The Role of AI in Enhancing Data Collection Methods
In today's fast-paced digital world, data is the new oil. It's the lifeblood of decision-making processes across industries, from healthcare to finance, and everything in between. But how do organizations ensure they're collecting the right data in the right way? Enter artificial intelligence (AI), a game-changer that is not just enhancing data collection methods but revolutionizing them entirely. With AI, we’re not just talking about faster data collection; we’re diving into a realm where accuracy, efficiency, and insightful analytics converge to transform the way businesses operate.
Imagine a world where tedious manual data entry is a thing of the past. With AI-powered tools, organizations can automate the collection process, allowing employees to focus on what truly matters: analysis and strategy. These tools can handle everything from automated surveys that gather consumer feedback to chatbots that engage users in real-time, collecting valuable insights without any human intervention. The result? A treasure trove of data that is both rich and reliable.
But the benefits of AI in data collection don't stop there. Think of AI as a meticulous librarian, ensuring that every piece of data is not only collected but also organized and validated. By leveraging advanced algorithms, AI can sift through mountains of data, identifying inconsistencies and correcting errors before they even reach the analysts' desks. This leads to a higher quality of datasets, which are crucial for making informed decisions.
Moreover, AI enables real-time data monitoring. Imagine being able to adjust your marketing strategy on the fly based on current consumer behavior. With AI, organizations can monitor data as it’s collected, allowing them to make timely decisions that can significantly impact their bottom line. This agility is essential in today’s competitive landscape, where the ability to pivot quickly can mean the difference between success and failure.
Another fascinating aspect of AI in data collection is its use of predictive analytics. By analyzing historical data, AI can forecast future trends and behaviors, allowing organizations to proactively gather relevant data. This foresight not only enhances strategic planning but also positions businesses to stay ahead of their competition. Imagine knowing what your customers will want before they even realize it themselves!
As we delve deeper into the world of AI, we also encounter the exciting realm of Natural Language Processing (NLP). This technology enhances survey design and analysis by enabling machines to understand and interpret human language. With NLP, organizations can gain insights into respondent sentiments and intentions, making it easier to tailor products and services to meet customer needs. It’s like having a crystal ball that reveals what customers are really thinking.
In conclusion, AI is not just a tool; it’s a transformative force in the realm of data collection. By enhancing accuracy, efficiency, and insights, AI is reshaping how organizations approach decision-making and strategic planning. As we continue to explore the capabilities of AI, one thing is clear: the future of data collection is bright, and those who embrace these technologies will undoubtedly lead the way.
- What is AI in data collection? AI in data collection refers to the use of artificial intelligence technologies to automate and enhance the process of gathering, validating, and analyzing data.
- How does AI improve data accuracy? AI improves data accuracy by reducing human errors, validating information in real-time, and employing advanced analytics to ensure reliable results.
- What are some AI tools for data collection? Common AI tools for data collection include automated surveys, chatbots, and data scraping software, which streamline the data gathering process.
- What challenges do organizations face when implementing AI? Organizations may face challenges such as cost, complexity of integration, and data privacy concerns when adopting AI technologies for data collection.
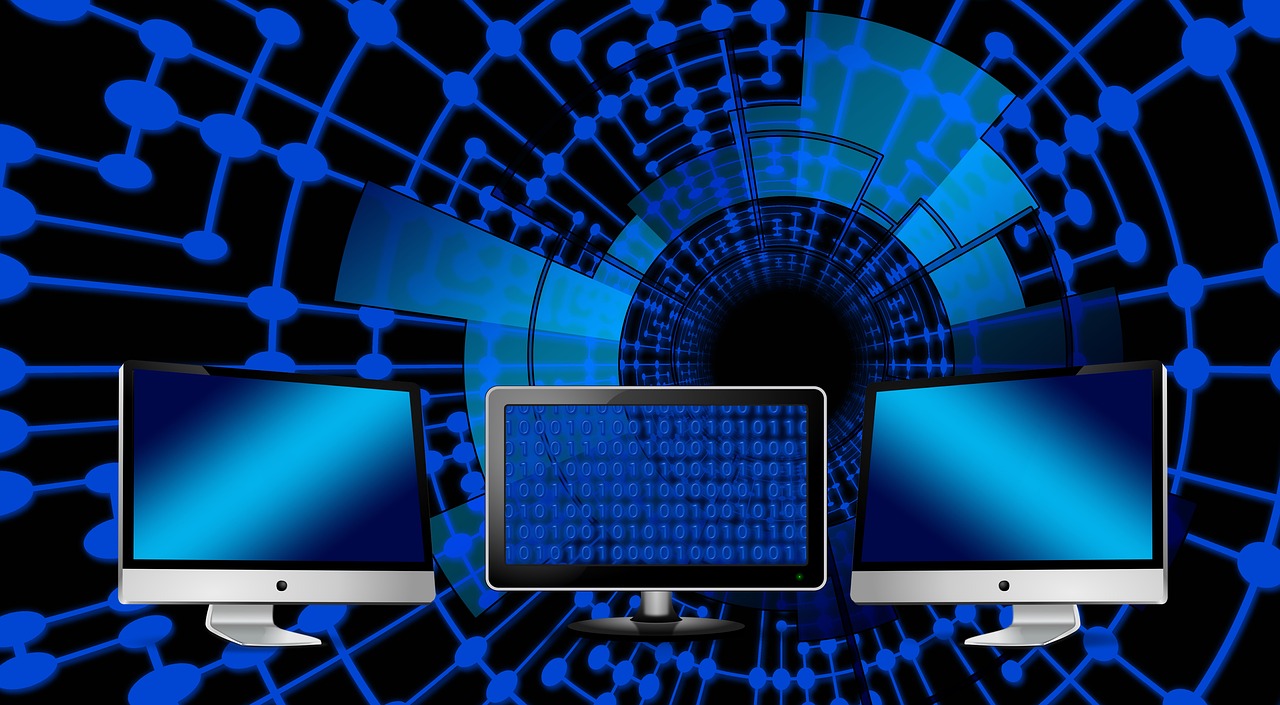
AI-Powered Data Collection Tools
In today's fast-paced world, the need for efficient and accurate data collection has never been more crucial. Enter , which are revolutionizing how organizations gather and analyze information. These innovative tools are designed to streamline the data collection process, enhance data quality, and ultimately improve decision-making across various sectors. Imagine having a personal assistant that not only gathers information but also analyzes it in real time—this is precisely what AI tools offer.
One of the most notable advancements in this field is the use of automated surveys. Traditional surveys can be time-consuming and prone to bias, but with AI, organizations can create dynamic surveys that adapt based on respondent feedback. This means that the questions can change in real time, providing more relevant data and improving the overall response rate. Additionally, AI algorithms can analyze responses on the fly, identifying trends and patterns that would take humans much longer to uncover.
