Innovations in Cognitive Research - Tools and Techniques
In the ever-evolving field of cognitive research, innovations are not just welcomed; they are essential. As we delve into the intricate workings of the human mind, new tools and techniques emerge, pushing the boundaries of what we know and how we understand cognitive processes. Imagine being able to watch thoughts flicker across the brain like fireworks, or using advanced algorithms to predict how a person might respond to a particular stimulus. These advancements are not just theoretical; they are reshaping the landscape of cognitive research in profound ways.
One of the most exciting aspects of these innovations is the integration of technology into research methodologies. From neuroimaging techniques that allow us to visualize brain activity in real-time to the use of artificial intelligence that can sift through vast amounts of data with astonishing speed, the tools at our disposal today are more powerful than ever. This article will explore these groundbreaking advancements, focusing on how they enhance our understanding of cognitive functions and improve research accuracy.
As we navigate through this exploration, consider the implications of these innovations. What does it mean for our understanding of memory, perception, and decision-making? How do these tools influence the way we conduct research and interpret findings? The answers to these questions not only highlight the importance of these innovations but also underscore the exciting future of cognitive research.
In the sections that follow, we will dive deeper into specific innovations, examining neuroimaging techniques, the role of artificial intelligence, virtual reality applications, and behavioral assessment tools. Each of these elements plays a crucial role in enhancing our research methodologies and ultimately, our understanding of the human mind.
Join us as we embark on this journey through the latest advancements in cognitive research, where science meets technology, and the possibilities are as vast as the human psyche itself. With every new tool and technique, we get a little closer to unraveling the mysteries of cognition, and who knows? Perhaps the next breakthrough is just around the corner.

Neuroimaging Techniques
Neuroimaging has truly transformed the landscape of cognitive research, providing researchers with the ability to visualize brain activity in real-time. Imagine being able to peek inside the human mind while it grapples with a complex problem or experiences a moment of insight. This is the power of neuroimaging techniques, which not only enhance our understanding of cognitive processes but also pave the way for groundbreaking discoveries in psychology and neuroscience.
There are several prominent neuroimaging methods that have emerged, each with its unique strengths and applications. The most widely used techniques include:
- Functional Magnetic Resonance Imaging (fMRI) - This method measures brain activity by detecting changes in blood flow, allowing researchers to see which areas of the brain are engaged during specific tasks.
- Electroencephalography (EEG) - EEG records electrical activity in the brain through electrodes placed on the scalp, providing insights into the timing of cognitive processes.
- Positron Emission Tomography (PET) - PET scans use radioactive tracers to visualize metabolic processes in the brain, helping researchers understand how different regions respond to various stimuli.
Each of these techniques offers unique advantages. For instance, fMRI provides excellent spatial resolution, allowing researchers to pinpoint exact locations of brain activity, while EEG excels in temporal resolution, capturing the rapid electrical changes that occur in response to stimuli. The choice of neuroimaging technique often depends on the specific research question at hand. For example, if a researcher is interested in how quickly the brain responds to a stimulus, EEG might be the preferred method. Conversely, if the focus is on understanding which brain areas are involved in complex cognitive tasks, fMRI would be more suitable.
Moreover, the integration of these various neuroimaging methods can lead to richer insights. By combining fMRI and EEG, researchers can correlate the timing of brain activity with its location, providing a more comprehensive view of cognitive processes. This multimodal approach is paving the way for a deeper understanding of how our brains work, especially in complex scenarios like decision-making, memory retrieval, and emotional regulation.
As technology continues to advance, we can expect even more innovative neuroimaging techniques to emerge. For instance, researchers are exploring the use of optogenetics, which allows for the precise control of neuronal activity using light, and magnetoencephalography (MEG), which offers even greater temporal resolution than EEG. These advancements will undoubtedly enhance our ability to study the human brain and its intricate workings.
In conclusion, neuroimaging techniques are at the forefront of cognitive research, providing invaluable insights into the complexities of the human mind. As we continue to refine these tools and develop new methodologies, the potential for understanding cognitive processes expands, bringing us closer to unraveling the mysteries of how we think, learn, and interact with the world around us.

