The Future of AI in Predictive Modeling
Artificial Intelligence (AI) is not just a buzzword; it’s a powerhouse driving innovation in predictive modeling. Imagine being able to forecast the future with striking accuracy, thanks to the incredible capabilities of AI. This article delves into how AI is reshaping predictive modeling, making it more efficient, precise, and indispensable across various sectors. From healthcare to finance, the implications of AI-driven predictive modeling are profound, potentially transforming how industries operate and make decisions.
As we stand on the brink of a technological revolution, AI in predictive modeling is akin to having a crystal ball that reveals patterns hidden within mountains of data. The ability to analyze historical data and predict future outcomes is not just a luxury anymore; it's essential for staying competitive in today's fast-paced world. Companies that harness the power of AI can anticipate market trends, optimize operations, and enhance customer experiences like never before.
But what does the future hold? As AI technologies continue to evolve, we can expect even more sophisticated models that can process vast amounts of data in real time. This will lead to quicker and more accurate predictions, ultimately driving better decision-making and strategic planning. Picture a world where businesses can predict customer behavior before it happens or where healthcare providers can foresee potential health crises in patients long before they manifest. This is not science fiction; it is the exciting future of predictive modeling with AI.
Furthermore, the integration of AI with other emerging technologies, such as the Internet of Things (IoT), will create a synergy that enhances predictive capabilities. With IoT devices generating a continuous stream of data, the potential for real-time analysis and insights becomes limitless. This fusion will enable organizations to respond to changes instantaneously, making them more agile and resilient.
However, as we embrace these advancements, we must also confront the challenges that come with them. Issues like data quality, algorithmic bias, and the need for transparency in AI decision-making processes are critical hurdles that must be addressed. Ensuring that AI models are fair, reliable, and understandable is paramount for ethical implementation.
In conclusion, the future of AI in predictive modeling is bright, filled with opportunities and challenges alike. As we continue to explore this fascinating intersection of technology and data, we must remain vigilant in addressing the ethical implications while maximizing the transformative potential AI offers. The journey is just beginning, and the possibilities are endless.
- What is predictive modeling? Predictive modeling is a statistical technique that uses historical data to forecast future outcomes by identifying patterns and trends.
- How does AI enhance predictive modeling? AI enhances predictive modeling through advanced algorithms that can process large datasets efficiently, resulting in more accurate predictions.
- What are some applications of AI in predictive modeling? AI is used in various sectors, including healthcare for patient diagnosis and treatment planning, and finance for risk assessment and fraud detection.
- What challenges does AI face in predictive modeling? Key challenges include data quality issues, algorithmic bias, and the need for transparency in AI decision-making processes.
- What is the future of AI in predictive modeling? The future includes advancements in technology, integration with IoT, and a focus on explainable AI to build trust in predictive outcomes.

Understanding Predictive Modeling
Predictive modeling is a statistical technique that serves as a powerful tool for forecasting outcomes based on historical data. Imagine it as a crystal ball that uses past events to predict future scenarios. By leveraging sophisticated algorithms, predictive modeling identifies patterns and relationships within data, transforming raw numbers into actionable insights. This process is the backbone of many artificial intelligence (AI) applications, enabling businesses and organizations to make informed decisions.
At its core, predictive modeling involves several key steps:
- Data Collection: Gathering relevant historical data is the first step. This data can come from various sources, such as databases, surveys, or even IoT devices.
- Data Preparation: Once collected, the data needs to be cleaned and formatted. This involves removing inaccuracies and filling in missing values to ensure the model has the best possible input.
- Model Selection: Choosing the right algorithm is crucial. Depending on the nature of the data and the desired outcome, different algorithms may be more effective.
- Training the Model: The selected model is trained using a portion of the data, allowing it to learn patterns and relationships.
- Testing and Validation: After training, the model is tested on unseen data to evaluate its predictive accuracy. This step is vital to ensure that the model performs well in real-world scenarios.
- Deployment: Once validated, the model can be deployed in a live environment where it can start making predictions.
The applications of predictive modeling are vast and varied. From healthcare to finance, organizations are harnessing its power to improve decision-making processes. For instance, in healthcare, predictive models can forecast patient outcomes, helping doctors tailor treatments more effectively. In finance, they assist in risk assessment and fraud detection, providing insights that can save companies millions.
However, it’s important to recognize that predictive modeling is not infallible. Its effectiveness hinges on the quality of the data used. Inaccurate or incomplete data can lead to misleading predictions, akin to trying to navigate a ship without a reliable map. Therefore, ensuring data integrity is paramount for successful predictive modeling.
In summary, understanding predictive modeling is essential for anyone looking to leverage AI for strategic advantage. As technology continues to evolve, the methods and tools for predictive modeling will only become more sophisticated, opening up new avenues for innovation and insight. The future is bright for those who can harness the power of predictive analytics to drive their decision-making processes.

AI Technologies Enhancing Predictive Modeling
In today's data-driven world, artificial intelligence (AI) has emerged as a game changer in the realm of predictive modeling. By leveraging advanced algorithms, AI technologies enhance the accuracy and efficiency of predictions, enabling organizations to make informed decisions. Two key players in this transformative landscape are machine learning and deep learning. These technologies not only process vast amounts of data but also uncover patterns that would be impossible for humans to detect. Imagine having a super-sleuth at your disposal, tirelessly analyzing trends and providing insights that lead to better outcomes—this is what AI brings to the table.
Machine learning, the first major technology, is like teaching a child to recognize patterns. Through exposure to data, it learns from examples and improves its predictions over time. This iterative learning process is essential for industries where accuracy is paramount, such as finance, healthcare, and marketing. For example, in finance, machine learning algorithms assess credit risk by analyzing historical loan data, identifying which applicants are likely to default. This capability not only enhances decision-making but also helps in mitigating financial losses.
Deep learning, on the other hand, takes predictive modeling to the next level. By utilizing neural networks, deep learning can model intricate relationships within data that simpler algorithms might miss. It’s akin to having a sophisticated brain that can process complex information and make predictions based on subtle cues. This technology is particularly effective in fields like image recognition and natural language processing. For instance, in healthcare, deep learning can analyze medical images to detect diseases at an early stage, significantly improving patient outcomes.
As we delve deeper into the capabilities of AI-enhanced predictive modeling, it's essential to recognize the various algorithms that power these technologies. Here’s a brief overview of the types of machine learning that are particularly relevant:
Type of Learning | Description | Applications |
---|---|---|
Supervised Learning | Trains a model on labeled data to make predictions | Classification, Regression |
Unsupervised Learning | Works with unlabeled data to find hidden patterns | Clustering, Anomaly Detection |
Both supervised and unsupervised learning play crucial roles in predictive modeling. Supervised learning, for instance, is like having a teacher guide you through a subject, providing examples until you can solve problems on your own. This method is widely used for tasks such as classifying emails as spam or not spam. On the flip side, unsupervised learning is more like exploring a new city without a map, discovering hidden gems and unexpected routes along the way. This approach is invaluable for identifying trends and anomalies in data that might otherwise go unnoticed.