Another exciting tool in the AI arsenal is the chatbot. These intelligent virtual assistants engage with users in natural language, making data collection feel more conversational. Whether it's gathering customer feedback or conducting market research, chatbots can interact with a large number of respondents simultaneously, ensuring that no valuable insights are missed. They can even be programmed to ask follow-up questions based on previous answers, further enriching the data collected.
Moreover, data scraping software has taken a giant leap forward thanks to AI. This technology allows organizations to extract vast amounts of data from various online sources quickly and efficiently. For instance, a company might want to analyze customer sentiment across social media platforms. AI-driven scraping tools can gather this data, filtering out noise and focusing on relevant conversations, which can then be analyzed for actionable insights.
To illustrate the effectiveness of these AI-powered tools, consider the following table that highlights some key features and benefits:
Tool | Features | Benefits |
---|---|---|
Automated Surveys | Dynamic questioning, real-time analysis | Higher response rates, relevant data |
Chatbots | Natural language processing, multi-user engagement | Increased interaction, deeper insights |
Data Scraping Software | Rapid data extraction, filtering capabilities | Comprehensive analysis, time-saving |
In summary, AI-powered data collection tools are not just enhancing traditional methods; they are transforming how organizations approach data gathering. With features that promote efficiency and accuracy, these tools enable businesses to harness the power of data like never before. As we continue to explore the capabilities of AI, the potential for innovation in data collection is boundless.
- What are AI-powered data collection tools? These are advanced technologies that utilize artificial intelligence to gather, analyze, and interpret data more efficiently than traditional methods.
- How do automated surveys work? Automated surveys adapt in real time based on respondent answers, allowing for more relevant data collection.
- Can chatbots improve response rates? Yes, chatbots engage users in a conversational manner, making it easier for them to provide feedback and insights.
- What is data scraping? Data scraping is the process of extracting data from websites and online sources, often used for market analysis and sentiment tracking.

Benefits of AI in Data Accuracy
In today's fast-paced digital landscape, where data drives decisions, the accuracy of that data is paramount. Artificial Intelligence (AI) has emerged as a game-changer in enhancing data accuracy, enabling organizations to make informed choices that can significantly impact their success. Imagine trying to navigate a ship without a compass; that's what decision-making feels like without accurate data. With AI, organizations can not only improve the precision of their data but also enhance the reliability of their insights.
One of the primary ways AI contributes to data accuracy is by reducing human errors. Traditional data collection methods often rely on manual input, which is prone to mistakes. AI algorithms, on the other hand, automate the data entry process, minimizing the risk of errors that can arise from fatigue or oversight. For instance, when using AI-driven tools, data is collected in real-time, ensuring that the information is not only accurate but also up-to-date. This is akin to having a personal assistant who never forgets a detail and always keeps you informed.
Moreover, AI excels in validating information. By cross-referencing data from multiple sources, AI systems can identify discrepancies and inconsistencies that might go unnoticed by human analysts. This capability is crucial for organizations that rely on large datasets for analysis. For example, if a company is analyzing customer feedback from various platforms, AI can quickly spot conflicting information and highlight areas that require further investigation. This level of scrutiny ensures that the insights drawn from the data are both trustworthy and actionable.
Additionally, the use of advanced analytics powered by AI allows organizations to sift through vast amounts of data efficiently. With techniques like machine learning, AI can uncover patterns and trends that would be impossible to detect manually. This process not only enhances data accuracy but also provides deeper insights into customer behaviors and market dynamics. Picture a detective piecing together clues; AI serves as the magnifying glass that brings hidden details to light.
Machine learning (ML) plays a pivotal role in enhancing data accuracy through robust validation processes. By continuously learning from new data, ML algorithms can identify and correct inconsistencies automatically. For example, if a dataset includes customer names with various spellings, an ML model can learn to recognize these variations and standardize them. This process ensures that the dataset remains clean and reliable for further analysis. As a result, organizations can trust that their data reflects reality, leading to better strategic decisions.
Another significant benefit of AI in data accuracy is the ability for real-time monitoring. With traditional methods, data collection can be a slow and cumbersome process, leading to outdated information. AI changes the game by enabling organizations to monitor data as it is collected. This immediacy allows for timely adjustments and decisions based on current information. For instance, if a retail company notices a sudden spike in online purchases, AI can alert them to potential stock shortages, allowing them to react swiftly and maintain customer satisfaction.
Furthermore, AI-driven predictive analytics can forecast trends and behaviors, helping organizations gather relevant data proactively. By analyzing historical data, AI can identify patterns that suggest future outcomes. For example, a healthcare provider might use predictive analytics to anticipate patient admission rates, allowing them to allocate resources more effectively. This proactive approach not only enhances data accuracy but also supports strategic planning, ensuring that organizations are prepared for future challenges.
Lastly, the incorporation of Natural Language Processing (NLP) in survey design and analysis is revolutionizing how organizations understand respondent sentiments and intentions. NLP enables AI to interpret open-ended survey responses, extracting meaningful insights from unstructured data. Imagine reading through hundreds of survey responses; it can be overwhelming. However, with NLP, AI can summarize key themes and sentiments, providing organizations with a clearer picture of customer opinions and preferences.
In conclusion, the benefits of AI in enhancing data accuracy are profound. By reducing human errors, validating information, leveraging advanced analytics, and utilizing real-time monitoring, organizations can significantly improve the reliability of their data. As we move forward in an increasingly data-driven world, embracing AI will be crucial for those looking to make informed, strategic decisions.
- How does AI reduce human errors in data collection? AI automates data entry and processing, minimizing the chances of mistakes that can occur with manual input.
- What role does machine learning play in data validation? Machine learning algorithms continuously learn from new data, enabling them to identify and correct inconsistencies automatically.
- Can AI help in real-time data monitoring? Yes, AI enables organizations to monitor data as it is collected, allowing for timely adjustments based on current information.
- What is predictive analytics and how does it relate to data accuracy? Predictive analytics uses historical data to forecast future trends, helping organizations gather relevant data proactively and enhance strategic planning.