Artificial Intelligence in Cognitive Studies
Artificial intelligence (AI) is not just a buzzword; it’s a transformative force in the realm of cognitive research. As researchers dive deeper into understanding the complexities of the human mind, AI tools are becoming indispensable allies. Imagine having a super-smart assistant that can sift through mountains of data in mere seconds, uncovering patterns and insights that would take humans days or even weeks to find. This is precisely what AI brings to the table in cognitive studies.
One of the most exciting aspects of AI in cognitive research is its ability to analyze complex data sets. Traditional methods of data analysis often struggle with the sheer volume and intricacy of information generated in cognitive experiments. However, AI algorithms can handle vast amounts of data with remarkable efficiency. For instance, when researchers collect data from neuroimaging techniques, they can use AI to process this information, pinpointing specific brain activity associated with various cognitive tasks. This not only enhances the accuracy of the findings but also speeds up the research process significantly.
Moreover, AI is instrumental in simulating cognitive processes, allowing researchers to create models that mimic human thought patterns. By using advanced algorithms, scientists can predict how individuals might respond in different scenarios, providing invaluable insights into decision-making, memory, and learning. This capability is akin to having a crystal ball that offers glimpses into the workings of the human mind, making it a powerful tool for both theoretical exploration and practical application.
At the heart of AI's contribution to cognitive studies are machine learning algorithms. These algorithms are designed to learn from data, improving their accuracy over time. In cognitive research, they can identify subtle patterns in behavior and cognition that might otherwise go unnoticed. For example, researchers can use machine learning to analyze responses from standardized tests, identifying correlations between different cognitive abilities.
Here’s a quick look at how machine learning algorithms are applied in cognitive studies:
- Pattern Recognition: Algorithms can detect patterns in large datasets, helping researchers understand how different cognitive functions are interconnected.
- Predictive Modeling: By analyzing past data, machine learning can predict future cognitive outcomes, aiding in early diagnosis of cognitive impairments.
- Data Classification: Algorithms can classify data into various categories, simplifying the analysis process and enhancing the clarity of research findings.
Deep learning, a subset of machine learning, takes this a step further by utilizing neural networks that mimic the human brain's architecture. These models excel in analyzing large datasets, making them particularly effective in cognitive research. Imagine trying to decode the complexities of human emotions or decision-making processes; deep learning models can process and analyze data from multiple sources, offering a comprehensive view of cognitive functions.
For instance, researchers studying language processing can feed vast amounts of linguistic data into deep learning models, allowing them to uncover intricate relationships between language use and cognitive function. This capability is akin to having a team of researchers working simultaneously, each focusing on different aspects of the data, thereby accelerating the research process.
Natural Language Processing (NLP) is another fascinating application of AI in cognitive studies. NLP tools enable researchers to analyze linguistic data, examining how language comprehension and production relate to cognitive processes. By using NLP, researchers can explore various dimensions of language, such as syntax, semantics, and pragmatics, providing deeper insights into how language affects thought.
For example, NLP can be used to analyze transcripts from interviews or conversations, helping researchers identify common themes or cognitive patterns in how people express themselves. This analysis can reveal not just what people say, but how they think and process information, offering a window into the cognitive mechanisms at play.
In summary, the integration of artificial intelligence in cognitive studies is revolutionizing how researchers approach the complexities of the human mind. From machine learning algorithms to deep learning models and natural language processing, AI is enhancing the accuracy and efficiency of cognitive research, paving the way for groundbreaking discoveries that could change our understanding of cognition forever.
- What is the role of AI in cognitive research? AI helps analyze large datasets, simulates cognitive processes, and enhances research accuracy and efficiency.
- How do machine learning algorithms contribute to cognitive studies? They identify patterns, make predictions, and classify data, providing valuable insights into cognitive functions.
- What are deep learning models? Deep learning models are advanced algorithms that mimic human brain architecture to analyze complex datasets.
- What is Natural Language Processing? NLP is a branch of AI that focuses on the interaction between computers and human language, aiding researchers in analyzing linguistic data.