As we look ahead, the integration of these AI technologies into predictive modeling promises exciting advancements. With the ability to analyze larger datasets faster and more accurately, businesses can anticipate market trends, optimize operations, and enhance customer experiences like never before. The future is not just about having data; it’s about having the right tools to extract meaningful insights from that data. So, buckle up! The journey into the world of AI-enhanced predictive modeling is just beginning, and the possibilities are endless.
- What is predictive modeling? Predictive modeling is a statistical technique used to forecast outcomes based on historical data.
- How does machine learning enhance predictive modeling? Machine learning algorithms learn from data and improve their predictions over time, making them essential for accurate forecasting.
- What are the main types of machine learning? The two main types are supervised learning and unsupervised learning, each serving different purposes in data analysis.
- What industries benefit from AI in predictive modeling? Industries such as healthcare, finance, marketing, and retail leverage AI for better decision-making and operational efficiency.

Machine Learning Algorithms
When we talk about predictive modeling, we can't ignore the pivotal role that play in this realm. These algorithms are like the brains behind the operation, learning from data and continuously improving their predictions over time. Imagine a student who gets better with practice; that’s how these algorithms work! They analyze vast amounts of historical data, recognize patterns, and make informed predictions that can significantly impact various sectors, including finance, healthcare, and marketing.
One of the most fascinating aspects of machine learning algorithms is their ability to adapt and evolve. As they process more data, they refine their models, leading to increasingly accurate forecasts. For instance, in the finance sector, these algorithms can predict stock market trends by analyzing past performance data alongside current market conditions. Similarly, in healthcare, they can forecast patient outcomes by examining historical health records and treatment responses.
Machine learning can be categorized into several types, but two of the most prominent are supervised learning and unsupervised learning. Each has its unique approach and application:
- Supervised Learning: This method involves training a model on labeled data, where the desired output is known. It’s particularly effective for tasks like classification and regression. For example, a supervised learning algorithm could be trained to recognize whether an email is spam or not based on previously labeled examples.
- Unsupervised Learning: In contrast, unsupervised learning deals with unlabeled data. It seeks to uncover hidden patterns or groupings within the data. This approach is incredibly valuable for exploratory analysis and anomaly detection. Imagine a detective piecing together clues without knowing what the final picture looks like; that’s unsupervised learning at work!
Beyond these two main categories, machine learning algorithms also include various techniques like decision trees, random forests, and support vector machines. Each technique has its strengths and is chosen based on the specific requirements of the task at hand. For example, decision trees are intuitive and easy to interpret, making them a popular choice for many applications. On the other hand, random forests can enhance prediction accuracy by combining multiple decision trees to reduce overfitting.
To sum it up, machine learning algorithms are the backbone of predictive modeling, providing the tools necessary to analyze data and make predictions. Their ability to learn and adapt makes them invaluable across various industries, paving the way for smarter decisions and improved outcomes. As technology continues to advance, the potential for these algorithms to transform predictive modeling is boundless.

Supervised Learning
Supervised learning is a powerful technique in the realm of predictive modeling, where the model is trained on a labeled dataset. This means that each training example is paired with an output label, allowing the model to learn the relationship between the input data and the desired output. Imagine teaching a child to recognize different types of fruits: you show them an apple and say, “This is an apple.” Over time, the child learns to identify apples based on the characteristics you’ve shared. Similarly, supervised learning algorithms analyze historical data to make predictions about future outcomes.
One of the key advantages of supervised learning is its ability to handle a variety of tasks, including classification and regression. Classification involves categorizing data into predefined classes. For instance, a supervised learning model can be trained to identify whether an email is spam or not based on labeled examples. On the other hand, regression is used for predicting continuous outcomes, such as forecasting sales figures based on historical sales data.
To illustrate how supervised learning works, let’s delve into some common applications:
- Healthcare: Predicting patient diagnoses based on historical patient records.
- Finance: Assessing credit risk by analyzing past loan applications.
- Marketing: Classifying customer feedback to improve product offerings.
While supervised learning can yield impressive results, it’s essential to have a quality dataset. The model's performance heavily relies on the accuracy and relevance of the training data. If the data is flawed or biased, the predictions will likely reflect those issues, leading to poor decision-making. Therefore, data preprocessing and cleaning are critical steps in the supervised learning process.
In conclusion, supervised learning serves as a cornerstone in predictive modeling, enabling organizations to harness data effectively. By training models on labeled datasets, businesses can gain valuable insights and make informed decisions that drive success. As technology continues to evolve, the potential for supervised learning applications will only expand, paving the way for more sophisticated and efficient predictive models.
What is supervised learning?
Supervised learning is a type of machine learning where a model is trained on labeled data to make predictions or classifications based on input features.
What are the main types of problems addressed by supervised learning?
Supervised learning primarily addresses two types of problems: classification (categorizing data into classes) and regression (predicting continuous outcomes).
How important is data quality in supervised learning?
Data quality is crucial in supervised learning; inaccurate or biased data can lead to misleading predictions and poor performance of the model.
Can supervised learning be used in real-time applications?
Yes, supervised learning models can be deployed in real-time applications, allowing for immediate predictions based on new incoming data.

Unsupervised Learning
Unsupervised learning is a fascinating branch of machine learning that dives into the depths of unlabeled data. Unlike its counterpart, supervised learning, which requires predefined labels to guide the model, unsupervised learning takes a more exploratory approach. Imagine wandering through a vast forest without a map; you rely on your instincts to discover hidden paths and beautiful vistas. This is essentially how unsupervised learning operates—it seeks to uncover hidden patterns and structures within the data without any explicit guidance.
In practical terms, unsupervised learning is incredibly valuable for tasks such as clustering and dimensionality reduction. For instance, businesses often use clustering algorithms to segment their customer base into distinct groups based on purchasing behavior. This segmentation allows companies to tailor their marketing strategies effectively, ensuring that they reach the right audience with the right message. Some common algorithms employed in unsupervised learning include:
- K-means Clustering: This algorithm partitions data into K distinct clusters based on feature similarity, making it easier to analyze and interpret.
- Hierarchical Clustering: This method builds a hierarchy of clusters, which can be visualized as a tree structure, allowing for a more nuanced understanding of data relationships.
- Principal Component Analysis (PCA): PCA reduces the dimensionality of data while preserving as much variance as possible, helping to visualize complex datasets.
The beauty of unsupervised learning lies in its ability to reveal insights that might not be immediately obvious. For example, in the realm of healthcare, unsupervised learning can identify patient subgroups that share similar characteristics or health outcomes, paving the way for personalized treatment plans. Similarly, in finance, it can detect unusual patterns in transaction data that may indicate fraudulent activities, alerting institutions to potential risks.
However, like any powerful tool, unsupervised learning comes with its own set of challenges. One major hurdle is the interpretation of results. Since there are no labels to guide the analysis, the findings can sometimes be ambiguous or difficult to understand. Additionally, the effectiveness of unsupervised learning heavily relies on the quality of the input data. Noisy or irrelevant data can lead to misleading conclusions, making it crucial to preprocess data adequately before applying unsupervised techniques.
As we look to the future, the importance of unsupervised learning is only set to grow. With the explosion of big data, organizations are increasingly turning to these methods to glean actionable insights from their vast troves of information. As AI technologies continue to evolve, the ability to effectively harness unsupervised learning will be a game-changer across various sectors, enabling smarter decision-making and driving innovation.