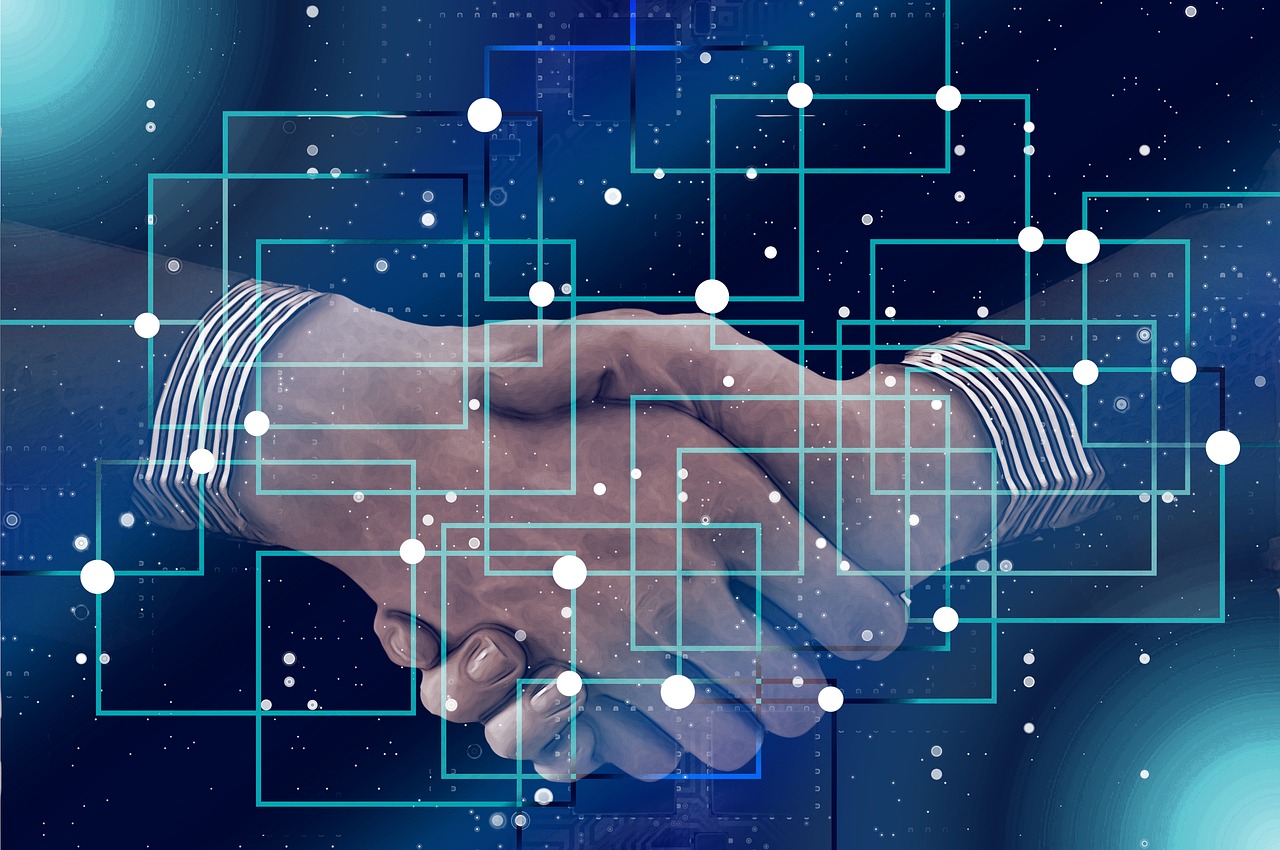
Machine Learning for Data Validation
In today's data-driven world, the integrity of information is paramount. Enter machine learning (ML), a game-changer in the realm of data validation. Imagine having a personal assistant who not only checks your work but also learns from your mistakes, becoming more efficient over time. That’s what ML does for data validation! By leveraging algorithms, it can automatically identify and rectify inconsistencies in datasets, ensuring that the information you rely on is as accurate as possible.
Machine learning algorithms analyze vast amounts of data to spot patterns and anomalies that might go unnoticed by human eyes. For instance, if a dataset contains sales figures from various regions, an ML model can quickly detect outliers—like an unusually high sales figure that doesn't match historical trends. This capability not only saves time but also significantly enhances the quality of the data being used for decision-making.
Moreover, the process of data validation becomes increasingly seamless as the machine learning model continues to learn from new data inputs. This means that over time, the model becomes better at predicting what constitutes valid data based on historical patterns. To illustrate this, consider the following table that outlines the stages of machine learning in data validation:
Stage | Description |
---|---|
Data Collection | Gathering datasets from various sources for analysis. |
Data Preprocessing | Cleaning and organizing data to prepare for analysis. |
Model Training | Using historical data to teach the algorithm how to identify valid data. |
Validation | Testing the model against new data to ensure accuracy. |
Continuous Learning | Updating the model with new data to improve its accuracy over time. |
As organizations increasingly rely on data for strategic planning, the need for accurate datasets becomes crucial. By implementing machine learning for data validation, companies can not only enhance the reliability of their information but also streamline their operational processes. Imagine the time saved when data validation is automated—teams can focus on analysis and decision-making rather than getting bogged down in data cleaning!
In conclusion, machine learning is not just a buzzword; it’s a transformative approach to ensuring data integrity. By harnessing its power, organizations can elevate their data validation processes, leading to more informed decisions and ultimately, a competitive edge in their respective markets.
- What is machine learning? Machine learning is a subset of artificial intelligence that enables systems to learn from data and improve their performance over time without being explicitly programmed.
- How does machine learning improve data validation? It automates the process of identifying and correcting inconsistencies in data, leading to higher quality datasets for analysis.
- Can machine learning models adapt to new data? Yes, machine learning models continuously learn from new data inputs, improving their accuracy and reliability over time.
- What are the benefits of using machine learning for data validation? The benefits include increased accuracy, reduced human error, and the ability to process large datasets quickly and efficiently.