Machine Learning Algorithms
Machine learning algorithms are truly the unsung heroes of cognitive research, acting as the bridge between raw data and meaningful insights. These algorithms are designed to learn from data, identify patterns, and make predictions, which is essential in understanding the complexities of human cognition. Imagine trying to find a needle in a haystack; that's what researchers face when dealing with vast amounts of cognitive data. However, with the right machine learning tools, they can sift through this data much more efficiently, pinpointing those valuable insights that can lead to breakthroughs in our understanding of the mind.
One of the most fascinating aspects of machine learning is its ability to adapt and improve over time. Just like how we learn from our experiences, these algorithms refine their processes based on new data inputs. For instance, if a machine learning model is used to analyze responses from cognitive tests, it can adjust its predictions based on the accuracy of previous outcomes. This iterative learning process not only enhances the accuracy of research findings but also opens the door to innovative approaches in cognitive studies.
Researchers employ various types of machine learning algorithms, each suited for different tasks within cognitive research. Some of the most commonly used algorithms include:
- Supervised Learning: This involves training a model on a labeled dataset, where the outcomes are known. It’s particularly useful for classification tasks, such as predicting whether a subject may have a cognitive impairment based on test scores.
- Unsupervised Learning: Unlike supervised learning, this method deals with unlabeled data. It helps researchers uncover hidden patterns or groupings in cognitive data, such as clustering similar cognitive profiles.
- Reinforcement Learning: This type of learning is based on the idea of agents making decisions in an environment to maximize rewards. In cognitive research, it can simulate decision-making processes and predict behavioral outcomes.
Moreover, the integration of machine learning algorithms with neuroimaging techniques has been a game-changer. By applying these algorithms to neuroimaging data, researchers can decode brain activity patterns associated with various cognitive functions. For example, a recent study utilized machine learning to analyze fMRI data, successfully predicting subjects' cognitive states based on their brain activity. This not only demonstrates the power of machine learning but also highlights its potential to uncover the intricate workings of the human brain.
As we look to the future, the role of machine learning in cognitive research is set to expand even further. With advancements in computational power and the availability of larger datasets, researchers will be able to explore more complex models and algorithms. This evolution promises to enhance our understanding of cognitive processes and may even lead to the development of personalized cognitive assessments tailored to individual needs.
Q: What are the benefits of using machine learning in cognitive research?
A: Machine learning offers improved accuracy in data analysis, the ability to handle large datasets, and the capacity to uncover patterns that may not be immediately obvious through traditional methods.
Q: How does supervised learning differ from unsupervised learning?
A: Supervised learning uses labeled data to train models, while unsupervised learning works with unlabeled data to find hidden structures or patterns.
Q: Can machine learning predict cognitive decline?
A: Yes, machine learning algorithms can analyze various data points to identify early signs of cognitive decline, helping in timely interventions.

Deep Learning Models
Deep learning models are a groundbreaking advancement in the realm of cognitive research, offering unparalleled capabilities for understanding the complexities of neural networks. These models, inspired by the structure and function of the human brain, consist of layers of interconnected nodes that can learn from vast amounts of data. Imagine a web of neurons firing in response to stimuli; that's essentially how deep learning operates, mimicking the way our brains process information.
One of the most exciting aspects of deep learning is its ability to handle large datasets. Traditional methods often struggle to extract meaningful insights from the overwhelming volume of data generated in cognitive studies. In contrast, deep learning models excel at identifying patterns and correlations that might go unnoticed by the human eye. For instance, researchers can input thousands of brain scans into a deep learning model, allowing it to learn the subtle differences between various cognitive states. This capability not only enhances the accuracy of research findings but also accelerates the pace of discovery.
Moreover, deep learning models are particularly adept at feature extraction. This means they can automatically identify which aspects of the data are most relevant for a specific cognitive task without requiring extensive manual input. For example, when analyzing brain activity during language comprehension tasks, a deep learning model can pinpoint which neural pathways are activated, providing researchers with insights into the cognitive processes involved. This level of analysis was previously unimaginable and opens up new avenues for understanding the intricacies of human cognition.
