How Artificial Intelligence is Enhancing Medical Imaging
In the ever-evolving landscape of healthcare, artificial intelligence (AI) is emerging as a game changer, particularly in the realm of medical imaging. Imagine a world where diagnoses can be made faster, more accurately, and with less human error. That world is not far off, thanks to the groundbreaking advancements in AI technology. By leveraging sophisticated algorithms and deep learning techniques, AI is transforming how medical professionals interpret images, ultimately improving patient outcomes. This article delves into the incredible role AI plays in medical imaging, exploring its advancements, applications, and the implications for both healthcare professionals and patients.
Recent breakthroughs in AI algorithms have revolutionized the field of medical imaging. These advancements have led to significant improvements in the accuracy and efficiency of image analysis, enabling healthcare providers to make faster diagnoses. For instance, AI systems can now analyze medical images with remarkable precision, identifying subtle changes that may be overlooked by the human eye. This enhanced capability not only speeds up the diagnostic process but also ensures better patient outcomes. Imagine a radiologist being able to focus more on patient interaction while AI handles the heavy lifting of image analysis. The synergy between AI and human expertise is paving the way for a new era in healthcare.
AI is truly revolutionizing radiology by acting as a powerful assistant to radiologists. By reducing the likelihood of human error and increasing productivity, AI tools are streamlining workflows in radiology departments. This means that radiologists can spend less time on routine image assessments and more time on complex cases that require their expertise. For example, AI can automatically flag potential issues in images, allowing radiologists to prioritize their workload effectively. The result? Enhanced patient care and a more efficient healthcare system.
At the forefront of AI applications in medical imaging are deep learning techniques. These methods allow AI systems to recognize intricate patterns in medical images, which significantly enhances diagnostic accuracy across various medical conditions. With deep learning, AI can learn from vast datasets, continually improving its performance as it analyzes more images. This is akin to a student who becomes more proficient in a subject by studying a wide range of materials. The more data AI has, the better it becomes at identifying anomalies and assisting in diagnoses.
One of the most effective tools in this arsenal is the Convolutional Neural Network (CNN). CNNs are designed specifically for image processing tasks, enabling the identification of anomalies in medical images with remarkable precision and speed. They work by mimicking the way humans perceive visual information, making them incredibly adept at detecting even the slightest irregularities. This capability is crucial in fields like oncology, where early detection of tumors can significantly impact treatment outcomes.
Another exciting development is transfer learning, which allows AI models to leverage existing knowledge from one domain to improve performance in medical imaging. This approach drastically reduces the need for extensive labeled datasets, which can be time-consuming and expensive to create. By utilizing pre-trained models, AI can quickly adapt to new imaging tasks, making it a valuable asset in various medical fields.
Despite the promising benefits, the implementation of AI in medical imaging is not without its challenges. Issues such as data privacy, integration with existing systems, and the need for regulatory approval must be addressed. For instance, the sensitive nature of medical data requires stringent security measures to protect patient information. Additionally, healthcare facilities must ensure that AI systems can seamlessly integrate into their current workflows to maximize efficiency. Overcoming these hurdles is essential to fully harness AI's potential in medical imaging.
AI technologies are increasingly being employed for early disease detection, enhancing the ability to identify conditions like cancer, cardiovascular diseases, and neurological disorders through advanced imaging techniques. The speed and accuracy of AI-driven analysis can lead to earlier interventions, which is crucial for improving patient survival rates.
In the field of oncology, AI plays a pivotal role in the early detection of tumors. By improving imaging analysis, AI allows healthcare providers to identify cancerous growths at an earlier stage, leading to timely interventions and personalized treatment plans. This not only enhances the chances of survival but also contributes to more effective management of the disease.
AI's role in cardiovascular imaging involves analyzing heart images to detect abnormalities, streamline diagnosis, and assist in the management of heart diseases more effectively. With AI, cardiologists can quickly assess the condition of the heart and identify potential issues before they escalate into serious problems. This proactive approach to healthcare is changing the way we think about disease management.
The future of AI in medical imaging promises continued advancements, with potential innovations in real-time imaging analysis, predictive analytics, and enhanced patient engagement through interactive technologies. As AI continues to evolve, we can expect even more sophisticated tools that will further improve diagnostic capabilities and patient care.
- What is AI in medical imaging? AI in medical imaging refers to the use of artificial intelligence technologies to analyze and interpret medical images, improving diagnostic accuracy and efficiency.
- How does AI improve patient outcomes? By providing faster and more accurate diagnoses, AI helps healthcare providers to initiate timely treatments, which can significantly enhance patient outcomes.
- What are the challenges of implementing AI in healthcare? Challenges include data privacy concerns, integration with existing systems, and the need for regulatory approval.
- What is deep learning in medical imaging? Deep learning is a subset of AI that uses neural networks to recognize patterns in large datasets, improving the accuracy of image analysis.
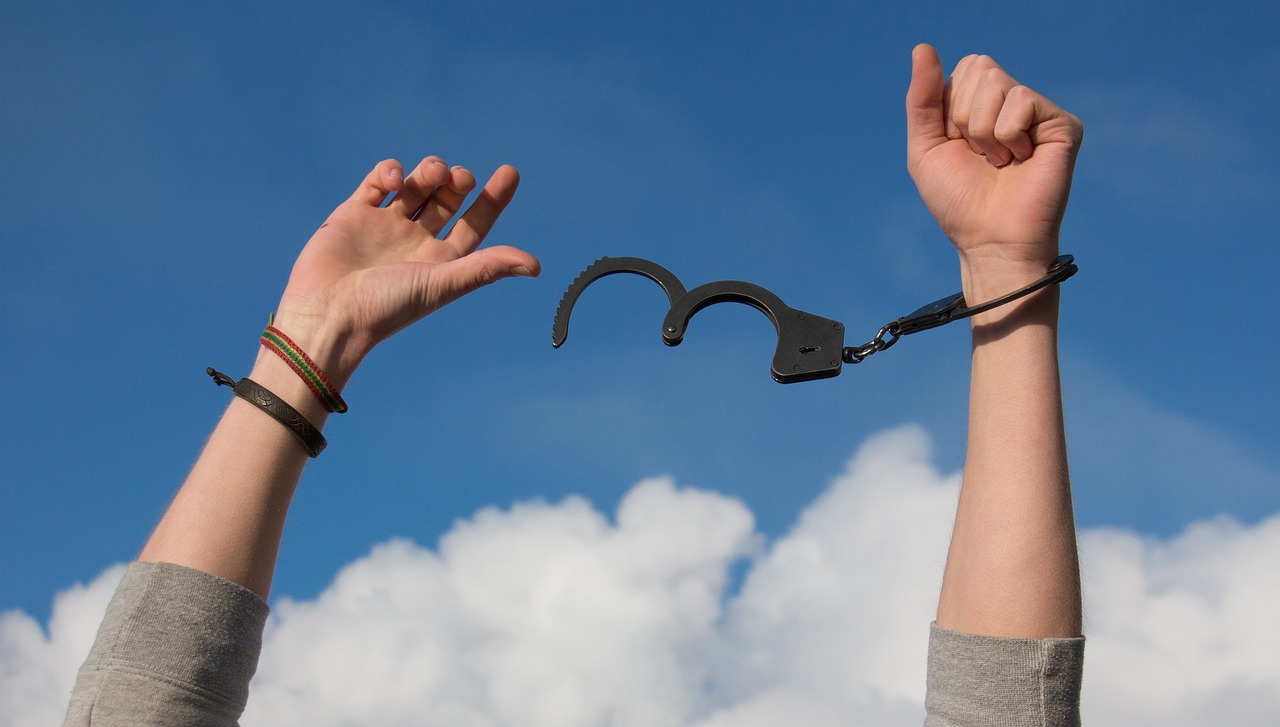
Advancements in AI Algorithms
Recent breakthroughs in artificial intelligence (AI) algorithms have significantly improved the accuracy and efficiency of medical imaging. Imagine a world where a doctor can diagnose a condition faster than ever before, thanks to the power of AI. This is not just a dream; it’s becoming a reality. Advanced algorithms are now capable of analyzing vast amounts of imaging data with incredible speed and precision, enabling healthcare professionals to make informed decisions quickly. The implications for patient outcomes are profound, as timely diagnoses can lead to earlier interventions and better treatment plans.