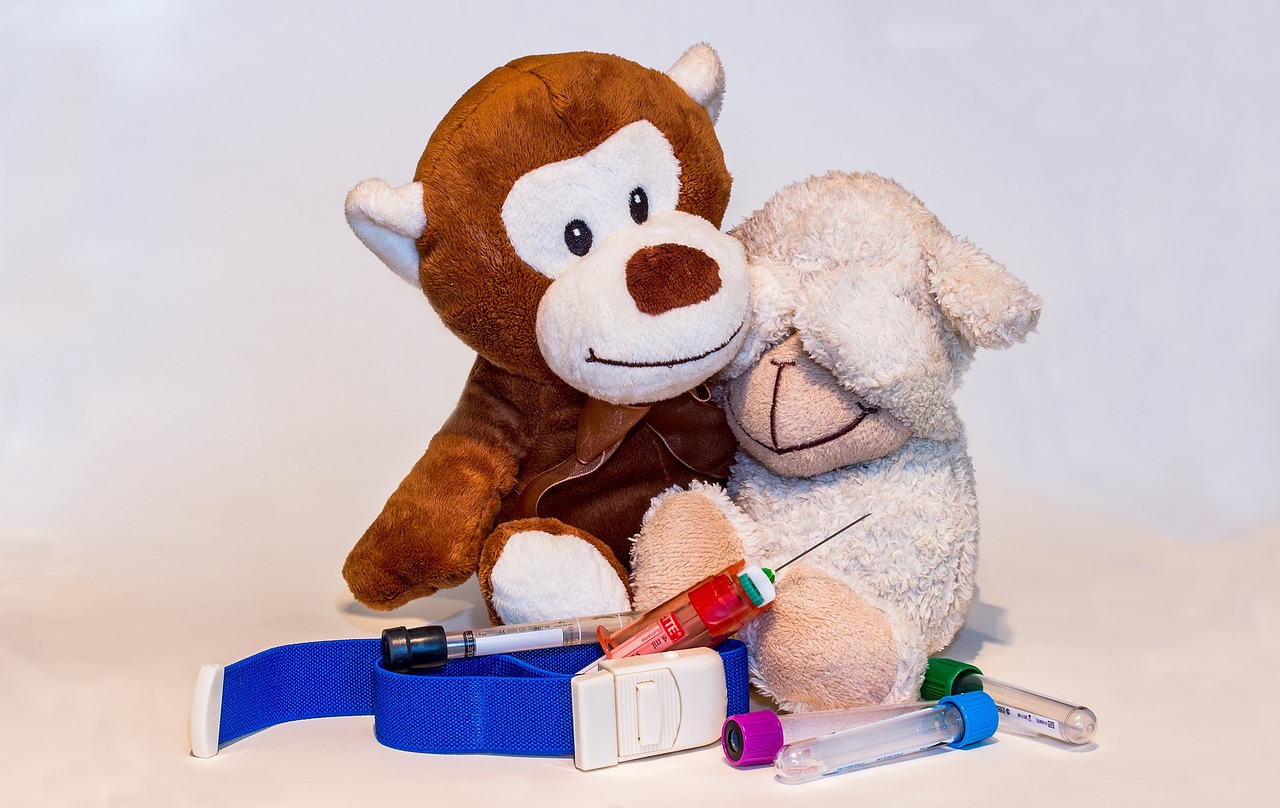
Real-Time Data Monitoring
In today's fast-paced world, the ability to monitor data in real-time has become a game-changer for organizations across various sectors. Imagine a ship sailing through turbulent waters; without a compass, steering becomes a daunting task. Similarly, real-time data monitoring acts as that compass, guiding businesses through the complexities of decision-making. With the advent of artificial intelligence, organizations can now harness the power of real-time insights to navigate their operational challenges effectively.
Real-time data monitoring allows businesses to collect and analyze data as it happens, rather than relying on outdated reports that may no longer reflect the current situation. This immediacy is crucial for making informed decisions quickly. For instance, consider a retail company that uses AI to track customer purchasing behavior. By analyzing data in real-time, they can identify trends, adjust inventory levels, and even tailor marketing strategies to meet customer demands instantaneously. This level of responsiveness not only enhances customer satisfaction but also boosts sales and operational efficiency.
Furthermore, real-time monitoring can significantly reduce risks associated with data collection. Imagine a healthcare provider that uses AI to monitor patient vitals continuously. If any abnormalities are detected, immediate alerts can be generated, allowing medical staff to respond swiftly. This capability can be the difference between life and death, showcasing just how critical real-time data monitoring can be in sectors where every second counts.
To illustrate the impact of real-time data monitoring, consider the following table that highlights its benefits across different industries:
Industry | Benefit of Real-Time Monitoring |
---|---|
Healthcare | Immediate alerts for patient care and treatment adjustments. |
Retail | Dynamic inventory management and enhanced customer experience. |
Finance | Real-time fraud detection and risk management. |
Manufacturing | Optimization of production processes and reduction of downtime. |
However, implementing real-time data monitoring does come with its set of challenges. Organizations need to invest in robust infrastructure and ensure that the data being collected is accurate and relevant. Additionally, they must address potential data privacy concerns, ensuring that sensitive information is protected while still allowing for effective monitoring. But the rewards far outweigh the challenges, as the ability to make data-driven decisions in real-time can lead to significant competitive advantages.
In conclusion, real-time data monitoring powered by AI is not just a luxury but a necessity for organizations aiming to thrive in today's competitive landscape. It empowers businesses to make swift, informed decisions, enhances operational efficiency, and ultimately drives success. As we continue to advance technologically, the importance of real-time data monitoring will only grow, paving the way for smarter, more agile organizations.
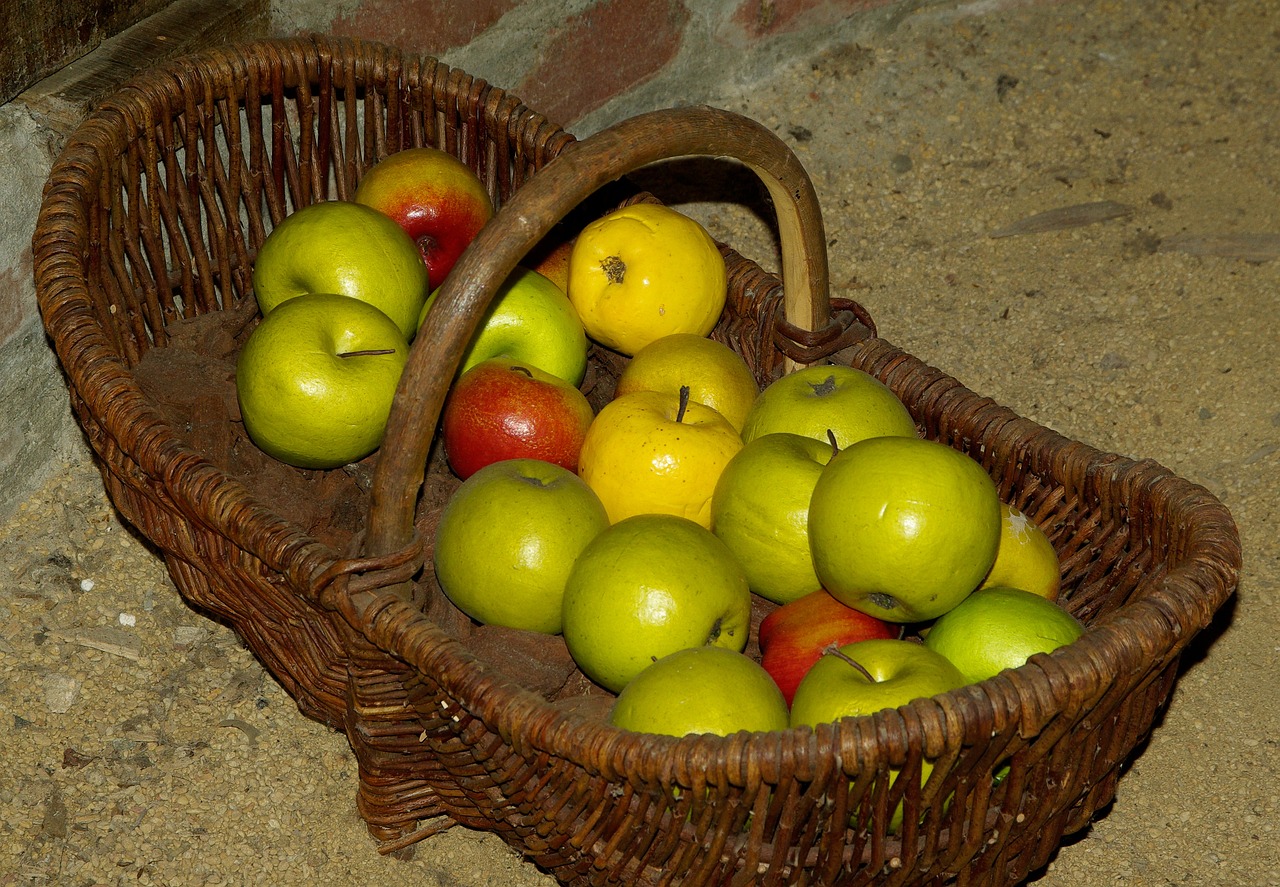
Predictive Analytics
Predictive analytics is like having a crystal ball that helps organizations foresee future trends and behaviors based on historical data. By utilizing sophisticated AI algorithms and statistical techniques, businesses can analyze past patterns to make informed decisions about the future. Imagine being able to anticipate customer needs before they even express them! This capability not only enhances customer satisfaction but also optimizes resource allocation, ultimately leading to increased profitability.