To illustrate the impact of deep learning models on cognitive research, consider the following table that summarizes some of their key advantages:
Advantage | Description |
---|---|
Data Handling | Can process and analyze vast datasets efficiently. |
Pattern Recognition | Identifies complex patterns and correlations in data. |
Feature Extraction | Automatically detects relevant features for cognitive tasks. |
Predictive Power | Can make predictions about cognitive states based on input data. |
In addition to their advantages, deep learning models also face challenges. For instance, they require substantial computational power and can be difficult to interpret. Researchers often refer to this issue as the "black box" problem, where the decision-making process of the model is not easily understood. However, ongoing advancements in explainable AI are working to address these concerns, making deep learning models not just powerful but also more transparent.
In conclusion, deep learning models are revolutionizing cognitive research by enabling scientists to delve deeper into the workings of the human mind. With their ability to analyze large datasets, recognize complex patterns, and provide insights into neural processes, these models are paving the way for a new era of cognitive understanding. As technology continues to evolve, we can only anticipate even greater breakthroughs in how we study and comprehend cognition.
- What are deep learning models? Deep learning models are algorithms that simulate the workings of the human brain to analyze complex data sets and identify patterns.
- How do deep learning models benefit cognitive research? They enhance data analysis, improve accuracy, and provide insights into neural processes that were previously difficult to obtain.
- What challenges do deep learning models face? They require significant computational resources and can be difficult to interpret, leading to the "black box" problem.

Natural Language Processing
Natural Language Processing (NLP) is an exciting field that bridges the gap between human language and computer understanding. In cognitive research, NLP tools are revolutionizing the way we examine language comprehension and production. Imagine trying to explain the nuances of human speech to a machine that only understands binary code—it's a daunting task! Yet, with advancements in NLP, researchers are making significant strides in decoding the complexities of language.
One of the primary applications of NLP in cognitive studies is analyzing vast amounts of linguistic data. This can include everything from social media posts to academic articles. By employing sophisticated algorithms, researchers can identify patterns in language use, detect sentiment, and even predict language development in individuals. For instance, if you were to analyze tweets about a trending topic, an NLP model could help categorize the emotional tone of those tweets, providing insights into public sentiment.
Moreover, NLP is not just about understanding language on a surface level; it delves deeper into how humans produce and comprehend language in real-time. By using techniques such as text mining and semantic analysis, researchers can explore how people structure their thoughts and communicate them effectively. This has profound implications for understanding cognitive processes like memory, attention, and even social interactions.
To illustrate the impact of NLP in cognitive research, consider the following table that summarizes some of its key applications:
Application | Description |
---|---|
Sentiment Analysis | Evaluates emotions expressed in text to understand public opinion. |
Language Modeling | Predicts the likelihood of a sequence of words, aiding in speech recognition. |
Text Classification | Categorizes text into predefined groups, useful for organizing research data. |
Chatbots and Virtual Assistants | Simulates human conversation, providing insights into language processing. |
As we continue to explore the depths of human cognition, NLP stands out as a powerful ally. It not only enhances our understanding of language but also sheds light on the underlying cognitive mechanisms that drive communication. With ongoing advancements in this field, we can expect to see even more innovative applications that will further illuminate the intricate relationship between language and cognition.
- What is Natural Language Processing? NLP is a branch of artificial intelligence that focuses on the interaction between computers and humans through natural language.
- How is NLP used in cognitive research? NLP is used to analyze and interpret linguistic data, helping researchers understand language comprehension and production.
- What are some applications of NLP? Applications include sentiment analysis, language modeling, text classification, and the development of chatbots.
- Why is NLP important for understanding cognition? NLP provides insights into how language is processed and produced, revealing cognitive mechanisms involved in communication.

Virtual Reality Applications
Virtual reality (VR) is not just for gaming anymore; it's making waves in the realm of cognitive research, offering an immersive experience that traditional methods simply can't match. Imagine stepping into a virtual world where researchers can simulate environments and situations tailored to test cognitive functions. This technology allows participants to engage with tasks in a way that feels natural and intuitive, making the data collected not only more reliable but also more insightful.
One of the most exciting aspects of VR in cognitive research is its ability to create controlled yet dynamic environments. Researchers can manipulate variables with ease, from altering the layout of a virtual room to changing the complexity of a task. For example, a study might involve navigating a virtual maze while measuring attention levels or memory recall. This level of control helps scientists isolate specific cognitive processes, leading to a better understanding of how the brain operates in various scenarios.
