owns genetic information and how it can be used. To address these concerns, researchers and institutions must prioritize the protection of individual privacy and establish clear guidelines for data usage.
Another pressing issue is the question of informed consent. When individuals participate in research studies, they often provide genetic material without fully understanding how their data will be used. This lack of transparency can lead to ethical dilemmas, especially when findings are used in ways that participants did not anticipate. It's crucial for researchers to communicate clearly about the potential uses of genetic data and to ensure that participants are fully informed before giving their consent.
Moreover, the concept of responsible innovation comes into play. As computational biology continues to evolve, researchers must consider the broader societal implications of their work. For instance, how do we ensure that advancements in personalized medicine benefit everyone, rather than just a select few? The potential for creating a divide between those who can afford cutting-edge treatments and those who cannot is a concern that cannot be overlooked. Ethical frameworks must be established to guide research practices and ensure equitable access to innovations.
Lastly, we must also consider the implications of genetic manipulation. Techniques such as CRISPR have revolutionized the field, allowing scientists to edit genes with unprecedented precision. However, this power comes with ethical responsibilities. What are the long-term effects of altering genetic material? How do we prevent the misuse of these technologies for non-therapeutic enhancements? These questions highlight the need for ongoing dialogue among scientists, ethicists, and the public to navigate the complex terrain of genetic engineering.
In summary, as computational biology advances, so do the ethical considerations that accompany it. Addressing issues of data privacy, informed consent, responsible innovation, and genetic manipulation is essential for fostering a research environment that respects individual rights and promotes societal well-being. The future of computational biology holds great promise, but it is our responsibility to ensure that this promise is realized ethically and equitably.
- What is data privacy in computational biology? Data privacy refers to the protection of personal genetic information from unauthorized access and misuse.
- Why is informed consent important? Informed consent ensures that participants understand how their genetic data will be used, allowing them to make educated decisions about their involvement in research.
- What are the risks of genetic manipulation? Genetic manipulation poses risks such as unintended consequences on health and the environment, as well as ethical concerns regarding its application.
- How can we ensure equitable access to advancements in personalized medicine? Establishing ethical frameworks and policies that promote accessibility and affordability is crucial to prevent disparities in healthcare.
Frequently Asked Questions
- What is computational biology?
Computational biology is an interdisciplinary field that uses computational techniques and models to understand biological systems and relationships. It plays a crucial role in analyzing complex biological data, such as genomic sequences and protein structures, to gain insights into various biological processes.
- How has computational biology impacted genomics?
Computational biology has revolutionized genomics by enabling researchers to process and analyze vast amounts of genetic data quickly. With advanced algorithms and machine learning, scientists can identify mutations, study gene functions, and understand the genetic basis of diseases more effectively than ever before.
- What role does machine learning play in drug discovery?
Machine learning is a game-changer in drug discovery. It helps researchers predict how potential drug candidates will behave in the human body, optimizing their development. By analyzing existing data, machine learning can identify promising compounds and streamline the entire drug discovery process.
- Can computational biology contribute to personalized medicine?
Absolutely! Computational biology allows for the design of personalized treatment plans based on individual genetic profiles. By analyzing a patient's genetic information, healthcare providers can tailor therapies that are more effective and have fewer side effects, ultimately improving patient outcomes.
- What are some challenges faced in computational biology?
Researchers in computational biology face several challenges, including data integration from diverse sources, limitations in computational resources, and the need for standardized methodologies. Addressing these issues is crucial for ensuring the reliability and reproducibility of research findings.
- What future trends can we expect in computational biology?
Future trends in computational biology include the integration of big data analytics, advancements in bioinformatics tools, and increased interdisciplinary collaborations. These trends are set to drive innovation and enhance our understanding of complex biological systems.
- What ethical considerations arise from advancements in this field?
As computational biology advances, ethical considerations such as data privacy, consent issues, and the responsible use of genetic information become increasingly important. Researchers must navigate these challenges to ensure ethical practices in both research and clinical applications.