One of the most exciting advancements in AI algorithms is the use of machine learning techniques, particularly in the realm of image analysis. These algorithms can learn from previous data, improving their performance over time. For instance, when trained on thousands of medical images, AI systems can identify patterns that may be invisible to the human eye. This capability not only enhances diagnostic accuracy but also helps in predicting the progression of diseases, allowing for proactive patient management.
Moreover, the integration of computer vision and AI has led to remarkable improvements in the interpretation of complex imaging modalities such as MRI, CT scans, and X-rays. These algorithms can segment images, identify specific structures, and even highlight areas of concern, all while reducing the workload on radiologists. In fact, studies have shown that AI can match or even surpass human experts in certain diagnostic tasks. This doesn’t mean that radiologists will be replaced; rather, they will be empowered to focus on more complex cases while AI handles the routine analysis.
To illustrate the advancements, consider the following table that summarizes key improvements in AI algorithms:
Advancement | Description | Impact on Medical Imaging |
---|---|---|
Enhanced Image Analysis | AI algorithms can analyze images with higher accuracy. | Faster and more reliable diagnoses. |
Pattern Recognition | Deep learning techniques identify complex patterns. | Improved detection of diseases. |
Automated Reporting | AI generates reports based on image findings. | Streamlined workflow for radiologists. |
These advancements are not just technical feats; they represent a shift in how healthcare professionals approach diagnostics. With AI handling the heavy lifting of image analysis, radiologists can spend more time interacting with patients and less time sifting through images. This change not only enhances productivity but also improves the overall patient experience, creating a more efficient healthcare environment.
As we continue to explore the capabilities of AI in medical imaging, it’s crucial to recognize the ongoing need for collaboration between technology developers and healthcare professionals. The ultimate goal is to create tools that genuinely assist in patient care while maintaining the human touch that is so vital in healthcare. The future is bright, and with each advancement in AI algorithms, we move closer to a world where medical imaging is not just a tool but a transformative force in healthcare.
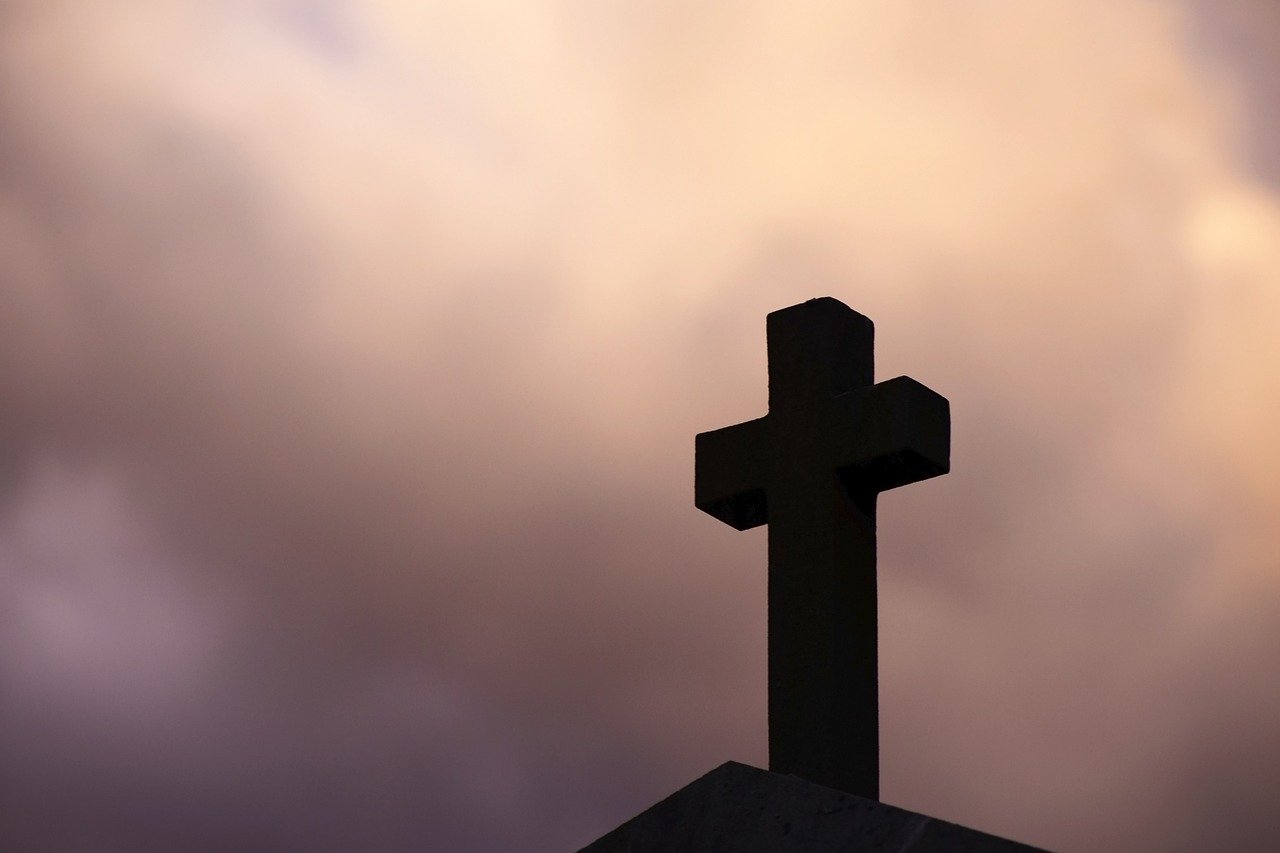
AI in Radiology
Artificial Intelligence (AI) is revolutionizing the field of radiology in ways that were once the stuff of science fiction. Imagine a world where radiologists are equipped with superhuman capabilities, able to interpret complex images in a fraction of the time it used to take. This is not just a dream; it’s becoming a reality! AI technologies are stepping in to assist radiologists, enhancing their ability to make accurate diagnoses while significantly reducing the chances of human error. This means that patients can expect faster and more reliable results, ultimately leading to improved care.
One of the most exciting aspects of AI in radiology is its ability to analyze vast amounts of data quickly. Traditional methods of image analysis can be time-consuming and prone to oversight. However, AI algorithms are designed to sift through images with remarkable speed and precision. For instance, when a radiologist examines a chest X-ray, AI can highlight potential areas of concern, such as nodules or lesions, allowing the radiologist to focus their attention where it’s needed most. This not only increases productivity but also enhances patient safety by ensuring that critical issues are not overlooked.
Furthermore, AI is not just a tool; it’s a partner in the diagnostic process. By integrating AI into their workflows, radiologists can streamline their operations. For example, AI systems can automatically categorize and prioritize cases based on urgency, ensuring that patients who need immediate attention are seen first. This efficiency is crucial in busy healthcare settings where every second counts. As a result, radiologists can spend more time on patient interaction and less time on routine tasks, fostering a more holistic approach to patient care.
However, the integration of AI in radiology does not come without its challenges. One major concern is the need for proper training and education for radiologists to effectively use these advanced tools. While AI can assist in image interpretation, it is essential for radiologists to maintain their skills and expertise. They must understand the limitations of AI and be able to critically evaluate the results it provides. This collaborative relationship between AI and human expertise is vital for achieving the best outcomes for patients.
In summary, the impact of AI on radiology is profound and far-reaching. It is transforming the way images are analyzed and diagnoses are made, leading to quicker and more accurate patient care. As AI technology continues to evolve, we can expect even more exciting advancements that will further enhance the capabilities of radiologists. The future looks bright for AI in radiology, paving the way for a healthier tomorrow.
- What is AI in radiology?
AI in radiology refers to the use of artificial intelligence technologies to assist radiologists in interpreting medical images, improving diagnostic accuracy and efficiency. - How does AI improve patient care?
AI enhances patient care by providing faster diagnoses, reducing human error, and allowing radiologists to focus on critical aspects of patient interaction. - Are radiologists still needed with AI?
Yes, radiologists are essential for interpreting results, making clinical decisions, and ensuring that AI tools are used effectively and ethically.