Moreover, VR can enhance participant engagement. Traditional testing methods often lead to boredom, which can skew results. In contrast, VR offers an interactive experience that captivates participants. This engagement is crucial, especially when studying populations that may find conventional methods challenging, such as children or individuals with cognitive impairments. By immersing these groups in a virtual environment, researchers can obtain more accurate data and insights.
Another major advantage of VR is its adaptability. Researchers can design experiments that cater to specific hypotheses or questions. For instance, consider the following applications:
- Memory Studies: Participants can be placed in a virtual setting that mimics real-life scenarios to study how memories are formed and recalled.
- Attention and Focus: VR can simulate distractions in a controlled environment to assess how attention shifts and how individuals manage cognitive load.
- Emotional Responses: By recreating emotionally charged situations, researchers can study how different environments affect emotional regulation and decision-making.
Additionally, VR can be used for training purposes. For example, it can simulate high-pressure situations that require quick thinking and problem-solving, providing a safe space for participants to practice and refine their skills. This application is particularly beneficial in fields such as psychology, where understanding real-world applications of cognitive theories is vital.
As VR technology continues to advance, we can expect even more innovative applications in cognitive research. The integration of VR with other technologies, such as artificial intelligence and neuroimaging, could lead to groundbreaking discoveries about the human mind. By leveraging these tools, researchers can create a holistic view of cognitive processes, paving the way for enhanced treatment options and interventions for cognitive disorders.
Q: How does virtual reality differ from traditional cognitive research methods?
A: VR offers an immersive and interactive environment that allows for greater control over variables and enhances participant engagement, unlike traditional methods that may be more static and less engaging.
Q: Can VR be used for all types of cognitive research?
A: While VR is versatile, it may not be suitable for every type of research. However, it is particularly effective for studies involving memory, attention, and emotional responses.
Q: What are the potential drawbacks of using VR in cognitive research?
A: Some drawbacks include the cost of VR equipment, potential motion sickness for participants, and the need for specialized training to design and implement VR studies effectively.
Q: How is data collected in VR studies?
A: Data can be collected through various means, including participant responses, biometric measurements, and real-time tracking of movements and interactions within the virtual environment.

Behavioral Assessment Tools
Behavioral assessment tools are absolutely vital in the realm of cognitive research. They serve as the measuring sticks for various cognitive functions, helping researchers gain a clearer picture of how individuals think, learn, and behave. Imagine trying to navigate through a dense fog without a map; that’s what cognitive researchers face without effective assessment tools. These tools not only quantify cognitive abilities but also provide insights into emotional and behavioral patterns. In recent years, innovations in technology have led to the development of more sophisticated and precise instruments that enhance our understanding of the human mind.
One of the most exciting developments in behavioral assessment is the integration of technology into traditional testing methods. For instance, standardized tests have been around for decades, but they are evolving. With the advent of digital platforms, tests can now be administered online, allowing for a more flexible and engaging experience for participants. This shift not only makes testing more accessible but also enables researchers to collect data more efficiently. Think about it: instead of flipping through pages of paper, participants can interact with dynamic assessments that adapt to their responses, providing a more personalized evaluation.
Moreover, wearable technology is making significant strides in cognitive research. These devices, which can be as simple as smartwatches or as complex as EEG headsets, offer real-time data on physiological responses. For example, a participant's heart rate variability can indicate stress levels during cognitive tasks, providing researchers with valuable insights into how emotions affect cognitive performance. This real-time feedback loop is like having a backstage pass to the cognitive processes at play, allowing researchers to observe how individuals respond under various conditions.
In addition to wearables, researchers are also utilizing advanced software tools that can analyze behavioral data from various sources. These tools can track eye movements, facial expressions, and even vocal tones, offering a comprehensive view of cognitive and emotional states. This multifaceted approach is akin to piecing together a puzzle; each piece of data adds depth and clarity to our understanding of cognitive functions. By combining different assessment tools, researchers can create a holistic profile of an individual's cognitive abilities.
However, it’s essential to consider the implications of these tools. While they provide a wealth of information, researchers must also be mindful of ethical considerations, such as data privacy and the potential for misuse of sensitive information. As we continue to innovate and improve our behavioral assessment tools, maintaining a strong ethical framework is crucial to ensure that the benefits of these advancements are realized without compromising individual rights.