At the core of predictive analytics lies the concept of data modeling. Organizations gather vast amounts of data from various sources, such as sales records, customer interactions, and market trends. By feeding this data into machine learning models, they can identify correlations and patterns that might not be immediately obvious. For instance, a retail company can analyze purchasing habits to predict which products are likely to be in high demand during specific seasons. This proactive approach allows them to stock up on inventory, ensuring they meet customer expectations without overextending their resources.
One of the most exciting aspects of predictive analytics is its ability to enhance strategic planning. With accurate forecasts, companies can make data-driven decisions that align with their long-term goals. For example, a tech startup can use predictive analytics to identify the best time to launch a new product based on market readiness and competitive landscape. This not only minimizes risks but also maximizes the chances of success. Furthermore, predictive insights can inform marketing strategies, helping organizations tailor their campaigns to resonate with their target audience.
However, it's essential to note that predictive analytics is not without its challenges. The quality of predictions heavily relies on the quality of the input data. Inaccurate or incomplete data can lead to misleading forecasts, which can be detrimental to business strategies. Therefore, organizations must invest in robust data collection methods and data cleansing processes to ensure the integrity of their predictive models.
In summary, predictive analytics transforms the way organizations approach data collection and decision-making. By harnessing the power of AI, businesses can not only anticipate future trends but also create strategies that are agile and responsive to changing market dynamics. As we move forward, the integration of predictive analytics into everyday business practices will undoubtedly become a crucial component of successful strategic planning.
- What is predictive analytics? Predictive analytics uses historical data and AI algorithms to forecast future events and trends.
- How can predictive analytics benefit my business? It can help you anticipate customer needs, optimize resource allocation, and enhance strategic planning.
- What challenges are associated with predictive analytics? Challenges include data quality, the need for robust data collection methods, and the potential for misleading forecasts if the input data is inaccurate.

Natural Language Processing in Surveys
Natural Language Processing (NLP) is a game changer in the world of surveys, and it’s not just a fancy tech term to throw around. Imagine being able to understand the subtleties of human language—like sarcasm, idioms, and emotional tones—without needing a human to interpret it. That's precisely what NLP brings to the table! By leveraging NLP, organizations can design surveys that are not only more engaging but also yield richer insights from the responses.
One of the most exciting aspects of NLP in surveys is its ability to analyze open-ended responses. Traditional surveys often limit respondents to predefined options, which can lead to a lack of depth in the data collected. However, with NLP, organizations can allow respondents to express their thoughts freely, and the technology can automatically categorize and analyze these responses. This means that instead of just knowing how many people liked a product, you can understand why they liked it, what they specifically appreciated, and even what improvements they suggest.
Moreover, NLP enhances the survey experience for respondents. Have you ever filled out a survey and felt like you were just clicking buttons without really expressing your opinion? With NLP, surveys can be more conversational and intuitive. For instance, chatbots powered by NLP can guide respondents through the survey, asking follow-up questions based on their answers. This not only keeps the respondent engaged but also ensures that the data collected is more relevant and insightful.
Let's look at some key benefits of implementing NLP in surveys:
- Improved Understanding: NLP can decode complex language patterns, allowing organizations to grasp the true sentiment behind responses.
- Enhanced Data Quality: By analyzing open-ended responses, NLP helps in identifying trends and themes that might be missed with closed-ended questions.
- Real-Time Insights: Organizations can receive immediate feedback and insights, enabling quicker decision-making.
However, the integration of NLP in surveys is not without its challenges. For example, the accuracy of NLP algorithms can vary based on the language used and the context of the responses. Misinterpretation of sarcasm or cultural references can lead to skewed data. Therefore, organizations must continuously refine their NLP models to ensure they are accurately capturing the nuances of human language.
In summary, the role of Natural Language Processing in surveys is transforming how organizations gather and interpret data. By enabling more natural interactions and deeper insights, NLP not only improves the quality of data collected but also enhances the overall respondent experience. As we move forward, it will be fascinating to see how this technology evolves and further shapes the landscape of data collection.