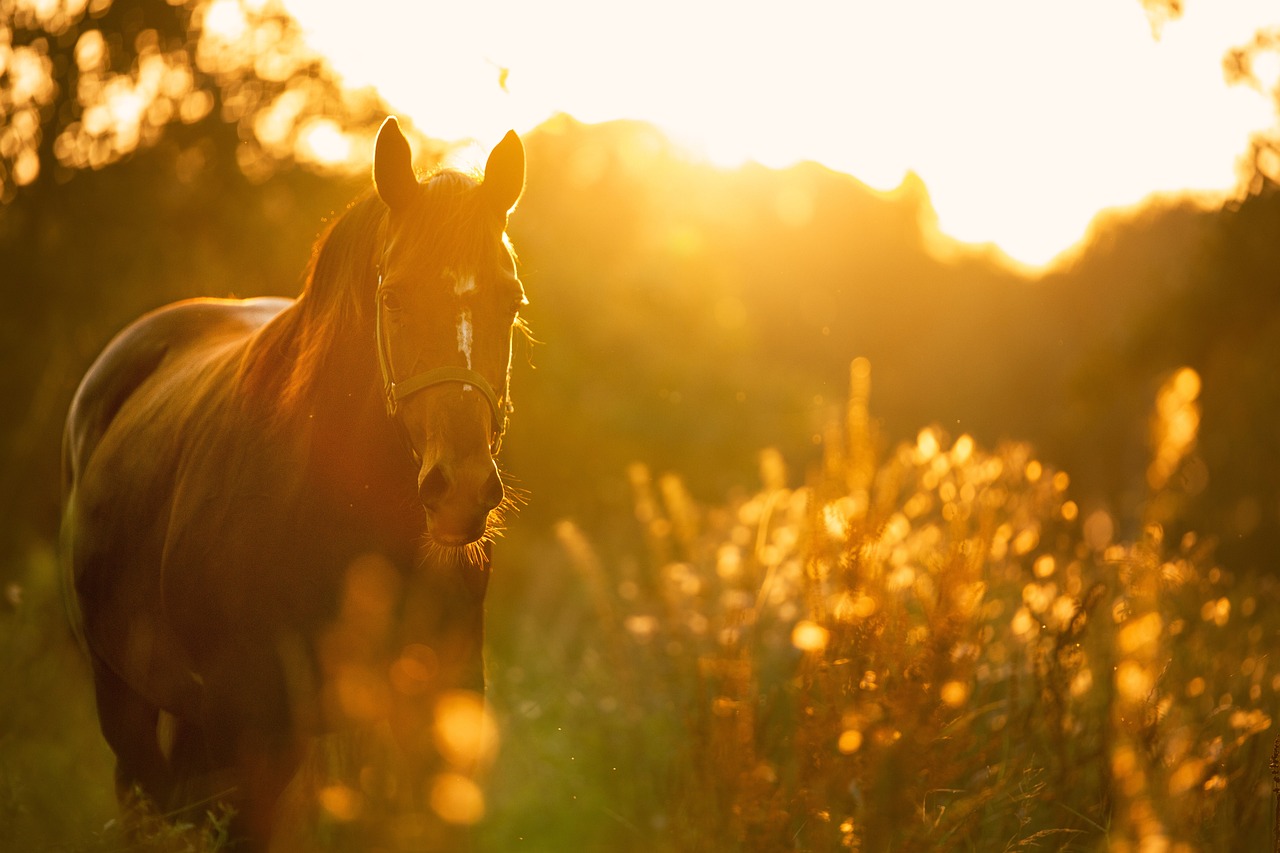
Deep Learning Techniques
Deep learning techniques are at the forefront of transforming how we approach medical imaging. Imagine a world where machines can learn to recognize patterns in medical images with the same precision as a seasoned radiologist. This is not just a dream; it's becoming a reality thanks to the power of deep learning. These techniques utilize complex algorithms that mimic the way the human brain processes information, allowing for sophisticated analysis of images that can lead to earlier and more accurate diagnoses.
One of the most significant advancements in deep learning for medical imaging is the development of Convolutional Neural Networks (CNNs). CNNs are designed to automatically and adaptively learn spatial hierarchies of features from images, making them exceptionally good at identifying anomalies. For instance, when a CNN is trained on thousands of images of healthy and diseased tissues, it can learn to differentiate between normal and abnormal patterns with remarkable accuracy. This ability to classify images not only enhances diagnostic accuracy but also dramatically speeds up the process, allowing healthcare professionals to focus more on patient care rather than image analysis.
Moreover, deep learning techniques can be applied across a variety of medical conditions. For example, in the realm of oncology, deep learning algorithms can analyze mammograms or CT scans to detect tumors at an early stage. The earlier a tumor is detected, the better the chances of successful treatment. Similarly, in cardiology, these algorithms can assess echocardiograms to identify heart abnormalities, ensuring timely intervention. The versatility of deep learning in medical imaging is truly astounding, as it can adapt to various imaging modalities, including MRI, CT, and X-ray.
However, the implementation of deep learning in medical imaging is not without its challenges. The need for extensive labeled datasets for training these algorithms can be a significant hurdle. To overcome this, techniques such as transfer learning are being employed. Transfer learning allows a model trained on one dataset to be fine-tuned for another, reducing the need for vast amounts of labeled data in medical imaging. This not only saves time but also makes it feasible to deploy deep learning models in clinical settings where data may be limited.
In summary, deep learning techniques are revolutionizing medical imaging, providing healthcare professionals with powerful tools to enhance diagnostic accuracy and improve patient outcomes. As we continue to explore and refine these technologies, the potential for deep learning to transform healthcare is limitless. The integration of such advanced techniques into everyday medical practice promises a future where diseases are detected earlier, treatments are more personalized, and patient care is significantly improved.
- What is deep learning in medical imaging?
Deep learning in medical imaging refers to the use of artificial intelligence algorithms, particularly convolutional neural networks, to analyze and interpret medical images for improved diagnosis and treatment. - How does deep learning improve diagnostic accuracy?
By using large datasets to train models, deep learning can recognize complex patterns in medical images that may be missed by human eyes, leading to more accurate diagnoses. - What are the challenges of implementing deep learning in healthcare?
Challenges include the need for large labeled datasets, concerns about data privacy, integration with existing healthcare systems, and regulatory approvals. - Can deep learning be used in all types of medical imaging?
Yes, deep learning techniques can be applied across various imaging modalities, including MRI, CT scans, X-rays, and ultrasound.
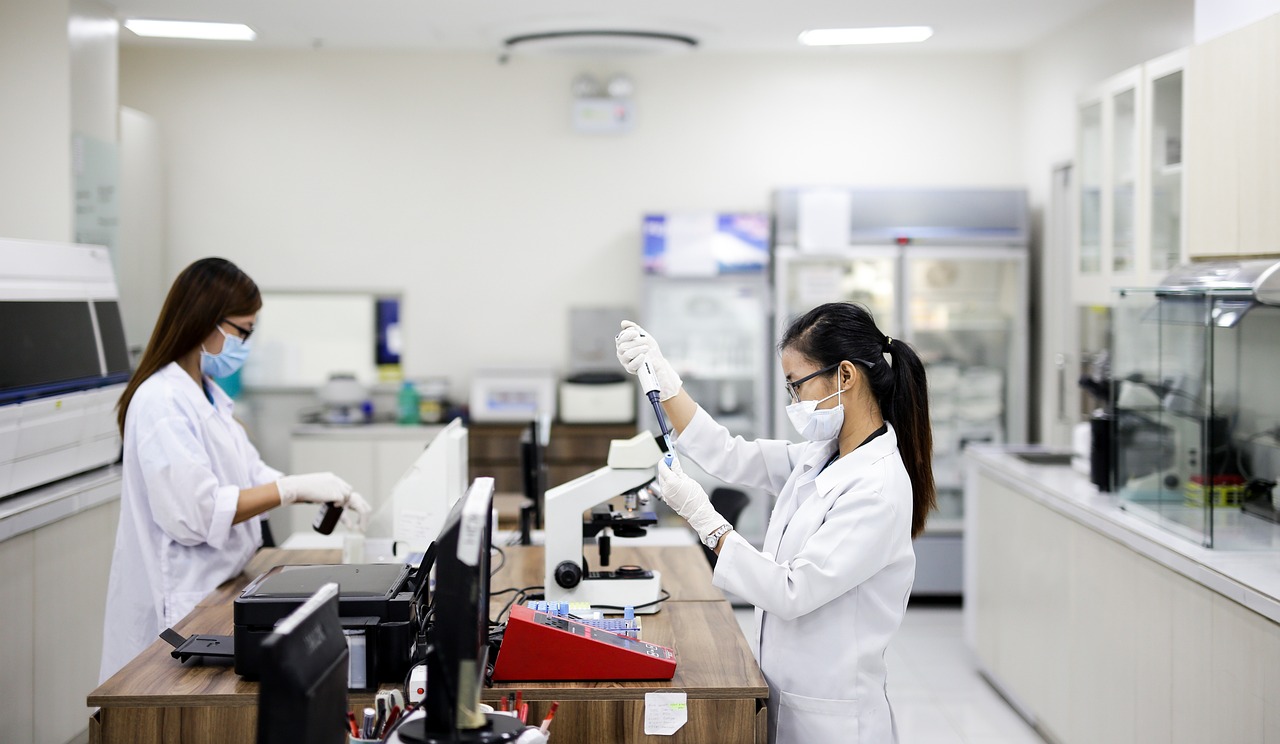
Convolutional Neural Networks
Convolutional Neural Networks, commonly known as CNNs, are a groundbreaking innovation in the realm of artificial intelligence, particularly in the field of medical imaging. These networks are designed to automatically and adaptively learn spatial hierarchies of features from images, making them incredibly effective for tasks such as image classification, object detection, and segmentation. Imagine a highly skilled radiologist who can analyze hundreds of images in a matter of seconds—this is what CNNs bring to the table!