What is the main difference between supervised and unsupervised learning?
Supervised learning requires labeled data to train models, while unsupervised learning works with unlabeled data, focusing on discovering patterns and structures within the data.
What are some common applications of unsupervised learning?
Unsupervised learning is commonly used in customer segmentation, anomaly detection, and data compression, among other applications.
How do I ensure the quality of data for unsupervised learning?
Data quality can be ensured through thorough preprocessing, which includes cleaning the data, removing duplicates, and addressing missing values before analysis.

Deep Learning Techniques
Deep learning is a revolutionary subset of machine learning that utilizes neural networks to analyze vast amounts of data and model complex relationships. Imagine a brain with interconnected neurons, where each neuron processes information and passes it along to others. This is essentially how deep learning works—it mimics the human brain's structure to improve understanding and prediction capabilities. By leveraging multiple layers of processing, deep learning can uncover intricate patterns that traditional algorithms might miss.
One of the standout features of deep learning is its ability to handle unstructured data, such as images, audio, and text. For instance, in image recognition, deep learning algorithms can identify objects in photos with remarkable accuracy. This capability is not just a technological marvel; it's transforming industries. In healthcare, deep learning models analyze medical images to detect diseases at early stages, while in finance, they evaluate transaction data to spot fraudulent activities.
To give you a clearer picture, let’s break down some of the most common deep learning techniques:
- Convolutional Neural Networks (CNNs): Primarily used for image processing, CNNs excel at recognizing spatial hierarchies in images. They automatically learn to detect features like edges, textures, and shapes, enabling them to classify images effectively.
- Recurrent Neural Networks (RNNs): These are particularly powerful for sequential data, such as time series or natural language. RNNs maintain a memory of previous inputs, making them ideal for tasks like language translation and speech recognition.
- Generative Adversarial Networks (GANs): GANs consist of two neural networks that work against each other. One generates data, while the other evaluates it. This technique is used to create realistic images, videos, and even music.
As we look to the future, the potential of deep learning seems limitless. It's not just about improving existing applications; it's about creating entirely new solutions that can revolutionize how we approach problems. For instance, in autonomous vehicles, deep learning algorithms analyze real-time data from sensors to make split-second driving decisions, enhancing safety and efficiency on the roads.
However, as powerful as these techniques are, they require significant computational resources and large datasets to train effectively. This brings us to the challenge of data availability and the need for robust infrastructure to support deep learning initiatives. As technology evolves, overcoming these barriers will be essential for unlocking the full potential of deep learning.

Applications Across Industries
Artificial Intelligence (AI) has revolutionized the way industries operate, particularly through the lens of predictive modeling. This transformative technology has found its way into various sectors, enhancing decision-making processes, operational efficiency, and customer satisfaction. Imagine a world where businesses can foresee market trends, healthcare professionals can predict patient outcomes, and financial institutions can detect fraud before it happens. This is not just a dream; it’s a reality being shaped by AI-driven predictive modeling.
In the healthcare sector, predictive modeling is making waves by assisting in patient diagnosis, treatment planning, and resource allocation. For instance, AI tools analyze vast amounts of patient data, identifying patterns that help predict health outcomes. This capability allows healthcare providers to optimize care delivery, ensuring that patients receive the right treatment at the right time. Consider the impact of predicting the onset of diseases like diabetes or heart conditions; early intervention can save lives and reduce healthcare costs significantly.
Moving to the financial industry, predictive modeling is a game changer. Financial institutions leverage AI algorithms to analyze market trends and customer behavior, enhancing their decision-making processes. For example, predictive analytics can help in risk assessment, fraud detection, and developing investment strategies. By sifting through historical data, AI can forecast potential market shifts, allowing investors to make informed decisions. This not only boosts profitability but also helps in maintaining trust with customers by ensuring their investments are safeguarded.
Moreover, the retail sector is also reaping the benefits of predictive modeling. Retailers can analyze consumer behavior to forecast demand for products, manage inventory efficiently, and personalize marketing strategies. Imagine walking into a store where the layout is tailored to your preferences, or receiving personalized offers based on your shopping habits. This level of customization is made possible through AI, creating a shopping experience that feels uniquely designed for each consumer.
In addition to these industries, predictive modeling is making strides in manufacturing as well. By predicting equipment failures before they occur, manufacturers can implement preventative maintenance strategies, reducing downtime and saving costs. This proactive approach not only enhances operational efficiency but also extends the lifespan of machinery, leading to significant savings over time.
The applications of AI-driven predictive modeling are vast and varied, with each industry harnessing its potential to drive innovation and improve outcomes. As we continue to explore the capabilities of AI, the possibilities seem endless, and the question remains: how will your industry adapt to this technological revolution?
- What is predictive modeling?
Predictive modeling is a statistical technique that uses historical data to forecast future outcomes. It identifies patterns and relationships within the data to make predictions.
- How does AI enhance predictive modeling?
AI enhances predictive modeling through advanced algorithms like machine learning and deep learning, allowing for more accurate predictions and the ability to process large datasets efficiently.
- What are some common applications of predictive modeling?
Common applications include healthcare for patient diagnosis, finance for risk assessment and fraud detection, and retail for inventory management and personalized marketing.
- What challenges does AI predictive modeling face?
Challenges include data quality issues, algorithmic bias, and the need for transparency in decision-making processes.