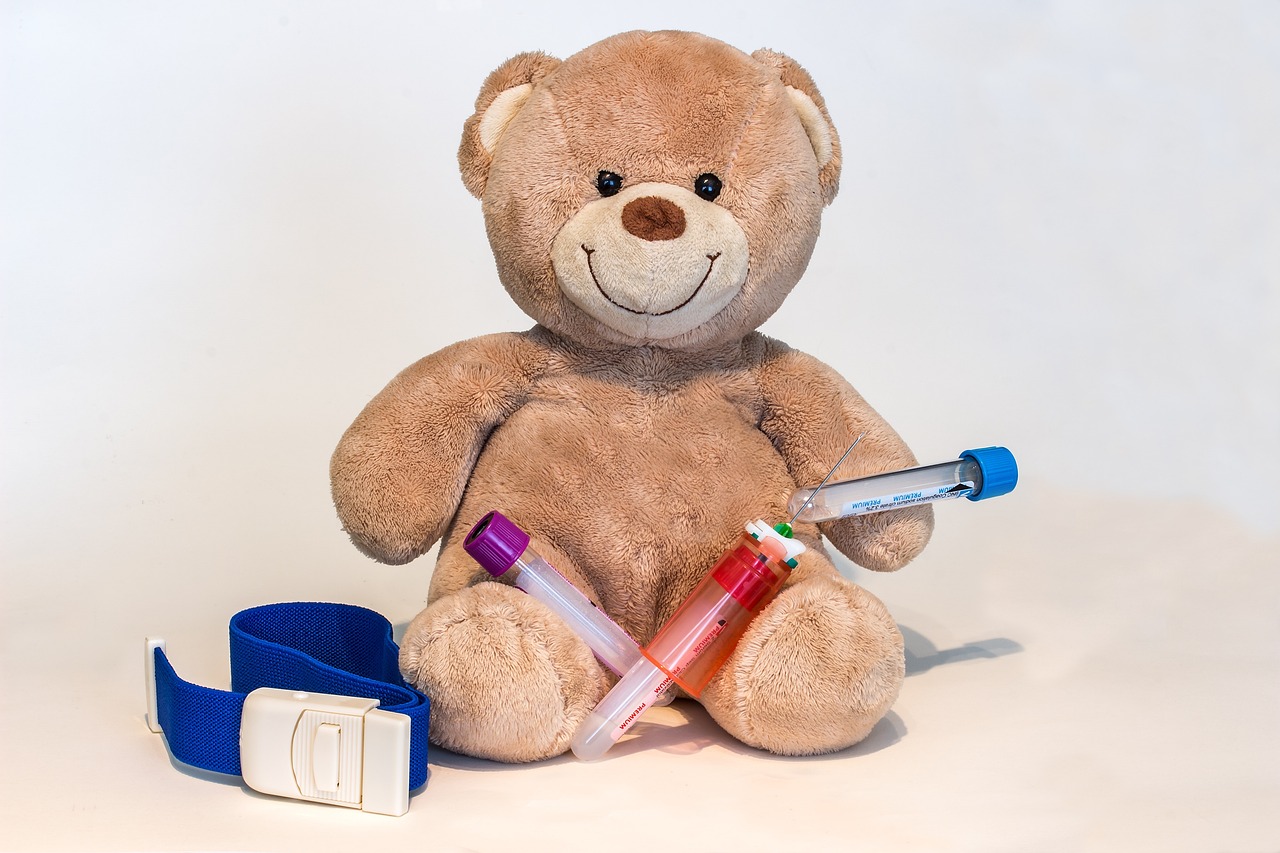
Challenges of Implementing AI in Data Collection
While the integration of artificial intelligence (AI) into data collection methods presents numerous advantages, it is not without its challenges. Organizations often find themselves navigating a complex landscape filled with potential pitfalls. One of the most significant hurdles is the cost associated with implementing AI technologies. Initial investments can be substantial, particularly for small to medium-sized enterprises. This includes expenses for software, hardware, and the necessary training for staff to effectively utilize these tools.
Moreover, the complexity of AI systems can be daunting. Many organizations may lack the technical expertise required to deploy and maintain these systems effectively. This complexity can lead to implementation delays, increased costs, and even project failures. It's crucial for businesses to ensure they have the right talent in place or to seek external partnerships to bridge this gap.
Another pressing concern is data privacy and security. As organizations collect more data, the risk of data breaches and misuse increases. With regulations such as the General Data Protection Regulation (GDPR) in Europe, companies must be vigilant about how they handle sensitive information. Failing to comply with these regulations can result in hefty fines and damage to reputation.
To mitigate these risks, organizations should consider the following strategies:
- Conducting thorough risk assessments before implementing AI solutions.
- Investing in robust cybersecurity measures to protect collected data.
- Ensuring transparency in data collection processes to build trust with consumers.
Additionally, the ethical considerations surrounding AI usage cannot be overlooked. As AI systems become more prevalent, organizations must grapple with the implications of bias in algorithms and the potential for discriminatory practices. Ensuring that AI is used responsibly and ethically is essential for maintaining public trust and avoiding legal repercussions.
Lastly, organizations need to be prepared for ongoing maintenance and updates. AI technologies are constantly evolving, and keeping systems up-to-date can require continuous investment and attention. This not only includes software updates but also retraining staff and adapting processes to leverage new capabilities.
In summary, while the benefits of AI in data collection are substantial, organizations must carefully navigate the challenges of cost, complexity, data privacy, ethical implications, and maintenance. By addressing these issues head-on, businesses can position themselves to harness the full potential of AI, driving innovation and improving decision-making processes.
Q1: What are the main challenges of implementing AI in data collection?
A1: The primary challenges include high costs, complexity of AI systems, data privacy and security issues, ethical considerations, and the need for ongoing maintenance.
Q2: How can organizations mitigate data privacy risks when using AI?
A2: Organizations can mitigate these risks by conducting thorough risk assessments, investing in cybersecurity measures, and ensuring transparency in their data collection processes.
Q3: Why is it important to address ethical considerations in AI?
A3: Addressing ethical considerations is crucial to prevent bias in AI algorithms, maintain public trust, and comply with legal standards.
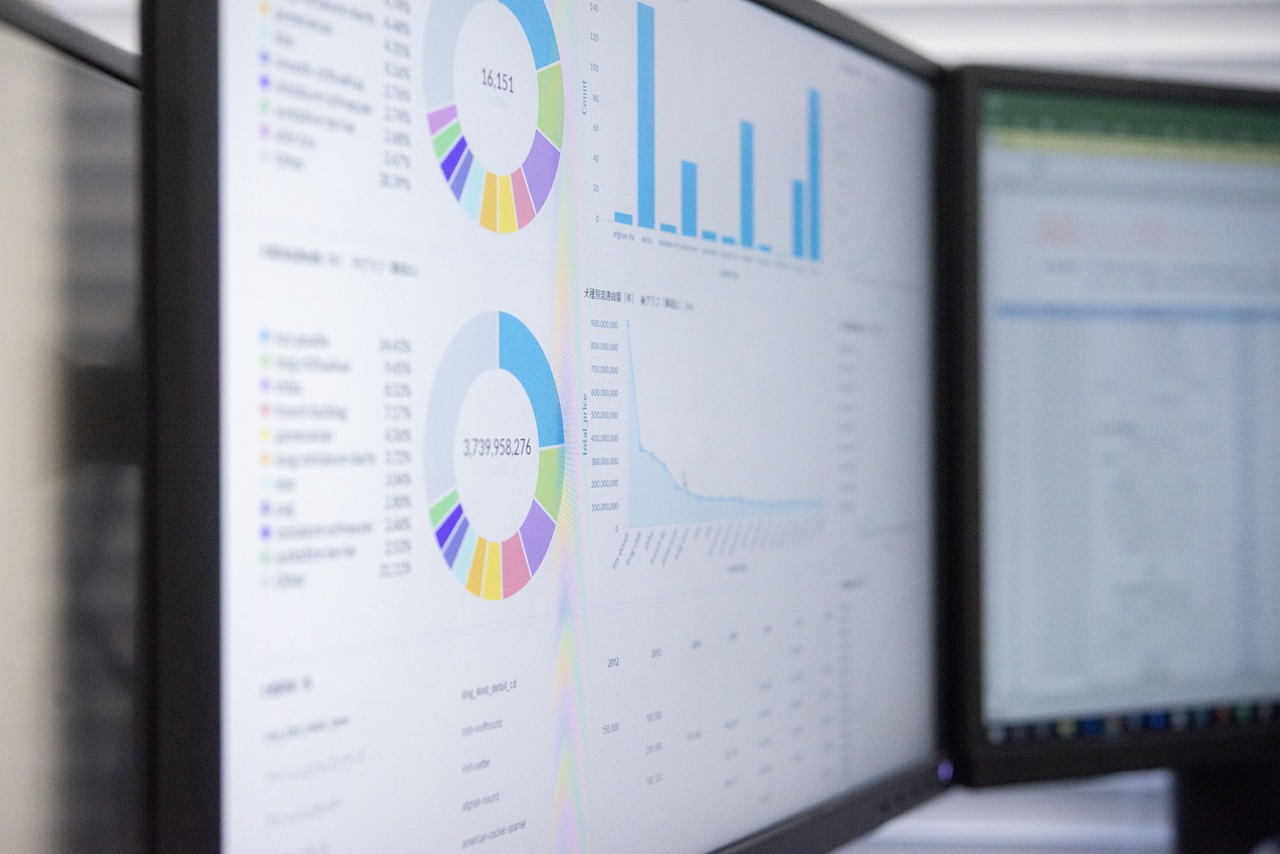
Data Privacy and Security Issues
As organizations increasingly integrate artificial intelligence into their data collection methods, the spotlight on data privacy and security issues becomes more pronounced. With AI tools capable of gathering vast amounts of sensitive information, it’s crucial to address how to protect this data from unauthorized access and breaches. Imagine a vault filled with your most valuable possessions, but instead of a lock and key, you have algorithms and protocols. How secure is that vault really? This is the question that organizations must grapple with.