In conclusion, behavioral assessment tools are at the forefront of cognitive research, bridging the gap between theoretical knowledge and practical application. With the integration of technology and innovative methodologies, researchers are better equipped than ever to explore the complexities of the human mind. As we look to the future, it’s clear that these tools will continue to evolve, providing deeper insights and enhancing our understanding of cognitive processes.
- What are behavioral assessment tools? Behavioral assessment tools are instruments used to measure various cognitive functions, emotional states, and behavior patterns in individuals.
- How has technology impacted behavioral assessment? Technology has led to the development of digital platforms and wearable devices that enhance the flexibility, engagement, and accuracy of assessments.
- Why are ethical considerations important in cognitive research? Ethical considerations ensure that the rights and privacy of participants are protected while using sensitive data collected through assessment tools.
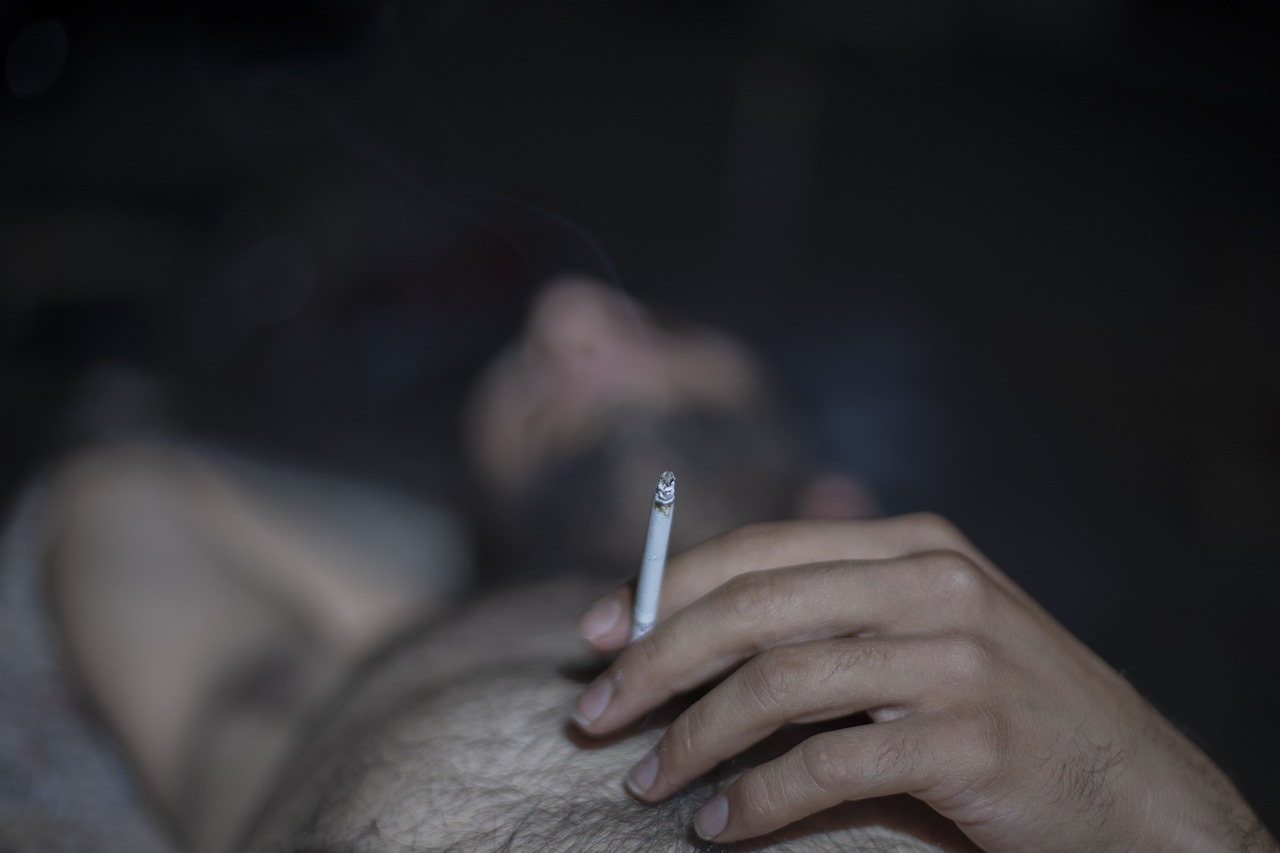
Standardized Testing Methods
Standardized testing methods have long been a cornerstone in the realm of cognitive assessment. These tests are designed to measure a variety of cognitive abilities consistently and objectively across different populations. The beauty of standardized tests lies in their ability to provide a reliable benchmark for evaluating cognitive functions, such as memory, attention, language skills, and problem-solving abilities. Imagine trying to gauge the intelligence of a group of students without a common yardstick—chaotic, right? That's where standardized tests come into play, creating a level playing field.