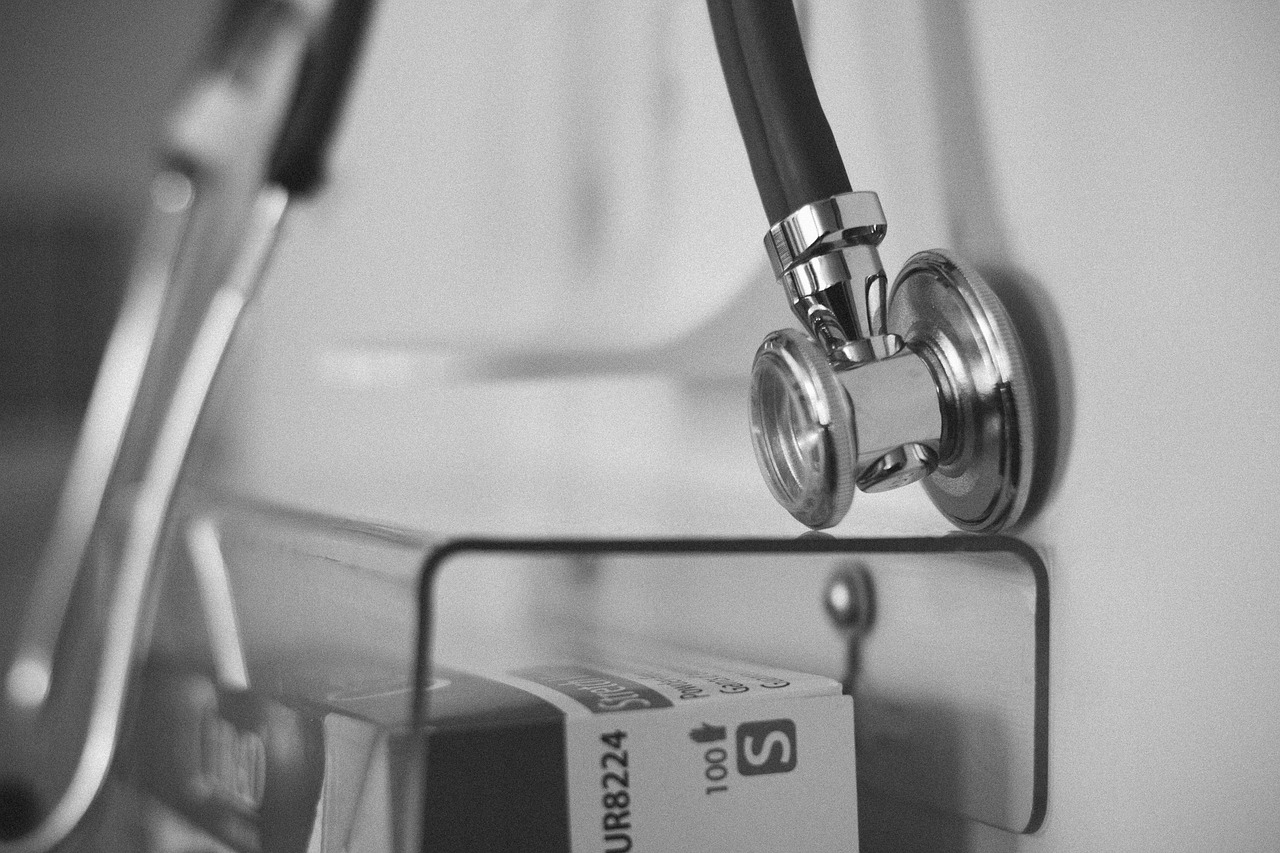
Healthcare Innovations
In the rapidly evolving world of healthcare, the integration of artificial intelligence (AI) into predictive modeling is nothing short of revolutionary. Imagine a world where doctors can predict a patient's health outcomes with pinpoint accuracy, much like a weather forecast that can tell you whether to carry an umbrella. AI-driven predictive modeling is transforming how healthcare professionals approach patient care, diagnosis, and treatment planning.
One of the most significant innovations is the use of predictive analytics to enhance patient diagnosis. By analyzing vast amounts of patient data, including medical histories, lab results, and even lifestyle choices, AI algorithms can identify patterns that may indicate potential health issues before they become critical. For instance, if a patient exhibits certain symptoms, an AI model can compare those symptoms against a database of similar cases to suggest possible diagnoses and recommend further testing.
Moreover, AI tools are proving invaluable in treatment planning. By assessing a patient's unique characteristics and previous treatment outcomes, these models can help healthcare providers tailor interventions that are more likely to succeed. This personalized approach not only improves patient outcomes but also optimizes resource allocation within healthcare systems, ensuring that the right treatments are administered to the right patients at the right time.
Additionally, AI is playing a crucial role in resource allocation within healthcare organizations. Hospitals can utilize predictive modeling to forecast patient admissions and discharges, allowing them to manage staffing levels and resource availability effectively. This proactive approach minimizes wait times and enhances the overall patient experience, creating a more efficient healthcare environment.
To illustrate the impact of AI in healthcare, consider the following table that highlights some key applications:
Application | Description |
---|---|
Patient Diagnosis | Utilizes historical data to predict possible health issues. |
Treatment Planning | Tailors interventions based on individual patient profiles. |
Resource Allocation | Forecasts patient flow to optimize staffing and resources. |
As we look to the future, the potential for AI in healthcare is vast. With ongoing advancements in technology and data analytics, we can expect even more innovative applications that will further enhance predictive modeling. The ability to predict health outcomes not only empowers healthcare providers but also gives patients a greater sense of control over their health journeys. Ultimately, the fusion of AI and predictive modeling is paving the way for a healthier, more informed society.
- What is predictive modeling in healthcare? Predictive modeling in healthcare uses statistical algorithms and machine learning techniques to analyze historical data and predict future health outcomes.
- How does AI improve patient diagnosis? AI enhances patient diagnosis by analyzing large datasets to identify patterns and correlations that may indicate potential health issues.
- Can AI help in treatment planning? Yes, AI can assist in treatment planning by recommending personalized interventions based on a patient's unique characteristics and previous treatment outcomes.
- What are the benefits of AI in resource allocation? AI can optimize staffing and resource management by forecasting patient admissions and discharges, leading to improved efficiency and reduced wait times.

Financial Forecasting
In the fast-paced world of finance, predicting future trends and behaviors is not just a luxury; it's a necessity. Financial forecasting, powered by AI, revolutionizes how businesses and investors approach their financial strategies. Imagine having a crystal ball that not only shows you the future but also provides insights based on vast amounts of historical data. That's what AI-driven predictive modeling offers. By analyzing patterns in financial data, AI algorithms can forecast market movements, assess risks, and even detect fraudulent activities before they escalate.
One of the most significant benefits of AI in financial forecasting is its ability to process and analyze large datasets quickly and efficiently. Traditional methods often struggle with the sheer volume of data generated in today's financial markets. However, with machine learning algorithms, organizations can sift through terabytes of information, identifying trends that would be impossible to detect manually. This capability allows for more informed decision-making and a proactive approach to risk management.
For instance, financial institutions utilize AI to enhance their investment strategies. By analyzing historical performance data, current market conditions, and even social media sentiment, AI can provide insights that guide investment decisions. This level of analysis can lead to better returns and reduced risks. Here are some of the specific areas where AI-driven financial forecasting is making a significant impact:
- Risk Assessment: AI models can evaluate the creditworthiness of borrowers by analyzing various factors, including payment history and economic conditions, leading to more accurate lending decisions.
- Fraud Detection: By recognizing unusual patterns in transaction data, AI can flag potentially fraudulent activities, allowing institutions to act swiftly and protect their assets.
- Market Trend Analysis: AI algorithms can analyze market trends in real time, providing traders with actionable insights that can lead to more profitable trades.
Moreover, AI’s predictive capabilities extend to customer behavior analysis. Financial institutions can forecast customer needs and preferences, allowing them to tailor their services accordingly. This personalized approach not only enhances customer satisfaction but also fosters loyalty, as clients feel understood and valued. With AI, the financial sector is moving towards a more customer-centric model, where data-driven insights lead to better service delivery.
As we look to the future, the role of AI in financial forecasting is set to expand further. With advancements in technology and an ever-increasing volume of data, the potential for more sophisticated predictive models is immense. Financial organizations that embrace these innovations will not only stay ahead of the competition but also navigate the complexities of the market with greater confidence.
What is financial forecasting?
Financial forecasting involves predicting future financial outcomes based on historical data, trends, and various economic indicators. It helps businesses make informed decisions regarding investments, budgeting, and resource allocation.
How does AI improve financial forecasting?
AI enhances financial forecasting by analyzing large datasets quickly, identifying patterns, and providing insights that traditional methods may overlook. This leads to more accurate predictions and better decision-making.
What industries benefit from AI-driven financial forecasting?