At the core of CNNs lies the convolutional layer, which applies a series of filters to the input image. These filters are adept at recognizing patterns and features, such as edges, textures, and shapes. As the image passes through multiple layers of convolutions, the network builds a comprehensive understanding of the image content. This process is akin to peeling an onion, where each layer reveals more intricate details about the underlying structure.
One of the most remarkable aspects of CNNs is their ability to identify anomalies in medical images with astonishing precision. For instance, when diagnosing conditions like tumors or fractures, CNNs can highlight areas of concern that may be overlooked by the human eye. This capability not only enhances diagnostic accuracy but also significantly reduces the time required for analysis, allowing healthcare professionals to focus on patient care rather than sifting through images.
To illustrate the effectiveness of CNNs in medical imaging, consider the following table that outlines their key advantages:
Advantage | Description |
---|---|
High Accuracy | CNNs can achieve higher accuracy levels in image classification compared to traditional methods. |
Automation | They automate the image analysis process, reducing the workload on radiologists. |
Scalability | CNNs can be trained on large datasets, improving their performance with more data. |
Feature Learning | They automatically learn relevant features from images, eliminating the need for manual feature extraction. |
Furthermore, CNNs can be fine-tuned for specific medical imaging tasks through a process called transfer learning. This technique allows a pre-trained CNN model to adapt to new datasets with minimal additional training. For example, a CNN trained on general images can be repurposed to analyze MRI scans or X-rays, drastically reducing the time and resources needed to develop a new model from scratch.
In summary, Convolutional Neural Networks have revolutionized the way medical imaging is approached. By leveraging their ability to learn and identify complex patterns, healthcare professionals can achieve faster and more accurate diagnoses, ultimately leading to improved patient outcomes. The integration of CNNs into medical imaging workflows is not just a trend; it’s a significant leap forward in how we harness technology to enhance healthcare.
- What are Convolutional Neural Networks?
CNNs are a type of deep learning model specifically designed to process and analyze visual data. - How do CNNs improve medical imaging?
They enhance diagnostic accuracy and efficiency by automatically identifying patterns and anomalies in medical images. - Can CNNs be used for other applications?
Yes, CNNs are versatile and can be applied to various fields, including autonomous driving, facial recognition, and more. - What is transfer learning in CNNs?
Transfer learning allows a pre-trained CNN model to be adapted for new tasks, saving time and computational resources.
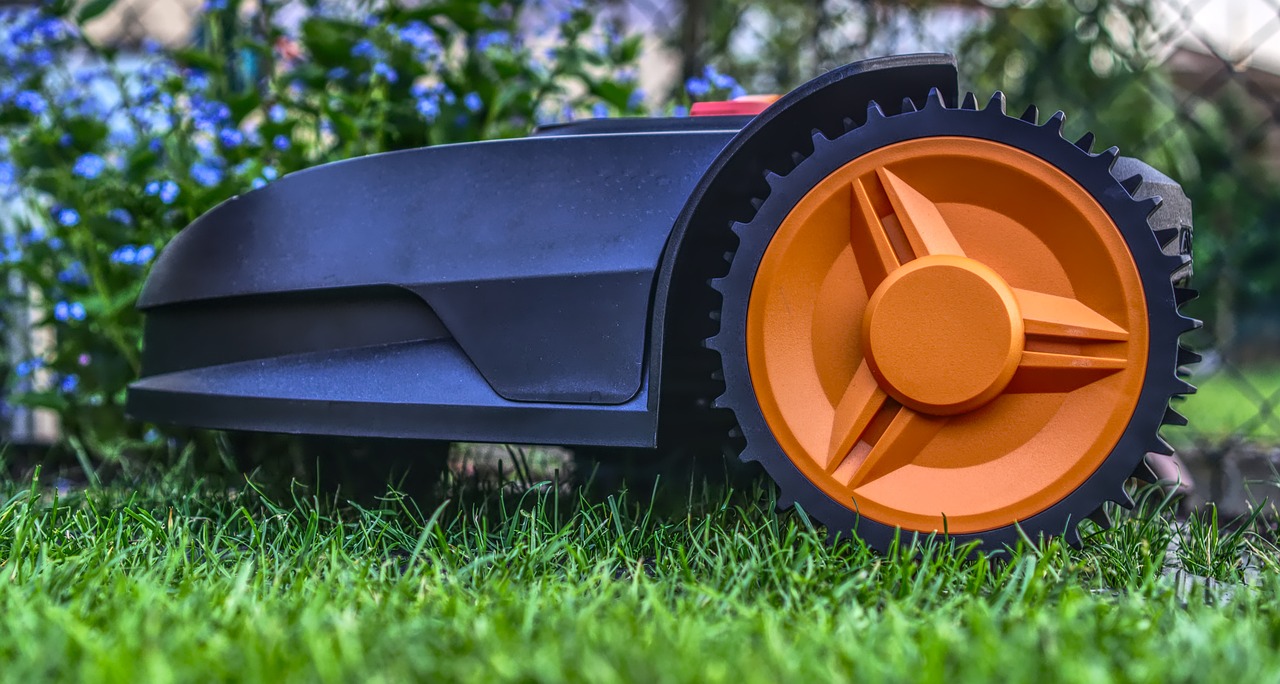
Transfer Learning Applications
Transfer learning is like having a seasoned chef teach you how to cook a gourmet meal without needing to start from scratch. In the world of artificial intelligence, particularly in medical imaging, this approach allows AI models to leverage knowledge gained from one task and apply it to another. This is especially beneficial in the medical field, where obtaining large labeled datasets can be both time-consuming and costly. By utilizing transfer learning, AI systems can achieve remarkable performance even with limited data.
For instance, imagine a model trained to identify common lung diseases using a vast dataset of chest X-rays. Once it has learned to recognize specific patterns and features, this model can then be fine-tuned for a different but related task, such as detecting early signs of pneumonia in a smaller dataset. This not only saves time but also enhances the model's accuracy and reliability in diagnosing conditions that may not have enough training data available.
One of the key benefits of transfer learning is its ability to reduce the amount of labeled data required for training. In many cases, healthcare professionals may not have access to extensive datasets due to privacy concerns or resource limitations. By applying transfer learning, AI models can be adapted to new medical imaging tasks without the need for large amounts of new data, making the technology more accessible and practical for healthcare providers.
Moreover, the applications of transfer learning in medical imaging are vast. Here are some notable areas where it's making a significant impact:
- Radiology: Transfer learning helps in adapting models trained on general imaging datasets to specific radiological tasks, improving diagnostic accuracy.
- Pathology: AI models can be fine-tuned to detect specific types of cancer in pathology slides, enhancing early detection rates.
- Ophthalmology: Models trained on retinal images can be adjusted to identify diabetic retinopathy with high precision.
In summary, transfer learning not only accelerates the development of AI applications in medical imaging but also enhances their effectiveness. By allowing models to transfer knowledge across different tasks, healthcare professionals can benefit from improved diagnostic tools that are both efficient and reliable. This innovative approach is paving the way for more personalized and timely patient care, ultimately leading to better health outcomes.
- What is transfer learning?