One of the most significant advancements in standardized testing is the incorporation of technology. Traditional paper-and-pencil tests are gradually being replaced by computer-based assessments that offer a more interactive experience. These digital platforms not only enhance engagement but also allow for immediate feedback, which is crucial for both researchers and participants. For instance, platforms that utilize adaptive testing can adjust the difficulty of questions based on a participant's responses, providing a more tailored assessment experience.
Furthermore, standardized tests are evolving to incorporate diverse methodologies. For example, tests such as the Wechsler Adult Intelligence Scale (WAIS) and the Stanford-Binet Intelligence Scales are now being complemented with innovative approaches that assess cognitive flexibility and emotional intelligence. This holistic view of cognitive assessment is essential, especially in our complex world where traditional metrics may not fully capture an individual's cognitive capabilities.
To better understand the impact of standardized testing methods, let’s take a look at some key characteristics that define these assessments:
Characteristic | Description |
---|---|
Reliability | The consistency of the test results over time and across different populations. |
Validity | The degree to which the test measures what it claims to measure. |
Standardization | The process of administering and scoring the test in a consistent manner. |
Norms | Statistical data that allows for comparison of an individual’s score against a representative sample. |
As we continue to refine these testing methods, researchers are also paying close attention to cultural and linguistic biases that may influence test outcomes. The goal is to ensure that standardized tests are not only fair but also reflective of the diverse backgrounds of test-takers. This is particularly important in cognitive research, where understanding the nuances of cognition across different demographics can lead to more inclusive and effective interventions.
In conclusion, standardized testing methods are evolving with the times, integrating technology and addressing biases to provide a more accurate representation of cognitive abilities. As researchers and practitioners continue to innovate in this area, we can expect these assessments to play an even more significant role in shaping our understanding of the human mind.
- What are standardized tests used for? Standardized tests are used to measure cognitive abilities, academic skills, and other psychological traits consistently across different populations.
- How do standardized tests ensure fairness? They are designed to be administered and scored in a consistent manner, with ongoing efforts to reduce cultural and linguistic biases.
- What is the difference between reliability and validity? Reliability refers to the consistency of test results, while validity refers to the accuracy of what the test measures.
- Are standardized tests being replaced by technology? While traditional methods are still in use, many standardized tests are now incorporating technology to enhance the testing experience and provide immediate feedback.

Wearable Technology
In recent years, has emerged as a groundbreaking tool in the field of cognitive research. These devices, which can be worn on the body, provide researchers with invaluable insights into cognitive processes by collecting real-time data on physiological responses. Imagine having a tiny lab on your wrist or in your pocket that continuously monitors your body's reactions as you go about your daily life. This is not just science fiction; it's the reality of how wearables are transforming our understanding of the human mind.
Wearable devices, such as smartwatches and fitness trackers, are equipped with sensors that can measure various physiological parameters, including heart rate, skin temperature, and even brain activity. This data is crucial for researchers aiming to link physiological responses with cognitive functions. For instance, when a person experiences stress, their heart rate may spike, and by analyzing this data, researchers can better understand how stress affects cognitive performance.
Moreover, the integration of wearable technology in cognitive studies allows for longitudinal data collection. Unlike traditional lab settings, where participants are often tested in isolated environments, wearables enable researchers to gather data over extended periods in naturalistic settings. This approach provides a more holistic view of cognitive functioning as it occurs in real life rather than in a controlled lab. For example, a researcher could study how cognitive performance varies during different daily activities, such as exercising, working, or socializing.