One of the primary concerns is the potential for data breaches. When companies utilize AI-powered tools, they often collect personal data, including names, emails, and even financial information. If these systems are not adequately secured, hackers could exploit vulnerabilities, leading to significant risks. The repercussions can be severe, not just in terms of financial loss but also in damage to reputation and trust. A breach can feel like a betrayal of trust, akin to someone breaking into your home and rifling through your belongings.
To mitigate these risks, organizations must implement robust security measures. This includes employing advanced encryption techniques, which can be likened to a digital lock that prevents unauthorized access. Additionally, regular security audits should be conducted to identify and rectify potential weaknesses in the system. It's essential to stay one step ahead of cybercriminals, much like a chess player anticipating their opponent's moves.
Furthermore, organizations must be transparent about their data collection practices. This means clearly communicating to users what data is being collected, how it will be used, and who it will be shared with. Creating a clear privacy policy can help build trust with consumers, as they feel more secure knowing how their information is handled. In this digital age, transparency is not just a courtesy; it's a necessity.
Another critical aspect is compliance with data protection regulations, such as the General Data Protection Regulation (GDPR) in Europe or the California Consumer Privacy Act (CCPA) in the United States. These regulations impose strict guidelines on how organizations must handle personal data. Non-compliance can lead to hefty fines and legal challenges, making it imperative for companies to stay informed about the laws governing data privacy. Think of these regulations as the rules of the road; ignoring them can lead to serious consequences.
In conclusion, while AI offers remarkable advantages in data collection, it also brings significant challenges related to data privacy and security. Organizations must prioritize the protection of sensitive information by implementing strong security measures, maintaining transparency, and adhering to legal regulations. By doing so, they can harness the power of AI while safeguarding the trust of their users.
- What are the main risks associated with AI in data collection?
The primary risks include data breaches, unauthorized access, and non-compliance with privacy regulations. - How can organizations ensure data privacy when using AI?
Organizations can implement strong encryption, conduct regular security audits, and maintain transparency with users about data usage. - What are the implications of non-compliance with data protection regulations?
Non-compliance can lead to significant fines, legal challenges, and damage to an organization's reputation.
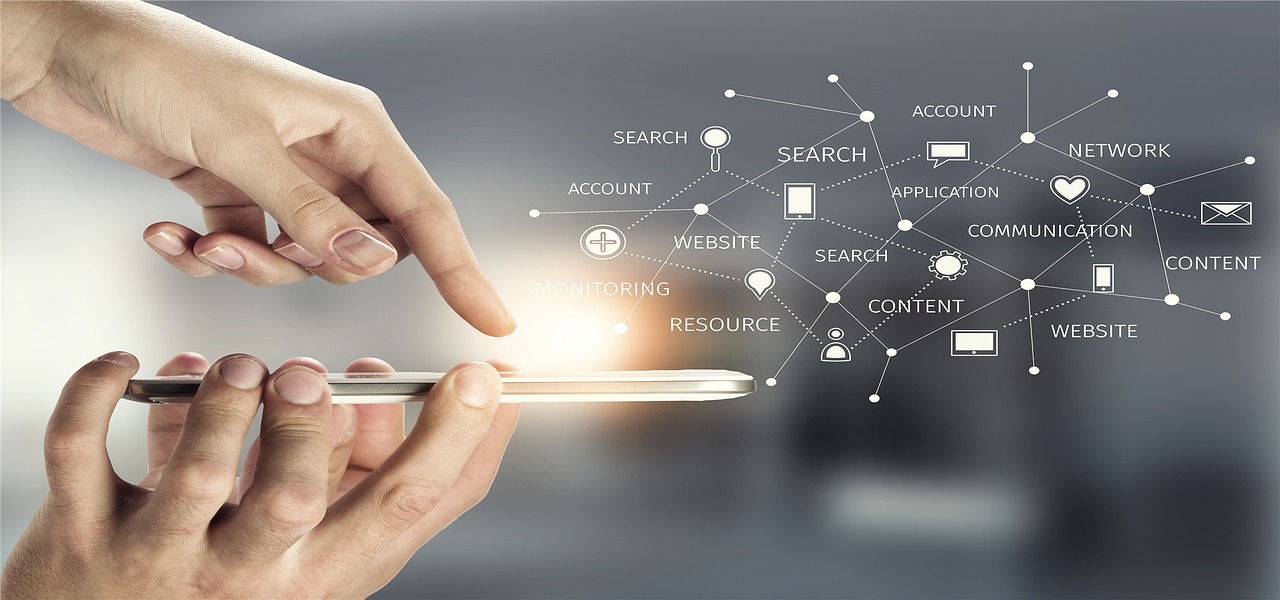
Cost Considerations
When it comes to integrating AI technologies into data collection processes, one of the most significant factors organizations must consider is the cost. While the benefits of AI can be transformative, the initial investment and ongoing expenses can be daunting. Understanding these costs is crucial for any business looking to enhance its data collection methods.