Transfer learning is a machine learning technique where a model developed for a particular task is reused as the starting point for a model on a second task. - How does transfer learning benefit medical imaging?
It reduces the need for large labeled datasets by allowing models to adapt knowledge from one domain to another, improving accuracy and efficiency. - Can transfer learning be applied to any medical imaging task?
While it is highly adaptable, the success of transfer learning depends on the similarity between the source and target tasks.
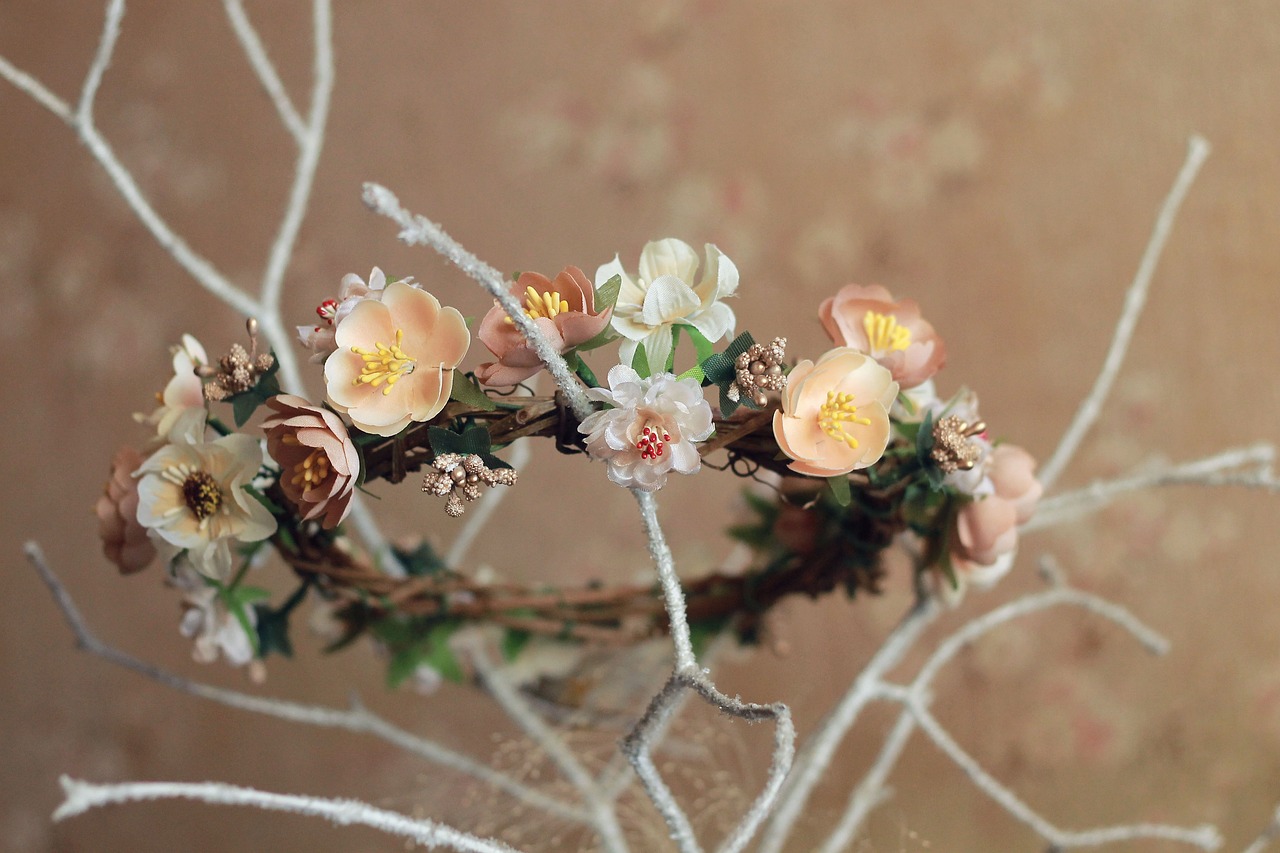
Challenges in Implementation
As promising as the integration of artificial intelligence in medical imaging may be, it’s not without its hurdles. One of the most pressing challenges is data privacy. With patient data being highly sensitive, ensuring that AI systems comply with regulations such as HIPAA in the United States is crucial. Healthcare providers must navigate a complex landscape of data protection laws while still utilizing AI tools that require access to large datasets for training and validation.
Another significant challenge is the integration of AI systems with existing medical imaging technologies. Many healthcare facilities have legacy systems that may not be easily compatible with new AI solutions. This can lead to increased costs and extended timelines for implementation, as organizations must invest in both new technology and the training of their staff to use these advanced tools effectively.
Additionally, there’s the regulatory approval process that AI technologies must undergo before they can be widely adopted in clinical settings. The path to regulatory clearance can be lengthy and complicated, often requiring extensive clinical trials to demonstrate the safety and efficacy of AI applications. This can slow down the pace at which these innovative solutions reach the market, delaying potential benefits for patients and healthcare providers alike.
Moreover, the interpretability of AI decisions raises concerns among healthcare professionals. Radiologists and clinicians need to understand the reasoning behind AI-generated recommendations to trust and effectively utilize these tools in their practice. If AI systems operate as "black boxes," it may lead to skepticism and reluctance to adopt these technologies, ultimately hindering their potential impact on patient care.
Lastly, there’s the issue of training and education. For AI to be successfully implemented in medical imaging, healthcare professionals must be equipped with the necessary skills to work alongside these technologies. This includes understanding how to interpret AI outputs and knowing when to rely on human judgment. Continuous education and training programs will be essential to bridge this knowledge gap and foster a collaborative environment where AI and human expertise coexist.
In summary, while the challenges in implementing AI in medical imaging are significant, they are not insurmountable. With concerted efforts from healthcare providers, technology developers, and regulatory bodies, the path towards a more efficient and accurate medical imaging landscape can be paved. Addressing these challenges head-on will ensure that the benefits of AI are realized, ultimately leading to improved patient outcomes and enhanced healthcare delivery.
- What are the main challenges in implementing AI in medical imaging?
Data privacy, system integration, regulatory approval, interpretability of AI decisions, and training for healthcare professionals are key challenges. - How does data privacy affect AI in healthcare?
AI systems require access to large datasets, which raises concerns about protecting sensitive patient information under various regulations. - Why is regulatory approval important for AI technologies?
Regulatory approval ensures that AI applications are safe and effective before they can be used in clinical settings. - What role does training play in AI implementation?
Training is crucial for healthcare professionals to effectively interpret AI outputs and integrate these tools into their workflows.