Various industries, including banking, insurance, and investment firms, benefit from AI-driven financial forecasting. These sectors use predictive models to assess risks, detect fraud, and optimize investment strategies.
Can AI completely replace human analysts in financial forecasting?
While AI significantly enhances predictive capabilities, human analysts still play a crucial role in interpreting results, understanding market nuances, and making strategic decisions based on AI-generated insights.

Challenges in AI Predictive Modeling
As we dive into the exciting world of AI predictive modeling, it’s essential to recognize that this powerful technology doesn’t come without its challenges. While AI can analyze vast amounts of data and uncover hidden patterns, several hurdles can impede its effectiveness. Understanding these challenges is crucial for anyone looking to leverage AI for predictive modeling.
One of the primary challenges facing AI predictive modeling is data quality and availability. The effectiveness of any predictive model is heavily reliant on the data it uses. If the data is inaccurate, incomplete, or biased, the predictions can be misleading. Imagine trying to predict the weather using faulty instruments; the results would be far from reliable, right? This analogy holds true for predictive modeling. Organizations must ensure they have access to high-quality data, which often requires significant effort in data cleaning and preprocessing.
Another significant issue is algorithmic bias. AI systems learn from historical data, and if that data contains biases—whether societal, racial, or economic—the AI can inadvertently perpetuate these biases in its predictions. For instance, if a financial model is trained on data that reflects historical lending practices that favored certain demographics, it may continue to discriminate against others in its predictions. This leads to unfair outcomes and raises ethical concerns about the use of AI in decision-making processes.
Furthermore, there’s a pressing need for transparency in AI predictive modeling. Many AI algorithms, especially deep learning models, operate as "black boxes," making it difficult for stakeholders to understand how decisions are made. This lack of transparency can erode trust in AI systems, particularly in critical areas such as healthcare and finance, where the stakes are incredibly high. Stakeholders need to comprehend and validate AI-driven decisions to ensure accountability and foster confidence in the technology.
Lastly, the rapid pace of technological advancement poses a challenge as well. The field of AI is evolving quickly, and keeping up with new algorithms, techniques, and best practices can be daunting for organizations. It’s akin to trying to catch a speeding train; if you’re not prepared, you might miss out on the opportunities that come with these advancements. Continuous learning and adaptation are necessary to stay relevant and make the most of AI predictive modeling.
In summary, while AI predictive modeling holds immense potential, it’s essential to navigate the challenges of data quality, algorithmic bias, transparency, and the fast-paced nature of technology. By addressing these issues head-on, organizations can harness the true power of AI for predictive modeling and unlock valuable insights that drive informed decision-making.
- What is predictive modeling? Predictive modeling is a statistical technique that uses historical data to forecast future outcomes.
- How does AI enhance predictive modeling? AI enhances predictive modeling by utilizing algorithms that can analyze large datasets and identify complex patterns more efficiently than traditional methods.
- What are the implications of algorithmic bias? Algorithmic bias can lead to unfair outcomes in decision-making processes, perpetuating existing societal biases.
- Why is transparency important in AI predictive modeling? Transparency helps stakeholders understand and trust AI-driven predictions, ensuring accountability in critical applications.
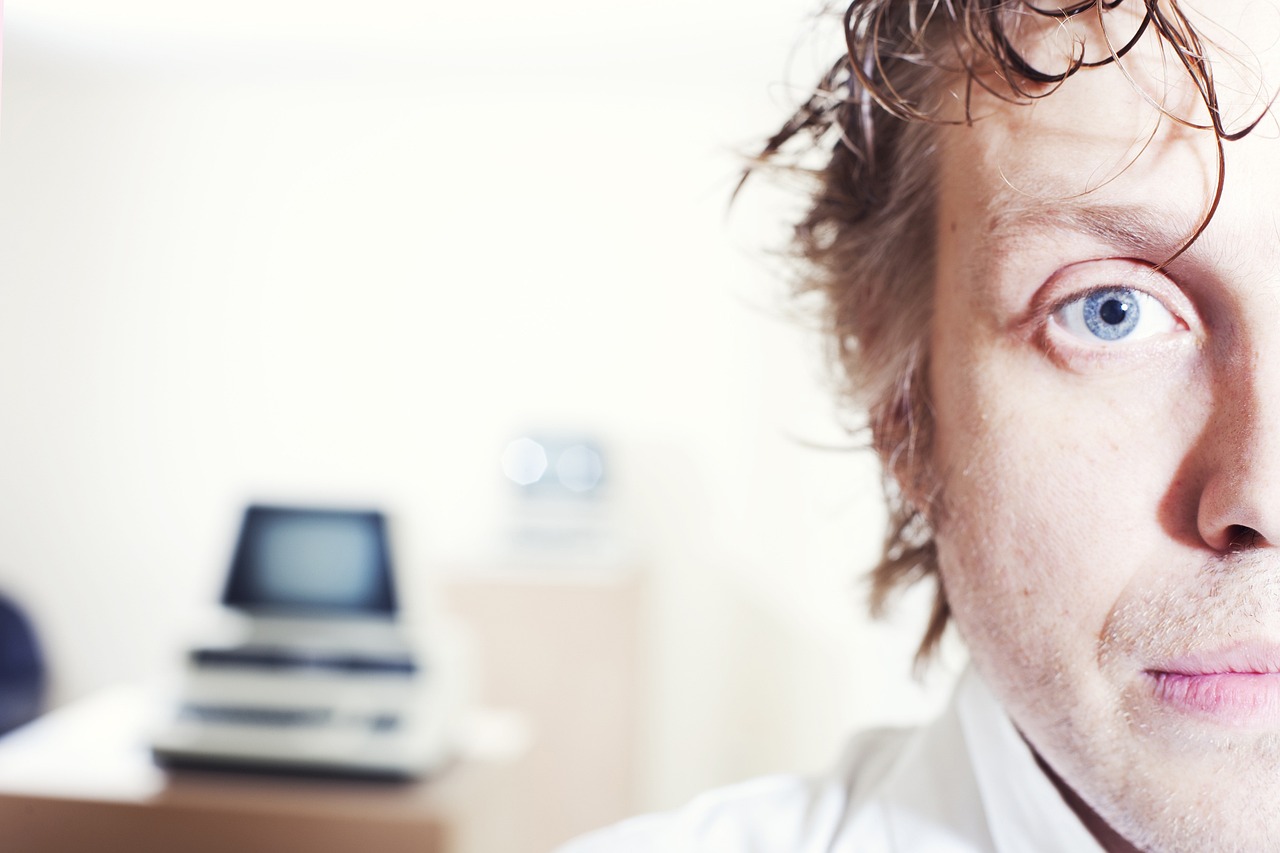
Data Quality and Availability
In the realm of predictive modeling, the significance of data quality and availability cannot be overstated. Imagine trying to bake a cake without the right ingredients; the outcome would likely be disappointing. Similarly, predictive models rely heavily on high-quality data to produce meaningful and accurate predictions. If the data fed into these models is flawed, whether due to inaccuracies, incompleteness, or outdated information, the predictions can lead to misguided decisions. This is particularly critical in sectors like healthcare and finance, where the stakes are incredibly high.