Another fascinating aspect of wearable technology is its ability to facilitate real-time feedback. Participants can receive immediate insights into their cognitive states, which can be particularly beneficial in cognitive training programs. Imagine a scenario where a person is practicing mindfulness; a wearable device could alert them when their heart rate indicates increased stress, prompting them to refocus and regain their calm. This kind of feedback loop not only enhances the participant's experience but also provides researchers with rich data on the efficacy of cognitive training techniques.
As we delve deeper into the realm of wearable technology, it’s essential to consider the implications for research ethics and data privacy. With the ability to collect vast amounts of personal data, researchers must ensure that they handle this information responsibly. Participants should always be informed about what data is being collected, how it will be used, and the measures in place to protect their privacy. This transparency is crucial in maintaining trust and encouraging participation in cognitive studies.
In conclusion, wearable technology is not just a trend; it’s a powerful tool reshaping cognitive research. By enabling real-time data collection and analysis in naturalistic environments, wearables are helping scientists unlock new insights into the human mind. As technology continues to evolve, we can expect even more innovative applications that will enhance our understanding of cognition and its complexities.
- What types of wearable technology are used in cognitive research? Wearable devices like smartwatches, fitness trackers, and EEG headsets are commonly used to collect physiological data during cognitive studies.
- How does wearable technology improve data collection? Wearables allow for continuous monitoring in real-world settings, providing a more comprehensive view of cognitive processes compared to traditional lab methods.
- Are there any privacy concerns with wearable technology? Yes, researchers must prioritize data privacy and transparency, ensuring participants are aware of how their data will be used and protected.
- Can wearables help in cognitive training? Absolutely! They provide real-time feedback that can enhance the effectiveness of cognitive training programs by helping individuals adjust their strategies on the fly.
Frequently Asked Questions
- What are neuroimaging techniques and why are they important in cognitive research?
Neuroimaging techniques are advanced methods that allow researchers to visualize brain activity in real-time. They are crucial because they provide insights into how different areas of the brain function during various cognitive tasks, helping us understand the underlying processes of thought, memory, and perception.
- How is artificial intelligence enhancing cognitive studies?
Artificial intelligence (AI) is transforming cognitive research by enabling the analysis of large and complex data sets more efficiently. AI algorithms can identify patterns and make predictions, which helps researchers gain deeper insights into cognitive processes and improve the accuracy of their findings.
- What role do machine learning algorithms play in cognitive research?
Machine learning algorithms are pivotal in processing and analyzing data in cognitive studies. They help researchers uncover hidden patterns, classify cognitive functions, and even predict outcomes based on previous data, making studies more robust and informative.
- Can you explain the significance of deep learning models in this field?
Deep learning models are significant because they mimic the way the human brain operates, allowing for a more nuanced analysis of neural networks. They excel in handling vast amounts of data, making them particularly useful for understanding complex cognitive tasks and improving research methodologies.
- How is natural language processing used in cognitive research?
Natural language processing (NLP) tools are used to analyze linguistic data, which is crucial for understanding language comprehension and production. By examining how individuals process language, researchers can gain insights into cognitive functions such as memory and attention.
- What are the benefits of using virtual reality in cognitive testing?
Virtual reality (VR) provides immersive environments that enhance participant engagement and allow for more realistic cognitive testing scenarios. This innovation transforms experimental designs, making it easier to study cognitive processes in a controlled yet dynamic setting.
- What are behavioral assessment tools and why are they important?
Behavioral assessment tools are essential for measuring cognitive functions and abilities. They provide standardized methods for evaluating various cognitive skills, which are crucial for understanding individual differences and informing treatment strategies in cognitive research.
- How do standardized testing methods contribute to cognitive research?
Standardized testing methods provide a reliable way to assess cognitive abilities across different populations. They ensure consistency and validity in measurements, making it easier to compare results and draw conclusions about cognitive performance and dysfunction.
- What role does wearable technology play in cognitive research?
Wearable technology is emerging as a valuable tool in cognitive research because it offers real-time data on physiological responses. This information helps researchers understand how cognitive processes unfold in everyday settings, providing a more holistic view of cognition in action.