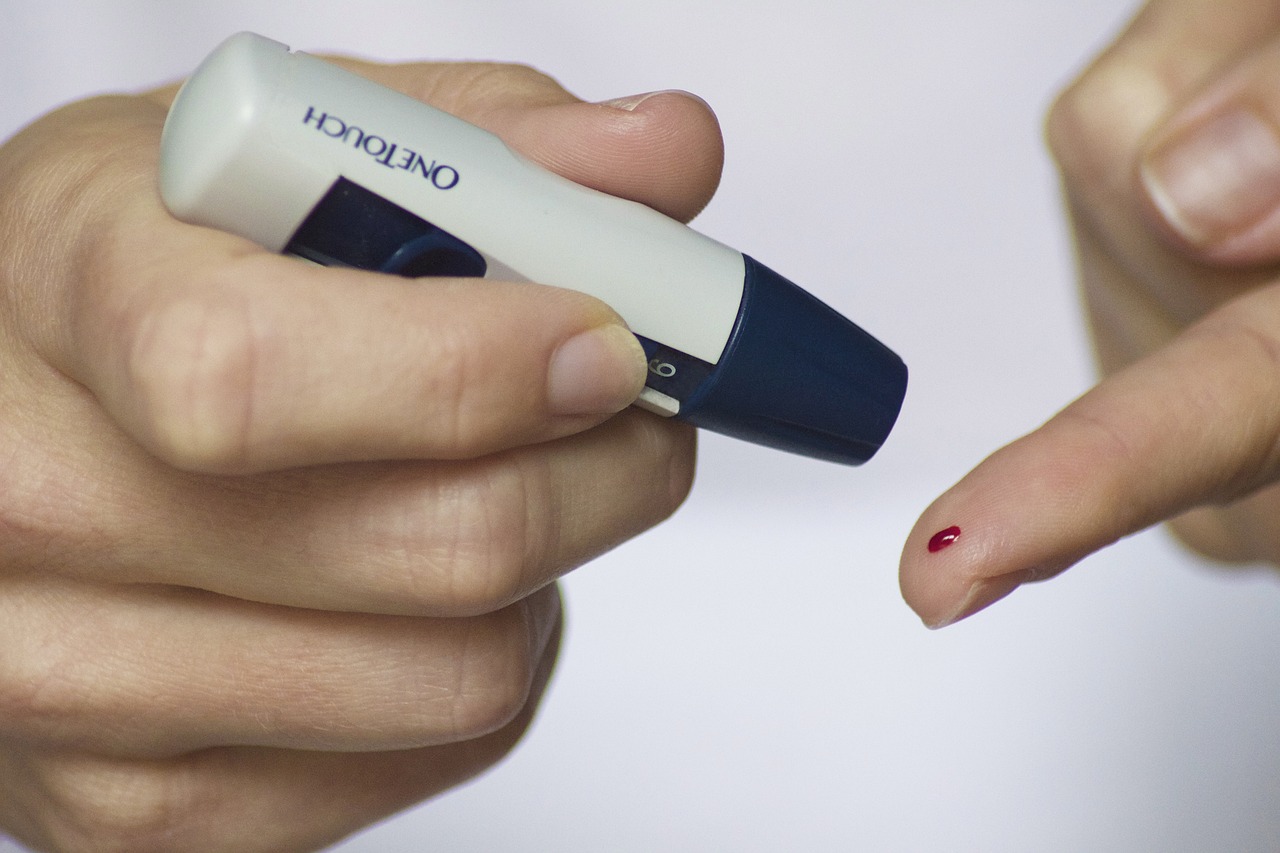
AI in Disease Detection
Artificial Intelligence (AI) technologies are increasingly making waves in the realm of disease detection, transforming how healthcare professionals identify and diagnose various conditions. Imagine having a powerful assistant that can sift through thousands of medical images in a fraction of the time it would take a human. This is precisely what AI brings to the table, enhancing the ability to pinpoint diseases like cancer, cardiovascular issues, and neurological disorders with remarkable precision.
One of the most compelling aspects of AI in disease detection is its capability for early diagnosis. Early detection is crucial in many medical scenarios, particularly in oncology where the difference between stage one and stage four cancer can be a matter of months. With AI algorithms analyzing imaging data, healthcare providers can detect tumors at a much earlier stage, leading to timely interventions and personalized treatment plans. This not only improves patient survival rates but also enhances the quality of life for many individuals.
In the field of oncology, AI applications are revolutionizing how tumors are identified. For instance, AI can analyze mammograms or CT scans, highlighting potential areas of concern that a radiologist might overlook. This technology acts as a second set of eyes, ensuring that no stone is left unturned. The integration of AI in oncology is not just about detection; it also extends to treatment planning and monitoring, helping oncologists tailor therapies to individual patients based on their unique tumor characteristics.
Similarly, in cardiovascular imaging, AI plays a pivotal role. Heart diseases are among the leading causes of death globally, making timely diagnosis and management essential. AI systems can analyze echocardiograms and MRI scans to detect abnormalities such as blockages or irregular heartbeats. By providing accurate assessments, AI helps cardiologists make informed decisions quickly, which is vital in emergency situations. Additionally, these systems can learn from past data, continuously improving their diagnostic capabilities over time.
As we move forward, the implications of AI in disease detection are profound. Consider the following key benefits:
- Increased Accuracy: AI algorithms are designed to recognize patterns that may be imperceptible to the human eye, leading to more accurate diagnoses.
- Faster Results: With AI handling the heavy lifting of image analysis, healthcare providers can receive results in real-time, expediting the decision-making process.
- Cost-Effective Solutions: By streamlining workflows and reducing the need for extensive manual reviews, AI can help lower healthcare costs.
However, it's essential to acknowledge that while AI is a powerful tool, it is not without challenges. Issues such as data privacy, the need for regulatory approval, and the integration of AI systems with existing healthcare infrastructure need to be addressed. The goal is to create a seamless experience where AI complements the skills of healthcare professionals rather than replacing them.
In conclusion, the integration of AI in disease detection is not merely a trend; it's a paradigm shift that is redefining the landscape of healthcare. As technology continues to evolve, we can expect even more innovative applications of AI, ultimately leading to better patient outcomes and a more efficient healthcare system.
Q1: How does AI improve disease detection?
A1: AI enhances disease detection by analyzing medical images with high accuracy, identifying patterns that may be missed by human eyes, and providing faster results.
Q2: Is AI replacing doctors in disease diagnosis?
A2: No, AI is not replacing doctors; rather, it is acting as a supplementary tool that aids healthcare professionals in making more informed decisions.
Q3: What are the challenges of using AI in healthcare?
A3: Some challenges include data privacy concerns, the need for regulatory approval, and ensuring seamless integration with existing healthcare systems.
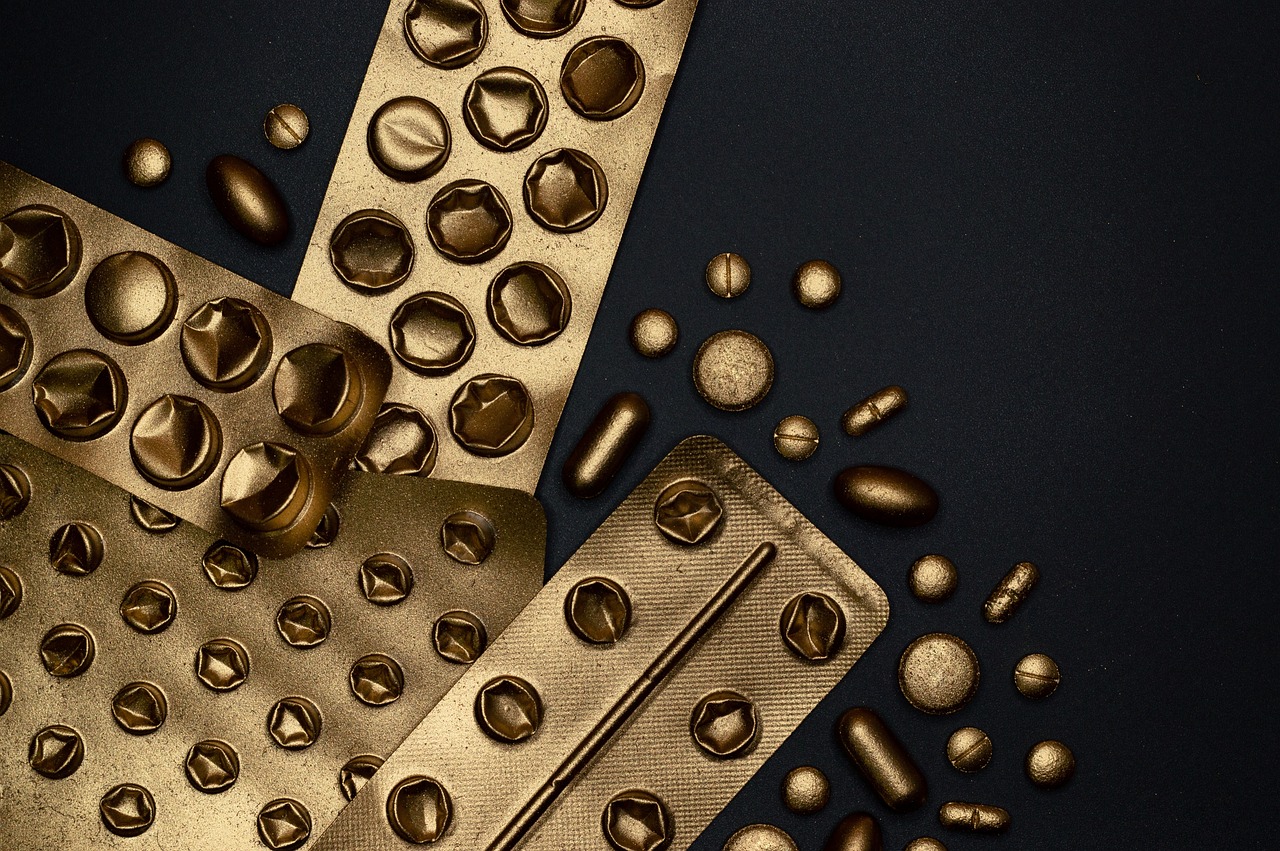
Oncology Applications
In the realm of oncology, the integration of artificial intelligence is nothing short of revolutionary. Imagine a world where detecting tumors is not only faster but also more accurate—this is the promise that AI brings to cancer diagnosis. With advanced imaging analysis, AI systems can sift through vast amounts of data, identifying patterns that might elude even the most seasoned oncologists. This capability is crucial because, in cancer treatment, every second counts. The earlier a tumor is detected, the better the chances for effective intervention and improved patient outcomes.