Furthermore, the availability of data is just as important as its quality. In many cases, organizations may have access to vast amounts of data, but if that data is not readily available or is siloed within departments, it becomes a barrier rather than a boon. To illustrate this, consider a company that has customer data scattered across different platforms. If they fail to integrate this data effectively, they risk missing out on valuable insights that could enhance their predictive modeling capabilities.
Addressing these challenges requires a multifaceted approach. Organizations must invest in robust data management systems that ensure data is not only collected but also cleaned and maintained. This involves:
- Data Cleaning: Regularly reviewing and correcting inaccuracies in datasets.
- Data Integration: Merging data from different sources to create a comprehensive view.
- Data Governance: Establishing policies and standards for data management to ensure quality and compliance.
Moreover, the rise of big data technologies and cloud computing has made it easier to access and analyze large datasets. However, organizations still need to be strategic about how they utilize these resources. For instance, employing advanced data analytics tools can help in identifying patterns and trends that might not be immediately apparent. This, in turn, enhances the predictive modeling process by providing a richer context for the algorithms to work with.
Ultimately, the journey towards effective predictive modeling hinges on a commitment to data quality and availability. By prioritizing these aspects, organizations can unlock the full potential of their predictive capabilities, leading to improved decision-making and better outcomes across various industries.
- What is predictive modeling? Predictive modeling is a statistical technique used to forecast outcomes based on historical data and patterns.
- Why is data quality important in predictive modeling? High-quality data ensures that the predictions made by models are accurate and reliable, leading to better decision-making.
- How can organizations improve data availability? By integrating data from various sources and investing in data management systems, organizations can enhance data availability.
- What role does AI play in predictive modeling? AI technologies, such as machine learning and deep learning, significantly improve the accuracy and efficiency of predictive modeling.

Algorithmic Bias
Algorithmic bias is a critical issue that arises when artificial intelligence models produce outcomes that are unfair or prejudiced due to biased training data. Imagine you're baking a cake, but instead of using the right ingredients, you throw in a random assortment of leftovers. The result? A cake that likely tastes terrible! Similarly, when AI models are trained on skewed or unrepresentative datasets, they can generate predictions that perpetuate existing inequalities or create new biases. This can be particularly detrimental in sensitive areas like hiring, law enforcement, and healthcare, where biased outcomes can have significant real-world consequences.
One of the primary sources of algorithmic bias is the data itself. If the data used to train an AI model reflects historical inequalities, the model will likely reproduce those biases in its predictions. For example, if a predictive model for job applications is trained on past hiring data that favored one demographic over another, it may unfairly disadvantage candidates from underrepresented groups. This not only raises ethical concerns but also undermines the effectiveness of AI solutions, as they fail to provide equitable outcomes.
Addressing algorithmic bias requires a multifaceted approach. Here are some strategies that can be employed:
- Data Auditing: Regularly auditing datasets for bias and ensuring they are representative of the population can help mitigate issues before they arise.
- Diverse Training Data: Incorporating diverse data sources can help create a more balanced model that reflects a wider range of experiences and backgrounds.
- Bias Detection Tools: Utilizing tools designed to detect and measure bias in AI models can provide insights and guide adjustments to improve fairness.
- Transparency and Accountability: Ensuring that AI systems are transparent about how decisions are made can help stakeholders understand and trust the outcomes.
Ultimately, the implications of algorithmic bias extend beyond technical challenges; they touch on fundamental issues of fairness and justice in our society. By prioritizing ethical considerations in AI development, we can work towards creating systems that not only enhance predictive modeling but also promote equity and inclusivity.
- What is algorithmic bias? Algorithmic bias refers to the tendency of AI models to produce prejudiced or unfair outcomes due to biased training data.
- How does algorithmic bias occur? It occurs when the data used to train AI systems reflects historical inequalities or is unrepresentative of the broader population.
- Why is addressing algorithmic bias important? Addressing algorithmic bias is crucial for ensuring fairness, equity, and trust in AI systems, particularly in sensitive areas like hiring and healthcare.
- What can be done to mitigate algorithmic bias? Strategies include data auditing, using diverse training data, employing bias detection tools, and promoting transparency in AI decision-making.
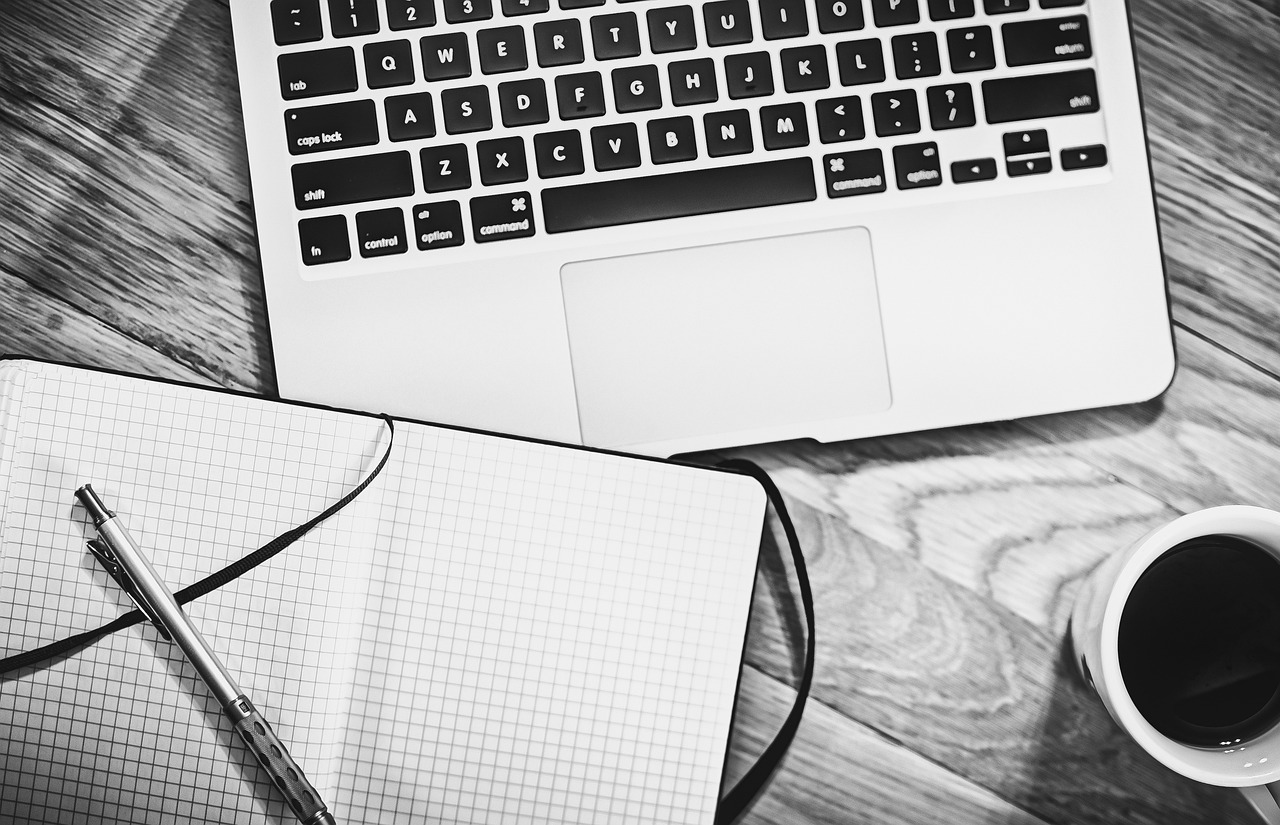
Future Trends in AI Predictive Modeling
The future of AI in predictive modeling is not just a distant dream; it's unfolding right before our eyes, and it's nothing short of exhilarating. With the rapid advancements in technology and the explosion of data availability, we're on the brink of a new era where predictive modeling becomes even more sophisticated and integral across various sectors. Imagine a world where decisions are not just made based on gut feelings but are driven by robust, data-backed insights. This is the promise of AI, and the trends shaping this future are as diverse as they are impactful.