Initially, organizations may face substantial upfront costs associated with purchasing AI software and hardware. These costs can vary widely based on the complexity of the system and the scale at which it will be deployed. For instance, a small business might spend a few thousand dollars on basic AI tools, while larger enterprises could invest hundreds of thousands or even millions in custom solutions.
Beyond the initial purchase, there are also maintenance costs to consider. AI systems require regular updates and maintenance to function effectively. This could mean hiring specialized personnel or contracting with external vendors to ensure that the technology remains up-to-date and secure. The ongoing costs can include:
- Software Licensing Fees: Many AI tools come with annual licensing fees that can add up over time.
- Training Costs: Employees may need training to effectively use new AI systems, which can incur additional costs.
- Support Services: Relying on external support for troubleshooting and system enhancements can further increase expenses.
However, it’s essential to view these costs through a broader lens. While the initial outlay may seem high, the potential return on investment (ROI) can be substantial. By improving data accuracy and collection efficiency, organizations can save money in the long run. For example, consider a company that implements AI-driven data collection tools to reduce manual data entry errors. The reduction in errors can lead to significant savings in both time and resources, ultimately justifying the initial investment.
Moreover, the competitive advantage gained from utilizing AI can translate into increased revenue. Businesses that leverage AI for data collection can make more informed decisions, respond to market trends faster, and enhance customer experiences, all of which can lead to higher profits.
To summarize, while the costs associated with implementing AI in data collection can be substantial, they should be weighed against the potential benefits. Organizations must conduct thorough cost-benefit analyses to determine the viability of AI integration within their specific contexts. By doing so, they can ensure that they are making a sound investment that will pay dividends in the future.
Q: What are the primary costs associated with AI in data collection?
A: The primary costs include initial software and hardware purchases, maintenance and support fees, and potential training costs for employees.
Q: How can organizations measure the ROI of AI investments?
A: ROI can be measured by comparing the cost savings from improved efficiency and accuracy against the initial and ongoing costs of the AI systems.
Q: Are there hidden costs in implementing AI?
A: Yes, hidden costs can include unexpected maintenance fees, costs associated with data privacy compliance, and the need for additional resources to manage AI systems.

Future Trends in AI and Data Collection
The landscape of data collection is evolving at an unprecedented pace, primarily driven by advancements in artificial intelligence (AI). As organizations increasingly rely on data to inform their decisions, the integration of AI into data collection processes is not just a trend but a necessity. One of the most significant trends we are witnessing is the rise of increased automation. With AI, mundane tasks such as data entry and processing are being automated, freeing up valuable time for data analysts to focus on more strategic initiatives. Imagine a world where data is collected and processed in real-time, allowing businesses to pivot based on current market conditions!
Moreover, the future of data collection will see enhanced data analytics capabilities. AI algorithms are becoming more sophisticated, allowing for deeper insights and more nuanced understanding of data patterns. This means that organizations will not only collect data but will also be able to analyze it in ways that were previously unimaginable. For instance, predictive analytics powered by AI can forecast trends, helping businesses to anticipate market shifts and adjust their strategies accordingly. This proactive approach is akin to having a crystal ball that reveals not just what is happening now, but what is likely to happen in the future.
Another notable trend is the growing importance of ethical AI practices. As AI becomes more prevalent in data collection, concerns around data privacy and bias are coming to the forefront. Organizations will need to ensure that their AI systems are transparent and fair, maintaining the trust of their customers. The ethical use of AI in data collection will not only protect sensitive information but also enhance the overall quality of the data collected. Companies that prioritize ethical practices will likely gain a competitive edge, as consumers increasingly favor businesses that demonstrate social responsibility.
As we look ahead, we can expect the following key trends to shape the future of AI and data collection:
- Seamless Integration: AI tools will increasingly integrate with existing data systems, creating a more cohesive data ecosystem.
- Enhanced User Experience: AI-driven interfaces will make data collection more user-friendly, encouraging higher participation rates in surveys and feedback mechanisms.
- Real-Time Insights: Organizations will leverage AI to gain real-time insights, allowing for immediate decision-making based on the latest data.
In conclusion, the future of AI in data collection is bright and full of potential. With advancements in automation, analytics, and ethical practices, organizations are poised to harness the power of AI to not only enhance their data collection methods but also transform their overall decision-making processes. As we embrace these changes, it’s essential to remain vigilant about the ethical implications of AI, ensuring that we use this powerful technology responsibly and effectively.
Q1: How will AI change data collection methods in the next few years?
A1: AI will automate many traditional data collection tasks, enhance data accuracy through advanced analytics, and enable real-time insights, making the process faster and more efficient.
Q2: What are the ethical considerations when using AI for data collection?
A2: Organizations must ensure transparency, avoid biases in AI algorithms, and prioritize data privacy to maintain consumer trust and comply with regulations.
Q3: Can small businesses benefit from AI in data collection?
A3: Absolutely! AI tools are becoming more accessible and affordable, allowing small businesses to leverage data insights just like larger organizations.
Frequently Asked Questions
- What is the role of AI in data collection?
AI plays a crucial role in enhancing data collection by improving accuracy, efficiency, and insights. It automates various processes, allowing organizations to gather and analyze data more effectively than ever before.
- How do AI-powered tools improve data accuracy?
AI algorithms significantly reduce human errors and validate information through advanced analytics. This ensures that the data collected is reliable and of high quality, which is essential for informed decision-making.
- What are some examples of AI-powered data collection tools?
Examples include automated surveys, chatbots for customer interaction, and data scraping software that can extract large amounts of information from various sources quickly and accurately.
- Can machine learning help with data validation?
Absolutely! Machine learning techniques can automatically identify and correct inconsistencies in datasets. This leads to higher quality data, making it easier to analyze and derive insights.
- What is real-time data monitoring, and why is it important?
Real-time data monitoring allows organizations to track data as it is being collected. This capability enables timely adjustments and decisions based on current information, which can be critical for staying competitive.
- How does predictive analytics enhance strategic planning?
Predictive analytics uses AI to forecast trends and behaviors, helping organizations proactively gather relevant data. This foresight is invaluable for strategic planning and making informed business decisions.
- What challenges do organizations face when implementing AI in data collection?
Organizations may encounter challenges such as high costs, complexity in integration, and concerns over data privacy and security when adopting AI technologies for data collection.
- How can organizations address data privacy and security issues?
Maintaining data privacy and security is essential. Organizations can mitigate risks by implementing robust security protocols, ensuring compliance with regulations, and being transparent about data usage.
- What are the cost implications of adopting AI technologies?
The financial implications include initial investments in technology, ongoing maintenance costs, and potential returns on investment. It's crucial for organizations to evaluate these factors before making a decision.
- What future trends should we expect in AI and data collection?
Emerging trends include increased automation, enhanced data analytics capabilities, and a growing emphasis on ethical AI practices. These trends will shape how organizations collect and utilize data in the future.