AI algorithms are designed to analyze medical images, such as CT scans, MRI scans, and X-rays, with remarkable precision. They can highlight suspicious areas that require further examination, thus aiding radiologists in making informed decisions. For instance, a study revealed that AI systems could identify malignant tumors with an accuracy rate of over 90%, significantly higher than traditional methods. This level of precision can lead to earlier diagnosis and treatment, which is critical in oncology where time is of the essence.
Moreover, AI's role extends beyond mere detection. It can also assist in personalizing treatment plans. By analyzing patient data and imaging results, AI can help oncologists tailor therapies to the individual needs of each patient, considering factors such as tumor type, genetic markers, and overall health. This personalized approach not only enhances the effectiveness of treatments but also minimizes side effects, making the patient experience more tolerable.
However, the journey of AI in oncology is not without its challenges. The implementation of AI systems requires comprehensive training and collaboration between technology developers and healthcare professionals. Additionally, there are concerns regarding data privacy and the ethical implications of AI decision-making in healthcare. As we navigate these challenges, it’s essential to maintain a focus on enhancing patient care while ensuring safety and efficacy.
To illustrate the impact of AI in oncology, consider the following table that summarizes key benefits:
Benefit | Description |
---|---|
Early Detection | AI enhances imaging techniques to identify tumors at an earlier stage, improving survival rates. |
Increased Accuracy | AI algorithms provide higher accuracy in detecting cancerous cells compared to traditional methods. |
Personalized Treatment | AI helps tailor treatment plans based on individual patient data and tumor characteristics. |
Reduced Workload | AI assists radiologists by automating routine tasks, allowing them to focus on complex cases. |
In conclusion, the applications of AI in oncology are paving the way for a new era in cancer care. As technology continues to evolve, we can expect even more innovative solutions that will not only enhance diagnostic capabilities but also revolutionize treatment modalities. The future of oncology is bright with AI, and it holds the potential to save countless lives.
- How does AI improve cancer detection? AI improves cancer detection by analyzing medical images with high accuracy, identifying patterns that may not be visible to the human eye.
- Can AI personalize cancer treatment plans? Yes, AI can analyze patient data and imaging results to help oncologists create personalized treatment plans tailored to individual needs.
- What are the challenges of implementing AI in oncology? Challenges include data privacy concerns, the need for regulatory approval, and ensuring seamless integration with existing healthcare systems.
- Is AI replacing oncologists? No, AI is designed to assist oncologists, not replace them. It enhances their capabilities and allows them to focus on more complex cases.
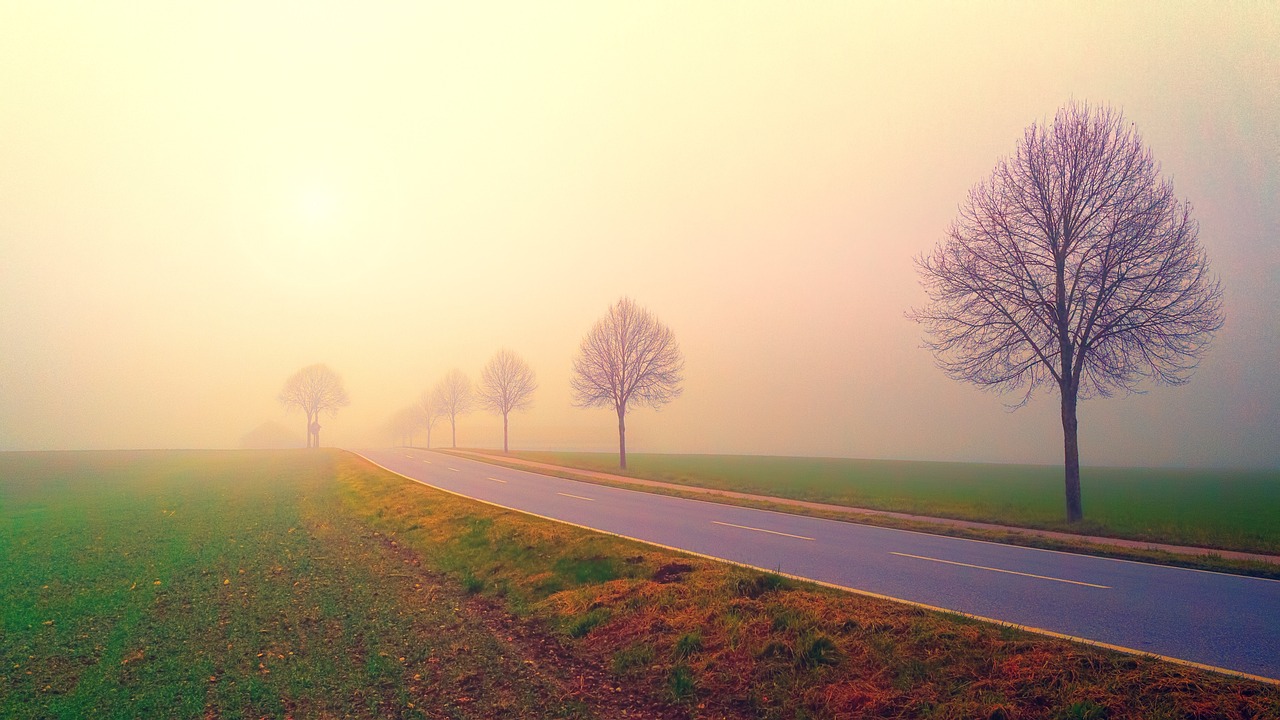
Cardiovascular Imaging
When it comes to , the integration of artificial intelligence (AI) is nothing short of revolutionary. Imagine having a highly skilled assistant that never tires, always pays attention to detail, and can analyze complex images in the blink of an eye. That's what AI brings to the table in the realm of heart health. By leveraging advanced algorithms, AI can sift through vast amounts of imaging data to detect abnormalities that might be missed by the human eye.
AI's role in cardiovascular imaging is multifaceted. It not only enhances the accuracy of diagnoses but also streamlines the workflow for healthcare professionals. With the ability to analyze echocardiograms, MRIs, and CT scans, AI tools can identify issues such as coronary artery disease, valvular heart disease, and even heart failure with remarkable precision. This means that patients can receive timely interventions, which is crucial for improving survival rates.
For instance, in a recent study, AI algorithms were able to predict cardiovascular events by analyzing heart images alongside patient history. This predictive capability is a game-changer, allowing for proactive management of heart conditions. The potential for personalized treatment plans becomes more tangible as AI continues to evolve, enabling healthcare providers to tailor interventions based on individual patient profiles.
Moreover, AI-driven cardiovascular imaging is not just about identifying existing conditions; it's also about prevention. By utilizing machine learning techniques, these systems can analyze trends and patterns in imaging data over time, helping to forecast potential risks before they escalate. For example, if a patient shows early signs of arterial plaque buildup, AI can alert healthcare providers to implement lifestyle changes or medical interventions sooner rather than later.
However, the implementation of AI in cardiovascular imaging does come with its challenges. Data privacy concerns and the need for regulatory approval are significant hurdles that must be navigated. Additionally, integrating AI tools with existing healthcare systems can be complex and requires careful planning. Yet, the benefits far outweigh these challenges, paving the way for a future where heart care is more efficient and effective.
In summary, the intersection of AI and cardiovascular imaging is transforming how we approach heart health. With ongoing advancements, we can expect to see even more innovative solutions that enhance diagnostic accuracy, improve patient outcomes, and ultimately save lives. As we look ahead, the potential for AI to revolutionize cardiovascular care is not just exciting; it's essential.
- What is cardiovascular imaging?
Cardiovascular imaging refers to various techniques used to visualize the heart and blood vessels to diagnose and monitor heart conditions. - How does AI improve cardiovascular imaging?