One of the most exciting trends is the integration of AI predictive modeling with Internet of Things (IoT) devices. As IoT continues to proliferate, the amount of real-time data being generated is staggering. This data can be harnessed to enhance predictive modeling by providing up-to-the-minute insights that help organizations make informed decisions swiftly. For instance, in smart cities, AI can analyze traffic patterns in real-time to optimize traffic flow, reducing congestion and improving urban living conditions. In healthcare, wearable devices can continuously monitor patient vitals, allowing predictive models to anticipate health issues before they become critical.
Another significant trend is the rise of Explainable AI (XAI). As AI systems become more complex, the need for transparency in how these systems make decisions becomes paramount. XAI aims to demystify AI models, providing stakeholders with clear insights into how predictions are made. This transparency is crucial in sectors like finance and healthcare, where understanding the rationale behind decisions can significantly impact trust and compliance. By making AI models more interpretable, organizations can foster a culture of accountability and ethical AI usage, ensuring that predictive modeling serves everyone fairly.
Moreover, the convergence of AI with other emerging technologies, such as blockchain and big data analytics, is poised to revolutionize predictive modeling. Blockchain can enhance data integrity and security, ensuring that the data fed into predictive models is reliable and tamper-proof. This is particularly important in industries where data manipulation can lead to disastrous outcomes, such as finance and supply chain management. On the other hand, big data analytics allows organizations to sift through massive datasets to uncover hidden patterns and insights that traditional methods might miss, further refining predictive accuracy.
In addition to these trends, we can expect to see an increased focus on personalization in predictive modeling. As consumers demand more tailored experiences, businesses will leverage AI to predict individual preferences and behaviors, creating highly customized offerings. This trend is already evident in sectors like retail, where AI algorithms analyze shopping patterns to recommend products uniquely suited to each customer. Imagine walking into a store where the AI knows your preferences and suggests items you’re likely to love, enhancing your shopping experience while boosting the retailer's sales.
As we look ahead, it's clear that the landscape of AI predictive modeling is evolving at a breathtaking pace. The convergence of technology, data availability, and the need for ethical standards will define how organizations leverage predictive insights. The future is not just about making predictions; it's about making informed, responsible decisions that can positively impact society. As we embrace these advancements, the possibilities are endless, and we're only just scratching the surface of what AI can achieve in predictive modeling.
- What is predictive modeling?
Predictive modeling is a statistical technique that uses historical data to forecast future outcomes by identifying patterns and trends.
- How does AI enhance predictive modeling?
AI enhances predictive modeling through advanced algorithms, enabling more accurate predictions and efficient processing of large datasets.
- What industries benefit from AI predictive modeling?
Industries such as healthcare, finance, marketing, and retail benefit significantly from AI predictive modeling by improving decision-making and operational efficiency.
- What are the challenges facing AI predictive modeling?
Challenges include data quality issues, algorithmic bias, and the need for transparency in AI decision-making processes.

Integration with IoT
The integration of Artificial Intelligence (AI) with the Internet of Things (IoT) is a game changer in the realm of predictive modeling. Imagine a world where your coffee machine knows exactly when you wake up and starts brewing your favorite blend just in time. This is not just a dream; it's the power of IoT combined with AI predictive capabilities. By harnessing real-time data from a myriad of connected devices, AI can analyze patterns and make predictions that were previously unimaginable.
Consider this scenario: a smart thermostat that learns your daily schedule and preferences. It collects data on your heating and cooling habits, analyzes this information, and predicts when to adjust the temperature for optimal comfort while saving energy. This not only enhances user experience but also contributes to energy efficiency, illustrating the profound impact of AI and IoT integration.
Moreover, the synergy between AI and IoT opens up new avenues for data-driven decision-making across various sectors. In healthcare, for instance, wearable devices can continuously monitor patient vitals and send this data to AI systems, which can predict potential health issues before they escalate. In smart cities, IoT sensors can collect traffic data, and AI can analyze this information to predict congestion patterns, optimizing traffic flow and reducing travel times.
To better understand the potential of this integration, let’s break down some key benefits:
- Real-time Analysis: IoT devices provide continuous streams of data, allowing AI to make real-time predictions that can significantly impact operational efficiency.
- Enhanced Accuracy: With more data points available from connected devices, AI algorithms can refine their predictions, resulting in improved accuracy and reliability.
- Scalability: The integration allows for scalable solutions that can adapt as more IoT devices come online, ensuring that predictive modeling evolves with technological advancements.
As we look to the future, the integration of AI and IoT will undoubtedly lead to more sophisticated predictive models that can adapt and respond to the complexities of our interconnected world. This evolution is not just about improving existing processes; it's about reimagining how we interact with technology in our daily lives, making it more intuitive and responsive to our needs.
Q1: What is the role of IoT in predictive modeling?
A1: IoT devices collect real-time data that AI algorithms can analyze to make accurate predictions, enhancing decision-making across various sectors.
Q2: How does AI improve the functionality of IoT devices?
A2: AI enhances IoT devices by enabling them to learn from data, adapt to user behavior, and make predictions that improve efficiency and user experience.
Q3: Can predictive modeling help in energy conservation?
A3: Yes, predictive modeling can analyze usage patterns from IoT devices to optimize energy consumption, leading to significant savings and reduced environmental impact.
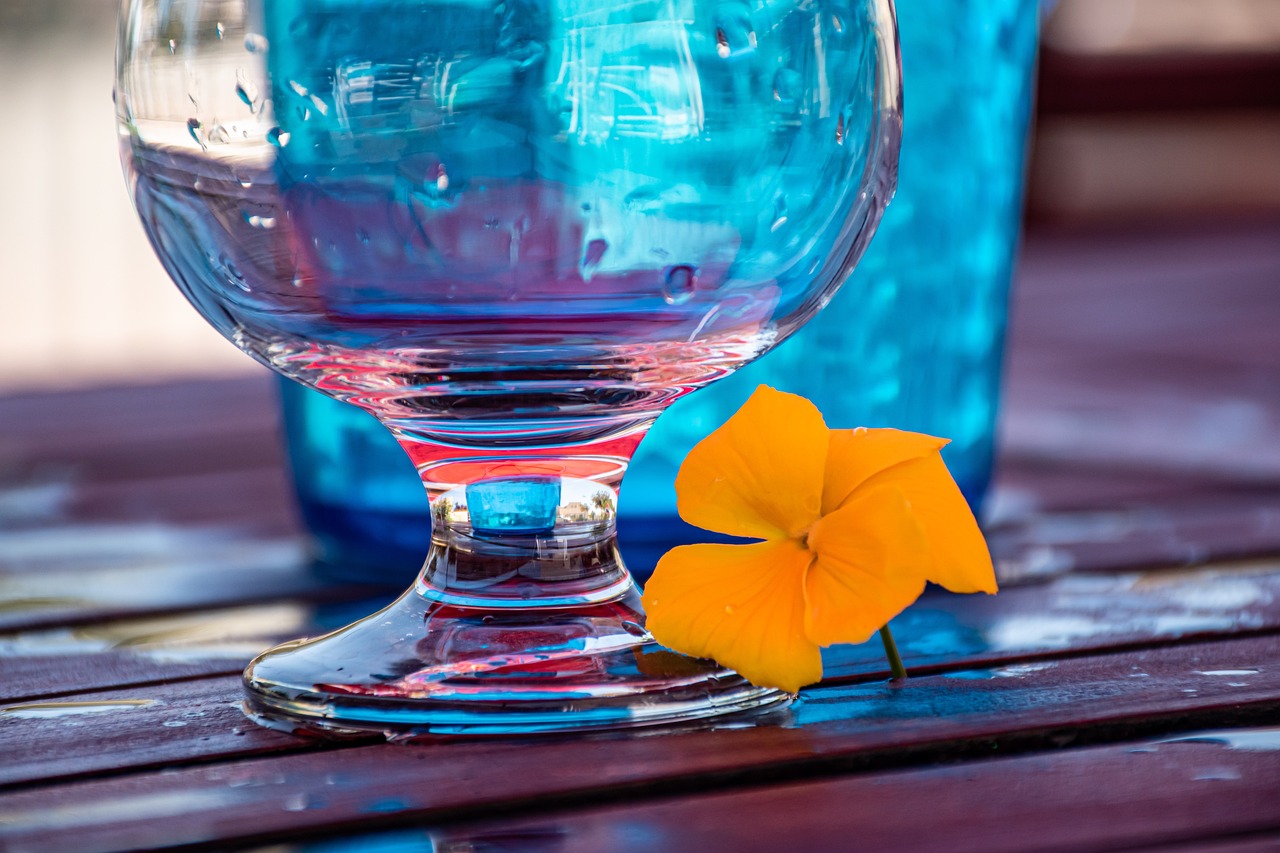
Explainable AI
As we dive deeper into the world of artificial intelligence, one term that is gaining traction is Explainable AI (XAI). But what exactly does this mean? In simple terms, XAI refers to methods and techniques in AI that make the outputs of AI systems understandable to humans. Think of it as the translator between complex algorithms and human comprehension. Just like a good teacher breaks down intricate concepts into digestible pieces, XAI aims to clarify how AI models arrive at their predictions.
The importance of Explainable AI cannot be overstated, especially in fields where decisions can significantly impact lives, such as healthcare and finance. Imagine a doctor relying on an AI model to diagnose a patient. If the AI suggests a treatment plan, the physician needs to understand the reasoning behind that recommendation. This understanding fosters trust, allowing healthcare professionals to make informed decisions that can lead to better patient outcomes.
Moreover, XAI addresses the growing concern regarding algorithmic bias. When AI systems make decisions, if those decisions are based on biased data, the outcomes can be unfair or discriminatory. By employing explainable models, stakeholders can scrutinize the decision-making process, ensuring that biases are identified and mitigated. This transparency is crucial for ethical AI implementation, as it allows organizations to take responsibility for their AI-driven decisions.
To illustrate the significance of Explainable AI, consider the following table that outlines key benefits:
Benefit | Description |
---|---|
Transparency | Provides clarity on how decisions are made, fostering trust among users. |
Accountability | Enables organizations to take responsibility for the outcomes of their AI systems. |
Bias Detection | Helps identify and mitigate biases within AI models, promoting fairness. |
Improved Performance | Facilitates better model refinement through understanding of decision processes. |
As we look to the future, the integration of Explainable AI into predictive modeling will not only enhance the credibility of AI systems but also empower users to engage with technology on a deeper level. By demystifying the black box of AI, we can ensure that these powerful tools are used responsibly and effectively across various sectors. This evolution is akin to turning on the lights in a dark room; it reveals the intricate workings of AI, allowing us to navigate the landscape with confidence.
- What is Explainable AI? Explainable AI refers to methods that make AI outputs understandable to humans, ensuring transparency in decision-making.
- Why is Explainable AI important? It enhances trust, accountability, and fairness in AI systems, especially in critical fields like healthcare and finance.
- How does Explainable AI address algorithmic bias? By providing insights into decision-making processes, it allows stakeholders to identify and mitigate biases in AI models.
- Can Explainable AI improve model performance? Yes, understanding decision processes can lead to better model refinement and performance over time.
Frequently Asked Questions
- What is predictive modeling?
Predictive modeling is a statistical technique that uses historical data to forecast future outcomes. By leveraging algorithms, it identifies patterns and makes predictions, which is essential for many AI applications.
- How does AI enhance predictive modeling?
AI enhances predictive modeling through technologies like machine learning and deep learning. These technologies allow for more accurate predictions and efficient processing of large datasets, making it easier to uncover insights and trends.
- What are the main types of machine learning used in predictive modeling?
The two main types of machine learning used in predictive modeling are supervised learning and unsupervised learning. Supervised learning uses labeled data for tasks like classification and regression, while unsupervised learning deals with unlabeled data to find hidden patterns.
- What role does deep learning play in predictive modeling?
Deep learning, a subset of machine learning, employs neural networks to model complex relationships in data. It excels in tasks such as image and speech recognition, significantly enhancing the predictive capabilities of AI systems.
- In which industries is predictive modeling applied?
Predictive modeling finds applications across various industries, including healthcare for patient diagnosis and treatment planning, and finance for risk assessment and fraud detection. It improves decision-making and operational efficiency through data-driven insights.
- What challenges does AI face in predictive modeling?
AI in predictive modeling faces several challenges, including data quality issues, algorithmic bias, and the need for transparency in decision-making processes. These challenges can lead to misleading predictions and ethical concerns.
- How important is data quality in predictive modeling?
Data quality is crucial in predictive modeling because inaccurate or incomplete data can result in misleading predictions and poor outcomes. High-quality, reliable data is essential for producing effective models.
- What is algorithmic bias, and why is it a concern?
Algorithmic bias occurs when AI models yield unfair or prejudiced outcomes due to biased training data. This is a significant concern as it can lead to unethical practices and reinforce existing inequalities in various applications.
- What are the future trends in AI predictive modeling?
The future of AI predictive modeling looks bright, with trends like the integration of AI with Internet of Things (IoT) devices for real-time data analysis and the rise of Explainable AI (XAI) to enhance transparency and trust in AI-driven decisions.
- How will IoT integration impact predictive modeling?
Integrating AI predictive modeling with IoT devices will enable real-time data analysis, leading to more accurate and timely predictions across various applications, from smart cities to healthcare systems.