AI improves cardiovascular imaging by enhancing the accuracy of diagnoses, streamlining workflows, and providing predictive analytics for better patient management. - What are some common AI applications in cardiovascular imaging?
Common applications include analyzing echocardiograms, MRIs, and CT scans to detect conditions like coronary artery disease and heart failure. - What challenges does AI face in cardiovascular imaging?
Challenges include data privacy concerns, the need for regulatory approval, and the integration of AI tools with existing healthcare systems.
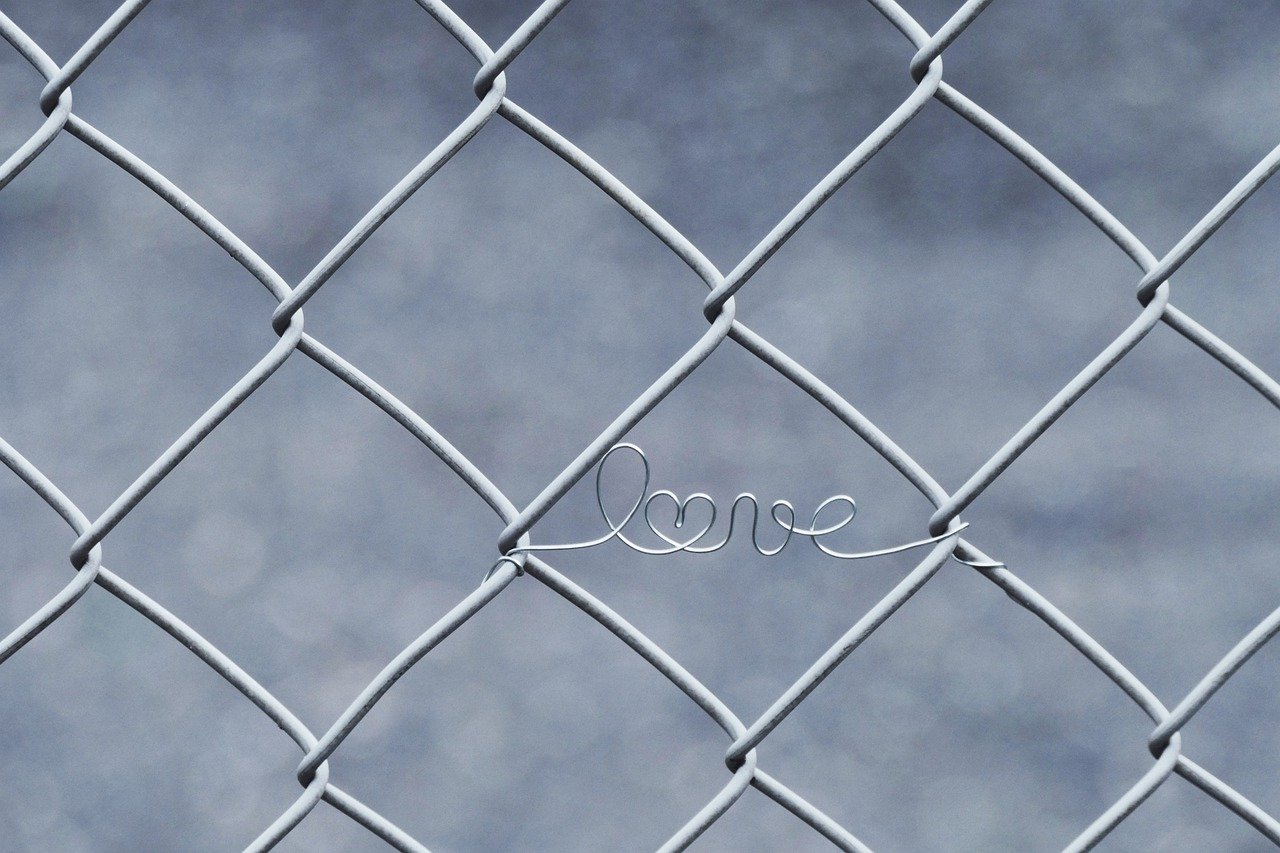
Future Trends in AI and Medical Imaging
The landscape of medical imaging is on the brink of a revolutionary transformation, largely fueled by the relentless advancements in artificial intelligence. As we look towards the future, several key trends are emerging that promise to reshape how healthcare professionals approach diagnostics and patient care. One of the most exciting prospects is the development of real-time imaging analysis. Imagine a world where radiologists can receive instant feedback on imaging results, allowing for quicker decision-making and intervention. This capability could significantly enhance the efficiency of diagnostic processes, ultimately improving patient outcomes.
Another trend to watch is the rise of predictive analytics. By harnessing vast amounts of data, AI algorithms can predict disease progression and potential health risks before they manifest. This proactive approach not only empowers healthcare providers to tailor treatment plans but also engages patients in their health journeys. The ability to foresee potential complications can lead to earlier interventions and better management of chronic conditions, which is a game-changer in patient care.
Moreover, enhanced patient engagement through interactive technologies is becoming increasingly relevant. AI-driven applications are being developed to provide patients with personalized information about their imaging results, treatment options, and health management strategies. This shift towards patient-centric care fosters a collaborative environment where patients feel more informed and involved in their healthcare decisions.
As AI continues to evolve, we can also expect to see a surge in the integration of multimodal imaging techniques. By combining various imaging modalities—such as MRI, CT, and PET scans—AI can offer a more comprehensive view of a patient's health. This holistic approach not only enhances diagnostic accuracy but also helps in understanding complex medical conditions that may not be apparent through a single imaging technique.
However, with these advancements come challenges that must be addressed. Issues related to data privacy and security remain paramount, as sensitive patient information is increasingly utilized to train AI models. Additionally, ensuring that AI systems are interoperable with existing healthcare infrastructure is crucial for seamless implementation. Regulatory hurdles also need to be navigated to ensure that AI technologies meet the necessary standards for clinical use.
In conclusion, the future of AI in medical imaging is bright, with the potential to revolutionize diagnostics and patient care. As we embrace these trends, it is essential to remain vigilant about the ethical implications and challenges that accompany such innovations. The journey ahead promises not only enhanced accuracy and efficiency but also a more personalized approach to healthcare, putting patients at the heart of the medical imaging revolution.
- What is the role of AI in medical imaging?
AI enhances the accuracy and efficiency of medical imaging by assisting in image analysis, reducing human error, and streamlining workflows. - How does real-time imaging analysis work?
Real-time imaging analysis uses AI algorithms to provide instant feedback on imaging results, allowing for quicker decision-making. - What are predictive analytics in healthcare?
Predictive analytics involve using data to forecast disease progression and potential health risks, enabling proactive patient management. - What challenges does AI face in medical imaging?
Challenges include data privacy concerns, the need for regulatory approval, and integration with existing healthcare systems.
Frequently Asked Questions
- What is the role of AI in medical imaging?
AI plays a transformative role in medical imaging by enhancing the accuracy and efficiency of image analysis. With advanced algorithms, it helps healthcare professionals make faster diagnoses, ultimately improving patient outcomes. Think of AI as a supercharged assistant that can sift through mountains of data and highlight what really matters, allowing doctors to focus on what they do best—caring for patients.
- How does AI improve the accuracy of diagnoses?
AI improves diagnostic accuracy primarily through deep learning techniques, which enable sophisticated pattern recognition. For instance, Convolutional Neural Networks (CNNs) can identify anomalies in images with remarkable precision. It's like having a highly trained eye that can spot even the tiniest irregularities that might go unnoticed by the human eye.
- What are the challenges in implementing AI in medical imaging?
While the benefits of AI in medical imaging are substantial, challenges remain. Issues like data privacy, the need for integration with existing healthcare systems, and regulatory approval can hinder widespread adoption. It's a bit like trying to fit a square peg in a round hole—there's a lot of work to be done to ensure everything aligns perfectly.
- Can AI help in early disease detection?
Absolutely! AI technologies are increasingly being used for early disease detection, particularly in areas like oncology and cardiovascular imaging. By analyzing medical images, AI can identify conditions such as tumors or heart abnormalities much earlier than traditional methods, which is crucial for timely interventions and better patient outcomes.
- What future trends can we expect in AI and medical imaging?
The future of AI in medical imaging looks promising, with potential innovations on the horizon. We can expect advancements in real-time imaging analysis, predictive analytics, and even enhanced patient engagement through interactive technologies. Imagine a world where AI not only assists doctors but also empowers patients with insights about their health—exciting, right?